ABSTRACT
Particle emissions from consumer-fused deposition modeling 3D printers have been reported previously; however, the complex processes leading to observed aerosols have not been investigated. We measured particle concentrations and size distributions between 7 nm and 25 μm emitted from a 3D printer under different conditions in an emission test chamber. The experimental data was combined with a moment lognormal aerosol dynamic model to better understand particle formation and subsequent evolution mechanisms. The model was based on particles being formed from nucleation of unknown semivolatile compounds emitted from the heated filament during printing, which evolve due to condensation of emitted vapors and coagulation, all within a small volume near the printer extruder nozzle. The model captured observed steady state particle number size distribution parameters (total number, geometric mean diameter and geometric standard deviation) with errors nominally within 20%. Model solutions provided a range of vapor generation rates, saturation vapor pressures and vapor condensation factors consistent with measured steady state particle concentrations and size distributions. Vapor generation rate was a crucial factor that was linked to printer extruder temperature and largely accounted for differences between filament material and brands. For the unknown condensing vapor species, saturation vapor pressures were in the range of 10−3 to 10−1 Pa. The model suggests particles could be removed by design of collection surfaces near the extruder tip.
Copyright © 2018 American Association for Aerosol Research
EDITOR:
1. Introduction
Fused deposition modeling (FDM) is the most common extrusion-based 3D printing technology in which a filament is heated to a semi-liquid state and deposited on a build plate in layers to construct a three-dimensional object (Zukas and Zukas Citation2015). FDM printers are popular with the general public due to their low-cost and ease of operation. These printers are found in small-scale manufacturing spaces, design offices, schools, libraries, and personal residences (Berman Citation2012; Gibson et al. Citation2010). It is known that commercial extrusion processing and degradation of thermoplastics produce both particles and volatile organic compounds (VOCs) (Adams et al. Citation1999; Hoff et al. Citation1982), some of which are toxic (Rutkowski and Levin Citation1986; Yoon et al. Citation2010). Concerns over potential hazardous exposures from 3D printer emissions have been raised since some are used in spaces not designed for manufacturing. Most concerning is susceptible population exposure, such as children. These concerns follow a similar pattern to those relating to photocopier and laser printer emissions (Khatri et al. Citation2013; Pirela et al. Citation2013). A number of studies have characterized emissions of gases and particles from FDM 3D printers to help assess exposure levels.
Studies have shown that the types and concentrations of VOCs emitted are linked to filament material. For example, considering just major emissions, ABS (acrylonitrile butadiene styrene) filaments emit styrene and ethylbenzene, PLA (polylactic acid) filaments emit lactide and methyl-methacrylate, and nylon filaments emit caprolactam (Azimi et al. Citation2016; Steinle Citation2016; Kim et al. Citation2015; Davis et al. Citation2016). Stefaniak et al. (Citation2017) found that a 3D printer gave a much lower emission rate than laser printers tested in the same chamber. Analysis suggested that some VOC concentrations from 3D printers can exceed recommended exposure limits (Azimi et al. Citation2017) resulting in potential adverse respiratory effects (Chan et al. Citation2017; House et al. Citation2017).
For particles, previous studies have reported a wide range of particle emissions that depended on filament material, printer type and operating conditions. Some of the variability between studies was also due to differences in testing conditions, measurement approaches and emission calculation methods. Maximum particle number concentrations measured during printing ranged from 103 to 106 particles/cm3 and depended on printer and filament properties (Azimi et al. Citation2016; Deng et al. Citation2016; Kim et al. Citation2015; Stabile et al. Citation2017; Steinle Citation2016; Stephens et al. Citation2013; Yi et al. Citation2016; Zhang et al. Citation2017; Zontek et al. Citation2017). Emitted particles were often less than 100 nm in diameter (Kim et al. Citation2015; Stabile et al. Citation2017; Steinle Citation2016; Stephens et al. Citation2013; Vance et al. Citation2017; Yi et al. Citation2016; Zhang et al. Citation2017). Average particle emission rates ranged from 107 to 1012 particles/min (Azimi et al. Citation2016; Kim et al. Citation2015; Stabile et al. Citation2017; Steinle Citation2016; Stephens et al. Citation2013; Yi et al. Citation2016), comparable to emissions from laser printers (He et al. Citation2007; Koivisto et al. Citation2010; Salthammer et al. Citation2012; Scungio et al. Citation2017), for which a standard test method and suggested emission thresholds have been developed (BAM Citation2012; UL Citation2013).
Zhang et al. (Citation2017) discussed the potential aerosol dynamic processes leading to particle formation and the observed evolution of the particle size distributions from FDM 3D printing; Vance et al. (Citation2017) also discussed the potential sources that might lead to particle formation based on their chemical analysis. However, the particle formation mechanism and processes involved have never been systematically investigated. Here we apply a method of moments model to simulate steady state particle concentrations recorded during printing. We perform a sensitivity analysis to investigate how each model parameter affects the number and size distribution of the emitted particles. Comparisons are then made between the steady state model solutions to data from specific printer runs to investigate the effect of extrusion nozzle temperature, filament brand (ABS from different manufacturers) and type of filament material (ABS vs. nylon) on the model parameters. The model provides conceptual insights on processes and factors leading to particle emissions from FDM 3D printers and to possible mitigation techniques.
2. Methods
2.1. Chamber experiment
Details of our systematic chamber measurements to characterize and identify the main variables affecting particle emissions from FDM 3D printers can be found in Zhang et al. (Citation2017). In these studies, experiments were carried out using a 1 m3 (1 × 1 × 1 m) stainless steel chamber with the printer in the center and sampling tubes extending approximately 10 cm away from the chamber inner walls. Particle and VOC free air at room temperature (23 ± 1°C) and low relative humidity (3.0% ± 0.2%) was supplied to the chamber at an air exchange rate of 1 hr−1 (16.7 L/min). The design of the chamber and clean air supply system followed the criteria of ASTM standard D6670 (ASTM Citation2013), ECMA-328 standard (ECMA Citation2015) and UL GREENGUARD Certified method (UL Citation2014). The procedures to measure the air exchange rate and wall losses, and to evaluate the chamber airtightness and air mixing characteristics, also followed the above standards. Particles were measured from at least 15 min before print started until 2-h after printing stopped. Aerosol measurement instrumentations included a condensation particle counter (CPC, TSI), a scanning mobility particle sizer (SMPS, TSI) and an optical particle counter (OPC, TSI) providing both total particle number concentrations and particle size distributions over the diameter range of 7 nm to 25 µm (7–300 nm for SMPS and 0.3–25 µm for OPC both with a time interval of 2 min).
Four contrasting experimental results were chosen from a large number of emission tests (n = 231) (Zhang et al. Citation2017) for model analysis. Conditions for each test are shown in . The four tests were selected to cover factors that influence emissions: filament brand, extrusion temperature and filament material. In all cases the filaments were 1.75 mm in diameter and all run on the same printer (printer A in Zhang et al. Citation2017, their Table 1) with a build plate temperature of 100°C and print time of ∼7 h printing the same object (a house). Although PLA is a commonly used material and its emissions have been reported (Zhang et al. Citation2017), it was not modeled in this study since particle concentrations from PLA never reached steady state during the printing periods on the tested printer. The method of moments model used is based on observed steady state concentrations.
Table 1. Specific print conditions for the selected tests.
2.2. Model description
The method of moments provides predictions of integrated particle characteristics with more computational efficiency than a fully coupled aerosol transport and dynamic model (Barrett and Webb Citation1998; Frenklach and Harris Citation1986; Wu and Biswas Citation1998; Yu et al. Citation2008). An advantage is that it tracks the lower-order moments of the distribution without knowing the details of the distribution, which is accomplished by arranging the moment governing equations in a closed form (McGraw Citation1997) so that they can be solved by computationally efficient numerical techniques. Assumptions must be made to achieve closed forms (Hulburt and Katz Citation1964). Here we assumed a lognormal aerosol size distribution (Yu and Liu Citation2016), as the shape of the measured distributions were close to lognormal. This increases the computational efficiency, but is less accurate (Seigneur et al. Citation1986). A lognormal aerosol moment model is useful to predict polydisperse particles properties in multi-dimensional multi-species aerosol flows (Brown et al. Citation2006). Our model is based on a lognormal moment method developed for simulating aerosol dynamics in aerosol reactors where the product aerosols are non-uniform (Biswas et al. Citation1989; Pratsinis Citation1988; Pratsinis et al. Citation1986). This model has been shown to be able to capture the particle size distribution characteristics through comparisons to known exact solutions for certain limiting cases. The method is applicable for modeling aerosol dynamics in complex systems when detailed particle distribution information is not required (Pratsinis Citation1988), which is the case for 3D printer particle emissions since the integral properties of the particles (i.e., total particle concentration and mean particle size) are important when considering emission levels and standards.
2.2.1. Control volume
The model was applied to a control volume where the vapors were emitted and aerosol dynamic processes of vapor nucleation resulting in new particle formation, vapor condensation onto existing particles and particle–particle interactions through coagulation were assumed to happen simultaneously. Outside of this control volume, it was assumed that the particles were simply diluted into the whole chamber domain without further aerosol dynamic processes occurring. Air was assumed to be advected through the control volume by the cooling fan attached to the printer extruder assembly.
The model assumed vapors are emitted from the hot filament, which includes the filament immediately exiting the extruder nozzle and the filament that was just recently deposited on the heated build plate or object being constructed. Therefore, the dimensions of the control volume were determined by the amount of filament recently extruded and expressed by a number of factors (). The width (b) was arbitrary set at 0.1 cm, which was approximately the width of the filament being extruded from the nozzle. The length of the control volume (x) was the effective length of the extruded filament. In the following study, we set an arbitrary value (50 cm) to simplify the model (i.e., eliminate a variable). With a filament diameter of 0.1 cm and length of 50 cm, the bottom of the control volume was 5 cm2, which was modeled to consist of recently extruded filament that is emitting vapors (e.g., this would be equivalent to printing a 2.23 by 2.23 cm square plane that emits vapors at the bottom of the control volume). In addition, we assumed the vapor emissions to be constant, which was reasonable given the small area and time to print compared to the overall printing period (i.e., emissions not effected by print shape, cooling of filament, etc.). The height of the control volume (hav) was calculated as a function of the length of the control volume and speed of air forced past the extruder tip by the cooling fan (see the online supplementary information [SI] Section S1 for equations). The simulation time was the time for vapor molecules to diffuse from the build plate (or layers) to the upper boundary layer (see the SI for equations).
Figure 1. Schematic of the control volume located just below the 3D printer extrusion nozzle and aerosol dynamic processes that are modeled within the control volume. The variable x is the length of the control volume defined by the effective length of extruded filament; hav is the height of the control volume; b is the width of the control volume, which is set at 1 mm.
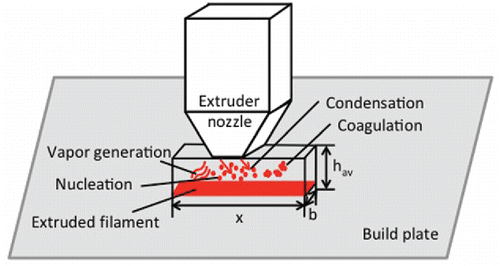
2.2.2. The lognormal moment model
We focused on the particle formation and growth including nucleation, condensation/evaporation and coagulation within the control volume. The following simplifying assumptions were made: (1) There were no external processes at or across the boundaries of the control volume (Biswas et al. Citation1989). (2) Particle losses to the chamber surfaces were neglected since loss rates were factors of 10−4 – 10−2 the typical emission rates; the loss coefficients due to deposition on surfaces were generally less than 10−4 s−1 calculated from the post-printing exponential decay curves in chamber experiments, while the calculated average particle emission rates (PERs, see Zhang et al. Citation2017) were 107 to 1011 #/s. (3) Particles were chemically homogeneous and no chemical reactions that would change the vapor properties over time were considered; i.e., the semivolatile gases that condense to form new particles or add mass to pre-existing particles were directly emitted from the heated filament, or if formed in the gas phase the reactions were rapid and the product concentrations directly proportional to emitted parent VOCs. (4) The temperature in the control volume was uniform, constant and equal to the average between the chamber (ambient) temperature and the extruder nozzle temperature.
In this study, the lognormal method of moments was used to solve the general dynamic equation (GDE) for aerosol processes, including new particle formation (NPF), particle growth by condensation (or shrinkage by evaporation) and coagulation (Friedlander Citation2000) by converting the GDE to closed form expressions for the moments (see SI section S2 for details). The key parameters of a lognormal distribution (total number concentration [N], geometric mean diameter [Dpg] and geometric standard deviation [σg]) are related to the first three moments of the distribution. The governing differential equations for the lognormal volume moment model were written in a dimensionless form in terms of moment change rates (Biswas et al. Citation1989; Pratsinis Citation1988). Here we only show the key equations and parameters, detailed explanation of the equations and the variables can be found in the SI.
The 0th moment (i.e., total particle number concentration, N) is affected by nucleation and coagulation and its rate of change is[1] where N’ is the dimensionless particle number concentration (N); θ is the dimensionless residence time; I’ is the dimensionless nucleation rate, which is related to saturation ratio (S = P/Ps, where P is the partial vapor pressure and Ps is the saturation vapor pressure at a given temperature); ξ is the dimensionless coagulation coefficient, which is related to identifiers of the particle size distributions (Dpg and σg).
The first moment (i.e., particle volume concentration) is affected by nucleation and condensation, and its rate of change is[2] where V is the dimensionless aerosol volume concentration; k* is the number of monomers in the critical size nucleus; f is a condensation factor used to correct condensation coefficients for multiple-vapor effects; η is the dimensionless condensation coefficient and is related to particle size distributions.
The second aerosol volume moment is affected by nucleation, condensation and coagulation, and its rate of change is[3] where V2 is the dimensionless second aerosol volume moment;
and ζ are the dimensionless condensation and coagulation coefficients (associated with particle size distributions).
A vapor monomer balance is necessary to solve the governing equations since nucleation and condensation both relate to the properties of vapors (Ps, R and f).[4] where R’ is the dimensionless form of vapor generation rate (R).
The differential equations Equations [Equation1[1] ]–[Equation4
[4] ] were solved using the VODE solver in Python. In this study, due to the complexity of vapor components in the control volume, and because the specific chemical species forming particles were unknown, it was difficult to predict the particle concentrations and size distributions from the initial conditions. Instead, the model can provide a possible range of parameters that could lead to the observed steady-state aerosol profiles, where particle number concentrations and size distributions remained relatively stable. In the following we report possible ranges of key parameters based on the model results and compare them between specific printer runs. The goal is to provide insight on how particles are formed from consumer FDM 3D printers.
3. Results and discussions
3.1. Experimental results from four contrasting printer runs
For the four different printer runs modeled in this study, the total particle number concentrations (N) and geometric mean diameters (Dpg) measured in the chamber during the printing period are shown in . Only SMPS data (particle electrical mobility diameters between 7 nm and 0.3 µm) are shown and used in the model since large particles (OPC data) contributed less than 1% to the total particle number emissions (Figure 3 of Zhang et al. Citation2017). A general consistency was seen in the total particle concentration time trends for the four runs. At the beginning of the print (i.e., filament extrusion began), the particle concentrations jumped to the maximum (2 × 105 – 1 × 106 particles/cm3) corresponding with the minimum Dpg (∼20 nm). Total number concentrations then rapidly decreased while particle size increased. After ∼30 min to 2 h of printing (depending mainly on filament material), the total number concentrations, as well as particle sizes, reached a steady state, and maintained relatively steady values until printing finished. Once finished, from then on there was an approximate exponential decay in particle number concentrations, mainly due to dilution by the continual addition of clean air into the chamber and some particle loss to surfaces. (Note the observed increase in mean size may be due to preferential loss of the smallest particles to surfaces and coagulation between small and large particles). and show that ABS brand d produced the most particles with the smallest average size, when operated under typical and reduced extrusion temperatures; with the lower extrusion temperature resulting in less total particle numbers, but of similar sizes. The less emitting ABS filament (a) produced smaller particle numbers, but of larger sizes. Nylon had even lower particle number emissions and larger mean sizes compared with ABS. These differences are believed to be driven by differences in the properties and concentration of the unknown condensable semivolatile vapors emitted from the heated filament, which are dependent on the extrusion temperature and the filament composition itself, as would be expected if the vapor source is simply evaporation from the filament. These contracts can be explored with the model.
Figure 2. Total particle number concentrations (N) and geometric mean diameters (Dpg) measured in chamber experiments. Time zero on the x-axis indicates the beginning of the print run. The vertical line indicates the time when printing stopped. The notation is filament material (filament brand) extruder temperature.
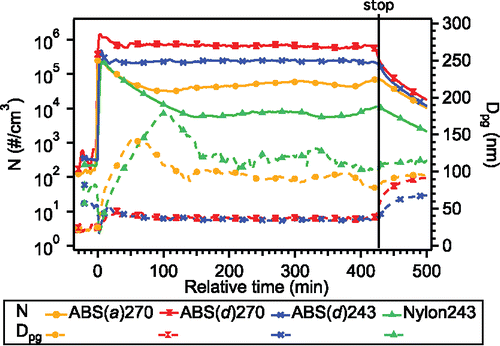
Figure 3. Average particle size distributions at steady state, the shaded areas are the mean values with one standard deviation; the lines are the corresponding lognormal fittings. The notation is filament material (filament brand) extruder temperature. ABS(d)270 and ABS(d)243 refer to the left y-axis, the rests refer to the right y-axis.
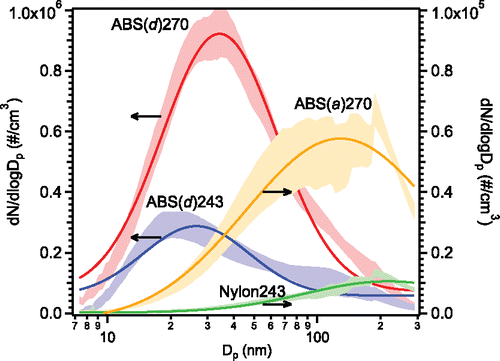
Table 2. Measured steady state conditions and model simulation results.
shows the average particle size distributions during the steady-state period, and their corresponding lognormal fits. It can be seen that the particle size distributions were generally lognormal.
From these observed particle emission time series trends, the following dynamic processes appear to be involved in the production of aerosols. The observed initial burst of small particles as printing starts, when few particles existed in the chamber or the control volume (total particle concentration <700 particles/cm3), is consistent with NPF of some fraction of the emitted vapors. These condensing vapors, referred to here as semivolatile compounds (SVCs) might be semivolatile organic compounds (SVOCs) or other compounds associated with the bulk polymer or trace additives in the filament. SVC supersaturated conditions leading to NPF could be reached in the control volume at the beginning of the printing due to vapor emissions with little loss onto pre-existing particles and cooling as the air moves away from the hot filament (Warren and Seinfeld Citation1984).
Once NPF has occurred and particles are present in the control volume, the SVCs continually being emitted during printing can be lost by condensation onto the pre-existing particles. This would lower supersaturation levels, lowering NPF rates, or even ending it. With lower NPF rates, total particle concentrations would decrease, as seen in the time series following the initial NPF burst (). At the same time, in the control volume particles become larger due to condensation and particle coagulation. All the while dilution is occurring due to background air in the chamber being forced through the control volume. At some point these processes produce steady-state aerosol size distributions in the overall chamber.
At steady state, the processes that decrease particle number concentration (i.e., coagulation, dilution and deposition) balance with NPF that increases particle number concentration. At the same time, the processes that increase particle size (i.e., condensation and coagulation) balance with NPF that produces smaller size particles. This view of processes is consistent with differences observed in the four contrasting printer runs shown in , resulting from differences in the emitted SVC concentrations and properties. Factors that contribute to the contrasting observations are explored with the model.
PLA is a commonly used filament, but not modeled here since it had a very different profile. An example is shown in Fig. S1. In general, the steady state condition was seldom observed for PLA. This might be because PLA filaments tend to be heated to lower temperatures than many other filament materials (210°C) and may produce SVCs that are less likely to condense. (In one case, PLA with an additive (Zhang et al. Citation2017) did produce significant particles, indicating that additives versus the bulk filament material can affect particle emissions).
3.2. Model results
Based on the governing equations, the steady state particle number concentration and size distribution are interrelated to the properties of the vapors that condense (i.e., SVCs), including vapor generation rate (R), saturation vapor pressure (Ps) and the condensation factor (f). Parameters related to coagulation were not specifically quantified since they were coupled within the differential equations. Model input parameters included R, f, T, Ps and x, outputs were the lognormal particle size distribution parameters N, Dpg and σg. To estimate the steady state conditions, the effective length of the control volume (x) was set at 50 cm and temperature as the average of the printer extruder and chamber temperatures. Because the properties of actual condensational vapors were unknown, exact solutions for particle concentrations cannot be obtained with the model. Instead we derived a range of solutions by changing one variable at a time while holding the other variables constant. The modeled steady state results were then compared with the observation data. For each printer run, the best-fit solution was selected and the results are discussed below. Best fit was defined as when differences in the model outputs and experimental data were minimized; i.e., the average of the absolute values of the errors of the steady state particle distribution characteristics (N, Dpg and σg) was minimum. There was generally a single best solution for each printer run (see SI Section S4.1 for details), we also investigated the solution with a slightly higher average error, but do not present the results here. The simulation results and errors are shown in . In general, the model simulation was able to capture the particle characteristics at steady state; the absolute errors were low, <5% for N, < 10% for Dpg and <21% for σg, indicating that the model can reasonably simulate the aerosol dynamic processes that produce particles from the FDM 3D printer we tested.
3.2.1. Sensitivity analysis: Parameters that affect particle emissions
A sensitivity analysis was used to explore how the model free parameters, R, f and Ps, are related to predicted properties of the steady state size distributions. These results were not linked to any specific filament or printer operating conditions, except that the extruder nozzle temperature was 270°C. In this analysis one parameter was varied while the other two were held fixed. Considering the computational demand, the sensitivity analysis was not carried out over all possible values in the ranges for every parameter, but was limited to selected values (see Secion S4.2). The summarized results are shown in , detailed figures can be found in the SI. The temperature was 420 K and the effective length was 50 cm; the dependent variables were steady state N, Dpg, and σg.
Figure 4. Sensitivity analysis on how particle concentrations and properties of the lognormal distribution depend on condensing vapor properties. The plots show the simulated steady state particle concentrations (N), geometric mean diameters (Dpg) and geometric standard deviations (σg) of lognormal size distributions as a function of (a) saturation vapor pressure (Ps), (b) vapor condensation factor (f) and (c) vapor generation rate (R). For every parameter evaluated, the controlled conditions are shown below the graphs.
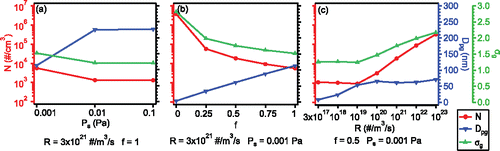
3.2.1.1. Saturation vapor pressure
Saturation vapor pressure (Ps) is related to particle formation since nucleation happens under supersaturated conditions (i.e., S = P/Ps > 1, where P is the vapor concentration). Ps is a property of the condensing vapors (SVCs); SVCs with lower Ps (at a given temperature) will result in higher vapor saturation ratios (S). Ps in our cases was evaluated to be in the range of 10−3 – 10−1 Pa, in order to match the observed steady state particle characteristics. In , as Ps decreased from 10−2 Pa to 10−3 Pa, the steady state N increased, which is consistent with NPF rates increasing with S, (i.e., lower Ps, with R and f fixed). As NPF is favored for lower Ps, the newly formed small particles drive the size distributions toward smaller average sizes (decrease in Dpg) and more spread in the distribution (increase in σg) (). (Note that coagulation will also affect the growth process and the width of the distribution). S is related to both Ps and the concentration of condensing vapor, P, which will be related to the vapor loss rate by condensation (f) and generation rate R.
3.2.1.2. Condensation factor
The condensation factor accounts for the possibility that multiple vapor species might be condensing onto pre-existing particles, since the identity of the SVC condensing during 3D printing is unknown. The condensation factor ranges from 0 to 1, with 0 indicating no condensation and 1 meaning that all the vapors will condense. Thus f represents the integrated property for all condensing species and a lower f favors NPF over condensation since it would lead to high vapor levels (P). shows the simulation results for multiple f values. As f approaches 0, i.e., decreasing condensation, implying more NPF, the total particle concentration increases and the particles are smaller in size as NPF dominates. On the contrary, if more SVCs condense on existing particles (higher f), NPF rates will decrease, fewer particles will be formed and the added condensation results in larger particles (higher Dpg). The distribution also narrows (smaller σg), since condensation tends to produce more monodisperse distributions (Seinfeld and Pandis Citation2006; Tsang et al. Citation1990).
3.2.1.3. Vapor generation rate
Vapor generation rate (R) represents the number of condensable molecules (SVCs) generated from the heated filament and is expected to be related to filament temperature. As shown in , there is an inflection point at R = 3 × 1019 #/m3/s. For R below this value, an increasing R has little effect on the total number of particles produced, but once this threshold is passed, increasing R results in increasing N; indicating that the inflection point of R represents a critical SVC emission rate at which NPF begins () (note, the inflection point is for a given f and Ps, and will change for different f and Ps combinations). At R below this point, the pre-existing particles grow in size (Dpg increases with R), but the distribution shape changes little (σg slightly decreases). The narrowing of size distributions by condensation is in some extent compensated by coagulation, which tends to make σg move toward a value of 1.32 (Hinds Citation1999), coagulation is also indicated by the slightly decrease of N in . Once R exceeds the critical value and NPF occurs, N increases, Dpg does not change significantly, pre-existing particles increasing in size and small particles added to the distribution, leading to fairly steady Dpg but larger σg ().
3.2.2. Model simulations compared to specific printer runs
Examples of model-predicted parameters for the best solutions (smallest average error) for the four printer runs are now compared. The comparisons are: (1) Same ABS filament run at different extruder tip temperatures, comparing effect of extruder temperature. (2) A high particle emitting ABS filament vs. a regular emitting ABS filament at the same extruder temperature, comparing filament manufacturer brands (or the effect of unknown additives). (3) ABS vs. nylon filament at the same extruder temperature, comparing bulk filament material. The measured particle steady state conditions (N, Dpg, σg) and model parameters (Ps, R, and f) for these comparisons are summarized in and .
Figure 5. Observed steady state particle size characteristics (N, Dpg, σg in upper panel) and model simulated condensing vapor properties (Ps, R, f in lower panel), grouped by 3 sets of comparisons. (a) compares extrusion temperature of the same filament; (b) compares ABS filament brands run at the same condition; (c) compares ABS and nylon materials run at the same condition.
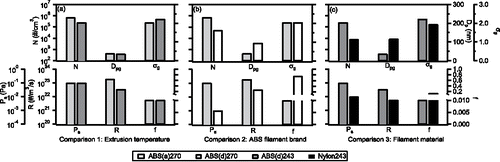
3.2.2.1. Comparison 1: Extruder tip temperature
For the same filament operated at different extrusion temperatures, the main difference was that the higher temperature produced more particles (larger N), but the size distribution was similar (differences of Dpg and σg within 10% whereas N was about 3 times higher) ( and ). The main effect of a higher filament temperature was a higher emission rate of the condensing vapors (R) (∼5 times higher). Since the same filament was used, the properties of the condensing vapors should be similar, as was found for the model parameters; Ps and f were similar in both cases ().
3.2.2.2. Comparison 2: High vs. regular emitting ABS brands
The high emitting ABS (brand d) produced more particles of smaller sizes than the regular ABS (brand a), but with similar spread in the distribution (σg) ( and ). These differences could be reproduced in the model by the high emitting ABS having a higher saturation vapor pressure (Ps), higher vapor generation rate (R) and lower condensation factor (f) ( and ). Higher Ps for the high emitting ABS is somewhat surprising since this lowers the saturation ratio (S = P/Ps), the driving force for NPF and condensation. One would expect S to be higher for the high emitting ABS filament since it produces a greater number of particles. However, the higher vapor emission rate (R) could compensate for this by leading to higher vapor concentrations (P), which increases S. A lower f also means that the vapor condensation route onto pre-existing particles is slower for the high emitting ABS, implying that P and S could reach higher levels since the condensational sink was impeded, which would increase the NPF rate (NPF is very sensitive to S). This would result in more particles of smaller sizes, as observed. Difference in Ps between these two ABS filament brands implies that the compounds forming the particles differ, consistent with the view that some unknown additives in the filament are responsible for the different particle emissions observed.
3.2.2.3. Comparison 3: ABS vs. nylon material
Similar to the above comparison, ABS produced substantially more particles than nylon filament, but particle mean size was smaller and the distribution broader. Relative differences in model-predicted vapor properties were also similar to the above comparison (i.e., compare and ). Both R and Ps for ABS were higher. Thus as above, high saturation levels (S = P/Ps) could be driven by the higher vapor concentrations (P), which were offset to some extent by high saturation vapor pressures (Ps). Again a lower condensation factor can enhance NPF and impede particle growth, leading to smaller mean particle sizes.
Overall, the model results indicate that known aerosol dynamic processes occurring very near the extruder nozzle can reasonably explain the observed steady state aerosol emissions produced by an FDM 3D printer, though the interplay between the variables is complex. In the above analysis we only compared model solution that gave the minimum errors. Comparing other solutions that gave larger average errors than the best fits tends to show that Ps plays a less distinct role than the SVC emission rate (R), i.e., the NPF process is largely driven by vapor concentration than saturation vapor pressure. The condensation factor also has effects on N and largely controls particle sizes. These results further emphasize the role of extrusion temperature on overall particle emissions.
4. Implications
The model simulations verified that particles can be formed from vapors emitted by the heated filaments and grow by vapor condensation and particle coagulation. The precursor vapors have very low saturation vapor pressures that can span a wide range (10−3 to 10−1 Pa). These are similar saturation vapor pressures to those found for NPF in chamber studies of secondary organic aerosols (SOA) formation, where Ps can range between 10−12 – 1 Pa (Pankow et al. Citation2001; Seinfeld et al. Citation2001; Tobias and Ziemann Citation2000) based on model estimates and 10−5 – 10−2 Pa based on measurements (Tao and McMurry Citation1989; Bilde and Pandis Citation2001). Similar to the model results, chamber SOA Ps associated with NPF also span large ranges.
The saturation vapor pressures of the VOCs sampled in the emission test chamber are many orders of magnitude higher than those that form particles. Many of the VOCs measured in chambers are similar to components that comprise the bulk filament material. For example, styrene, the most abundant VOC detected for ABS filament (Azimi et al. Citation2016; Steinle Citation2016), has a saturation vapor pressure of ∼103 Pa at 25°C (Chao et al. Citation1983) and the estimated partial pressure in the chamber was on the order of 10−2 Pa, conditions far from saturated(S ∼ 10−5). Other detected VOCs from ABS (e.g., ethyl benzene, methylene chloride, acetaldehyde) all have saturation vapor pressures larger than 103 Pa at room temperature and so would not contribute to the aerosols formed. The major VOC detected from nylon, caprolactam, has a Ps of 0.25 Pa at 25°C, which is the closest to what the model predicted, though at a lower temperature (EPA Citation1988).
Chemical analysis of the particles can provide insight on the condensing species. Measurements of 3D printer emitted particles with an Aerosol Chemical Speciation Monitor (ACSM, Ng et al. Citation2011) showed that the mass spectrum of particles emitted from ABS have no pattern similar to spectra expected for any ABS monomers (i.e., acrylonitrile, 1,3-butadiene, and styrene), based on spectra from NIST Chemistry WebBook (Stein Citation2016). In addition, pyrolysis gas chromatographic mass spectral analysis showed that raw ABS filament had fragments of ABS monomers, while particles formed from a 3D printer running that ABS filament collected on a filter, and subsequently analyzed in the same way as the filament, did not contain these fragments. This is consistent with the Raman spectra results from Vance et al. (Citation2017). Instead, particle spectrum showed major components thought to be associated with filament additives, such as fatty acids. Therefore, for ABS, the particles are not formed from the bulk ABS material, but some unknown additives that account for a very small fraction of the filament. As noted in material safety data sheets provided by manufacturers, ABS filaments are composed of 90% – 100% of thermoplastics polymers, with the rest as N,N’-Ethylenebis stearamide, typical antioxidants and pigments. Plasticizers like phthalates have saturation vapor pressure of 10−7 – 10−2 Pa at ambient temperature (Wu et al. Citation2016), organic dyes such as 1-aminoanthraquinone and 4-nitro-4′-aminoazobenzene derivatives have saturation vapor pressure of 10−4 – 10−2 Pa at temperature of 120 – 165°C (Bradley et al. Citation1960), both might be potential sources of SVCs. Inorganic compounds including metals commonly used in dyes and pigments may also be a potential source of SVCs; it was found that metallic aerosols were formed from metal associated vapor precursors during combustion (Biswas et al. Citation1992; Biswas et al. Citation1997; Sethi and Biswas Citation1990; Wang and Biswas Citation2000; Wu and Biswas Citation2000), and metals like Na, Al and transition metals like Fe, Cr, Ni were found in the particles emitted from ABS and PLA (Stefaniak et al. Citation2017; Stenile Citation2016; Zontek et al. Citation2017).
As noted, we found that the vapor generation rate, R, was a critical parameter accounting for differences between observed steady state total particle number concentrations when contrasting filament types and brands. For example, unlike Ps (r2 = 0.64) and f (r2 = 0.28), a higher correlation was found between measured N and model-predicted R (r2 = 0.96), for the four experiments discussed above. When expanding the comparison to all model runs having solutions with average errors between model and measured N, Dpg, σg less than 10% (n = 38), only simulated N and R was correlated (r2 = 0.90) (see S4.3). Since we have shown a linkage between R and extruder temperature, the model results support the view that nozzle (or filament) temperature is a critical parameter affecting total aerosol emissions. This has been noted by other investigators (Deng et al. Citation2016; Stabile et al. Citation2017; Zhang et al. Citation2017) and is reinforced by our modeling results.
Since 3D printing can emit large numbers of potentially toxic particles, designing printers with the aim to reduce emissions would be beneficial. One approach would be to reduce the particle formation at the source. Since particles are formed from SVCs emitted from the heated filament, and the emissions increase with extrusion temperature, a mitigation strategy is to reduce the extrusion temperature, as has been noted. However, the filament must be heated to a certain level to be extruded from the nozzle and produce a print object of sufficient quality. Identifying and removing filament additives that form particles would also be a viable approach, but would require extensive tests since the specific chemicals forming aerosols are unknown at this time.
An alternative is to remove the generated particles. A sealed enclosure with an effective filtration system can reduce overall particle emissions to some extent (Azimi et al. Citation2016; Citation2017), but has implications on the size and complexity of the printer and how it is operated. This modeling work shows that the particles are formed near the extruder nozzle and then dispersed by the extruder cooling fan. A potential mitigation method would be to collect the extremely small and highly mobile newly formed particles near the extruder nozzle before they substantially grow in size and are advected into the surroundings. Collection of particles to a surface by diffusion and thermophoretic forces may prove to be effective. For example, the distance particles with diameters of 3–50 nm travel in 1 s by diffusion is in range of 1.2 mm (3 nm particles) to 0.07 mm (50 nm particles) and by thermophoresis with a temperature gradient of 247 K/0.01 m the distance is ∼1 mm for particle less than about 50 nm (temperature gradient due to difference in nozzle and ambient air temperature, see SI section S5 for details). Thermophoretic force might affect the particle tracks (Kommu et al. Citation2004) and previous model results showed ∼40% increase in aerosol diffusional deposition rates onto surfaces when considering thermophoresis at high temperature (Bai and Biswas Citation1990). This indicates the small aerosols (e.g., <50 nm) might be collected by a combination of diffusion and thermophoresis before they grow and are advected out of the control volume. Modification of the forced air nozzle cooling system may be necessary. In addition, the model result that all aerosol dynamic processes happen within a small area close to the extruder nozzle and extruded filament provided insight when considering exposures in an indoor environment (e.g., proximity to the printer). For example, the particle size distributions (e.g., mean size) are not expected to significantly change for different locations within a room (assuming the printer is the only source), since particles are only diluted as they disperse to the surroundings, causing a uniform decrease in concentration for particles of all sizes.
5. Conclusions
We used a lognormal moment model to study the aerosol dynamic processes of particles formed from a FDM 3D printer. The model was based on the theory that particles are formed from nucleation of semivolatile vapors emitted from the heated filament, and then grow by vapor condensation and particle coagulation, all of which occur within a small control volume near the printer extruder nozzle. These dynamic processes are interrelated and depend on a number of key properties of the condensing vapors, including vapor emission rate, saturation vapor pressure and a condensation factor. Because the specific vapors emitted by the heated filament that undergo gas-to-particle conversion are unknown, the model could not be solved in a closed form. Instead ranges of solutions of the noted variables leading to the observed steady state particle size distribution characteristics (total particle number concentration, geometric mean diameter and geometric standard deviation) were found. Operating conditions, like filament material, filament brand and extrusion temperature influenced the steady state particle characteristics and could be related to the differences in the model predicted properties. The combined effects of multiple aerosol dynamic processes, which can be represented by emission rates and properties of condensable vapors, govern the particle emissions from FDM 3D printers. Vapor emission rate from the filament was a key parameter and linked to the printer extruder (filament) temperature. Possible mitigation strategies involving removing the newly formed small particle near the extruder nozzle are also suggested by the model results.
UAST_1464115_Supplemental_File.zip
Download Zip (4 MB)Additional information
Funding
References
- Adams, K., Bankston, J., Barlow, A., Holdren, M. W., Meyer, J., and Marchesani, V. J. (1999). Development of Emission Factors for Polypropylene Processing. J. Air Waste Manag. Assoc., 49(1):49–56. doi:10.1080/10473289.1999.10463782.
- ASTM. (2013). ASTM Standard D6670-13, Standard Practice for Full-Scale Chamber Determination of Volatile Organic Emissions from Indoor Materials/Products. American Society for Testing and Materials International, West Conshohocken, PA.
- Azimi, P., Fazli, T., and Stephens, B. (2017). Predicting Concentrations of Ultrafine Particles and Volatile Organic Compounds Resulting from Desktop 3D Printer Operation and the Impact of Potential Control Strategies. J. Ind. Ecol., 21(S1):S107–S109. doi:10.1111/jiec.12578.
- Azimi, P., Zhao, D., Pouzet, C., Crain, N. E., and Stephens, B. (2016). Emissions of Ultrafine Particles and Volatile Organic Compounds from Commercially Available Desktop Three-Dimensional Printers with Multiple Filaments. Environ. Sci. Technol., 50(3):1260–1268. doi:10.1021/acs.est.5b04983.
- Bai, H., and Biswas, P. (1990). Deposition of Lognormally Distributed Aerosols Accounting for Simultaneous Diffusion, Thermophoresis and Coagulation. J. Aerosol Sci., 21(5):629–640. doi:10.1016/0021-8502(90)90118-H.
- BAM, Federal Institute for Materials Research and Testing. (2012). Test Method for the Determination of Emissions from Hardcopy Devices within the Award of the Blue Angel Ecolabel for Equipment with Printing Function according to RAL-UZ-171. BAM, St. Augustin, Germany.
- Barrett, J. C., and Webb, N. A. (1998). A comparison of some approximate methods for solving the aerosol general dynamic equation. J. Aerosol Sci., 29(1–2):31–39. doi:10.1016/S0021-8502(97)00455-2.
- Berman, B. (2012). 3-D Printing: The New Industrial Revolution. Bus. Horiz., 55(2):155–162. doi:10.1016/j.bushor.2011.11.003.
- Bilde, M. and Pandis, S. N. (2001). Evaporation Rates and Vapor Pressures of Individual Aerosol Species Formed in the Atmospheric Oxidation of α- and β-Pinene. Environ. Sci. Technol., 35(16):3344–3349. doi:10.1021/es001946b.
- Biswas, P., Li, X. M., and Pratsinis, S. E. (1989). Optical Wave-Guide Preform Fabrication – Silica Formation and Growth in a High-Temperature Aerosol Reactor. J. Appl. Phys., 65:2445–2450. doi:10.1063/1.342814.
- Biswas, P., Lin, W. Y., and Wu, C. Y. (1992). Formation and Emission of Metallic Aerosols from Incinerators. J. Aerosol Sci., 23(Suppl. 1):273–276. doi:10.1016/0021-8502(92)90402-H.
- Biswas, P., Wu, C. Y., Zachariah, M. R., and McMillin, B. (1997). Characterization of Iron Oxide-Silica Nanocomposites in Flames: Part II. Comparison of Discrete-Sectional Model Predictions to Experimental Data. J. Mater. Res., 12(3):714–723. doi:10.1557/JMR.1997.0106.
- Bradley, R. S., Bird, C. L., Jones, F. (1960). The Vapour Pressures and Heats of Sublimation of Some Disperse Dyes. Trans. Faraday Soc., 56:23–28. doi:10.1039/tf9605600023.
- Brown, D. P., Kauppinen, E. I., Jokiniemi, J. K., Rubin, S. G., Biswas, P. (2006). A Method of Moments Based CFD Model for Polydisperse Aerosol Flows with Strong Interphase Mass and Heat Transfer. Comput. Fluids., 35:762–780. doi:10.1016/j.compfluid.2006.01.012.
- Chan, F., Rajaram, N., House, R., Kudla, I., Lipszyc, J., and Tarlo, S. M. (2017). Potential Respiratory Effects From 3-D Printing. Presented at the American Thoracic Society, Washington, DC.
- Chao, J., Lin, C. T., and Chung, T. H. (1983). Vapor Pressure of Coal Chemicals. J. Phys. Chem. Ref. Data., 12(4):1033–1063. doi:10.1063/1.555695.
- Davis, A., Black, M., Zhang, Q., Wong, J., and Weber, R. (2016). Fine Particle and Chemical Emissions from Desktop 3D Printers. ASHRAE Annual Conference, St. Louis, MO.
- Deng, Y., Cao, S.-J., Chen, A., and Guo, Y. (2016). The Impact of Manufacturing Parameters on Submicron Particle Emissions from a Desktop 3D Printer in the Perspective of Emission Reduction. Build. Environ., 104:311–319. doi:10.1016/j.buildenv.2016.05.021.
- ECMA. (2015). ECMA-328 Standard 7th Edition, Determination of Chemical Emission Rates from Electronic Equipment. ECMA International, Geneva. www.ecma-international.org.
- EPA. (1988). Health and Environmental Effects Profile for Caprolactam. ECAO-CIN-G018. Environmental Criteria and Assessment Office, Office of Health and Environmental Assessment, Office of Research and Development. U. S. Environmental Protection Agency, Cincinnati, OH.
- Frenklach, M., and Harris, S. J. (1986). Aerosol Dynamics Modeling Using the Method of Moments. J. Colloid Interf. Sci., 118(1):252–261. doi:10.1016/0021-9797(87)90454-1.
- Friedlander, S. K. (2000). Smoke, Dust and Haze: Fundamentals of Aerosol Dynamics. Oxford University Press, New York, NY.
- Gibson, I., Rosen, D. W., and Stucker, B. (2010). Additive Manufacturing Technologies. Springer US, Boston, MA.
- He, C., Morawska, L., and Taplin, L. (2007). Particle Emission Characteristics of Office Printers. Environ. Sci. Technol., 41(17):6039–6045. doi:10.1021/es063049z.
- Hinds, W. C. (1999). Aerosol Technology: Properties, Behavior, and Measurement of Airborne Particles (2nd ed). Wiley, New York.
- Hoff, A., Jacobsson, S., Pfäffli, P., Zitting, A., and Frostling, H. (1982). Degradation Products of Plastics: Polyethylene and Styrene-Containing Thermoplastics—Analytical, Occupational and Toxicologic Aspects. Scand. J. Work Environ. Health., 8(2):1–60.
- House, R., Rajaram, N., and Tarlo, S. M. (2017). Case Report of Asthma Associated with 3D Printing. Occup. Med. (Lond.), 67(8):652–654. doi:10.1093/occmed/kqx129.
- Hulburt, H. M., and Katz, S. (1964). Some Problems in Particle Technology: A Statistical Mechanical Formulation. Chem. Eng. Sci., 19(8):555–574. doi:10.1016/0009-2509(64)85047-8.
- Khatri, M., Bello, D., Gaines, P., Martin, J., Pal, A. K., Gore, R., and Woskie, S. (2013). Nanoparticles from Photocopiers Induce Oxidative Stress and Upper Respiratory Tract Inflammation in Healthy Volunteers. Nanotoxicology., 7(5):1014–1027. doi:10.3109/17435390.2012.691998.
- Kim, Y., Yoon, C., Ham, S., Park, J., Kim, S., Kwon, O., and Tsai, P.-J. (2015). Emissions of Nanoparticles and Gaseous Material from 3D Printer Operation. Environ. Sci. Technol., 49(20):12044–12053. doi:10.1021/acs.est.5b02805.
- Koivisto, A. J., Hussein, T., Niemelä, R., Tuomi, T., and Hämeri, K. (2010). Impact of particle emissions of new laser printers on modeled office room. Atmos. Environ., 44(17):2140–2146. doi:10.1016/j.atmosenv.2010.02.023.
- Kommu, S., Khomami, B., and Biswas, P. (2004). Simulation of Aerosol Dynamics and Transport in Chemically Reacting Particulate Matter Laden Flows. Part II: Application to CVD Reactors. Chem. Eng. Sci., 59:359–371. doi:10.1016/j.ces.2003.05.010.
- McGraw, R. (1997). Description of Aerosol Dynamics by the Quadrature Method of Moments. Aerosol Sci. Technol., 27(2):255–265. doi:10.1080/02786829708965471.
- Ng, N. L., Herndon, S. C., Trimborn, A., Canagaratna, M. R., Croteau, P. L., Onash, T. B., Sueper, D., Worsnop, D. R., Zhang, Q., Sun, Y. L., and Jayne, J. T. (2011). An Aerosol Chemical Speciation Monitor (ACSM) for Routine Monitoring of the Composition and Mass Concentration of Ambient Aerosol. Aerosol Sci. Tech., 45(7):780–794. doi:10.1080/02786826.2011.560211.
- Pankow, J. F., Seinfeld, J. H., Asher, W. E., and Erdakos, G. B. (2001). Modeling the Formation of Secondary Organic Aerosol. 1. Application of Theoretical Principles to Measurements Obtained in the α-Pinene/, β-Pinene/, Sabinene/, Δ 3 -Carene/, and Cyclohexene/Ozone Systems. Environ. Sci. Technol., 35(6):1164–1172. doi:10.1021/es001321d.
- Pirela, S., Molina, R., Watson, C., Cohen, J., Bello, D., Demokritou, P., and Brain, J. (2013). Effects of Copy Center Particles on the Lungs: A Toxicological Characterization Using a Balb/c Mice Model. Inhal. Toxicol., 25(9):498–508. doi:10.3109/08958378.2013.806614.
- Pratsinis, S. E. (1988). Simultaneous Nucleation, Condensation, and Coagulation in Aerosol Reactors. J. Colloid Interf. Sci., 124(2):416–427. doi:10.1016/0021-9797(88)90180-4.
- Pratsinis, S. E., Kodas, T. T., Dudukovic, M. P., and Friedlander, S. K. (1986). Aerosol Reactor Design: Effect of Reactor Type and Process Parameters on Product Aerosol Characteristics. Ind. Eng. Chem. Proc. DD., 25(3):634–642. doi:10.1021/i200034a007.
- Rutkowski, J. V., and Levin, B. C. (1986). Acrylonitrile–Butadiene–Styrene Copolymers (ABS): Pyrolysis and Combustion Products and Their Toxicity—A Review of the Literature. Fire Mater., 10(3–4):93–105. doi:10.1002/fam.810100303.
- Salthammer, T., Schripp, T., Uhde, E., and Wensing, M. (2012). Aerosols Generated by Hardcopy Devices and Other Electrical Appliances. Environ. Pollut., 169:167–174. doi:10.1016/j.envpol.2012.01.028.
- Scungio, M., Vitanza, T., Stabile, L., Buonanno, G., Morawska, L. (2017). Characterization of Particle Emission from Laser Printers. Sci. Total Environ., 586:623–630. doi:10.1016/j.scitotenv.2017.02.030.
- Seigneur, C., Hudischewskyj, A. B., Seinfeld, J. H., Whitby, K. T., Whitby, E. R., Brock, J. R., and Barnes, H. M. (1986). Simulation of Aerosol Dynamics: A Comparative Review of Mathematical Models. Aerosol Sci. Technol., 5(2):205–222. doi:10.1080/02786828608959088.
- Seinfeld, J. H., Erdakos, G. B., Asher, W. E., and Pankow, J. F. (2001). Modeling the Formation of Secondary Organic Aerosol (SOA). 2. The Predicted Effects of Relative Humidity on n Formation in the α-pinene-, β-Pinene-, Sabinene-, Δ3-Carene-, and Cyclohexene-Ozone Systems. Environ. Sci. Technol., 35(9):1806–1817. doi:10.1021/es001765+.
- Seinfeld, J. H., and Pandis, S. N. (2006). Atmospheric Chemistry and Physics. John Wiley & Sons, Inc, Hoboken, NJ.
- Sethi, V., and Biswas, P. (1990). Modeling of Particle Formation and Dynamics in a Flame Incinerator. J. Air Waste Manage. Assoc., 40:42–46. doi:10.1080/10473289.1990.10466664.
- Stabile, L., Scungio, M., Buonanno, G., Arpino, F., and Ficco, G. (2017). Airborne Particle Emission of a Commercial 3D Printer: The Effect of Filament Material and Printing Temperature. Indoor Air., 27:398–408. doi:10.1111/ina.12310.
- Stefaniak, A. B., LeBouf, R. F., Yi, J., Ham, J., Nurkewicz, T., Schwegler-Berry, D. E., Chen, B. T., Wells, J. R., Duling, M. G., Lawrence, R. B., Martin Jr., S. B., Johnson, A. R., and Virji, M. A. (2017). Characterization of Chemical Contaminants Generated by a Desktop Fused Deposition Modeling 3-Dimensional Printer. J. Occup. Environ. Hyg., 14(7):540–550. doi:10.1080/15459624.2017.1302589.
- Stein, S. E. “Mass Spectra” in NIST Chemistry WebBook, NIST Standard Reference Database Number 69, Eds. P. J. Linstrom and W. G. Mallard, p. 20899: National Institute of Standards and Technology, Gaithersburg, MD. doi:10.18434/T4D303, (retrieved January 15, 2016).
- Steinle, P. (2016). Characterization of emissions from a desktop 3D printer and indoor air measurements in office settings. J. Occup. Environ. Hyg., 13(2):121–132. doi:10.1080/15459624.2015.1091957.
- Stephens, B., Azimi, P., El Orch, Z., and Ramos, T. (2013). Ultrafine Particle Emissions from Desktop 3D Printers. Atmos. Environ., 79:334–339. doi:10.1016/j.atmosenv.2013.06.050.
- Tao, Y., and McMurry, P. H. (1989). Vapor Pressures and Surface Free Energies of C14-C18 Monocarboxylic Acids and C5 and C6 Dicarboxylic Acids. Environ. Sci. Technol., 23(12):1519–1523. doi:10.1021/es00070a011.
- Tobias, H. J., and Ziemann, P. J. (2000). Thermal Desorption Mass Spectrometric Analysis of Organic Aerosol Formed from Reactions of 1-Tetradecene and O3 in the Presence of Alcohols and Carboxylic Acids. Environ. Sci. Technol., 34(11):2105–2115. doi:10.1021/es9907156.
- Tsang, T. H., Cook, S. M., and Marra, M. E. (1990). Dynamic Behavior of Condensation and Evaporation of Polydisperse Volatile Aerosols. Aerosol Sci. Technol., 12(2):386–398. doi:10.1080/02786829008959354.
- UL. (2013). UL 2819, GREENGUARD Certification for Chemical and Particle Emissions for Electronic Equipment. Underwriters Laboratories, Marietta, GA.
- UL. (2014). UL 2823, GREENGUARD Certification Program Method for Measuring and Evaluating Chemical and Particle Emissions from Electronic Equipment Using Dynamic Environmental Chambers. Underwriters Laboratories, Northbrook, IL.
- Vance, M. E., Pegues, V., Van Montfrans, S., Leng, W., and Marr, L. C. (2017). Aerosol Emissions from Fused-Deposition Modeling 3D Printers in a Chamber and in Real Indoor Environments. Environ. Sci. Technol., 51:9516–9523. doi:10.1021/acs.est.7b01546.
- Wang, Z. -M., and Biswas, P. (2000). Nickel Speciation and Aerosol Formation during Combustion of Kerosene Doped with Nickel Nitrate Aerosol in a Premixed Burner. Aerosol. Sci. Tech., 33(6):525–535. doi:10.1080/02786820050195368.
- Warren, D. R., and Seinfeld, J. H. (1984). Nucleation and Growth of Aerosol From a Continuously Reinforced Vapor. Aerosol Sci. Technol., 3(2):135–153. doi:10.1080/02786828408959003.
- Wu, C. -Y., and Biswas, P. (1998). Study of Numerical Diffusion in a Discrete-Sectional Model and Its Application to Aerosol Dynamics Simulation. Aerosol Sci. Technol., 29:259–378. doi:10.1080/02786829808965576.
- Wu, C-Y., and Biswas, P. (2000). Lead Species Aerosol Formation and Growth in Multicomponent High-Temperature Environments. Environ. Eng. Sci., 17(1):41–60. doi:10.1089/ees.2000.17.41.
- Wu, Y., Eichler, C. M. A., Chen, S., and Little, J. C. (2016). Simple Method To Measure the Vapor Pressure of Phthalates and Their Alternatives. Environ. Sci. Technol., 50(18):10082–10088. doi:10.1021/acs.est.6b02643.
- Yi, J., LeBouf, R. F., Duling, M. G., Nurkiewicz, T., Chen, B. T., Schwegler-Berry, D., Virji, M. A., and Stefaniak, A. B. (2016). Emission of Particulate Matter from a Desktop Three-Dimensional (3D) Printer. J. Toxicol. Env. Health. Part A., 79(11):453–465. doi:10.1080/15287394.2016.1166467.
- Yoon, H. I., Hong, Y-C., Cho, S-H., Kim, H., Kim, Y. H., Sohn, J. R., Kwon, M., Park, S-H., Cho, M-H., and Cheong, H-K. (2010). Exposure to Volatile Organic Compounds and Loss of Pulmonary Function in the Elderly. Eur. Respir. J., 36(6):1270–1276. doi:10.1183/09031936.00153509.
- Yu, M., Lin, J., and Chan, T. (2008). A New Moment Method for Solving the Coagulation Equation for Particles in Brownian Motion. Aerosol Sci. Technol., 42(9):705–713. doi:10.1080/02786820802232972.
- Yu, M., and Liu, Y. (2016). Methods of Moments for Resolving Aerosol Dynamics. In K. Volkov (Ed.), Aerosols – Science and Case Studies. InTech.
- Zhang, Q., Wong, J. P. S., Davis, A. Y., Black, M. S., and Weber, R. J. (2017). Characterization of Particle Emissions from Consumer Fused Deposition Modeling 3D Printers. Aerosol Sci. Technol., 51(11):1275–1286. doi:10.1080/02786826.2017.1342029.
- Zontek, T. L., Ogle, B. R., Jankovic, J. T., and Hollenbeck, S. M. (2017). An Exposure Assessment of Desktop 3D Printing. J. Chem. Health Saf., 24(2):15–25. doi:10.1016/j.jchas.2016.05.008.
- Zukas, V., and Zukas, J. A. (2015). An Introduction to 3D Printing. First Edition Design Publishing, Sarasota, FL.