ABSTRACT
Sensitivity of secondary organic aerosol (SOA) concentrations in the South Coast Air Basin (SoCAB) of California to nitrogen oxide (NOx) emission is simulated using gas-phase chemistry and gas-particle partitioning modules. These modules are implemented into a three-dimensional air quality model applied for high-pollution summer meteorology and 2008 emissions. To test sensitivity, NOx emissions in all locations and at all times are scaled by factors ranging from 0.1 to 10.0 in separate model runs. The basin-wide average SOA concentration exhibits a ‘turnover’ NOx emission multiplicative factor, above and below which the average SOA concentration decreases. For the entire SoCAB, this critical NOx emission factor is ∼0.3; while the magnitude of SOA concentrations changes with time, this peak value (∼0.2–0.3) appears to be relatively independent of the hour of the simulated day. When considering individual locations within the SoCAB, this peak factor shows a slightly broader range. Projected emissions for 2023 indicate a decrease in basin-average SOA concentration; the response at individual locations, however, can be either positive or negative, indicating the need for location-specific considerations. Ensembles of module simulations based on parameter values selected using efficient sampling techniques (Latin Hypercube method) are used to identify parameters to which SOA predictions are significantly sensitive. Total SOA predictions are most sensitive (in no particular order) to concentrations of O3, unsaturated species formed from the gas-phase oxidation of monoaromatic compounds, and substituted products from long-chain alkane oxidation. Secondary inorganic aerosol species, likely through influencing aerosol liquid water, control at least partially the formation of SOA upwind. In addition, the rate at which unsaturated bicyclic oxidation products of monoaromatic compounds are oxidized by hydroxyl radical impacts significantly SOA prediction. These findings emphasize the need for consideration of long-chain alkanes and monoaromatic species when designing emission control strategies.
© 2018 American Association for Aerosol Research
EDITOR:
1. Introduction
Atmospheric particulate matter (PM), also referred to as atmospheric aerosol, is an extensive environmental issue due to its enhanced concentrations in many parts of the globe and to its many detrimental impacts. A wealth of observations of ambient PM mass concentrations indicates that organic aerosol (OA) constitutes the largest fraction by mass of fine PM (that characterized by a diameter that does not exceed 2.5 micron) in many areas of the world (Hallquist et al. Citation2009). Atmospheric OA can be described as either primary, meaning that it was emitted directly to the atmosphere in the particle phase, or secondary, meaning that the constituent species was formed chemically in situ. Fieldwork and modeling studies have indicated that the bulk of atmospheric OA globally and in certain areas is likely to be secondary in nature (Kanakidou et al. Citation2005; Zhang et al. Citation2007; Jimenez et al. Citation2009).
The formation of secondary organic aerosol (SOA) depends strongly on a number of factors, which are best understood through a description of the SOA formation process. An atmospheric organic species is oxidized to form some suite of organic products. For a subset of these products, some fraction of the mass resides in a condensed phase, the extent of which depends on the product vapor pressure. The oxidation process can occur homogeneously in the gas phase, homogeneously in an aqueous phase, or heterogeneously. Factors that influence these phenomena include the concentrations of the reactants and meteorological parameters. For example, relative humidity (RH) influences the aerosol liquid water content (LWC) that provides an aqueous medium for reaction, temperature impacts both reaction rate constants and vapor pressures, and wind speed and direction influence the enhancement or dilution of species in a given location.
In addition, the concentrations of other chemical species indirectly impact SOA formation. In addition to RH, the presence of PM from sulfate and other secondary inorganic species determine LWC (Carlton and Turpin Citation2013; Hodas et al. Citation2014; Guo et al. Citation2015). The photolysis of ozone (O3) generates hydroxyl radical (OH), which initiates many relevant gas-phase, aqueous-phase, and heterogeneous oxidation processes (Seinfeld and Pandis Citation2006).
Nitrogen oxides (NOx = nitric oxide (NO) + nitrogen dioxide (NO2)) influence SOA formation in several ways. The interaction of NOx with volatile organic compounds (VOC) (that also serve as SOA precursors) in the presence of sunlight leads to the formation of tropospheric O3 (Seinfeld and Pandis Citation2006). Enhanced O3 leads to increased atmospheric oxidation capacity, which increases the rate at which SOA reactions occur.
Due to the peroxy radical chemistry that occurs after most VOC–OH reactions, NOx directly determines VOC oxidation product distributions through peroxy–NOx chemistry, which thus affects product vapor pressures and influences gas–condensed phase partitioning. For example, Song et al. (Citation2005) recognized the probable change in product distribution as a result of changes in NOx level in SOA generated from the oxidation of aromatic species. Further, Ng et al. (Citation2007) performed chamber experiments and observed that SOA yields for monoterpenes and isoprene decrease as NOx increases. Ng et al. (Citation2007) confirmed earlier findings by Presto et al. (Citation2005) for monoterpenes. However, Ng et al. (Citation2007) observed that SOA yields for sesquiterpenes increase substantially as NOx increases. Chamber experiments by Eddingsaas et al. (Citation2012) confirmed that the SOA yield for α-pinene oxidation decreases as NOx increases. However, they were able to show that use of highly acidic seed particles increases SOA yield relative to the use of mildly acidic seeds only at high NOx levels. Chan el al. (Citation2009) observed lower yields for SOA from polycyclic aromatic hydrocarbon oxidation under high-NOx conditions relative to those observed when oxidation occurred at low NOx levels. Loza et al. (Citation2014) observed flat or increasing SOA yields for long-chain alkanes as NOx increases. The chemical nature of the parent hydrocarbon and the nature of preexisting aerosol play crucial roles in determining the impact of NOx on the SOA yield.
A third mechanism by which NOx influences SOA formation is through formation of the nitrate radical (NO3). Without photolytic loss, NO3 tends to be enhanced at night after formation by the reaction between NO2 and O3. Certain VOCs, particularly those with unsaturated carbon–carbon double bonds, are susceptible to oxidation by NO3, with the products likely to form SOA (Ng et al. Citation2017). For example, field studies performed by Rollins et al. (Citation2012) in the city of Bakersfield, California showed that particulate organic nitrates increase with increases of NOx at night. Brown et al. (Citation2013) showed the probable importance of biogenic VOC–NO3 reactions leading to SOA in the urban nocturnal atmosphere above Houston, Texas.
The impacts of NOx and other parameters on SOA concentrations are highly non-linear, making experimental investigations of their relationships both difficult and time consuming. As such, modeling techniques are particularly useful for evaluating our understanding of SOA formation, including the response of SOA to variation in NOx levels and its sensitivity to other parameters. For example, Camredon et al. (Citation2007) coupled a gas-phase oxidation scheme with a thermodynamic condensation module to study the impact of NOx levels on SOA yield from the oxidation of 1-octane from first principles. They found that SOA yield increases for NOx levels less than 1 ppb. However, SOA yield decreases for NOx levels between 1 and 100 ppb. Three-dimensional modeling applications have considered the effect of NOx on SOA both globally and in specific regions (Tsigaridis and Kanakidou Citation2003; Megaritis et al. Citation2013; Pye et al. Citation2015; Zheng et al. Citation2015; Matsui and Koike Citation2016; Zhao et al. Citation2017) and from specific emission sources globally (Afshar-Mohajer and Henderson Citation2017).
The work described here focuses on application of modeling techniques. The modules are applied to simulate air quality in the South Coast Air Basin (SoCAB) of California. (See Figure S1 in the online supplementary information [SI].) Due to its history of poor air quality, the SoCAB has received much attention with regard to scientific research and regulation over the past few decades. Despite significant improvements in the air quality of the SoCAB, it is still considered one of the areas in the United States with the worst degradation of its air, particularly with respect to PM. As such, it is used as a model testbed for illustrating the sensitivity of SOA formation to chemical parameters. Over the past two decades, significant effort has been placed on simulation of SOA in the SoCAB, including source apportionment (Kleeman et al. Citation2007), determination of SOA dynamics (Vutukuru et al. Citation2006), generation of PM isopleths and a SOA reactivity scale (Nguyen and Dabdub Citation2002a; Carreras-Sospedra et al. Citation2005), and simulation of phase partitioning preference for SOA species (Chang et al. Citation2010). Other efforts focused on understanding the influence of aerosol aging on SOA formation (Jathar et al. Citation2016), specific mobile source impacts on SOA (Jathar et al. Citation2017), health impacts of SOA (Hu et al. Citation2017), and the importance of SOA during a given field campaign (Hayes et al. Citation2015). The current work focuses more exclusively on the sensitivity of SOA predictions to specific chemical parameters, including reaction rate coefficients and the concentrations of species including NOx, with a specific focus on the sensitivity as a function of space within the SoCAB and of time of day.
2. Methods
In this work, we focus on the SOA formation process associated with gas-phase oxidation of VOCs followed by gas-particle partitioning of the oxidation products. Because of the relatively dry nature of the SoCAB in the summer, the influence of LWC (and therefore aqueous-phase oxidation) in fine particles is thought to be less significant though not negligible (Chang et al. Citation2010), especially in locations for which atmospheric transport limits the importance of secondary PM. Focus is placed here on the chemical mechanism that describes gas-phase oxidation processes. The gas-particle partitioning module that determines how an oxidation product distributes itself among the phases, the host three-dimensional (3D) air quality model (AQM), and the relevant input parameters for the AQM associated with the simulations also are described. Despite evidence for the importance of organic compounds of intermediate volatility in SOA formation in the SoCAB (Jathar et al. Citation2017), this work focuses predominantly on SOA formation from the oxidation of aromatic species.
In addition to considering full 3D AQM simulations, a zero-dimensional (0D) version of the gas-phase mechanism and the phase-partitioning module was developed, allowing for the application of Latin Hypercube Sampling (LHS) (Rodriguez and Dabdub Citation2003) to assess the sensitivity of predicted SOA concentrations to a variety of parameters. While this 0D model allows time to proceed, the system does not have any physical dimensions and is not subject to transport mechanisms into or out of the domain.
2.1. Gas-phase chemical mechanism
No existing chemical mechanism is capable of predicting exactly the gas-phase chemistry associated with SOA formation, primarily due to the complexity of the associated pathways and the large number of species involved. However, the Caltech Atmospheric Chemistry Mechanism (CACM) (Griffin et al. Citation2002) seeks to balance chemical detail with computational demand and serves as the host chemical mechanism for this work. The CACM has been updated several times since its initial development (Griffin et al. Citation2005; Jordan et al. Citation2008; Xu et al. Citation2015; Dawson et al. Citation2016). The equations in this mechanism describe the time rate of change of concentrations of all relevant species; these are based on kinetic rate equations. For example, the loss rate of a model VOC compound (lumped to represent several different VOC so as to decrease computational burden) is determined by reaction rate constants, oxidant concentrations, and the concentration of the VOC itself. Similar expressions are derived for secondary, tertiary, etc. products and may include both loss and formation. The result is a highly coupled, non-linear set of hundreds of stiff ordinary differential equations that when solved provide time series of all chemical species in the system. It is important to note that purely inorganic, purely organic, and mixed inorganic-organic reactions are all considered in the mechanism.
The version used here is that described by Dawson et al. (Citation2016), which has a specific focus on aromatic gas-phase chemistry associated with toluene and m-xylene, as described by Xu et al. (Citation2015). In its current application, the mechanism describes 606 reactions, 200 stable chemical species (meaning that differential equations are solved to determine their concentrations), and 84 radical species for which pseudo-steady state is assumed (Dawson et al. Citation2016). Of the stable chemical species, 29 are primary VOC and 139 are secondary organic species. The mechanism assumes a constant mixing ratio of oxygen (O2) relative to nitrogen as well as a constant mixing ratio of methane.
2.2. Gas-particle partioning module
For the 139 secondary organic species described in Section 2.1, it must be considered whether some of their mass partitions to the condensed phase based on their volatility. Guidelines established by Pun et al. (Citation2002) and refined by Griffin et al. (Citation2005) and Dawson et al. (Citation2016) are used to determine the 55 species included in the gas-particle partitioning module. Based on similarity in functionality, source, and properties, these 55 compounds are lumped into 17 representative compounds described by a specific structure and resulting properties such as molecular weight, estimated vapor pressure, and estimated Henry's law constant. The concentrations of the 17 lumped partitioning compounds are fed to the partitioning module, along with other requisite information, including meteorological values and the concentrations of other species upon which the organic gas-particle partitioning depends.
Five of the lumped species that are included in the partitioning module come directly from oxidation of aromatic compounds in the updated version of CACM used. These are noted as C1–5 in this manuscript. Compound C1 represents furanone compounds that are formed from the oxidation of dicarbonyl compounds formed upon ring fragmentation of the parent aromatic compound, and compound C2 represents nitro-phenol compounds formed upon addition of hydroxyl and nitro functional groups to an aromatic ring. Like C1, compound C3 represents ring fragmentation products but in contrast are peroxy acyl compounds formed from aldehyde oxidation. Compound C4 represents aromatic ring fragmentation products that contain an epoxide group. Lastly, compound C5 represents bicyclic peroxide compounds that form after isomerization of peroxy radicals formed upon OH addition to the aromatic ring.
The Model to Predict the Multi-phase Partitioning of Organics (MPMPO) (Griffin et al. Citation2003) simulates the phase partitioning of the 17 SOA species by allowing a fraction of the mass to go into an organic liquid phase (other SOA species plus primary OA), to go into an aqueous phase (determined by LWC controlled by inorganic aerosol and RH), or to remain in the gas phase. The system is constrained by a mass balance, the input for which comes from the results of CACM combined with the lumping protocol. For the organic liquid phase, partitioning theory (Pankow Citation1994) drives the model; Henry's law drives aqueous-phase partitioning. SCAPE2 is used to simulate inorganic aerosol formation and LWC (Meng et al. Citation1998). Instantaneous equilibrium is assumed for organics, and the resulting series of coupled equations is solved numerically (Griffin et al. Citation2003), providing the phase distribution. The mass remaining in the gas-phase is redistributed to the original CACM species to allow further participation in gas-phase chemistry. This methodology inherently does not consider particle-phase reactions, but this is overcome using vapor pressure adjustments to match chamber experimental data (Xu et al. Citation2015). The combined and updated CACM-MPMPO (termed aroCACM/MPMPO 1.0) as described and implemented by Dawson et al. (Citation2016) is used here. This model assumes that primary OA is non-volatile and conservative. The model simulates size distributions using six size-bins in the fine aerosol mode, and it is assumed that SOA forms only in the fine mode. The SOA concentrations presented in this work represent the sum over the six fine aerosol size bins.
2.3. Three-dimensional air quality model
The host 3D Eulerian AQM is the UCI-CIT AQM. In the UCI-CIT model, the SoCAB is described by an 80 × 30 grid (only a subset of which are used), with each cell being 5 × 5 km2. Five vertical layers extend to a top height of 1100 m. In each cell, the time rate of change of the concentration of each species is determined by emission, transport among cells in both the horizontal and vertical directions, deposition, and chemistry (both consumption and generation, including phase partitioning). The coupled, non-linear set of simultaneous differential equations (adjusted advective-diffusion equations for each species) is solved using operator splitting and a variety of techniques for numerical integration as described in detail in Nguyen and Dabdub (Citation2001; Citation2002b). Figure S2 in the SI provides a schematic of the interaction between CACM, MPMPO, and other modules in the UCI-CIT AQM; an additional description of CACM/MPMPO also appears in the SI. The evaluation of CACM/MPMPO has been described in detail in previous publications. This includes both 0D (Xu et al. Citation2015) and 3D applications (Griffin et al. Citation2002; Pun et al. Citation2002; Griffin et al. Citation2005; Dawson et al. Citation2016).
Simulations (described subsequently) include 72 h of simulated time, but the inputs are not date specific. Thus, results are considered representative of a time of peak photochemical activity in the SoCAB. All results shown represent model output for the second simulation day in order to minimize the impact of prescribed initial conditions.
2.4. Cases for simulation
The base case for the model runs is a simulation of three summer days. For this simulation, requisite input information includes lateral boundary conditions (BCs), initial conditions (ICs), meteorological parameters, and emission inventories. The BCs and ICs and meteorological inputs must be grid-location and time specific. Meteorology includes temperature, RH, wind speed, wind direction, and radiation parameters that allow the determination of photolysis rate constants. The corresponding input files were developed as described in Carreras-Sospedra et al. (Citation2010).
The emissions inventory also must be both grid-location and time specific. The inventory used (current for 2008) was developed based on the 2012 Air Quality Management Plan (AQMP) from the South Coast Air Quality Management District (SCAQMD, Citation2013). For the sake of the sensitivity analysis, emissions of NOx were varied across the entire spatial domain and the entire simulation time by a constant factor for each model sensitivity run, while holding all other inputs the same between runs. For the NOx sensitivity studies, it should be noted that the emissions of NO and NO2 were altered by the same amount, meaning that the NO/NO2 ratio in the emissions was not varied. Following the methodology described in Dawson et al. (Citation2016), high SOA-yield aromatics from the 2012 AQMP were assumed to be 30% toluene and 30% m-xylene with the remaining 40% following the original CACM scheme for high SOA-yield aromatics. In addition, a projected 2023 emissions inventory from SCAQMD was utilized to investigate predicted decadal-scale changes in simulated SOA in the SoCAB.
For 3D simulations, results are shown both for SoCAB-wide averages, as well as for individual locations. Three locations within the SoCAB are considered based on different emissions characteristics and extent of influence of secondary chemistry. These include Central Los Angeles (CELA) (upwind, less subject to secondary chemistry due to transport), Riverside (RIVR) (downwind, more influenced by secondary chemistry), and Pomona (POMA) (intermediate location). These were selected because the progression from upwind to downwind has been shown to be important in understanding SOA formation in the SoCAB (Chang et al. Citation2010).
2.5. Latin hypercube sampling (LHS)
Beyond chemical sensitivity to NOx and beyond predictions of SOA based on future emissions inventories, it is important to consider that other factors influence the predicted levels of SOA throughout the SoCAB. The aroCACM/MPMPO 1.0 module is an ideal tool with which to investigate these factors. For each input parameter, a probability distribution needs to be known or assumed. Monte Carlo simulation randomly selects values of the input parameters from within the appropriate probability distributions and considers the collective response of the dependent variable (in this case, SOA concentrations) to determine sensitivity. One negative aspect of the traditional Monte Carlo approach is the enormous computational burden associated with the large required number of sampled cases. Selection of a small subset of the individual cases from the entire suite of cases available to characterize the entire system would minimize this burden. The LHS is a statistical technique to generate near-random samples of a given set of parameters from a multidimensional distribution. McKay et al. (Citation1979) were the first to demonstrate the efficiency of this sampling method. Eglājs and Audze (Citation1977) and Iman et al. (Citation1981) proposed equivalent techniques. Previous researchers have implemented LHS on computational studies related to air quality modeling (Bergin et al. Citation1999; Martien et al. Citation2003; Rodriguez and Dabdub Citation2003; Lee and Russell Citation2007; Beddows et al. Citation2017).
For the LHS technique, a 0D version of aroCACM/MPMPO 1.0 is used. However, for the initial conditions in the 0D applications, the model outputs from the UCI-CIT base case run at 2:00PM, midnight, and 6:00 AM (local time) on the second simulation day are extracted. Three locations are again considered to investigate spatial dependence. In this case, CELA, RIVR, and Long Beach (LGBH, characterized by a mix of primary and secondary influence) are used. For each location and each starting time, 10,000 model runs (2 h of simulated time) are processed in 0D mode.
The parameters varied include initial mixing ratios of all species present, calculated Henry's Law constants and vapor pressures of SOA species, and reaction rate constants associated with toluene and m-xylene oxidation, whose oxidation products account for the bulk of the modeled SOA (Dawson et al. Citation2016). To approximate the sensitivity of the model output to the various input parameters, we adopt a form of the equation typically used to propagate model uncertainties using LHS that we relabel as the sensitivity :
[1] where
is the sensitivity of species i to input parameter l,
is the nominal value of parameter l,
is the variance of parameter l,
is the number of parameters varied as part of the LHS simulations, and
is the time-averaged, normalized regression coefficient for species i and parameter l, as described in Rodriguez and Dabdub (Citation2003). The final term is included to retain the sign of the correlation. We assume log-normal probability distributions for each parameter l with a geometric standard deviation of 0.2. It is important to note that this distribution is not meant to represent the uncertainty of any particular parameter l, and the results of the LHS analysis here are used solely to interpret qualitatively the model results by identifying which species and reaction pathways likely contribute most to SOA formation under various model conditions.
3. Results and discussion
3.1. SOA response to NOx emissions
Basin-wide, daily average SOA concentrations as a function of scaling of NOx emissions across all time and space are exhibited in . Similar to changes in O3 production rate (Kleinman et al. Citation1997), a maximum is observed in the simulated SOA concentration versus NOx level. The steps in the scaling are equally divided between increases and decreases by a factor of 10. That is, the scaling factor of 1.0 is the base case, and runs are made at equal log-based steps between 0.1 and 10.0. Based on the total of 11 runs, a maximum average SOA of approximately 0.7 μg m−3 is observed at a scaling factor of 0.28. This factor is defined as the turnover point, but it should be noted that this is the maximum SOA concentration simulated for the NOx scaling factors considered. It is possible that a scaling factor not considered actually would lead to an even larger predicted SOA concentration. Increasing NOx leads to decreases in SOA (10% relative to the base case factor of 1 at a factor of 1.3 increase in NOx emission), though increasing NOx emission could not realistically be considered for a control strategy. Within the scale of the results shown in , control of NOx by more than a factor of 10 would be required in order to see improvement in SOA concentrations based solely on NOx control. Still, decreasing NOx emissions by a factor of 10 (without considering any simultaneous VOC control) leads to an increase in predicted SOA of 24%. In addition to the overall level of simulated SOA, the NOx level impacts the relative contribution of species to the total simulated SOA.
Figure 1. Left: Domain-wide 24-h average (second model day) SOA concentrations as a function of NOx emissions scaling factors. Specified lumped SOA species C1–C5 represent newly added aromatic degradation products as described by Dawson et al. (Citation2016) (furanones, nitro-phenols, peroxy nitrates, epoxides, and peroxy-bicyclic rings, respectively). Other SOA encompasses all other SOA species from previous versions of CACM/MPMPO, including small amounts from monoaromatic species and all other VOCs. Bars on the right indicate base case 2008 and 2023 domain-wide 24-h average concentrations. Right: As in left but expressed as fraction of total domain-wide 24-h average SOA concentrations.
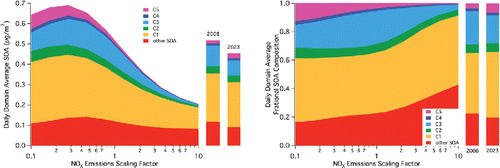
The contribution of two non-linear phenomena explains the results shown in . First, up to a certain point, decreasing NOx emissions in an urban area is expected to lead to an increase in O3 formation because decreasing NOx allows more OH to react with VOCs in the O3 formation process. This includes a positive feedback on OH, as O3 photolysis is a major pathway to OH formation. Beyond this peak, as NOx levels get smaller, hydrogen oxide (HOx = OH plus hydroperoxy radicals (HO2)) self-reaction pathways dominate such that OH oxidation of VOCs is limited. In general, this relates to the ability of the OH radical to initiate consumption of VOCs. Such simulated results for this episode are indicated in Figures S3 and S4 in the SI. It should be noted that the critical inflection point in O3 levels occurs at a higher scaling factor than is simulated for SOA, both for conditions at 2:00PM local time and across the day-long period.
Once the initial VOC oxidation occurs, the second phenomenon contributes to the NOx impact on SOA through altering the product distribution. Upon oxidation, VOCs generally form organic peroxy radicals (RO2) and/or HO2. At high NOx levels, RO2 preferentially reacts with NO. However, at decreased NOx levels, RO2 will react with HO2 or other RO2. For many VOCs, including monoaromatics, the product distribution under lower NOx conditions (for example, peroxides from HO2-RO2 or RO2-RO2 reactions) favors partitioning to the condensed phase (Song et al. Citation2005; Lane et al. Citation2008; Chan et al. Citation2010). This shift in product distribution at least partially explains the shift in inflection point values when comparing O3 and SOA responses to changes in NOx emissions.
The competition between these phenomena can be further elucidated by considering the specific lumped compounds that change most significantly between the base case and the case in which NOx emissions are scaled by a factor of 0.28. The two lumped compounds that show the largest increase between the two cases are C1 (furanones) and C5 (peroxy-bicyclic rings). On an absolute scale, the increase is largest for C1 (just under approximately 0.1 μg m−3), where that of C5 is approximately half that value. However, on a relative scale (compared to its base case), the increase in C5 is much larger (approximately a factor of 5). Both C1 and C5 are formed from the degradation of monoaromatic compounds, through OH addition to the aromatic ring, addition of O2, isomerization to form a bicyclic compound, and addition of a second O2. It is the fate of this second resulting RO2 that leads to either C1or C5. Reaction with NO initiates a chain that leads to C1; reaction with HO2 or other RO2 leads to C5. Thus, more C5 would be expected if only NOx level were considered. Because of the change in oxidation capacity, however, an increase in the “high NOx” furanone pathway is observed despite the decrease in NOx. This underscores that NOx control may have counteracting effects on O3 and SOA.
In addition to the domain-wide considerations discussed with respect to , it is important to consider the behavior at individual locations throughout the SoCAB because differences in emissions and meteorology between grid locations (as well as what is upwind of each grid cell) determine predicted SOA response to changes in NOx. show plots similar to except they exhibit the daily average SOA concentrations for a specific grid cell containing the named city.
Figure 2. 24-h average (second model day) SOA concentrations and relative species contribution as a function of NOx emissions scaling factors in the grid cell for Central Los Angeles (CELA). Notation as in .
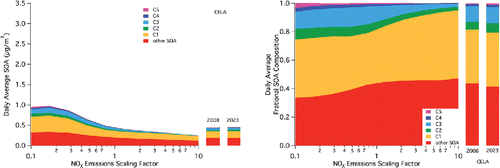
The CELA site () is influenced to a significant extent by mobile sources due to its proximity to the intersections of multiple freeways in the SoCAB; it is also an upwind site as it is located on the western side of the basin (predominant wind direction is westerly). As such, simulated SOA concentrations in CELA are smaller relative to downwind locations due to the lack of chemical aging at this location. However, the SOA concentrations in CELA are larger than the basin-wide averages due to inclusion of grid cells on the edges of the domain in the average calculation. The SOA in CELA appears in to show relatively little response to changes in NOx emission; however, this is because the scales in are kept the same to show the relative importance of SOA at the three sites. The relative contributions indicate changes in the chemical constituents of the simulated SOA. An overturn of the predicted average SOA with decreasing NOx is observed at a scaling factor of 0.17. An increase of 117% is observed in SOA when NOx emissions are decreased by a factor of 10. Again, despite the C1/furnanone route being a “high NOx” route, this species drives most of the increase, along with a smaller but significant increase in the C3/peroxy nitrate species. Thus, in the upwind location, the change in SOA appears to be driven predominantly by the change in oxidation capacity associated with decreasing NOx, as opposed to changes in product distribution.
In the intermediate POMA site (), the magnitude of simulated SOA concentrations is larger than in CELA (due to a combination of local emissions and increased processing times) and also larger than the average basin-wide values. The simulated daily average SOA concentration exhibits a slight turnover at a NOx emissions scale of 0.17. The increase between the base case and the maximum at 0.17 is 76%. Like in CELA, these increases are driven by increases in the C1/furanone and C3/peroxy nitrate species.
At the downwind RIVR site (), it is observed that the simulated average SOA concentration is larger than the upwind sites and the average basin-wide value. In RIVR, the peak in simulated SOA as a function of NOx appears at the scaling factor of 0.28, implying that the factor necessary to observe this turnover critical point increases as distance downwind within the SoCAB increases. The relative increase in SOA between the base case and the peak case in RIVR is 97%, larger than that simulated in POMA and similar to that observed in CELA. This increase is driven by increases in all species, including those mentioned previously (C1/furnanone and C3/peroxy nitrate) and those in which smaller impacts were seen upwind (C2/nitrophenols, C4/epoxides, and C5/bicyclic-peroxy compounds, as well as other SOA). Because increases in all of the species are observed, including “low NOx” processes such as that associated with the C5/bicyclic-peroxy compounds, it appears that the changes are driven by both the impact on oxidation capacity and the impact on product distribution. It should be stressed that a major metropolitan area such as the SoCAB would be considered “high NOx” even at a scaling factor of 0.28 across the basin and across time. Thus, it is stressed that “low NOx” products appear important, even under “high NOx” conditions. These also could be more important downwind as the fraction of NOx converted into other forms of active nitrogen (that is, alkyl nitrates, nitric acid, etc.) increases through photochemical processing.
As the basin-wide average masks important findings at individual sites within the SoCAB, the daily average also can mask important findings on time scales shorter than a day. As such, the response of basin-wide average SOA concentrations to basin-wide NOx scaling at specific hours is considered. As an example, simulated basin-wide SOA concentrations for the last hour of the second simulated day are shown in . This time was selected as it exhibited the largest average basin-wide SOA concentration (∼1.0 μg m−3 at a NOx scale factor of 0.28) while considering all NOx scaling factors and all hours of the second simulated day. The resulting increase in simulated SOA during the last hour (28% relative to the base case during the last hour) when decreasing NOx by 72% was driven by increases in all of the SOA species, including C1-C5 and other SOA. This indicates that the change is caused by both oxidation capacity and product distribution.
All simulated basin-wide average SOA concentrations are shown as a function of NOx scale factor for each hour of the second simulated day in the SI (Figures S5–S28). When considering these supplemental figures, it is possible to discern several important diurnal profiles. For example, when considering the base case, the diurnal profile of basin-wide predicted SOA concentration emphasizes that SOA concentrations are predicted to be highest at night despite photochemistry peaking during the day. This profile is possible under the given meteorological and emissions scenarios because some nocturnal chemistry is expected to lead to SOA formation. In addition, lower temperatures at night favor partitioning to the condensed phase, and boundary layer dynamics tend to dilute concentrations during the day and enhance concentrations at night.
The critical NOx scaling factor (below which predicted basin-wide average SOA concentrations begin to decrease again) varies by hour. Between simulation hours 10 and 14 on the second model day, this value is 0.17, while for the remainder of the day, the value is 0.28, which is the same as the basin-wide average, as well as that calculated for the downwind cells. Thus, the sensitivity of SOA to NOx depends on both space and time.
The temporal dependence of SOA sensitivity to NOx is further underscored when considering the SOA constituents that drive this sensitivity. At night, an increase in all monoaromatic-derived species (C1-C5) is observed as NOx decreases, emphasizing contributions to changes from shifts in both oxidation potential and product distribution. During the daylight hours, on average, species C2/nitrophenols and C4/epoxides become unimportant. It should be noted again that species C1/furanones, C3/nitro-peroxys, and C5/bicyclic-peroxy compounds represent the net effect between changes in product distribution and oxidation potential.
In addition to considering the impact of NOx scaling on SOA, particularly that from aromatic VOCs, it is possible to consider the impact of NOx scaling on primary OA and on inorganic PM species. Figure S29 shows the basin-wide daily (second model day) average concentrations of these species as a function of NOx scaling. This figure shows that total non-SOA PM is not impacted and that the only constituents whose concentrations are altered are aerosol inorganic nitrate and carbonate. As nitrate increases (with increasing NOx), carbonate correspondingly decreases in order to maintain electroneutrality in the fine inorganic/aqueous PM.
3.2. Future SOA
also allow the assessment of future SOA predictions. In , the projected emissions for 2023 show a basin-wide, daily average SOA concentration that decreases by approximately 12% relative to 2008. A slight increase in the C5/bicyclic-peroxy compounds is offset by decreases in all other species; this emphasizes that chemistry favored by “low NOx” conditions is countered by decreases in VOC emissions and by changes in oxidation potential associated with changes in VOC and NOx (as would be expected by an O3 isopleth). For reference, this approximate 12% decrease in SOA was associated with a 43% decrease in basin-wide NO emissions and a basin-wide 36% decrease in NO2 emission over the 15 years. Basin-wide VOC emissions over the same time period were decreased in the range of 10 to 42% depending on the VOC species considered (). Contrarily, the simulated O3 mixing ratios show little effect at 2:00 PM when considering emissions reductions between 2008 and 2023, and the daily SoCAB wide average mixing ratio shows a slight increase (Figures S3 and S4).
Figure 6. Comparison of changes in total daily emissions within the SoCAB between 2008 and 2023. Codes not defined elsewhere: CO (carbon monoxide), HCHO (formaldehyde), MEK (methyl ethyl ketone), KETO (higher ketones), BALD (benzaldehyde), PHEN (phenols), MGLY (methyl glyoxal), ALKL (short-chain alkanes), ALKM (medium-chain alkanes), ALKH (long-chain alkanes), ETHE (ethene), OLEL (short-chain alkenes), OLEH (long-chain alkenes), ISOP (isoprene), BIOL (low-SOA-yield monoterpenes), BIOH (high-SOA-yield monoterpenes), AROH (high-SOA-yield monoaromatics), AROL (low-SOA-yield monoaromatics), MEOH (methanol), ALD2 (higher aldehydes), SO2 (sulfur dioxide), SO3 (sulfur trioxide), HONO (nitrous acid), MCR (methacrolein), and ACID (carboxylic acids).
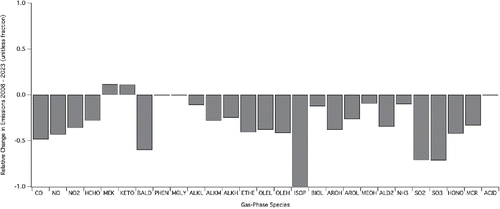
As with the response to NOx emissions, specific locations need to be considered for the 2023 projections as the results presented in may be skewed by extremes (either positive or negative) incorporated into the average. Due to the logic associated with the upwind-to-downwind progression and for consistency, the 2023 projections are included in . In CELA, only a small change (3%) in average SOA and no discernable change in its composition can be observed (), likely due to the transport of material prior to its reaction to form SOA, as discussed previously. In the intermediate location of POMA (), a desired decrease in SOA is achieved, with a decrease on the order of 10% driven by a change in other SOA, not a change in the C1-C5 species attributed to aromatic oxidation. Finally, in RIVR (), despite decreases in both VOCs and NOx across the basin, an increase in simulated SOA concentration of 7% is projected for 2023. This is driven by an increase in the C1/furnanone pathway and by a smaller increase in the C5/bicyclic-peroxy compound pathway, emphasizing that despite decreases in both NOx and VOCs, both “high NOx” and “low NOx” pathways remain relevant for projected SOA composition and concentration.
3.3. Latin hypercube sampling
Representative LHS results are shown in for CELA (2:00PM), LGBH (6:00 AM), and RIVR (12:00 AM). Similar results are shown in the supplemental Figures S30–S35 (same locations, two additional times of the day). and S30–35 show the estimated contribution to sensitivity of specific monoaromatic-derived SOA species (C1-C5) and total SOA (noted along the x-axis) to a variety of parameters (indicated by the shading). These parameters include reaction rate constants (noted by Ri), Henry's law constants of specific SOA species (HLC), and the initial concentrations (extracted from 3D results as described above) of a variety of species. RP or RPR species are those considered reactive, UR are those considered unreactive, and AP and PAN are those that include nitrate functionality. Specific parent VOCs that appear in and S30–35 include m-xylene (MXYL), toluene (TOLU), and PHEN. (See caption to .) Inorganic species include ammonia (NH3), NOx, HONO, hydrochloric acid (HCl), nitric acid (HNO3), and O3. Only parameters that have associated contributions to sensitivity that exceed 0.1 (absolute value) are included. Negative sensitivities indicate a decrease in concentration of the species noted along the x-axis when the parameter indicated by the shading increases.
Figure 7. Relative contributions of various parameters (indicated by the shading) to overall sensitivity of SOA constituents and total SOA (listed along the x-axis) based on LHS output for conditions at 2:00 PM local time in CELA (Central Los Angeles). Only those sensitivities with an absolute value greater than 0.1 are shown.
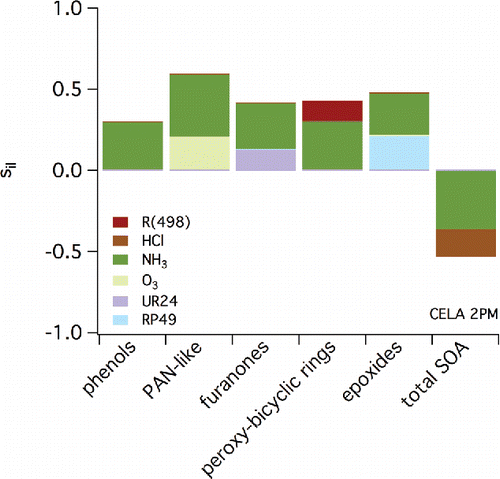
When considering the sensitivity of individual SOA model species to initial conditions, C1/furnanones are impacted most positively by UR24 (based on it appearing in eight of nine LHS output plots for the three locations and times considered). UR24 is a cyclic, furanone compound formed from the NO reaction with RO2 formed after initial OH oxidation of an aromatic. Similarly, the sensitivity of modeled C2/phenols SOA is positively related to the mixing ratios of UR22, which is a dinitrophenol compound derived from oxidation of gas-phase phenols. A mechanistic argument can be used to explain the negative sensitivity of C3/PAN-like species to NO levels. Upon degradation, products that result from monoaromatic oxidation chemistry can lead to the formation of peroxy acetyl radicals. If NO levels increase, these radicals are more likely to react with NO to form NO2, carbon dioxide, and a new RO2; this process will limit the reaction of NO2 with the peroxy acetyl radicals to form the PAN-like compounds. The sensitivity of C3/PAN-like species to NO is strongest at 6:00 AM when NO mixing ratios are expected to be high due to motor vehicular activities associated with rush hour. The concentrations of C4/epoxide species are linked directly to the sensitivity of specific SOA constituent RP49, which is a precursor to the epoxide products contained in group C4. Reaction 498 (shown as impacting C5/bicyclic-peroxy compound SOA) represents the rate at which isomerization occurs in RO2 formed after OH addition to aromatic rings; this chemistry was highlighted when considering “high NOx” versus “low NOx” chemistry previously. The C5/bicyclic-peroxy compound species also is highly sensitive to the initial concentration of species AP13, which represents a nitrate formed from the bicyclic radicals formed in Reaction 498. This is true across time and space, indicating the overall importance of this pathway in determining SOA concentrations across the SoCAB.
In addition to the sensitivity of specific SOA constituents shown in , the sensitivity of the total simulated SOA can be investigated. The species AP12 also is shown to have a significant contribution to sensitivity associated with total SOA, especially overnight. This species is a hydroxy nitrate derived from ALKH; in the context of this study, it is included in the Other SOA category, which explains why it does not impact any sensitivity on the C1–C5 constituents. The contribution of the species to total SOA sensitivity highlights ALKH and other VOCs that have intermediate volatility as important precursors for SOA, confirming the findings of Jathar et al. (Citation2017). Similarly, species UR24 (included in C1) impacts total SOA.
At specific locations and times, inorganic species beyond NOx contribute to the sensitivity of total SOA. In LGBH at 6:00 AM, HONO is an important model species. This is likely because HONO in the early morning can serve as a NOx reservoir; when photolysis increases, HONO releases both OH and NO, causing a rapid increase in O3 and other secondary pollutant formation. Of significant interest is the sensitivity of total SOA (and specific SOA constituents) to NH3 in CELA at several times. Within aroCACM/MPMPO 1.0, there are no direct interactions between NH3 and organic species. However, the presence of NH3 and other secondary inorganic aerosol species will impact directly the aerosol LWC, which then influences the ability of SOA species to partition to the aqueous phase. The NH3 also determines the acidity of the aqueous phase, which impacts whether a water-soluble SOA species dissociates. These findings support the importance of aerosol LWC in SOA partitioning, even in a dry area such as the SoCAB. The fact that this is most important in the CELA location corroborates the finding of Chang et al. (Citation2010) that partitioning of SOA species to the aqueous phase in the SoCAB is relatively more important in areas less influenced by secondary chemistry due to transport processes.
4. Conclusions
This study investigates the sensitivity of predicted SOA concentrations in the SoCAB to chemical parameters. Domain-wide NOx scaling factors across the temporal duration of the simulations indicate that a critical NOx scaling factor likely exists. Namely, unless NOx is reduced beyond a specific amount or combined with appropriate reductions in VOC emissions, an increase in predicted SOA is expected. When considering more refined spatial and temporal analysis, the critical scaling factor appears to vary with both space and time. The most important chemistry with respect to SOA formation is related to furnanone (high NOx pathway) and bicyclic-peroxy (low NOx pathway) products. This represents the net contribution of changes in oxidation potential and changes in product distribution.
For specific locations at the peak of photochemistry, at rush hour, and overnight, we have used LHS to consider the sensitivity of predicted SOA concentrations in the SoCAB to specific reaction rate constants, other chemical parameters, and concentrations of specific precursors. Results highlight the importance of understanding atmospheric degradation of monoaromatic compounds, especially as related to isomerization of radicals that controls ring-retention (nitro-phenols and bicyclic-peroxy compounds) versus ring-opening (furanones) products. The LHS findings support the influence of NOx compounds (and other forms of active nitrogen) on SOA. In upwind locations, inorganic PM precursors also appear to influence SOA.
The results shown here, indicating that an increase in NOx would likely decrease SOA in the SoCAB, are in contrast to the findings of Carlton et al. (Citation2010) who indicated more broadly that NOx emissions enhance average SOA concentrations. This is due to the high-NOx, low biogenic VOC atmosphere associated with the SoCAB. With respect to SOA, ALKH and monoaromatic compounds are of particular importance. The present work shows that consideration of temporal and spatial resolution of responses to changes in emissions is important.
UAST_1446507_supplemental_file.zip
Download Zip (664.2 KB)Acknowledgments
The authors wish to thank Jialu Xu for her assistance with the development of aroCACM/MPMPO 1.0 and its implementation into the UCI-CIT model.
Additional information
Funding
References
- Afshar-Mohajer, N., and Henderson, B. (2017). How Does a 10-Fold Pulse Increase of Aircraft-Related NOx Impact the Global Burdens of O3 and Secondary Organic Aerosol (SOA)? Air Qual. Atmos. Health., 10:929–938. doi:10.1007/s11869-017-0483-y.
- Beddows, A. V., Kitwiroon, N., Williams, M. L., and Beevers, S. D. (2017). Emulation and Sensitivity Analysis of the Community Multiscale Air Quality Model for a UK Ozone Pollution Episode. Environ. Sci. Technol., 51:6229–6236. doi:10.1021/acs.est.6b05873.
- Bergin, M. S., Noblet, G. S., Petrini, K., Dhieux, J. R., Milford, J. B., and Harley, R. A. (1999). Formal Uncertainty Analysis of a Lagrangian Photochemical Air Pollution Model. Environ. Sci. Technol., 33:1116–1126. doi:10.1021/es980749y.
- Brown, S. S., et al. (2013). Biogenic VOC Oxidation and Organic Aerosol Formation in an Urban Nocturnal Boundary Layer: Aircraft Vertical Profiles in Houston, TX. Atmos. Chem. Phys., 13:11317–11337. doi:10.5194/acp-13-11317-2013.
- Camredon, M., Aumont, B., Lee-Taylor, J., and Madronich, S. (2007). The SOA/VOC/NOx System: An Explicit Model of Secondary Organic Aerosol Formation. Atmos. Chem. Phys., 7:5599–5610. doi:10.5194/acp-7-5599-2007.
- Carlton, A. G., Pinder, R. W., Bhave, P. V., and Pouliot, G. A. (2010). To What Extent can Biogenic SOA be Controlled? Environ. Sci. Technol., 44:3376–3380. doi:10.1021/es903506b.
- Carlton, A. G., and Turpin, B. J. (2013). Particle Partitioning Potential of Organic Compounds is Highest in the Eastern US and Driven by Anthropogenic Water. Atmos. Chem. Phys., 13:10203–10214. doi:10.5194/acp-13-10203-2013.
- Carreras-Sospedra, M., Griffin, R. J., and Dabdub, D. (2005). Calculations of Incremental Secondary Organic Aerosol Reactivity. Environ. Sci. Technol., 39:1724–1730. doi:10.1021/es0495359.
- Carreras-Sospedra, M., Vutukuru, S., Brouwer, J., and Dabdub, D. (2010). Central Power Generation Versus Distributed Generation – An Air Quality Assessment in the South Coast Air Basin of California. Atmos. Environ., 44:3215–3223. doi:10.1016/j.atmosenv.2010.05.017.
- Chan, A. W. H., Chan, M. N., Surratt, J. D., Chhabra, P. S., Loza, C. L., Crounse, J. D., Yee, L. D., Flagan, R. C., Wennberg, P. O., and Seinfeld, J. H. (2010). Role of Aldehyde Chemistry and NOx Concentrations in Secondary Organic Aerosol Formation. Atmos. Chem. Phys., 10:7169–7188. doi:10.5194/acp-10-7169-2010.
- Chan, A. W. H., Kautzman, K. E., Chhabra, P. S., Surratt, J. D., Chan, M. N., Crounse, J. D., Kurten, A., Wennberg, P. O., Flagan, R. C., and Seinfeld, J. H. (2009). Secondary Organic Aerosol Formation From Photooxidation of Naphthalene and Alkylnaphthalenes: Implications for Oxidation of Intermediate Volatility Organic Compounds (IVOCs). Atmos. Chem. Phys., 9:3049–3060. doi:10.5194/acp-9-3049-2009.
- Chang, W. L., Griffin, R. J., and Dabdub, D. (2010). Partitioning Phase Preference for Secondary Organic Aerosol in an Urban Atmosphere. PNAS, 107:6705–6710. doi:10.1073/pnas.0911244107.
- Dawson, M. L., Xu, J. L., Griffin, R. J., Dabdub, D. (2016). Development of aroCACM/MPMPO 1.0: A Model to Simulate Secondary Organic Aerosol from Aromatic Precursors in Regional Models. Geosci. Model Develop., 9:2143–2151. doi:10.5194/gmd-9-2143-2016.
- Eddingsaas, N. C., Loza, C. L., Yee, L. D., Chan, M., Schilling, K. A., Chhabra, P. S., Seinfeld, J. H., and Wennberg, P. O. (2012). Alpha-pinene Photooxidation Under Controlled Chemical Conditions – Part 2: SOA Yield and Composition in Low- and High-NOx Environments. Atmos. Chem. Phys., 12:7413–7427. doi:10.5194/acp-12-7413-2012.
- Eglājs, V., and Audze, P. (1977). New Approach to the Design of Multifactor Experiments. Prob. Dynamics Strengths (in Russian), 35:104–107.
- Griffin, R. J., Dabdub, D., and Seinfeld, J. H. (2002). Secondary Organic Aerosol – 1. Atmospheric Chemical Mechanism for Production of Molecular Constituents. J. Geophys. Res., 107: doi: 10.1029/2001JD000541.
- Griffin, R. J., Dabdub, D., and Seinfeld, J. H. (2005). Development and Initial Evaluation of a Dynamic Species-Resolved Model for Gas-Phase Chemistry and Size-Resolved Gas/Particle Partitioning Associated with Secondary Organic Aerosol Formation. J. Geophys. Res., 109: doi: 10.1029/2004JD005219.
- Griffin, R. J., Nguyen, K., Dabdub, D., and Seinfeld, J. H. (2003). A Combined Hydrophobic-Hydrophilic Model for Predicting Secondary Organic Aerosol Formation. J. Atmos. Chem., 44:171–190. doi:10.1023/A:1022436813699.
- Guo, H., et al. (2015). Fine-Particle Water and pH in the Southeastern United States. Atmos. Chem. Phys., 15:5211–5228. doi:10.5194/acp-15-5211-2015.
- Hallquist, M., et al. (2009). The Formation, Properties and Impact of Secondary Organic Aerosol: Current and Emerging Issues. Atmos. Chem. Phys., 9:5155–5236. doi:10.5194/acp-9-5155-2009.
- Hayes, P. L., et al. (2015). Modeling the Formation and Aging of Secondary Organic Aerosols in Los Angeles during CalNex 2010. Atmos. Chem. Phys., 15:5773–5801. doi:10.5194/acp-15-5773-2015.
- Hodas, N., Sullivan, A. P., Skog, K., Keutsch, F. N., Collett, J. L., Decesari, S., Facchini, M. C., Carlton, A. G., Laaksonen, A., and Turpin, B. J. (2014). Aerosol Liquid Water Driven by Anthropogenic Nitrate: Implications for Lifetimes of Water-Soluble Organic Gases and Potential for Secondary Organic Aerosol Formation. Environ. Sci. Technol., 48:11127–11136. doi:10.1021/es5025096.
- Hu, J., Jathar, S., Zhang, H. L., Ying, Q., Chen, S. H., Cappa, C. D., and Kleeman, M. J. (2017). Long-term Particulate Matter Modeling for Health Effect Studies in California – Part 2: Concentrations and Sources of Ultrafine Organic Aerosols. Atmos. Chem. Phys., 17:5379–5391. doi:10.5194/acp-17-5379-2017.
- Iman, R. L., Helton, J. C., and Campbell, J. E. (1981). An Approach to Sensitivity Analysis of Computer Models, Part 1. Introduction, Input Variable Selection and Preliminary Variable Assessment. J. Quality Technol., 13:174–183. doi:10.1080/00224065.1981.11978748.
- Jathar, S. H., Cappa, C. D., Wexler, A. S., Seinfeld, J. H., and Kleeman, M. J. (2016). Simulating Secondary Organic Aerosol in a Regional Air Quality Model Using the Statistical Oxidation Model – Part 1: Assessing the Influence of Constrained Multi-Generational Aging. Atmos. Chem. Phys., 16:2309–2322. doi:10.5194/acp-16-2309-2016.
- Jathar, S. H., Woody, M., Pye, H. O. T., Baker, K. R., and Robinson, A. L. (2017). Chemical Transport Model Simulations of Organic Aerosols in Southern California: Model Evaluation and Gasoline and Diesel Source Contributions. Atmos. Chem. Phys., 17:4305–4318. doi:10.5194/acp-17-4305-2017.
- Jimenez, J. L., et al. (2009). Evolution of Organic Aerosols in the Atmosphere. Science, 326:1525–1529. doi:10.1126/science.1180353.
- Jordan, C. E., Ziemann, P. J., Griffin, R. J., Lim, Y. B., Atkinson, R., and Arey, J. (2008). Modeling SOA Formation from OH Reactions with C8-C17 n-alkanes. Atmos. Environ., 42:8015–8026. doi:10.1016/j.atmosenv.2008.06.017.
- Kanakidou, M., et al. (2005). Organic Aerosol and Global Climate Modelling: A Review. Atmos. Chem. Phys., 5:1053–1123. doi:10.5194/acp-5-1053-2005.
- Kleeman, M. J., Ying, Q., Lu, J., Mysliewic, M. J., Griffin, R. J., Chen, J. J., and Clegg, S. (2007). Source Apportionment of Secondary Organic Aerosol During a Severe Photochemical Smog Episode. Atmos. Environ., 41:576–591. doi:10.1016/j.atmosenv.2006.08.042.
- Kleinman, L. I., Daum, P. H., Lee, J. H., Lee, Y. N., Nunnermacker, L. J., Springston, S. R., Newman, L., Weinstein-Lloyd, J., and Sillman, S. (1997). Dependence of Ozone Production on NO and Hydrocarbons in the Troposphere. Geophys. Res. Lett., 24:2299–2302. doi:10.1029/97GL02279.
- Lane, T. E., Donahue, N. M., and Pandis, S. N. (2008). Effect of NOx on Secondary Organic Aerosol Concentrations. Environ. Sci. Technol., 42:6022–6027. doi:10.1021/es703225a.
- Lee, S., and Russell, A. G. (2007). Estimating Uncertainties and Uncertainty Contributors of CMB PM2.5 Source Apportionment Results. Atmos. Environ., 40:9616–9624. doi:10.1016/j.atmosenv.2007.08.022.
- Loza, C. L., et al. (2014). Secondary Organic Aerosol Yields of 12-Carbon Alkanes. Atmos. Chem. Phys., 14:1423–1439. doi:10.5194/acp-14-1423-2014.
- Martien, P. T., Harley, R. A., Milford, J. B., and Russell, A. G. (2003). Evaluation of Incremental Reactivity and Its Uncertainty in Southern California. Environ. Sci. Technol., 37:1598–1608. doi:10.1021/es026174t.
- Matsui, H., and Koike, M. (2016). Enhancement of Aerosol Responses to Changes in Emissions Over East Asia by Gas-Oxidant-Aerosol Coupling and Detailed Aerosol Processes. J. Geophys. Res., 121:7161–7171. doi: 10.1002/2015JD024671.
- McKay, M. D., Beckman, R. J., and Conover, W. J. (1979). A Comparison of Three Methods for Selecting Values of Input Variables in the Analysis of Output From a Computer Code. Technometrics, 21:239–245.
- Megaritis, A. G., Fountoukis, C., Charalampidis, P. E., Pilinis, C., and Pandis, S. N. (2013). Response of Fine Particulate Matter Concentrations to Changes of Emissions and Temperature in Europe. Atmos. Chem. Phys., 13:3423–3443. doi:10.5194/acp-13-3423-2013.
- Meng, Z., Dabdub, D., and Seinfeld, J. H. (1998). Size-Resolved and Chemically Resolved Model of Atmospheric Aerosol Dynamics. J. Geophys. Res., 103:3419–3435. doi:10.1029/97JD02796.
- Ng, N. L., et al. (2007). Effect of NOx Level on Secondary Organic Aerosol (SOA) Formation From the Photooxidation of Terpenes. Atmos. Chem. Phys., 17:2103–2162. doi:10.5194/acp-17-2103-2017.
- Ng, N. L., et al. (2017). Nitrate Radicals and Biogenic Volatile Organic Compounds: Oxidation, Mechanisms, and Organic Aerosol. Atmos. Chem. Phys., 17:2103–2162. doi:10.5194/acp-17-2103-2017.
- Nguyen, K., and Dabdub, D. (2001). Two-Level Time-Marching Scheme using Splines for Solving the Advection Equation. Atmos. Environ., 35:1627–1637. doi:10.1016/S1352-2310(00)00443-X.
- Nguyen, K., and Dabdub, D. (2002a). NOx and VOC Control and Its Effects on Formation of Aerosols. Aerosol Sci. Technol., 36:560–572. doi:10.1080/02786820252883801.
- Nguyen, K., and Dabdub, D. (2002b). Semi-Lagrangian Flux Scheme for the Solution of the Aerosol Condensation/Evaporation Equation. Aerosol Sci. Technol., 36:407–418. doi:10.1080/027868202753571232.
- Pankow, J. F. (1994). An Absorption Model of the Gas-Aerosol Partitioning Involved in the Formation of Secondary Organic Aerosol. Atmos. Environ., 28:189–193. doi:10.1016/1352-2310(94)90094-9.
- Presto, A. A., Huff Hartz, K. E., and Donahue, N. M. (2005). Secondary Organic Aerosol Production from Terpene Ozonolysis 2. Effect of NOx Concentration. Environ. Sci. Technol., 39:7046–7054. doi:10.1021/es050400s.
- Pun, B. K., Griffin, R. J., Seigneur, C., and Seinfeld, J. H. (2002). Secondary Organic Aerosol – 2. Thermodynamic Model for Gas/Particle Partitioning of Molecular Constituents. J. Geophys. Res., 107: doi:10.1029/2001JD000542.
- Pye, H. O., et al. (2015). Modeling the Current and Future Roles of Particulate Organic Nitrates in the Southeastern United States. Environ. Sci. Technol., 49:14195–14203. doi:10.1021/acs.est.5b03738.
- Rodriguez, M. A., and Dabdub, D. (2003). Monte Carlo Uncertainty and Sensitivity Analysis of the CACM Chemical Mechanism. J. Geophys. Res., 108: doi:10.1029/2002JD003281.
- Rollins, A. W., et al. (2012). Evidence for NOx Control Over Nighttime SOA Formation. Science, 337:1210–1212. doi:10.1126/science.1221520.
- SCAQMD. (2013). Final 2012 Air Quality Management Plan, Tech. rep., South Coast Air Quality Management District, Diamond Bar, California.
- Seinfeld, J. H., and Pandis, S. N. (2006). Atmospheric Chemistry and Physics: From Air Pollution to Climate Change. John Wiley and Sons, Hoboken, NJ.
- Song, C., Na, K. S., and Cocker III, D. R. (2005). Impact of the Hydrocarbon to NOx Ratio on Secondary Organic Aerosol Formation. Environ. Sci. Technol., 39:3143–3149. doi:10.1021/es0493244.
- Tsigaridis, K., and Kanakidou, M. (2003). Global Modelling of Secondary Organic Aerosol in the Troposphere: A Sensitivity Analysis. Atmos. Chem. Phys., 3:1849–1869. doi:10.5194/acp-3-1849-2003.
- Vutukuru, S., Griffin, R. J., and Dabdub, D. (2006). Simulation and Analysis of Secondary Organic Aerosol Dynamics in the South Coast Air Basin of California. J. Geophys. Res., 111: doi:10.1029/2005JD006139.
- Xu, J. L., Griffin, R. J., Liu, Y., Nakao, S., Cocker III, D. R. (2015). Simulated Impact of NOx on SOA Formation from Oxidation of Toluene and m-xylene. Atmos. Environ., 101:217–225. doi:10.1016/j.atmosenv.2014.11.008.
- Zhang, Q., et al. (2007). Ubiquity and Dominance of Oxygenated Species in Organic Aerosols in Anthropogenically-Influenced Northern Hemisphere Midlatitudes. Geophys. Res. Lett., 34: doi:10.1029/2007GL029979.
- Zhao, B., et al. (2017). A Modeling Study of the Nonlinear Response of Fine Particles to Air Pollutant Emissions in the Beijing-Tianjin-Hebei Region. Atmos. Chem. Phys., 17:12031–12050. doi:10.5194/acp-17-12031-2017.
- Zheng, Y., Unger, N., Hodzic, A., Emmons, L., Knote, C., Tilmes, S., Lamarque, J. F., and Yu, P. (2015). Limited Effect of Anthropogenic Nitrogen Oxides on Secondary Organic Aerosol Formation. Atmos. Chem. Phys., 15:13487–13506. doi:10.5194/acp-15-13487-2015.