ABSTRACT
The fraction of outdoor aerosol that penetrates into indoor environments plays an important role in determining the contribution of outdoor particles to the total lung dose of particles in human exposure. The objective of this study was to investigate the physical processes affecting migration of outdoor traffic particles into indoor environments. Particle number size distributions were measured by a fast mobility particle sizer system in both indoor and outdoor environments of a house located in close proximity to a busy street in Bologna (Italy) in the period February–April 2012. Indoor to outdoor (I/O) ratios for submicron particle number concentrations showed strong dependence on particle size and meteorological conditions. The loss rates of particles due to deposition, coagulation, and evaporation were determined using dynamic mass balance and coagulation models. Higher loss rates were found for small particles (nucleation and Aitken mode) indoors than for larger particles (accumulation mode). The coagulation and evaporation processes made a significant contribution to the loss of traffic nanoparticles indoors, especially during the day time. Application of positive matrix factorization to the indoor and outdoor particle size distributions showed a substantial loss of traffic-generated nucleation mode particles in the indoor environment, with evaporation playing a major role.
Copyright © 2017 American Association for Aerosol Research
EDITOR:
1. Introduction
Traffic-generated particles have been well-known as typically the most abundant particles in cities (Vu et al. Citation2015). Vu et al. (Citation2016) found that they represent approximately 56.5% of total particles by number at an urban background location in London, UK. Due to their high deposition efficiency, these particles easily penetrate into the deeper regions of the human lung; and as a consequence, they account for up to 71% of deposited ambient particle number in the alveolar region. In addition, growing evidence of their adverse effects on human health has been provided from both toxicological and epidemiological studies (Von Klot et al. Citation2011; de Kok et al. Citation2006; Salam et al. Citation2008). Recent studies have reported that traffic particles are linked to heart attack, strokes, mild cognitive impairment and wheezing illness, including asthma and lung function decline, especially in children and elderly people (Gent et al. Citation2009; Lepeule et al. Citation2014; Ranft et al. Citation2009; Suh and Zanobetti Citation2010). Therefore, traffic-generated particulate matter has been receiving much public attention.
People are exposed to traffic-generated particles through two routes: directly in the outdoor environment, or from indoor particles of outdoor traffic origin (such as in the home or office). As people spend the majority of their time (approximately 90%) indoors (Harrison et al. Citation2009), indoor particles of outdoor origin may contribute a significant fraction to the total lung dose of particles in human exposure. Moreover, some epidemiological studies show the importance of the investigation of indoor particulate matter (PM) of outdoor origin because of its influence on the relationship between exposure to PM and short-term mortality (Chen et al. Citation2012, Citation2013). For the above reasons, determination of the loss mechanisms of outdoor traffic aerosols which penetrate from outdoors into indoor environments and influence indoor exposure to traffic particles is highly relevant to the protection of public health.
When outdoor particles penetrate into indoor environments, they are controlled by three main factors: the penetration factor, the deposition rate and the air exchange rate (Hussein et al. Citation2006). The penetration and deposition processes of particles not only greatly depend upon the building characteristics, such as building envelope and surface area, but also on the characteristics of the particles, especially particle size (Hussein et al. Citation2006; Chen and Zhao 2011). Furthermore, other processes such as evaporation and coagulation could have significant effects on the particle number losses in a house in an area with busy traffic because of the high number concentration and volatile components (Zhu et al. Citation2005). Based on a dynamic mass balance model, numerous studies on indoor/outdoor modeling of particles have been conducted during the last decade, but there are limited modeling studies of indoor particle ingress from outdoors at heavily trafficked sites. Zhu et al. (Citation2005) conducted experiments in four two-bedroom apartments near the 405 Freeway in Los Angeles, CA, USA to investigate the penetration of freeway traffic ultrafine particles into indoor environments. The indoor/outdoor (I/O) ratios of particles, penetration and deposition rates in those studies were discussed, but other important processes affecting the loss of traffic particles such as coagulation or evaporation were not completely quantified.
To understanding better the behavior of traffic particles when they enter into indoor environments, we conducted measurements of both indoor and outdoor particle number size distributions in a house close to high traffic flows. The objective of this study was to investigate the indoor/outdoor (I/O) ratios of particle number and factors which determine these ratios. In addition, this study quantified the physical loss processes affecting the behavior of traffic particles in the indoor environment. Furthermore, we applied the Positive Matrix Factorization (PMF) model to estimate the loss of indoor particles of different outdoor origins.
2. Methods
2.1. Experimental design
The design is described by Zauli Sajani et al. (Citation2015). Particle number size distributions (PNSD) were measured by a fast mobility particle sizer (FMPS) system (model 3091, 5.6–560 nm; TSI Inc., Shoreview, MN, USA) in both the indoor and outdoor environments of a house in a busy traffic area of Bologna (Italy) in two sampling campaigns. The first sampling campaign was conducted from 22 February to 7 March and the second from 16 to 30 April. The indoor sampling room was on the ground floor in an unoccupied two story building which is close to a high traffic density street (31,000 vehicles/day) and near a two-way street canyon (Zauli Sajani et al. Citation2015). The sampling room was constructed with a marble floor (surface area of 16.9 m2), acrylic painted walls (total vertical surface area of 64.4 m2) and ceiling (ceiling height of 3.7 m). The FMPS spectrometer measured the size-resolved number concentration of particles every second. This FMPS was connected to a switching valve (Mod 11sc 200, Pneumoidraulica Engineering S.rl., Italy) to measure both indoor and outdoor size distribution every 10 min.
Air exchange rate was controlled by a mechanical system which consisted of an external fan connected to an air pipe (length = 1.2 m) to force the outdoor air into the center of the room (at a height of 2 m). In this way, the outdoor air forced into the room by the mechanical system caused a positive pressure indoors. Air could exit the room though a grid. The air inflow was measured using a TESTO 417 anemometer. In order to evaluate the loss of particles within the air injection system, an additional three-day experiment was performed to measure nearly contemporaneous particle size distributions at the inlet of the fan and the outlet of the pipe using an FMPS system. The detail of this experiment is given in the online supplementary information (SI). The test showed there was a very minor loss of particles in the air inflow system. Tapes on the door cracks were used to prevent air leaks. The fan was set at a suitable speed to obtain a stable of 0.5 h−1. The air exchange rate was also measured via CO2 decay data and from this system a similar value of
to that derived from the anemometer measurements was obtained.
The penetration factor (P) is defined as the fraction of particles in the infiltration air that enters indoors through the building envelope (Chen and Zhao 2011). In this study, the penetration of particles into the room was driven through the air pipe by a mechanical air exchange system. There was only a minor loss of particles in the air inlet system and the air leaks through cracks were minimized using tape. Therefore, the penetration factor with this novel experimental design in our study during was close to 1 (Figure S1, see the SI).
2.2. Data preparation
The FMPS system can measure particle size ranging from 5.6 to 560 nm; however, we did not include the size bins below 13 nm in our calculation because a high uncertainty in measurements of particles in these size bins was reported from previous studies (Jeong and Evans 2009; Kaminski et al. Citation2013). There were many cases of zero data in several of the largest particle size bins for unknown reasons in the second sampling campaign; thus we also did not include the size bins over 300 nm for this campaign. The switching valve allowed the FMPS to measure outdoor and indoor size distributions every 10 min; we therefore averaged the data from each 10 min period, apart from the 2 min samples at the beginning of each period, to avoid any mixing of outdoor and indoor air streams. The data were analyzed using R programing. We used the “Open-air” package developed by Carslaw and Ropkins (Citation2012) for polar plots and time average. Contour plots were performed using the Sigma Plot software, version 13.0. An indoor mass balance model and coagulation model were written in R by the author (Tuan V. Vu).
2.3. Indoor mass balance model
The influence of outdoor particles on the change of the concentration of indoor particles with a certain diameter has commonly been described using a mass balance model in previous studies in the following equation (Chen and Zhao Citation2011; Hussein et al. Citation2005).[1] where, Cin is the indoor particle concentration (particles/cm3) in each FMPS size bin; Cout is the outdoor particle concentration (particles/cm3) in each FMPS size bin; P is the penetration factor;
is the air exchange rate (h−1);
is the deposition rate (h−1) of particles with a certain diameter; S is the emission rate of particles (particles/h); V is volume of the room (cm3), and t is the time (h); Jother: particle production or loss rate due to other indoor processes such as coagulation, nucleation, condensation or evaporation (particles/cm3/h).
The flat was unoccupied and unfurnished during sampling campaigns; hence there was no indoor source (S = 0). We also assumed there was no particle formation indoors by chemical reactions, thus the other indoor processes term (Jother) in Equation (Equation1[1] ) is mainly controlled by coagulation and evaporation. We defined the terms kc and ke as the loss rates of indoor particles with a certain diameter due to coagulation and evaporation processes (h−1). The kc and ke take negative values if these processes produce particles. The mass balance model in Equation (Equation1
[1] ) was transformed to the following equation:
[2]
The total loss rate of particles with a certain diameter (kloss) is defined by the sum of the loss rate due to deposition, coagulation and evaporation processes: kloss = kd + kc + ke. Thus, Equation (Equation2[2] ) is converted to Equation (Equation3
[3] ):
[3]
To solve Equation (Equation3[3] ), the indoor concentration is modeled using a numerical backward difference for each time step of the specified period (Δt) (Chen and Zhao Citation2011):
[4]
In this study, the outdoor and indoor switching valve time was 10 min, thus the time step between two outdoor measurements (Δt) is 20 min (1/3 h). The penetration factor is approximately one based on our novel experimental design and the air exchange rate () was controlled at 0.5 h−1. Consequently, if the loss rate (kloss) of particles in a certain size bin and its outdoor number concentration are known, the indoor number concentration can be estimated from the observed outdoor concentration.
Conversely, to determine the total loss rate (kloss) of particles at a certain diameter, the kloss values (kloss > 0) were varied until the modeled Cin in Equation (Equation4[4] ) best fitted with observed Cin. To evaluate goodness-of-fit in the comparison of the model to the observed data set, we used two criteria: the root mean square error (RMSE) and the Pearson correlation coefficient (r) between indoor data sets estimated by the mass balance model and observed by measurement.
2.3.1. Coagulation model
To estimate the loss rate of indoor particles due to coagulation, the following equation (Müller Citation1928; Seinfeld and Pandis Citation2012) was utilized:[5] where, v-ṽ and ṽ are the volumes of two coagulating particles, v is the volume of the new particle due to coagulation, and N is the time-dependent number concentration (particles cm−3) of particles with a volume of v, v-ṽ or ṽ. βv-ṽ,ṽ is the coagulation rate coefficient (coagulation kernel) of the two colliding particles (cm3 particle−1s−1). All the collision kernel calculations were calculated in the R program, based on the equations given in previous work (Jacobson Citation1994; Alam Citation1987; Sceats Citation1989; Jacobson and Seinfeld Citation2004). The coagulation rates of two particles were calculated and are shown in Figure S2 (in the SI). The details of the model are given in the SI.
2.4. Positive matrix factorization (PMF) model
The PMF technique has been widely applied to particle number size distributions (PNSD) in order to identify the sources of particles (Vu et al. Citation2016). In this study PMF was applied to investigate the change of particle number size distributions when they enter into a house from outdoors, by application to both the indoor and outdoor particle size distributions together. A profile of 336 hourly indoor and outdoor PNSDs collected during the first sampling campaign were input into the US EPA PMF model version 5. The dataset collected during the second sampling campaign was not used in this model because of its very high uncertainties in the several last size bins. Details of the application of the PMF method to PNSD datasets are reported in previous studies (Vu et al. Citation2015, Citation2016).
3. Results and discussion
3.1. Indoor/Outdoor (I/O) ratios
3.1.1. Diurnal patterns of I/O ratios
shows the I/O ratios of submicron particles for two sampling campaigns (00:00–06:00; 06:00–12:00; 12:00–18:00; 18:00–24:00). In general, a small particle has a lower I/O ratio than a large one due to its higher loss rate by deposition and coagulation. The I/O ratios for ultrafine particles (<100 nm) ranged between 0.2–0.8, while those of accumulation mode particles (>100 nm) ranged from 0.4 to 0.8. These levels and trends of I/O ratio values are in agreement with previous studies for houses or apartments in the absence of indoor sources (Hussein et al. Citation2005, Citation2006; Long et al. Citation2001; Chao et al. Citation2003). For example, Hussein et al. (Citation2006) found I/O ratio values during a period without indoor sources in a house (naturally ventilated) in Prague, Czech Republic varied in the range between 0.4–0.8 for ultrafine particles and 0.7–0.8 for accumulation mode particles.
Figure 1. I/O ratio as a function of particle diameter at different times (00:00–06:00; 06:00–12:00; 12:00–18:00, and 18:00–24:00). The upper and lower dashed lines show the 95% confidence interval.
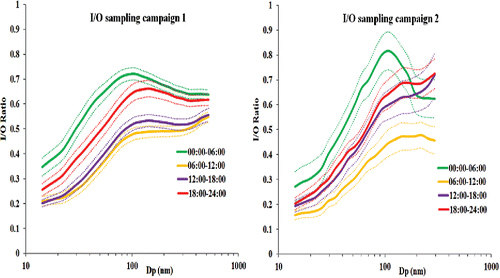
The I/O ratios at different times of day presented similar trends in the two campaigns, and were found to be lower in daytime, particularly during traffic rush hours (6:30–9:30 AM), than at nighttime (00:00–06:00). In addition, the I/O ratios were found to be higher at the weekend (Figure S3 in the SI). A higher loss rate of ultrafine particles during the daytime may be explained by the influence of coagulation and evaporation. The concentration of ultrafine particles in both outdoor and indoor environments during daytime was much higher than during nighttime. Because of the increased number concentration during daytime, the loss rate of indoor particles in this time period due to coagulation processes would be faster, leading to lower I/O ratios.
Particle volatility may also explain the loss of ultrafine particles during daytime. Kittelson (Citation1998) and Shi and Harrison (Citation1999) found that particles below 50 nm from traffic emissions consisted of a significant fraction of volatile components. When these particles enter a house, they can lose their volatile components by deposition and shrink in size, as they do in the atmosphere (Harrison et al. Citation2016). Zhu et al. (Citation2005) investigated the penetration of ultrafine particles into an unoccupied house near a freeway in Los Angeles, US. Their study found that the I/O ratio decreases when ultrafine particle size decreases to around 10–20 nm, but then increases again for particles below 10 nm.
3.1.2. Effects of meteorological parameters on the I/O ratios
Figure S4 (in the SI) shows the dependence of I/O ratios on wind speed and direction. I/O ratios, particularly for accumulation mode particles (Dp > 100 nm) increased when the wind speed increased. The effect of wind speed is to dilute the local traffic emissions which would then lead to higher I/O ratios. There was no clear influence of wind direction on the I/O ratios of small particles, but it had a significant effect on the I/O ratios of particles larger than 100 nm. Higher I/O ratios were identified from the SW wind direction. The reasons for this are unclear, but may relate to a different particle composition relating to this wind sector.
3.2. Total loss rates of indoor particles
A mass balance model based on Equation (Equation4[4] ) was applied to the PNSD datasets at different times (06:00–12:00; 12:00–18:00; 18:00–24:00) to estimate the loss rate (kloss) of particles. The kloss values varied with intervals of 0.01 between 0 and 10. The kd values were selected when the best fit (a minimum value of RMSE) between indoor concentrations modeled from Equation (Equation4
[4] ) and those measured by real observation was found. The performance of the mass balance model in predicting the total loss rate was tested by the Pearson correlation coefficient (r) value (Figure S5 in the SI). The results show that the mass balance model could perform very well in the prediction of nucleation mode particles during the day time. For example, the correlation coefficient (r) between the modeled and measured concentration of particles of 14.3 nm for the dataset from 6:00–12:00 was higher than 0.82 for the first sampling campaign and 0.86 for the second sampling campaign.
shows the total loss rate (kloss) of particles in different time periods. A higher loss rate was found in the period 06:00–12:00 than at nighttime, suggesting that coagulation and/or evaporation play very important roles in the loss of particles during the daytime in a house heavily affected by road traffic emissions. As shown in Figure S6 (see the SI), the particle number concentration for both outdoor and indoor environments during the daytime was much higher than that during the nighttime, resulting in a higher particle loss due to the coagulation process.
Figure 2. Total loss rates (kloss) of indoor particles in different time periods: (a) campaign 1; (b) campaign 2.
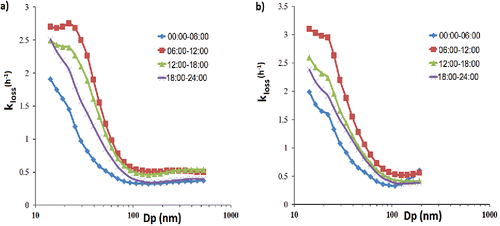
This result is in agreement with the higher I/O ratio at nighttime that was discussed in Section 3.1. The loss rate for a particle strongly depends upon its size. The total loss rate of a particle increases exponentially when its size decreases from 100 nm to 22.5 nm. This is due to the greater increase in coagulation and evaporation effects on small particles (the Aitken mode particles) than large particles (accumulation mode particles).
The total loss rate of particles increased slightly or was unchanged when their sizes decreased to lower than 22.5 nm. Evaporation is probably a major reason for this. Previous studies have reported an important role of nanoparticle evaporation in the evolution of particle size distribution at sites downwind of a road (Jacobson et al. Citation2005; Zhang et al. Citation2004; Dall'Osto et al. Citation2011). Kuhn et al. (Citation2005) found that traffic particles in the size range 20–40 nm consist of many semi-volatile components. They may become particles of 20 nm or less due to the evaporation of volatile components (Harrison et al. Citation2016). Therefore, the loss of particles of 20 nm or less did not increase due to the production of these particles by the evaporation of larger ones.
3.3. Estimation of the contribution of deposition, coagulation and evaporation processes to the total loss rate of indoor particles
To estimate the contribution of each process, the following assumptions were made:
• | The contribution of evaporation at nighttime (00:00–06:00) is very small, and ke = 0. As seen in Figure S6 (see the SI), the concentration of particles at nighttime is much lower than that in daytime due to reduced traffic emissions. Therefore, we assumed that the evaporation of the traffic generated particles at nighttime has a negligible effect on the particle population in order to calculate the deposition rate at nighttime. | ||||
• | The processes are considered to act independently. Although coagulation can be enhanced by evaporation and deposition (Jacobson et al. Citation2005; Rim et al. Citation2012), it is a function only of the number density, coagulation kernel and size distribution. To a first approximation it is reasonable to treat the processes as independent. | ||||
• | The deposition (h−1) rate of indoor particles is constant during different sampling time periods. The particle diffusivity is the main determinant of deposition efficiency in the sub-micrometer size range. This is a function of particle size and hence time-dependent for a given size range. |
The coagulation rate of indoor particles in different time periods was calculated by applying the coagulation model to the indoor particle size distributions and the total loss rate was estimated by the mass balance model as shown in Section 2.2. Therefore, the deposition rate can be estimated by using the nighttime data sets (00:00–06:00): At nighttime, kd = kloss − kc due to ke = 0. For other specific time periods: ke = kloss − (kd + kc).
3.3.1. Loss rates of indoor particles due to coagulation
A coagulation model was applied to the indoor particle size distributions to estimate the loss/gain of particles due to coagulation only during a 20 min period (a time step in our mass balance model is 20 min). presents the coagulation loss/gain rate (kc) of indoor particles with a certain diameter in different sampling time periods (). The loss rate of particles due to coagulation only showed strong dependence on their sizes. Smaller particles have higher coagulation loss rates than larger ones. There was not much difference of the coagulation loss rate curves of indoor particles between the time periods of 06:00–12:00, 12:00–18:00, and 18:00–24:00 because of their similar indoor particle number size distribution during these periods (Figure S6 in the SI). The coagulation loss rate of particles during nighttime (00:00–06:00) was found to be much lower than during other periods due to the lower concentration of smaller particles.
Figure 3. Loss rate, kc (h−1) of indoor particles due to coagulation only for different sampling time periods.
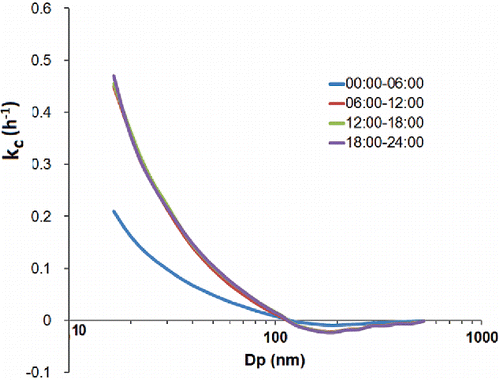
The loss/gain of particle concentration for a particle with a specific size is shown in Figure S7 (see the SI). Because the particle size distributions of indoor particles are more stable than particle number concentration, the loss or gain of indoor particles with a certain diameter depends primarily on their concentration. It was also found that the influence of coagulation on the loss or gain of particles increases when the total number concentration increases. For example, particles of 22.1 nm may lose a maximum of 4.3% of their concentrations due to coagulation if the total number concentration is lower than 5000 particles/cm3. When total particle number increases to 15,000 particles/cm3, more than 15% of those particles could be lost by coagulation.
3.3.2. Deposition loss rates of indoor particles
The deposition rate (kd) was estimated based on the application of the mass balance model to nighttime data sets (00:00–06:00): kd = kloss at nighttime − kc at nighttime.
The estimated particle deposition rates are shown in Figure S8 (see the SI). They ranged from 0.3 to 1.97 h−1, closely depending upon particle size. For submicron particles, a smaller particle has a higher deposition rate. The deposition rate of a particle with a diameter of 30 nm is three times higher than that of a particle with a diameter of 100 nm. This finding is consistent with previous studies (Hussein et al. Citation2006; Long et al. Citation2001; Chao et al. Citation2003). In addition, we compare this deposition rate calculated by a mass balance model with those calculated by theoretical models developed by Lai and Nazaroff (Citation2000). For particles with a diameter smaller than 150 nm, our estimated deposition rate fits very well with the result from models with an assumption of friction velocity (u*) ranging from 35 to 40 cm s−1. For larger particles, the deposition rate was found to be much higher than the Lai and Nazaroff (Citation2000) deposition rate curve. This may be due to their assumption of a perfectly smooth surface, which has a marked effect on the deposition of accumulation mode particles (Lai and Nazaroff Citation2000). Zhao and Wu (Citation2007) found that the wall roughness has significant effects on the particle deposition by enhancing the particle concentration gradient near the wall.
3.3.3. Evaporation loss rates of indoor particles
As discussed above, we assumed the contribution of evaporation processes to the total loss of indoor particles during the nighttime (00:00–06:00) was insignificant. For a specific sampling time period (06:00–12:00; 12:00–18:00, and 18:00–24:00): ke = kloss − (kd + kc).
Values of kloss, kd, and for kc particles with a certain diameter in different sampling time periods were estimated above, and therefore ke was estimated and is shown in . This shows a significant loss of particles in the sub-100 nm size range by evaporation. The modes in the curves in are at 29 nm, which is very typical of fresh traffic exhaust (Charron and Harrison Citation2003). Research in the atmosphere has shown a loss of such particles by evaporation as air is advected into an urban park (Harrison et al. Citation2016).
3.3.4. Uncertainty calculation
The uncertainty in the penetration factor was estimated from the measurements of upstream and downstream particle number size distributions in the pumped air inlet system. Due to the larger effects of diffusion, small particles in the nucleation range show larger uncertainty. It was found that the relative standard error of penetration factor for particles smaller than 20 nm can up to 18.1%, while that for particles with diameter larger than 52.3 nm is less than 5%. The uncertainty in the air exchange rate was estimated to be lower than 3% due to the well-controlled fan velocity. As can be seen from Equation (Equation3[3] ), the uncertainty in the total loss rate derives from the errors in the penetration factor, the particle number concentration and the air exchange rate. Unfortunately, there was no comprehensive evaluation of the uncertainty in the particle number concentration measured by the FMPS, and therefore the relative standard error of kloss was estimated based on the relative standard error of the penetration factor and the air exchange rate. The uncertainty in the coagulation loss rate derives from the error in the particle number size distribution and the coagulation rate coefficient. In our study, the loss rate due to coagulation is averaged over four time periods (00:00–06:00; 06:00–12:00; 12:00–18:00; 18:00–24:00), and therefore the variation in particle number size distribution within each time period will contribute to the uncertainty in the estimate of kc. The coagulation rate coefficient is affected by Brownian motion, Van der Waals and viscous forces, gravitational collection, turbulent inertial motion and shear, and the fractal geometry of particles which causes difficulty in estimation of uncertainty in the coagulation models. Therefore in this study we have only calculated the uncertainty in the estimate of coagulation loss rate caused by the variation of particle number size distribution in each time period. Uncertainty in the deposition rate was propagated from uncertainties of total loss rate and coagulation loss rate at nighttime. The uncertainty in kloss, kd and kc were propagated to ke which causes large overall uncertainty in ke. The relative standard error of ke for particles at a diameter of 14.3 nm was up to 26%. Details of uncertainty calculation are included in the SI.
3.3.5. Discussion on contribution (%) of deposition, coagulation, and evaporation processes to the total loss rate of indoor particles in different time periods
The contribution (%) of each process to total loss of indoor particles was calculated as the following equation: contribution of each process = keach process/ktotal loss rate *100. The result is shown in Figure S9 (see the SI). The contribution of each process varies substantially according to particle diameter. The highest contribution of evaporation to total loss was found for particles in the size range between 29 nm and 60 nm. The contribution of each process also depends on the time of day. A larger contribution of evaporation and coagulation was found during daytime. The contributions of deposition, coagulation and evaporation to the total loss of indoor ultrafine particles (16.5 nm < Dp < 100 nm) were 48.7, 12.7, and 37.5%, respectively, during the daytime, while the corresponding values during the 18:00–24:00 period were 62.2, 15.3, and 22.5%, respectively. From midnight to 06:00, the contribution of evaporation was assumed to be zero.
The chemical components of diesel-exhaust nanoparticles are predominantly semi-volatile hydrocarbons (Alam et al. Citation2016). Such compounds tend to adsorb strongly to indoor surfaces, causing evaporation of the parent particles (Van Loy et al. Citation2001; Weschler and Nazaroff Citation2008), as seen in this environment.
3.4. Investigation of the processes affecting outdoor particles in indoor environments using a receptor model (PMF)
PMF was applied to both indoor and indoor size distributions simultaneously and a PMF solution of four main factors was selected. The profiles of the four factors including traffic accumulation mode, traffic fresh nucleation mode, traffic aged nucleation mode and urban accumulation mode are shown in .
The first factor shows the main outdoor particle number size distribution in the size range between 52 nm and 93 nm, with the mode around 70 nm. The diurnal pattern of this factor shows a very high peak corresponding to morning and evening traffic rush hours. This factor has been attributed to the traffic solid particle (soot) mode (Ristovski et al. Citation2006; Ogulei et al. Citation2007; Harrison et al. Citation2011). This traffic accumulation mode which mostly consists of black carbon and organic compounds shows similar outdoor and indoor particle number size distributions.
The outdoor PNSD of the second factor is dominated by the accumulation mode with the modal diameter of 107 nm. The diurnal pattern shows its contribution to increase at midnight and midday, with a lesser contribution in the early morning and afternoon. This factor could be associated with the urban background accumulation mode which may consist of the aged solid particle mode from traffic emissions during daytime and other domestic combustion such as biomass burning as well as secondary constituents during nighttime (Vu et al. Citation2016; Janhäll et al. Citation2010). The indoor concentration of the urban background accumulation mode particles was lower than outdoors for both number and volume metrics. This decrease could be partly explained by the dissociation behavior of secondary aerosols, particularly NH4NO3, leading to high loss rates of these particles indoors (Talbot et al. Citation2016). Zauli Sajani et al. (Citation2015) reported that indoor levels of ammonium and nitrates in PM2.5 were much lower than outdoors in the same experimental set-up.
The two last factors show major modes at diameters of 19 nm and 34 nm, respectively. They both show a larger contribution during daytime with the highest contribution around 7:00–9:00 AM. This suggests that these factors are associated with traffic fresh and aged nucleation modes (Ogulei et al. Citation2007; Gu et al. Citation2011). As shown in , traffic nucleation modes which mainly contain volatile compounds show a huge decrease in the particle number concentrations when they enter into the house.
In summary, particles of each type have a different behavior when they are transported from outdoors to the indoor environment. Traffic-generated soot particles have a longer lifetime indoors due to their chemical stability and typical diameter in the accumulation mode. Urban accumulation mode particles, which probably contain more secondary constituents, present higher loss rates than traffic accumulation mode particles. This also shows clearly how the nucleation mode particles are subject to substantial losses within the indoor environment, as a result of their higher concentrations, and hence coagulation rates, diffusivity and hence depositional efficiency, and tendency for evaporation.
UAST_1343453_Supplementary_File.zip
Download Zip (1.4 MB)Funding
This research is supported by the European Union through the FP7-MC-ITN 315760, HEXACOMM.
References
- Alam, M. K. (1987). The Effect of Van Der Waals and Viscous Forces on Aerosol Coagulation. Aerosol Sci. Technol., 6:41–52.
- Alam, M. S., Zeraati-Rezaei, S., Stark, C. P., Liang, Z., Xu, H., and Harrison, R. M. (2016). The Characterisation of Diesel Exhaust Particles–Composition, Size Distribution and Partitioning. Faraday Discuss., 189:69–84.
- Carslaw, D. C., and Ropkins, K. (2012). Open air—an R package for Air Quality Data Analysis. Environ. Modell. Softw., 27:52–61.
- Chao, C. Y., Wan, M., and Cheng, E. C. (2003). Penetration Coefficient and Deposition Rate as a Function of Particle Size in Non-Smoking Naturally Ventilated Residences. Atmos. Environ., 37:4233–4241.
- Charron, A., and Harrison, R. M. (2003). Primary Particle Formation from Vehicle Emissions During Exhaust Dilution in the Roadside Atmosphere. Atmos. Environ., 37:4109–4119.
- Chen, C., and Zhao, B. (2011). Review of Relationship Between Indoor and Outdoor Particles: I/O Ratio, Infiltration Factor and Penetration Factor. Atmos. Environ., 45:275–288.
- Chen, C., Zhao, B., and Weschler, C. J. (2012). Indoor Exposure to “Outdoor PM10”: Assessing Its Influence on the Relationship Between PM10 and Short-Term Mortality in US Cities. Epidemiol., 23:870–878.
- Chen, R., Zhou, B., Kan, H., and Zhao, B. (2013). Associations of Particulate Air Pollution and Daily Mortality in 16 Chinese Cities: An Improved Effect Estimate After Accounting for the Indoor Exposure to Particles of Outdoor Origin. Environ. Pollut., 182:278–282.
- Dall'Osto, M., Thorpe, A., Beddows, D. C. S., Harrison, R. M., Barlow, J. F., Dunbar, T., Williams, P., and Coe, H. (2011). Remarkable Dynamics of Nanoparticles in the Urban Atmosphere. Atmos. Chem. and Phys., 11:6623–6637.
- de Kok, T. M., Driece, H. A., Hogervorst, J. G., and Briedé, J. J. (2006). Toxicological Assessment of Ambient and Traffic-Related Particulate Matter: A Review of Recent Studies. Mutat. Res., 613:103–122.
- Gent, J. F., Koutrakis, P., Belanger, K., Triche, E., Holford, T. R., Bracken, M. B., and Leaderer, B. P. (2009). Symptoms and Medication Use in Children With Asthma and Traffic-Related Sources of Fine Particle Pollution. Environ. Health Perspect., 117:1168.
- Gu, J., Pitz, M., Schnelle-Kreis, J., Diemer, J., Reller, A., Zimmermann, R., Soentgen, J., Stoelzel, M., Wichmann, H. E., Peters, A., and Cyrys, J. (2011). Source Apportionment of Ambient Particles: Comparison of Positive Matrix Factorization Analysis Applied to Particle Size Distribution and Chemical Composition Data. Atmos. Environ., 45:1849–1857.
- Harrison, R. M., Delgado-Saborit, J. M., Baker, S. J., Aquilina, N., Meddings, C., Harrad, S., Matthews, I., Vardoulakis, S., and Anderson, H. Measurement and Modeling of Exposure to Selected Air Toxics for Health Effects Studies and Verification by Biomarkers. Research Report, Health Effects Institute 2009, 143:3–96, discussion 97–100.
- Harrison, R. M., Beddows, D. C., and Dall'Osto, M. (2011). PMF Analysis of Wide-Range Particle Size Spectra Collected on a Major Highway. Environ. Sci. Technol., 45:5522–5528.
- Harrison, R. M., Jones, A. M., Beddows, D. C., Dall'Osto, M., and Nikolova, I. (2016). Evaporation of Traffic-Generated Nanoparticles During Advection from Source. Atmos. Environ., 125:1–7.
- Hussein, T., Hämeri, K., Heikkinen, M. S., and Kulmala, M. (2005). Indoor and Outdoor Particle Size Characterization at a Family House in Espoo–Finland. Atmos. Environ., 39:3697–3709.
- Hussein, T., Glytsos, T., Ondráček, J., Dohányosová, P., Ždímal, V., Hämeri, K., Lazaridis, M., Smolík, J., and Kulmala, M. (2006). Particle Size Characterization and Emission Rates During Indoor Activities in a House. Atmos. Environ., 40:4285–4307.
- Jacobson, M. Z. (1994). Developing, Coupling, and Applying a Gas, Aerosol, Transport, and Radiation Model to Study Urban and Regional Air Pollution. PhD Thesis, University of California, Los Angeles.
- Jacobson, M. Z., and Seinfeld, J. H. (2004). Evolution of Nanoparticle Size and Mixing State Near the Point of Emission. Atmos. Environ., 38:1839–1850.
- Jacobson, M. Z., Kittelson, D. B., and Watts, W. F. (2005). Enhanced Coagulation Due to Evaporation and Its Effect on Nanoparticle Evolution. Environ. Sci. Technol., 39:9486–9492.
- Janhäll, S., Andreae, M. O., and Pöschl, U. (2010). Biomass Burning Aerosol Emissions from Vegetation Fires: Particle Number and Mass Emission Factors and Size Distributions. Atmos. Chem. Phys., 10:1427–1439.
- Jeong, C.-H., and Evans, G. J. (2009). Inter-Comparison of a Fast Mobility Particle Sizer and a Scanning Mobility Particle Sizer Incorporating an Ultrafine Water-Based Condensation Particle Counter. Aerosol Sci. Technol., 43:364–373.
- Kaminski, H., Kuhlbusch, T. A., Rath, S., Götz, U., Sprenger, M., Wels, D., Polloczek, J., Bachmann, V., Dziurowitz, N., and Kiesling, H.-J. (2013). Comparability of Mobility Particle Sizers and Diffusion Chargers. J. Aerosol Sci., 57:156–178.
- Kittelson, D. B. (1998). Engines and Nanoparticles: A Review. J. Aerosol Sci., 29:575–588.
- Kuhn, T., Krudysz, M., Zhu, Y., Fine, P. M., Hinds, W. C., Froines, J., and Sioutas, C. (2005). Volatility of Indoor and Outdoor Ultrafine Particulate Matter Near a Freeway. J. Aerosol Sci., 36:291–302.
- Lai, A. C., and Nazaroff, W. W. (2000). Modeling Indoor Particle Deposition from Turbulent Flow onto Smooth Surfaces. J. Aerosol Sci., 31:463–476.
- Lepeule, J., Litonjua, A. A., Coull, B., Koutrakis, P., Sparrow, D., Vokonas, P. S., and Schwartz, J. (2014). Long-Term Effects of Traffic Particles on Lung Function Decline in the Elderly. Am. J. Respir. and Crit. Care Med., 190:542–548.
- Long, C. M., Suh, H. H., Catalano, P. J., and Koutrakis, P. (2001). Using Time- and Size-Resolved Particulate Data To Quantify Indoor Penetration and Deposition Behavior. Environ. Sci. Technol., 35:2089–2099.
- Müller, H. (1928). Zur Allgemeinen Theorie Ser Raschen Koagulation. Fortschrittsberichte über Kolloide und Polymere, 27:223–250.
- Ogulei, D., Hopke, P. K., Chalupa, D. C., and Utell, M. J. (2007). Modeling Source Contributions to Submicron Particle Number Concentrations Measured in Rochester, New York. Aerosol Sci. Technol., 41:179–201.
- Ranft, U., Schikowski, T., Sugiri, D., Krutmann, J., and Krämer, U. (2009). Long-Term Exposure to Traffic-Related Particulate Matter Impairs Cognitive Function in the Elderly. Environ. Res., 109:1004–1011.
- Rim, D., Green, M., Wallace, L., Persily, A., and Choi, J. I. (2012). Evolution of Ultrafine Particle Size Distributions Following Indoor Episodic Releases: Relative Importance of Coagulation, Deposition and Ventilation. Aerosol Sci. Technol., 46:494–503.
- Ristovski, Z., Jayaratne, E., Lim, M., Ayoko, G. A., and Morawska, L. (2006). Influence of Diesel Fuel Sulfur on Nanoparticle Emissions from City Buses. Environ. Sci. Technol., 40:1314–1320.
- Salam, M. T., Islam, T., and Gilliland, F. D. (2008). Recent Evidence for Adverse Effects of Residential Proximity to Traffic Sources on Asthma. Curr. Opin. Pulm. Med., 14:3–8.
- Seinfeld, J. H., and Pandis, S. N. (2012). Atmospheric Chemistry and Physics: From Air Pollution to Climate Change. John Wiley & Sons, Inc., Hoboken, New Jersey.
- Sceats, M. G. (1989). Brownian Coagulation in Aerosols—The Role of Long Range Forces. J. Colloid Interf. Sci., 129:105–112.
- Shi, J. P., and Harrison, R. M. (1999). Investigation of Ultrafine Particle Formation during Diesel Exhaust Dilution. Environ. Sci. Technol., 33:3730–3736.
- Suh, H. H., and Zanobetti, A. (2010). Exposure Error Masks The Relationship Between Traffic-Related Air Pollution and Heart Rate Variability (HRV). J. Occup. Environ. Med., 52:685.
- Talbot, N., Kubelova, L., Makes, O., Cusack, M., Ondracek, J., Vodička, P., Schwarz, J., and Zdimal, V. (2016). Outdoor and Indoor Aerosol Size, Number, Mass and Compositional Dynamics at an Urban Background Site During Warm Season. Atmos. Environ., 131:171–184.
- Van Loy, M. D., Riley, W. J., Daisey, J. M., and Nazaroff, W. W. (2001). Dynamic Behavior of Semivolatile Organic Compounds in Indoor Air. 2. Nicotine and Phenanthrene with Carpet and Wallboard. Environ. Sci.Technol., 35:560–567.
- Vu, T. V., Delgado-Saborit, J. M., and Harrison, R. M. (2015). Review: Particle Number Size Distributions from Seven Major Sources and Implications for Source Apportionment Studies. Atmos. Environ., 122:114–132.
- Vu, T. V., Beddows, D. C., Delgado-Saborit, J. M., and Harrison, R. M. (2016). Source Apportionment of the Lung Dose of Ambient Submicrometre Particulate Matter. AAQR, 16:1548–1557.
- Von Klot, S., Cyrys, J., Hoek, G., Kühnel, B., Pitz, M., Kuhn, U., Kuch, B., Meisinger, C., Hörmann, A., and Wichmann, H.-E. (2011). Estimated Personal Soot Exposure is Associated with Acute Myocardial Infarction Onset in a Case-Crossover Study. Prog. Cardiovas. Discuss., 53:361–368.
- Weschler, C. J., and Nazaroff, W. W. (2008). Semivolatile Organic Compounds in Indoor Environments. Atmos. Environ., 42:9018–9040.
- Zauli Sajani, S., Ricciardelli, I., Trentini, A., Bacco, D., Maccone, C., Castellazzi, S., Lauriola, P., Poluzzi, V., Harrison, R. M. (2015). Spatial and Indoor/Outdoor Gradients in Urban Concentrations of Ultrafine Particles and PM 2.5 Mass and Chemical Components. Atmos. Environ., 103:307–320.
- Zhang, K.M., Wexler, A.S., Zhu, Y.F., Hinds, W.C., and Sioutas, C. (2004). Evolution of Particle Number Distribution Near Roadways. Part II: the ‘Road-to-Ambient'process. Atmos. Environ., 38:6655–6665.
- Zhao, B., and Wu, J. (2007). Particle Deposition in Indoor Environments: Analysis of Influencing Factors. J. Hazardous Mater., 137:439–448.
- Zhu, Y., Hinds, W. C., Krudysz, M., Kuhn, T., Froines, J., and Sioutas, C. (2005). Penetration of Freeway Ultrafine Particles into Indoor Environments. J. Aerosol Sci., 36:303–322.