ABSTRACT
The Aerodynamic Aerosol Classifier (AAC) is a novel instrument that selects aerosol particles based on their relaxation time or aerodynamic diameter. Additional theory and characterization is required to allow the AAC to accurately measure an aerosol’s aerodynamic size distribution by stepping while connected to a particle counter (such as a Condensation Particle Counter, CPC). To achieve this goal, this study characterized the AAC transfer function (from 32 nm to 3 μm) using tandem AACs and comparing the experimental results to the theoretical tandem deconvolution. These results show that the AAC transmission efficiency is 2.6–5.1 times higher than a combined Krypton-85 radioactive neutralizer and Differential Mobility Analyzer (DMA), as the AAC classifies particles independent of their charge state. However, the AAC transfer function is 1.3–1.9 times broader than predicted by theory. Using this characterized transfer function, the theory to measure an aerosol’s aerodynamic size distribution using an AAC and particle counter was developed. The transfer function characterization and stepping deconvolution were validated by comparing the size distribution measured with an AAC-CPC system against parallel measurements taken with a Scanning Mobility Particle Sizer (SMPS), CPC, and Electrical Low Pressure Impactor (ELPI). The effects of changing AAC classifier conditions on the particle selected were also investigated and found to be small (<1.5%) within its operating range.
Copyright © 2018 American Association for Aerosol Research
EDITOR:
1. Introduction
A new instrument, the Aerodynamic Aerosol Classifier (AAC), was recently developed by Tavakoli and Olfert (Citation2013) and released commercially by Cambustion Ltd. It classifies nanoparticles based on their aerodynamic diameter , an equivalent particle diameter with unit density (
) that has the same settling velocity as the particle of interest. This characteristic is the main consideration where particle inertia dominates, such as respiratory deposition (Finlay Citation2001), atmospheric lifetime/settling (Hinds Citation1999), and particle separation/collection using filters, cyclones, and impactors (Kulkarni et al. Citation2011).
The particle relaxation time (τ) is related to three common equivalent particle diameters by[1] where m is the particle mass, B is the particle mobility, Cc is the Cunningham slip correction, μ is the surrounding gas viscosity,
is the particle effective density,
is the mobility diameter,
is the particle material density,
is the volume equivalent diameter, and χ is the particle shape factor (Hinds Citation1999; DeCarlo et al. Citation2004). The nomenclature for this study has been included in the supplemental information (SI) Section S1.
The AAC selects particles of a single aerodynamic diameter (in reality, a narrow range of aerodynamic diameters distributed about its setpoint) by passing the aerosol sample between concentric cylinders spinning at the same speed. The centrifugal force applied to each particle is controlled by the classifier speed, while their residence time in the classifier is controlled by the total classifier axial flow (i.e., combined sheath and sample flows). Thus, only particles within this narrow aerodynamic diameter range follow the correct trajectory and pass through the AAC classifier (Tavakoli and Olfert Citation2013). Particles with aerodynamic diameters smaller than the AAC setpoint have insufficient radial trajectory and thus remain entrained in the sheath flow, while larger aerodynamic diameters have excessive radial trajectory and impact the outer surface of the classifier.
The TSI Aerodynamic Particle Sizer (APS) Spectrometer and Dekati Electrical Low Pressure Impactor (ELPI) are other commercial instruments that measure aerodynamic size distributions of aerosols. The APS measures the aerodynamic size distribution of an aerosol by accelerating it through a nozzle and measuring the particle’s time-of-flight between two laser beams relative to the gas velocity (Wilson and Liu Citation1980). The ELPI is a cascade impactor that quantifies the particle collection on each stage by measuring the particles grounding their charge upon impact with each plate (Keskinen et al. Citation1992). While the APS and ELPI both characterize the aerodynamic size distribution rapidly (order of sub-seconds) compared to the AAC (order of minutes), the measurement resolution, scan range, and number of points per decade are significantly easier to change in the AAC (i.e., based on classifier speed, sheath flow, and number of setpoint steps). The ELPI size distribution measurement range and resolution are limited by the number impactor stages and their corresponding cut-off diameters. The APS measurement range and resolution depends on many factors, including the particle acceleration produced by the nozzle and temporal resolution of the two laser beams.
Furthermore, other effects must be accounted for in the APS and ELPI. The APS nozzle can produce a large velocity gradient (up to 1/3 the speed of sound) between the particles and surrounding gas, resulting in liquid particle deformation/breakup (Baron Citation1986; Chen et al. Citation1990). Due to the APS operating outside the Stokes regime its measurements must also be corrected for particle density, becoming significant for aerodynamic diameters greater than 5 μm with densities greater than 2 g/cm3 (Chen et al. Citation1990). The ELPI requires high sample flow rates (>10 L/min) and low pressures (which may alter the properties of volatile particles) to measure submicron particles. In addition, the particle charging fractions must be known to accurately apply the inversion within the ELPI.
By classifying particles independent of their charge, the AAC avoids the multiple-charging artifacts that are present in electrostatic classifiers (Tavakoli et al. Citation2014), such as a Differential Mobility Analyzer (DMA, Knutson and Whitby Citation1975) or Centrifugal Particle Mass Analyzer (CPMA, Olfert and Collings Citation2005). The contribution of multiple-charged particles is often neglected, but can become significant depending on the aerosol charge distribution, as well as the setpoint of the electrostatic classifier relative to the source particle size distribution (Johnson et al. Citation2013). The DMA classifies particles based on their electrical mobility diameter by applying an electrical field to induce a known electrostatic force (Knutson and Whitby Citation1975). Each particle’s residence time in the classifier is controlled by the total axial classifier flow (i.e., combined sheath and sample flows). Thus, only particles with a narrow range of electrostatic force to drag ratios or mobility diameters follow the correct trajectory and pass through the DMA (Stolzenburg Citation1988). However an aerosol’s charge distribution can vary with particle morphology, size, and composition (Lall and Friedlander Citation2006; Lall et al. Citation2006; Ouf and Sillon Citation2009; Gopalakrishnan et al. Citation2013, Citation2015) affecting the accuracy of size distributions measured by electrostatic classifiers.
While the transfer function of various DMA designs has been extensively characterized using tandem classifier configurations (Hummes et al. Citation1996; Fissan et al. Citation1996; Birmili et al. Citation1997; Martinsson et al. Citation2001; Karlsson and Martinsson Citation2003; Li et al. Citation2006), to date there has only been limited characterization of the AAC in the literature. Tavakoli et al. (Citation2014) characterized the AAC transfer function using two alternative methods due to the existence of only one AAC at that time (i.e., the first prototype, which is physically quite different to the commercially available version). Using polystyrene latex (PSL) particles and assuming their atomized size distribution was normally distributed with a known effective density, the theoretical transfer function convolution was compared to the PSL concentrations measured upstream and downstream of the AAC using a Condensation Particle Counter (CPC, Agarwal and Sem Citation1980). The prototype was also characterized using an AAC-DMA system, however the tandem deconvolution required both the theoretical DMA and AAC transfer functions. Thus, differences between the theoretical and experimental DMA transfer functions as well as uncertainty in particle effective density likely introduced errors into the AAC transfer function characterization.
Therefore, this study follows Tavakoli et al.’s (Citation2014) recommendation and characterizes the AAC transfer function using a tandem AAC (TAAC) system to quantify the transmission efficiency and transfer function broadening from non-ideal particle behavior such as diffusion and impaction. Based on this characterized transfer function, its deconvolution is also developed allowing the AAC and a particle counter (such as a CPC or electrometer) system to accurately measure the aerodynamic size distribution of an aerosol source from 32 nm to 3 μm. This upper size limit is dictated by the 3775 CPC used for this study and could be expanded up to 6 μm with the appropriate particle counter. The AAC transfer function characterization and deconvolution are validated by comparing the size distribution measured with an AAC-CPC system against parallel measurements taken with a Scanning Mobility Particle Sizer (SMPS, Wang and Flagan Citation1990), CPC, and an ELPI.
2. Experimental setup
2.1. AAC transfer function characterization
The tandem AAC (TAAC) setup used to characterize the AAC transfer function is shown in . The upstream AAC (AAC 1) was set at a constant setpoint while the downstream AAC (AAC 2) stepped through the domain of the classified particles
and recorded the number concentration of the twice-classified particles (N2) measured by a TSI 3775 CPC. The 3775 CPC utilizes a 300 cm3/min aerosol sample flow and has a 4 nm minimum detectable particle size. Before and after stepping, AAC 2 was bypassed to directly measure the particle number concentration passing through AAC 1 (N1).
At reference classifier conditions (296.15 K and 101,325 Pa), the AAC can classify aerodynamic particle diameters from 32 nm to 3 μm at low flow (LF, 0.3/3 L/min sample/sheath flow) and from 202 nm to 6.8 μm at high flow (HF, 1.5/15 L/min sample/sheath flow) by changing the classifier speed (20 to 700 rad/s at LF and 20 to 500 rad/s at HF). Thus, five different aerodynamic sizes, spaced equally across each of these ranges logarithmically, were selected to characterize the AAC transfer function. At low flow, AAC 1 was set at 50, 125, 325, 825, and 2100 nm, while at high flow, AAC 1 was set at 300, 600, 1200, and 2400 nm. These size range endpoints (e.g., 50 nm at low flow) were selected to be offset from the AAC’s classification bounds (e.g., 32 nm at low flow), thus allowing AAC 2 to characterize both sides of the distribution classified by AAC 1. At each of these AAC 1 setpoints, five independent AAC 2 scans were completed, including measuring N1 prior to (pre) and after (post) each AAC 2 scan. To limit uncertainties from an unstable aerosol source, a scan was repeated if its pre or post N1 measurement stability and/or the agreement between these values was not within 6%. This threshold was selected based on the other uncertainties within the experimental setup and only increases the propagated particle concentration uncertainty from 11.2% to 12.7% with the 10% CPC measurement uncertainty (TSI Citation2007) and assuming an indirect AAC uncertainty contribution of 5%. These experiments were repeated with the AAC positions reversed to determine the transfer function variations between them.
The AAC transfer function was not characterized at aerodynamic diameter setpoints larger than 3000 nm, as this was above the 3 μm particle size limit of the 3775 CPC. Attempts were made to measure 4800 nm AAC classified particle number concentrations using a Palas GmbH Welas® digital 1000H optical aerosol spectrometer. While the optical data showed the AAC is capable of classifying particles in this size range, the twice-classified number concentrations (N2) were too low for the Welas® to accurately quantify. Given that the particle concentration was detectable after classification by one AAC, but not two, indicates that the losses in the AAC at this size range should also be considered. Thus, characterizing the AAC transfer function above 3 μm with a larger underlying size distribution and/or using a different particle counterFootnote1 should be considered in future work.
2.2. AAC size distribution deconvolution and validation
The AAC-CPC system used to characterize different aerosol sources in parallel to SMPS, CPC, and ELPI measurements is shown in . The AAC stepped through the aerodynamic diameter domain of the source and recorded the corresponding classified particle number concentration (N) measured by a 3775 CPC. For both the TAAC and size distribution validation measurements, the conductive tubing lengths of each aerosol sample flow path were the same and therefore no particle loss correction was required. HEPA filtered make-up air was added as close as possible to the ELPI inlet to maintain a similar sample flow rate and thus particle line losses as the other instrument sample lines.
Figure 2. AAC-CPC system used to measure the aerodynamic size distribution of an aerosol. This system was validated by comparing against ELPI, SMPS, and CPC measurements in parallel.
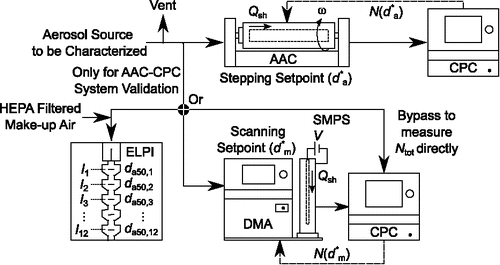
A TSI SMPS, consisting of Krypton-85 radioactive source, 3080 DMA (with 3081 long column), and 3775 CPC in series, measured the aerosol’s mobility size distribution. The SMPS was operated with an aerosol and sheath flow of 0.3 and 3 L/min, respectively, corresponding to a 14.6–661 nm mobility scan range or 13.4–629 nm aerodynamic scan range for a particle density of 914 kg/m3. Similar to the AAC-CPC system, the DMA setpoint was changed and the corresponding classified particle number concentration (N) was measured and recorded as a function of its mobility diameter setpoint. However, the DMA voltage was scanned exponentially to reduce the overall measurement time to 3 min, compared to an AAC-CPC measurement time of 10–15 min. To avoid introducing disagreement between different CPCs, the same 3775 CPC was switched between downstream of the AAC and DMA. The AAC or DMA was also bypassed to allow the CPC to directly measure the total particle number concentration (
) of the polydispersed aerosol.
A Dekati Classic ELPI® quantified the aerosol’s aerodynamic size distribution from 43.3 nm to 8.59 μm using a corona charger to produce a known particle charge distribution before passing the sample through 12 impactor stages. The cutoff aerodynamic diameter for each impactor decreases with each sequential stage. The current produced from the particles colliding with each impactor plate was then independently measured. By using the measured currents (Ii) and knowing the cutoff size for each stage (da50,i), the aerodynamic size distribution of the aerosol was determined.
The AAC transfer function characterization and deconvolution were validated by comparing the lognormal distribution parameters fitted to the size distributions determined from the AAC-CPC system to those fitted to the SMPS, CPC, and ELPI measurements of the same aerosol source.
2.3. Aerosol generation
The polydispersed aerosols used for these experiments were generated by atomizing different oils with a BGI Collison or TSI 9302 constant output nebulizer. Through multiple design iterations and testing of the particle generation inputs (compressed air control, liquid volume or feed rate, and dilution system), either system was able to produce a total particle number concentration stable within 6% over the 10–15 min measurement time. DOS (Bis(2-ethylhexyl) sebacate) and 702 diffusion pump oils were selected due to their low vapor pressures (i.e., resistance to evaporation) and forming particles with a known spherical morphology and density (DOS: 914 kg/m3 and 702: 1070 kg/m3). These characteristics allowed an accurate conversion between aerodynamic and mobility size distributions. The AAC transfer function was characterized using DOS, then validated (including its inversion) with both sources.
The BGI Collison nebulizer produced a slightly narrower DOS particle mobility size distribution with a larger count median diameter (CMD) and higher total particle number concentration than the TSI nebulizer (312 nm, 1.90 GSD, and 5.05 × 107 cm−3 versus 250 nm, 1.97 GSD, and 1.97 × 107 cm−3, respectively). This source was used to collect any of the TAAC data where AAC 1’s aerodynamic diameter setpoint was greater than 300 nm. The TSI 9302 constant output nebulizer was used to collect the remaining TAAC DOS data (i.e., where <300 nm). During validation, the TSI nebulizer produced a 702 pump oil particle mobility size distribution with a 287 nm CMD, 1.73 GSD, and 2.46 × 107 cm−3 total particle number concentration. To decrease the particle number concentration produced by the TSI nebulizer for either fluid, the liquid feed rate was controlled to 6 mL/h using a Harvard Apparatus Model 22 syringe pump.
For either nebulizer, a liquid trap was used to remove excess atomized fluid, while a Cambustion rotating disk diluter was used to control the particle number concentration upstream of the different experimental setups. The dilution ratio was set to ensure the CPC operated below its photometric mode (< 5 × 105 particles per cm3) in any of the previously described experimental setups (i.e., measuring N1 or N2 in the TAAC setup and ,
or
in the validation setup).
3. Theory
The particle relaxation time selected by the AAC (τ*) was determined by Tavakoli and Olfert (Citation2013) to be[2] where ω is the classifier rotational speed, r1 is the classifier inner radius, r2 is the classifier outer radius, L is the classifier length,
is the sheath flow rate entering the classifier, and
is the sheath flow rate leaving the classifier.
Previous studies have developed different models that can be used represent the AAC transfer function (TF) as shown in over the non-dimensional particle relaxation time domain or the particle relaxation time (τ) normalized by the AAC setpoint (τ*). The transfer function is the probability of each particle passing through the classifier as a function of the classifier measurand. For example, at the peak
, the non-diffusing transfer functions estimate 100% transmission efficiency for particles with that relaxation time (τ = τ*). Tavakoli and Olfert (Citation2013) developed the non-diffusing (ND) transfer function following particle streamline theory and considered diffusion (D) by assuming that it spreads the particles in a Gaussian distribution about the ND model. Further characteristics of this non-diffusing transfer function are discussed in SI Section S2. The non-diffusing and diffusing log-normal (Log) approximations of the AAC transfer function were calculated by applying the theory developed by Stolzenburg and McMurry (Citation2008) to represent the DMA transfer function log-normally. This theory can be directly applied to the AAC given that the diffusing AAC particle streamline transfer function determined by Tavakoli and Olfert (Citation2013) has the same form as the DMA (Stolzenburg Citation1988).
Figure 3. Different theoretical representations of the balanced flow AAC transfer function (TF), where the non-diffusing (ND) and diffusing (D) transfer functions were developed by Tavakoli and Olfert (Citation2013) and the log-normal (Log) transfer functions were developed following Stolzenburg and McMurry (Citation2008). The diffusing transfer functions shown represent an AAC setpoint equivalent to a 75 nm particle mobility diameter.
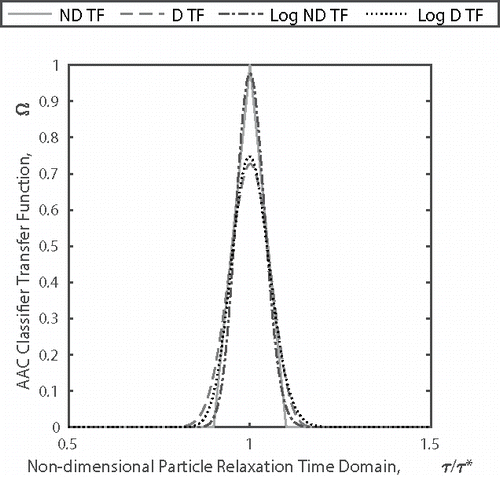
3.1. AAC transfer function characterization
Non-ideal particle behavior within the AAC, such as particle diffusion and impaction, must be considered for accurate size distribution measurements. This study follows Martinsson et al.’s (Citation2001) methodology used to characterize the DMA transfer function and quantifies the non-ideal particle behavior within the AAC using a tandem AAC (TAAC) system and comparing its results against its theoretical tandem deconvolution. The non-diffusing or triangular AAC transfer function developed by Tavakoli and Olfert (Citation2013) was selected for the TAAC deconvolution due to ease of parameterization and is represented as
[3] where β and δ are non-dimensional classifier flow parameters that are defined as
[4]
[5]
The aerosol flow rate entering and leaving the classifier is denoted by and
, respectively. Due to current instrument configuration, this study only considered balanced (B) classifier flows (
and
). Therefore δ = 0 and the non-diffusing AAC transfer function (Equation (Equation3
[3] )) simplifies to
[6]
To capture the non-ideal particle behavior, a transmission efficiency and transfer function width factor
were introduced into this triangular transfer function (similar to Martinsson et al. Citation2001) as follows:
[7]
As demonstrated in SI Section S3, the transmission efficiency scales the transfer function’s integrated area to quantify particle losses, such as diffusion and impaction. The transfer function width factor scales the transfer function full width at half maximum (FWHM) to quantify its broadening due to particle diffusion and other sources, such as classifier flow effects and setpoint variations. This parameterized transfer function is represented in .
Figure 4. Idealized (Equation (Equation3[3] )) and non-idealized (Equation (Equation7
[7] )) balanced flow AAC transfer function.
![Figure 4. Idealized (Equation (Equation3[3] )) and non-idealized (Equation (Equation7[7] )) balanced flow AAC transfer function.](/cms/asset/d97d5f25-75c8-4939-8b3e-036658faa48e/uast_a_1440063_f0004_b.gif)
Based on this transfer function, the theoretical particle number concentration ratio measured between upstream (N1) and downstream (N2) of AAC 2 was determined using the TAAC deconvolution as follows:(8) where
is the classification agreement between AAC 1 and 2 (i.e., τ*2/τ*1), and
is the counting efficiency at aerodynamic diameter
of the particle counter downstream of the tandem AACs.
To simplify this deconvolution, this study assumed that the particle counting efficiency was constant over the narrow aerodynamic diameter range stepped through by AAC 2 (i.e., ), and that AAC 1 and 2 had the same transfer function width factor (i.e., μΩ, 1 = μΩ, 2). To maximize validity of the latter, AAC 1 and 2 were operated with the same sample and sheath flow rates for each TAAC data-point collected. Since N1 was measured directly, the losses within AAC 1 were already accounted for and λΩ, 1 = 1 was used for the theoretical TAAC deconvolution. By comparing this theoretical N2/N1 concentration ratio (Equation (Equation8
(8) )) to the concentrations measured experimentally and minimizing the difference between them using chi-squared minimization, the transmission efficiency (λΩ,2) and transfer function width factor (μΩ,2) for AAC 2 were determined.
3.2. AAC size distribution deconvolution
As developed in the SI (full derivation SI Section S4), the theory to convert the raw measurements collected using an AAC (operating with balanced classifier flows) and particle counter (such as a CPC or electrometer) to represent the aerodynamic size distribution of an aerosol source
can be summarized as
[9] where Ni is particle concentration measured downstream of the AAC, ηi is the counting efficiency of the particle counter downstream of the AAC, and β*NI can be found from
[10]
As per SI Section S5, the logarithmic ratio to shift from the particle relaxation time to aerodynamic diameter domain was found to be
[11] where Kim et al. (Citation2005) determined
,
, and
. The Cunningham slip correction factor (Cc) and mean free path (λ) can be determined from SI Equations (S5.2) and (S5.3), respectively. When the CPC is connected to the AAC serially, the internal AAC software automatically records the CPC measurements, calculates the size distribution inversion (i.e., Equations (Equation9
[9] )–(Equation11
[11] )), and outputs the results in a tab delimited text file.
In the interest of completeness, this study also developed the supporting theory of the AAC steady-state unbalanced (UB) flow inversion in SI Section S8. However, future work is required to experimentally validate this theory. The unbalanced classifier flow configuration is harder to implement as it requires three independent flow control points, rather than two. Similar to a DMA, Tavakoli and Olfert (Citation2013) predicted unbalanced flows will produce a trapezoidal-shaped AAC transfer function with higher classification resolution as the aerosol sample to sheath ratio (β) decreases.
4. Results and discussion
4.1. AAC transfer function characterization
The AAC transmission efficiency, transfer function width, and classification agreement factors (each an average of five AAC 2 scans at each AAC 1 setpoint) for two different AACs (denoted as AAC A and AAC B) are shown in . The error bars represent the 95% confidence interval (CI) of each average assuming a t-distribution.
4.1.1. Transmission efficiency, 
To estimate the AAC’s transmission efficiency across its entire classification range, the transmission efficiencies (λΩ,AAC) measured experimentally were fitted through chi-squared minimization following:[12] where
is the classifier entrance/exit transmission efficiency and
is the diffusional transmission efficiency defined by Karlsson and Martinsson (Citation2003) as
(13)
The non-dimensional deposition parameter is determined by
[14] where
is the length of a circular tube with the same diffusion deposition as the classifier, D is the diffusion coefficient of the particles, and
is the aerosol flow rate into the classifier.
The DMA transmission efficiency (λΩ,DMA) was also estimated using Equation (Equation12[12] ) with input parameters experimentally determined by Karlsson and Martinsson (Citation2003), and multiplying by the fraction of particles with a single negative charge state. The particle charge states were estimated following Wiedensohler (Citation1988). Even though the AAC effective deposition length is significantly longer than the DMA (
of 46.0 m versus 7.1 m, respectively) and its entrance/exit losses are higher (
of 0.8 versus 0.98, respectively), the charging fraction is the dominate factor for the DMA transmission efficiency and limits it to less than 30%. Thus, the AAC classifying independent of particle charge not only avoids multiple-charging artifacts, but allows for transmission efficiencies approximately 2.6–5.1 times higher than a DMA for the same particle size.
4.1.2. Transfer function width-factor, 
Contrary to the AAC transfer function theory developed by Tavakoli and Olfert (Citation2013), its transfer function is 1.5–2.4 times (i.e., ratio of DMA and AAC ’s at each particle size) experimentally broader than the DMA transfer function characterized by Birmili et al. (Citation1997). The DMA transfer function width starts to deviate from theory below 100 nm due to particle diffusion with a maximum difference of less than 14%. The AAC transfer function width deviates from theory across its entire measurement range and may be due to a classifier flow effect. This is supported by the AAC transfer function broadening as classifier flow increases (i.e.,
). The trend of the AAC transfer function broadening at high flow (i.e., positive power coefficient, b) is also in contrast to the broadening trends observed in the AAC at low flow or DMA (i.e., negative power coefficients, b). This may also be an indication of a classifier flow effect. Further investigation is required to prove this hypothesis and develop possible improvements. This additional transfer function broadening can be negated by operating the AAC at a higher classifier resolution (i.e., higher sheath to sample flow ratio).
Based on fitting the experimental data of this study and Birmili et al. (Citation1997), the transfer function width factor of the AAC or a 3071 DMA
across their entire classification range can be estimated from
[15] where
is the particle diameter in nm and the transfer function broadening fitted coefficients are summarized in .
Table 1. Fitted coefficients to estimate transfer function broadening as function of particle size.
The additional error from using these curves to estimate the AAC transfer function broadening ranges from −4.2% to 3.7% for the flow-dependent fits (i.e., AAC LF and HF) and from −12.8% to 17.4% for the flow-independent fit (i.e., AAC All). The flow-independent fit considered all of the AAC transfer function broadening factors experimentally measured at both low and high flow, and is intended to be used as an approximation of the AAC transfer function broadening at other flow conditions where the AAC transfer function has not yet been characterized.
This study focused on spherical particles to establish the fundamental AAC classification theory, however future work should expand the transfer function characterization to non-spherical particles or aggregates. Based on theory (Tavakoli and Olfert Citation2013), varying particle morphology does not affect the AAC classification setpoint (i.e., the AAC classifies particles based solely on their relaxation time). However, varying effective particle density (often resulting from varying particle morphology) will affect the particle’s equivalent mobility diameter relative to its aerodynamic diameter and thus change the losses and broadening of the AAC transfer function due to particle diffusion. It is predicted varying effective density will only significantly affect the AAC transfer function where diffusion dominates (i.e., small mobility particle sizes, <100 nm) or large particles with high effective densities where impaction on the classifier inlet and outlet begin to dominate.
4.1.3. Setpoint agreement, τ*12
The classification agreement between AAC A and AAC B was 3% or better for 16 of the 18 data-points. It is hypothesized that the linear classification agreement trends observed are due to applying a linear sheath flow rate calibration that neglects the temperature changes of the sheath air caused by frictional heating from varying classifier speeds.
4.2. AAC size distribution validation
The AAC transfer function characterization and deconvolution were validated by comparing the size distributions and particle concentrations measured with an AAC-CPC system against parallel measurements taken with an SMPS and a CPC as shown in . The aerodynamic size distributions measured by the AAC were converted to mobility size distributions using the equations derived in SI Section S6. Including the AAC transfer function transmission efficiency and width factors within the transfer function deconvolution (NI) greatly improved agreement with the SMPS and CPC measurements as shown in . Without this correction, the AAC at low or high flow underestimated the total particle number concentration measured by 20.2%–28.6% as shown by the “Raw” measurements in .
Table 2. Comparison of mobility size distributions measured by SMPS (corrected for multiple-charging), and AAC (converted using known effective density) in parallel. The direct AAC-CPC systems measurements (denoted as Raw) were corrected for transfer function losses and broadening (denoted by NI).
The mobility size distributions measured by the SMPS were corrected for multiple-charging (MC) effects following He and Dhaniyala (Citation2013), while the particle charge state fractions were estimated following Wiedensohler (Citation1988) and Gunn and Woessner (Citation1956). This correction greatly improved agreement with the AAC and CPC measurements, increasing the SMPS CMD by 13.5%, 20.0%, and 16.6%, and decreasing the SMPS
by 50.8%, 56.8%, and 55.7% from the raw SMPS measurements for the DOS Constant Output, DOS Collison, and 702 pump oil Constant Output aerosol sources, respectively.
The agreement between the corrected SMPS and AAC size distribution measurements ranged from 1.3% to 5.9% for mobility count median diameter (CMD) and 0.1% to 4.5% for geometric standard deviation (GSD). The corrected AAC measurements total particle number
agreement with the CPC ranged from 0.1% to 6.6% as summarized in . The SMPS estimated total particle number concentration disagreement with the CPC was more than twice that of the AAC, with the multiple-charge corrected SMPS measurements overestimating the total particle number concentration by 11.8%, 19.7%, and 10.4% for the DOS Constant Output, DOS Collison, and 702 pump oil Constant Output aerosol sources, respectively.
The size distribution measured by the ELPI was slightly narrower and of larger aerodynamic count median diameter (CMD) compared to the distributions measured by the AAC as shown in . Considering the resolution of the Classic ELPI (approximately 5 size classes per decade), there was reasonable agreement between the aerodynamic size distributions determined by the AAC and ELPI in parallel of the same aerosol source, as shown in (within 18.2% of CMD
, 7.7% of GSD, and 22.4% of total particle number concentration). It should be noted that the spatial resolution of newer commercial cascade impactors has increased by an order of magnitude due to improved inversion techniques (Bau and Witschger Citation2013; Järvinen et al. Citation2014) and future work should consider the AAC agreement with these instruments.
Table 3. Comparison of aerodynamic size distributions measured by ELPI and AAC (corrected for transfer function losses and broadening, denoted as NI) in parallel.
4.3. Theoretical effect of varying AAC operating conditions
It was observed that the same particle will have a different aerodynamic diameter depending on the surrounding gas conditions. To account for this effect, DeCarlo et al.’s (Citation2004) theory regarding the relationship between a particle’s aerodynamic diameter and its volume equivalent diameter (an intrinsic particle property) was expanded as outlined in SI Section S7. The effects of varying AAC classifier conditions on the aerodynamic diameter selected were found to only be a function of Knudsen number (Kn, ratio of the gas mean free path to the particle diameter as per SI Equation (S7.4)) as shown in SI Figure S7.1. While the change in aerodynamic diameter is significant when measuring in different regimes, continuum versus free molecular, for example, the shift becomes less than 1.5% when only considering the AAC classifier operating range (0 to 40°C and 0.9 to 1.1 atm) as shown in SI Figures S7.2 and S7.3.
5. Conclusions and summary
The AAC transfer function was characterized using a tandem AAC system from 32 nm to 3 μm. The AAC transmission efficiency increases as particle size increases, and ranges from 44% and 80% across its classification limits. Since the AAC classifies particles independent of their charge state, its transmission efficiency is 2.6–5.1 times higher than a DMA for the same particle size. However, the AAC transfer function width deviates greater from theory than the DMA. While preliminary results indicate this additional broadening may be due to a classifier flow effect, additional research is required to validate this hypothesis.
The AAC transfer function characterization and deconvolution were validated by comparing the size distribution measured with an AAC-CPC system against parallel measurements taken with SMPS, CPC, and ELPI. It was determined that both the SMPS multiple-charge correction and AAC transfer function characterization must be considered to measure an aerosol size distribution accurately. Agreement between the corrected measured size distributions was within 6.6% for all lognormal distribution parameters measured (CMD, GSD, and ). The aerodynamic size distribution measured by the ELPI also agreed within 22.4% of the AAC low- and high-flow measurements. The effects of changing classifier conditions on the particle selected was also investigated and found to be small (<1.5%) within the AAC operating range.
Acknowledgments
This research would not have been possible without the support from Cambustion Ltd, The Rt. Hon. Sir Winston S. Churchill Society of Edmonton and C-FER Technologies.
UAST_1440063_Supplemental_File.zip
Download Zip (371.8 KB)Notes
1 Such as an optical particle counter optimized for the size range of interest or an electrometer if the particle charge distribution is known.
References
- Agarwal, J. K., and Sem, G. J. (1980). Continuous Flow, Single-Particle-Counting Condensation Nucleus Counter. J. Aerosol Sci., 11(4):343–357.
- Baron, P. A. (1986). Calibration and Use of the Aerodynamic Particle Sizer (APS 3300). Aerosol Sci. Technol., 5(1):55–67.
- Bau, S., and Witschger, O. (2013). A Modular Tool for Analyzing Cascade Impactors Data to Improve Exposure Assessment to Airborne Nanomaterials. J. Phys.: Conf. Ser., 429(1):12002.
- Birmili, W., Stratmann, F., Wiedensohler, A., Covert, D., Russell, L. M., and Berg, O. (1997). Determination of Differential Mobility Analyzer Transfer Functions Using Identical Instruments in Series. Aerosol Sci. Technol., 27(2):215–223.
- Chen, B. T., Cheng, Y. S., and Yeh, H. C. (1990). A Study of Density Effect and Droplet Deformation in the TSI Aerodynamic Particle Sizer. Aerosol Sci. Technol., 12(2):278–285.
- DeCarlo, P. F., Slowik, J. G., Worsnop, D. R., Davidovits, P., and Jimenez, J. L. (2004). Particle Morphology and Density Characterization by Combined Mobility and Aerodynamic Diameter Measurements. Part 1: Theory. Aerosol Sci. Technol., 38(12):1185–1205.
- Finlay, W. H. (2001). The Mechanics of Inhaled Pharmaceutical Aerosols: An Introduction. San Diego: Academic Press.
- Fissan, H., Hummes, D., Stratmann, F., Büscher, P., Neumann, S., Pui, D. Y. H., and Chen, D. (1996). Experimental Comparison of Four Differential Mobility Analyzers for Nanometer Aerosol Measurements. Aerosol Sci. Technol., 24(1):1–13.
- Gopalakrishnan, R., McMurry, P. H., and Christopher J. Hogan, J. (2015). The Bipolar Diffusion Charging of Nanoparticles: A Review and Development of Approaches for Non-Spherical Particles. Aerosol Sci. Technol., 49(12):1181–1194.
- Gopalakrishnan, R., Thajudeen, T., Ouyang, H., and Hogan, C. J. (2013). The Unipolar Diffusion Charging Of Arbitrary Shaped Aerosol Particles. J. Aerosol Sci., 64(Supplement C):60–80.
- Gunn, R., and Woessner, R. H. (1956). Measurements of the Systematic Electrification of Aerosols. J. Colloid Sci., 11(3):254–259.
- He, M., and Dhaniyala, S. (2013). A Multiple Charging Correction Algorithm for Scanning Electrical Mobility Spectrometer Data. J. Aerosol Sci., 61:13–26.
- Hinds, W. C. (1999). Aerosol Technology: Properties, Behavior, and Measurement of Airborne Particles. 2nd ed. Hoboken, NJ: Wiley-Interscience Publication.
- Hummes, D., Neumann, S., Fissan, H., and Stratmann, F. (1996). Experimental Determination of the Transfer Function of a Differential Mobility Analyzer (DMA) in the Nanometer Size Range. Part. Part. Syst. Charact., 13(5):327–332.
- Järvinen, A., Aitomaa, M., Rostedt, A., Keskinen, J., and Yli-Ojanperä, J. (2014). Calibration of the New Electrical Low Pressure Impactor (ELPI+). J. Aerosol Sci., 69:150–159.
- Johnson, T. J., Symonds, J. P. R., and Olfert, J. S. (2013). Mass-Mobility Measurements Using a Centrifugal Particle Mass Analyzer and Differential Mobility Spectrometer. Aerosol Sci. Technol., 47(11):1215–1225.
- Karlsson, M. N. A., and Martinsson, B. G. (2003). Methods to Measure and Predict the Transfer Function Size Dependence of Individual {DMAs}. J. Aerosol Sci., 34(5):603–625.
- Keskinen, J., Pietarinen, K., and Lehtimäki, M. (1992). Electrical Low Pressure Impactor. J. Aerosol Sci., 23(4):353–360.
- Kim, J., Mulholland, G., Kukuck, S., and Pui, D. (2005). Slip Correction Measurements of Certified PSL Nanoparticles Using a Nanometer Differential Mobility Analyzer (nano-DMA) for Knudsen Number From 0.5 to 83. J. Res. Natl. Ins. Standards Technol., 110(1):31.
- Knutson, E. O., and Whitby, K. T. (1975). Aerosol Classification by Electric Mobility: Apparatus, Theory, and Applications. J. Aerosol Sci., 6(6):443–451.
- Kulkarni, P., Baron, P. A., and Willeke, K., editors (2011). Aerosol Measurement: Principles, Techniques, and Applications. 3rd ed. Hoboken, NJ: Wiley.
- Lall, A. A., and Friedlander, S. K. (2006). On-Line Measurement of Ultrafine Aggregate Surface Area and Volume Distributions by Electrical Mobility Analysis: I. Theoretical Analysis. J. Aerosol Sci., 37(3):260–271.
- Lall, A. A., Seipenbusch, M., Rong, W., and Friedlander, S. K. (2006). On-Line Measurement of Ultrafine Aggregate Surface Area and Volume Distributions by Electrical Mobility Analysis: II. Comparison of Measurements and Theory. J. Aerosol Sci., 37(3):272–282.
- Li, W., Li, L., and Chen, D.-R. (2006). Technical Note: A New Deconvolution Scheme for the Retrieval of True DMA Transfer Function from Tandem DMA Data. Aerosol Sci. Technol., 40(12):1052–1057.
- Martinsson, B. G., Karlsson, M. N. A., and Frank, G. (2001). Methodology to Estimate the Transfer Function of Individual Differential Mobility Analyzers. Aerosol Sci. Technol., 35(4):815–823.
- Olfert, J. S., and Collings, N. (2005). New Method for Particle Mass Classification - The Couette Centrifugal Particle Mass Analyzer. J. Aerosol Sci., 36(11):1338–1352.
- Ouf, F.-X., and Sillon, P. (2009). Charging Efficiency of the Electrical Low Pressure Impactor’s Corona Charger: Influence of the Fractal Morphology of Nanoparticle Aggregates and Uncertainty Analysis of Experimental Results. Aerosol Sci. Technol., 43(7):685–698.
- Stolzenburg, M. (1988). An Ultrafine Aerosol Size Distribution Measuring System, Ph.D. Dissertation. University of Minnesota, Ann Arbor.
- Stolzenburg, M. R., and McMurry, P. H. (2008). Equations Governing Single and Tandem DMA Configurations and a New Lognormal Approximation to the Transfer Function. Aerosol Sci. Technol., 42(6):421–432.
- Tavakoli, F., and Olfert, J. S. (2013). An Instrument for the Classification of Aerosols by Particle Relaxation Time: Theoretical Models of the Aerodynamic Aerosol Classifier. Aerosol Sci. Technol., 47(8):916–926.
- Tavakoli, F., Symonds, J. P. R., and Olfert, J. S. (2014). Generation of a Monodisperse Size-Classified Aerosol Independent of Particle Charge. Aerosol Sci. Technol., 48(3):i–iv.
- TSI (2007). Model 3775 Condensation Particle Counter: Operation and Service Manual Revision D.
- Wang, S. C., and Flagan, R. C. (1990). Scanning Electrical Mobility Spectrometer. Aerosol Sci. Technol., 13(2):230–240.
- Wiedensohler, A. (1988). An Approximation of the Bipolar Charge Distribution for Particles in the Submicron Size Range. J. Aerosol Sci., 19(3):387–389.
- Wilson, J. C., and Liu, B. Y. H. (1980). Aerodynamic Particle Size Measurement by Laser-Doppler Velocimetry. J. Aerosol Sci., 11(2):139–150.