Abstract
The chemical composition of aerosol particles strongly influences the earth’s climate, including their effects as sediments reducing the reflectivity of snow surfaces. Source apportionment and transportation pathways of such particles help to assess their contribution to regional surface-radiative heating. The chemical composition of particles in the free troposphere was studied at the Jungfraujoch (3580 m asl) in the Swiss Alps, using the laser mass spectrometer LAMPAS 2 during the CLACE-1 campaign. Statistical methods and lab measurements of reference particles were used to investigate the source and aging of field-measured particles. Desert sand, igneous rock and river sediment were used as model systems to differentiate between aged and non-aged atmospheric particles. Dried-up snow-water particles showed a particle composition typically found at the measurement site, largely influenced by carbonaceous and organic particles from local sources. During on-line measurements between 17 and 24 March 2000, sand particles from two sandstorm events were a significant source for less-aged mineral particles. Our results show that desert-sand particles can be transported over long distances and that silicate-rich particles in particular are only slightly altered in the atmosphere. We assume that both, the carbonaceous fraction as well as the fresh mineral particles lead to a warming effect at the measurement site due to their intrinsic properties of low solubility and strong light absorption, decreasing the surface reflectivity of the Alpine snow layer. The results allow for a better assessment of environmental influences and particles’ impact on the local climate in the Alpine region at the Jungfraujoch.
Copyright © 2021 American Association for Aerosol Research
EDITOR:
1. Introduction
The influence of different kinds of aerosol on the earth´s climate was studied extensively in a large number of publications in recent years (Allen, Landuyt, and Rumbold Citation2016; Isaksen et al. Citation2009; Koch et al. Citation2009; Nabat et al. Citation2015; Shindell et al. Citation2009) and in the past (Albrecht Citation1989; Charlson et al. Citation1991; Haywood and Shine Citation1995; Twomey Citation1977). Correct interpretation of light scattering versus absorption of certain types of aerosols will lead to a better understanding and prediction of the future climate evolution. Whether a certain aerosol has a warming or a cooling effect on the atmosphere, depends on various physical and chemical parameters as for example particle size, chemical composition, site of action and water content (Kandler et al. Citation2009; Tegen, Lacis, and Fung Citation1996). Usually a distinction is made between strongly hydrophilic particles consisting of easily soluble components (sulfates, nitrates, salts) and hydrophobic particles like black carbon (BC) or organic matter (Andreae and Gelencser Citation2006). The former ones are associated with climate cooling due to their light scattering abilities (Charlson et al. Citation1991), BC with warming by absorption of radiation in a broad spectral range (Hansen and Nazarenko Citation2004; Ramanathan and Carmichael Citation2008). Aggregated mineral-dust particles are associated with light-absorbing abilities as well (Sokolik and Toon Citation1999).
The strong light-absorbing properties of aerosol particles were found to play an important role in places where the reflectivity of the earth's surface is very high (Gautam et al. Citation2013). In the alpine region, for example, the white snow with its high albedo is partly covered by aggregated mineral dust particles as well as BC, further enhancing the warming effect of those particles by reduction of snow surface reflectivity. This effect is well known for BC, but in case of mineral dust strongly depends on the solubility and hence chemical processing of these particles before deposition (Ming et al. Citation2008). The differentiation between fresh and aged mineral particles is therefore essential for assessing the influence of the particles on the regional snow albedo. In this context, the role of mineral dust is often neglected, but it has been shown to be an important contributor to the reduced surface reflectivity (Gautam et al. Citation2013; Liou et al. Citation2014). The lower absorption of the mineral dust compared to BC is thereby compensated by its larger deposition volumes, which play a particularly important role in the vicinity of large mineral dust sources. Especially the influence of regional particle sources and less-aged particles that were fast transported from a greater distance is very important and has still not been fully clarified (Henning et al. Citation2003). Such effects can be studied notably well in remote places where low particle concentrations are expected and where urban and rural influences can be well assessed. Many measurements on the Jungfraujoch in the Swiss Alps in recent years show that this site is particularly suitable for this purpose (Cozic et al. Citation2008; Henning et al. Citation2003; Hinz et al. Citation2005).
Various off-line analytical methods have been used for determination of the chemical composition of aerosol particles. Such methods are, for example, atomic absorption spectroscopy (AAS), proton-induced x-ray emission (PIXE) (Matschullat et al. Citation2000), ion chromatography (Henning et al. Citation2003) and gas-chromatography coupled with mass spectrometry (GC-MS) (Zheng et al. Citation2005). A prerequisite for all these methods is the collection of particles on filters or by means of impactors. The analysis of the filter samples often allows a quantitative statement about the overall chemical composition of the particle population at the sampling location. However, differentiating between the individual particle sources using these methods is very challenging, and it is still not possible to prevent changes in the particles during sampling and storage. In order to avoid these disadvantages, it is necessary to measure the particles at the place of their origin. On-line mass spectrometric methods are particularly suitable for this. There is only a small number of such techniques available, including multiple- and single-particle aerosol mass spectrometry (MPMS and SPMS) (Hinz and Spengler Citation2007).
It is particularly difficult to differentiate between externally and internally mixed particles when particle ensemble measurements are used, as in the case of MPMS. Although MPMS allows to quantitatively determine the chemical composition of particles and to identify certain particle sources by Positive Matrix Factorization (PMF), the results are based on many parameters and vary depending on the interpretation of the initially determined factors (Ulbrich et al. Citation2009). Another prerequisite for a reliable determination of particle sources using PMF is the availability of a sufficiently large data set. It is therefore important to be able to measure individual particles separately. Single-particle aerosol mass spectrometry offers optimal conditions for this, as individual particles are vaporized, ionized and detected one after another. Even a small number of measured particles makes it possible to draw conclusions about the origin of the particles and environment to which these particles were exposed (Hinz et al. Citation1999).
The analysis of single particles is often ambiguous, if only one ion polarity is monitored. A low mass resolution, which is typical for such devices, can lead to overlapping signals, making it difficult to correctly assign the detected ions. Bipolar time-of-flight mass spectrometry, detecting ions of both polarities from the same particle, therefore has been found to be crucial for correct assignment of signals. (Hinz, Kaufmann, and Spengler Citation1996). On-line measurement in bipolar single-particle mass spectrometry is a powerful tool for describing the native state of atmospheric particles. We report on the differentiation of fresh and aged internally mixed particles, based on comparing ion-abundance ratios in mass spectra of online-measured airborne particles at the Jungfraujoch with those in mass spectra of representative particles collected from locations that are expected to be important particle sources at the measurement site. Their chemical composition, origin and aging behavior are important parameters in this context and were therefore investigated for the isolated place of the Jungfraujoch. The results of this study should provide a deeper insight into the influence of regional and less-aged mineral particles on the surface reflectivity of snow layers in such remote regions.
2. Methodology
2.1. Samples collection and handling
Three different types of particles where chosen as model systems in laboratory measurements: Alpine rock stone, Morocco desert sand and Rhine river sediment. Each of these populations represents a possible class of mineral atmospheric aerosol particles, fresh mineral dust in case of the sand or eroded rock in case of Aarmassif granite. The sediment particles demonstrate a certain evolution of eroded mineral particles processed by anthropogenic and natural influences.
A fourth type of particles was also investigated. Those particles were retrieved from dried-up snow water, and it is expected that they reflect the particle composition in the surrounding atmosphere over a longer period of time. These particles precipitate during snowfall and accumulate in different snow layers. It is expected that most mineral atmospheric particle populations measured at the Jungfraujoch site are to some extent affected by these mineral particle classes or in the case of snow water residues reflect similar chemical features.
Snow and rock stone samples were collected at the top region of the high-alpine site Jungfraujoch in the Swiss Alps (3580 m asl) on 27 March 2000, during the field campaign “Cloud and Aerosol Characterization Experiment (CLACE-1).” Sampling spots for the snow samples were located at the exit area of the station facing the glacier while rock was sampled at the Jochplateau. Fresh snow was obtained from the top (0–30 cm) and pressed snow (30–45 cm) from deeper snow layers and molten to yield a total volume of more than 4 liters of water. The water was stored in plastic bottles at 4 °C in a refrigerator. For aerosol measurements, about 1.5 liters of snow water were slowly evaporated to dryness, and the remaining residues were ground with an agate mortar. The rock stone samples were stored in the refrigerator at 4 °C. For measurement, small pieces (<1 cm3) were dried in a desiccator overnight and ground with a mortar to yield a fine powder.
Rhine sediment was sampled in 2011 near Lörrach in Germany, dried and size selected. The inhomogeneous powder with particle sizes smaller than 2 mm was then ground with a ball mill to obtain particle sizes below 10 µm, which is in the measurement range of the LAMPAS 2 (Laser Mass Analyzer for Particles in the Airborne State) instrument (Trimborn, Hinz, and Spengler Citation2000).
Morocco sand was sampled in February 2005 near Laayoune in Morocco and treated the same way as the rock samples. Measurement of the finely dispersed powders from rock, sand and snow water residues was carried out with the LAMPAS 3 mass spectrometer (Hinz et al. Citation2011) in the laboratory. The powders from rock, sand and river sediment were additionally microwave digested with aqua regia and measured by inductively coupled plasma - optical emission spectrometry (ICP-OES).
2.2. Instrumentation
Direct analysis of single tropospheric aerosol particles was carried out during the field campaign CLACE-1 on ten days in March 2000 at the Jungfraujoch with the LAMPAS 2 instrument. A detailed description of the instrument can be found elsewhere (Trimborn, Hinz, and Spengler Citation2000). An overview of the whole dataset was published earlier (Hinz et al. Citation2005). The measured spectra were revised, partly recalibrated in greater detail, and crowded spectra containing saturated ion signals were filtered out. Only for 17, 18 and 22 till 24 March, the quality and number of spectra were sufficiently high for statistically reliable evaluation. Vacuum aerodynamic particle size was determined by calibrating the instrument with polystyrene latex spheres with diameters between 0.2 µm and 5.7 µm. Single-particle analyses of rock, sand and dried water samples were performed in the laboratory with an improved version of the previously described instrument. The LAMPAS 3 instrument was developed with reduced instrumental size and optimized for user-friendly long-term operation. A sketch of the instrument is shown in Figure S1. The dry powders were dispersed and transferred into the LAMPAS 3 instrument through a differentially pumped inlet system, consisting of a nozzle, a skimmer and an orifice. Particles in the size range between 0.2 and 10 µm were optically detected by two continuous cw-laser beams (λ = 532 nm) inside the main chamber of the mass spectrometer. Particle sizes were determined based on their size-dependent velocities. Afterwards, an actively triggered 337 nm pulsed UV laser evaporated and ionized the detected particles. The positively and negatively charged ions were then simultaneously analyzed using a bipolar time-of-flight mass spectrometer at a mass resolution (M/ΔM) of about 300 for both polarities. LAMPAS 2 and 3 are comparable in terms of particle detection, ionization and ion separation and show the same spectral quality and intensities. The main difference is the more compact design of LAMPAS 3.
Figure 1. Sum spectra measured with LAMPAS 3 from (a) ground rock stone from the top region of the Jungfraujoch (307 spectra), (b) ground Morocco desert sand (452 spectra), (c) ground river sediment from Rhine river in Germany (660 spectra), and (d) dried snow water residues from the top region of the Jungfraujoch (265 spectra).
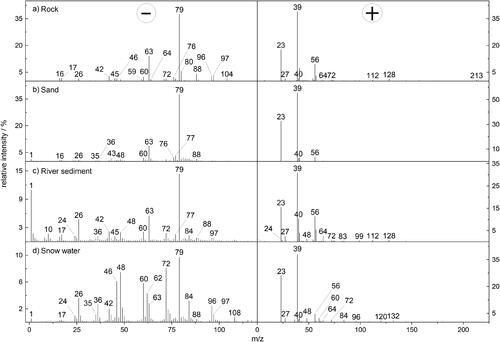
An ICP-OES instrument (Varian 720ES) was used for the quantification of aqua-regia-soluble components in rock, sand and river sediment. The amount of snow water residue was too small for an ICP-OES analysis. Microwave digestion was carried out twice for each sample (0.3 g/50 ml). Three repeated measurements (technical replicates) per sample were performed and the mean values were calculated. The values of both digestions were also averaged. Reference samples were measured at the beginning and end of each run, 18 samples were measured in total. Calibration was performed using several dilutions of an ICP multi-element standard (Carl Roth, Karlsruhe), with the following concentrations: As, Cd, Co, Cr, Cu, Na, Ni, Pb, S, Zn = 0.1, 0.5, 1.0 and 5 mg/l; K, Ca = 51.1, 60.5, 101 and 155 mg/l; Fe, Al = 101, 110.5, 151 and 205 mg/l; Mg, Mn = 1, 5, 11 and 55 mg/l; P = 1, 5, 10 and 100 mg/l.
2.3. Data evaluation
For data evaluation and classification of particles, a hierarchical clustering algorithm (HCA) was used (Ward Citation1963). This algorithm is part of the software package Perseus (Version 1.5.3.0) from the Max Planck Institute of Biochemistry (MPIB, Martinsried, Germany) (Tyanova et al. Citation2016). For HCA, predefined clusters are gradually combined into larger and larger clusters based on their similarity. This merging is visualized by a dendrogram (tree diagram), where the length of the branches until the merging reflects the similarity of the clusters. Pre-processing of data for finding initial cluster centers was done by a k-means algorithm. The iterative clustering process sorts mass spectra with similar composition into classes. 50 start clusters were created by the k-means algorithm to account for even rare clusters. We used a Pearson correlation distance metric for the HCA clustering. Euclidean distance was tested likewise but was found to join similar clusters only in a very late stage of the HCA (see Figure S3). Fuzzy clustering as an alternative clustering approach allows to assign a membership degree to each spectrum and is therefore more robust against false assignments, but due to its non-discrete nature it builds up artificial spectra patterns to which each spectrum participates according to its membership degree. To build up a joining tree like in HCA, we rather wanted to use mean spectra patterns, where each spectrum contributes equally. This allowed us to distinguish more strictly between the groups. The clustering quality is comparable between fuzzy clustering and k-means, while k-means performs better in case of speed and computing power (Azar, El-Said, and Hassanien Citation2013).
We found that highly intense signals of potassium and sodium are the dominating factors for the cluster separation. Both signals show very low dependence on the actual content of sodium or potassium in the particles but rather appear as ubiquitous background signals. Even more, the high intensity (often not completely resolved within the dynamic range of the detector) of both signals deplete and distort the remaining signals in the spectra. This effect is typical for laser desorption ionization (LDI) MS of aerosol particles due to the ionic nature of potassium and sodium in various compounds (Hinz et al. Citation2005). We therefore removed the potassium and sodium signals from all spectra, followed by normalization to the total ion count (TIC) of the remaining signals. The intensity ratios of signals in positive- and negative-ion spectra, as used for statistical evaluation, remained the same in our approach since no scaling (logarithmic, square root) was applied to the spectra.
The approach we present here is different from common methods, which are, for example, based on the determination of particle classes using the entire mass spectrum and leading to a large number of clusters and a suppression of less abundant spectra types. The effort of a manual separation and sorting after such clustering is high, compared to statistical identification of particle classes based on significant ion species (Kamphus et al. Citation2010; Schmidt et al. Citation2017). Most classification approaches rely on the idea that all particles exhibit signals of marker ions that belong primarily to one particle class, e.g., Mg+, Al+, and Fe+ for mineral particles. These procedures are well suited for the identification of different kinds of particles in one dataset. To identify for example mineral particles only by using such marker signals in an aged population, based on representative mineral particle spectra patterns, is considerably more difficult because of the changes, the freshly emitted particles undergo in the atmosphere. For comparison of different datasets consisting of fresh and aged particles, the above methods would lead to a sharp separation into two groups. The prediction of the source for an atmospherically aged particle is not possible by only using the unmodified patterns of the fresh particles for a comparison.
The differentiation of aged from non-aged particles and their assignment to different particle sources that we provide here, involves a multi-step process: first, the identification of descriptive ions for each model population and second, the identification of signals associated with atmospheric aging in the online-measured single-particle spectra and artificial aging of each model system by these signals. The sum spectra of the model systems were thus recalculated with respect to the identified aging markers. For this process it was assumed that all mineral particles age at the same rate and form the same main chemical components. In our case, however, the intensity ratios of the other signals after simulated aging were of particular importance, as the classification was carried out using only descriptive ion signals specific to each mineral and not taking into account those identified as aging markers. Our aim was not to generate spectra as close to reality as possible, but to generate spectra that allow the classification of atmospherically aged particles using our model systems.
Finally, the HCA of aged and unaged particle populations for classification was carried out. As mentioned before, we used only descriptive ion signals for separation of the aged populations, while for HCA of unaged populations, additional signals were selected. A detailed workflow for selecting descriptive ions, artificial aging and the application of the HCA algorithm is shown in Figure S2.
Figure 2. Dendrograms resulting from a HCA clustering method based on a Pearson correlation distance metric for the single particle spectra from Jungfraujoch atmospheric aerosols, ground and dispersed snow water residues and river sediment. (a) Column clustering for the descriptive signals from and additional carbon cluster ions. (b) The three main cluster that can be further divided into 11 sub-clusters, and (c) after lowering the row tree threshold from 0.86 to 0.50.
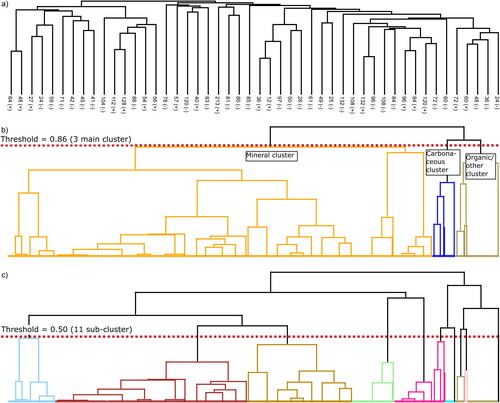
In our study, we computed 180 and 72 h back trajectories for two time periods of interest, using the Hybrid Single-Particle Lagrangian Integrated Trajectory (HYSPLIT) model (Rolph, Stein, and Stunder Citation2017; Stein et al. Citation2015). We used the meteorological data from the National Centers for Environmental Prediction (NCEP) and the National Center for Atmospheric Research (NCAR) called NCEP/NCAR Reanalysis. HYSPLIT generated back trajectories for air masses arriving at the Jungfraujoch research station (3580 m a.s.l., 46°33´N, 7°59´E) every two hours from 17 March (0900 UTC) until 18 March (2100 UTC), from 21 March (2300 UTC) until 23 March (2300 UTC) and from 24 March (0600 UTC) until 24 March (1800 UTC), which corresponds roughly to both Sahara-desert storm events during the measurement period.
3. Results and discussion
3.1. Chemical signatures of rock, sand, river sediment, and snow water residues by SPMS and offline ICP-OES methods
Summed spectra patterns from rock, sand, river sediment and snow water residues are shown in . A total number of 307, 452, 660, and 265 spectra were evaluated from rock, sand, river sediment, and snow water, respectively.
Using sum spectra from a sufficient number of single particle spectra is necessary because of the high inhomogeneity of mineral species. The following evaluation of our data is based on the assumption that all individual particles are completely evaporated/ablated within the mass spectrometer, which is not necessarily the case (Cai, Zelenyuk, and Imre Citation2006). Typical recurring signal patterns of Na+ and K+ (m/z 23 and 39) were found in all particle populations in exceptionally high intensities. The reasons for this were discussed above (Section 2.3). In case of ground rock stone, any modification of the particles prior to the measurement could be excluded. Besides sodium and potassium ions, positive-ion mass spectra from rock stone in were mainly characterized by signals of aluminum (m/z 27), calcium (m/z 40), iron (m/z 56 and 112), titanium oxide (m/z 64) and iron oxide (m/z 72 and 128). Negative-ion mass spectra showed intense signals of PO2– at m/z 63 and PO3– at m/z 79, common components of mineral crust particles and attributed to the P2O5 structures of apatite (El Asri et al. Citation2009). Despite the highly intense signals of PO2– and PO3– in the mass spectra, the amount of phosphorus measured by ICP-OES was lower than 0.1 wt% in all particle populations (see ). The LDI process obviously supports the generation of phosphate ions in the plume by charge exchange reactions (Reilly et al. Citation2000).
Table 1. Comparison between relative intensities (TIC normalized) taken from sum spectra of different mineral particle populations measured by LAMPAS, and aqua-regia-digested samples measured by ICP-OES. Assignments were made based on typical ions found for the respective signals (masses in brackets) in SPMS lab and field studies (Hinz et al. Citation2006; Murphy et al. Citation2006).
This means that the formation of phosphate ions through the absorption of electrons in the plume is clearly preferred over the formation of other ions. Silicon oxide ions SiO2–, SiO3–, HSiO3–, Si2O2–, and Si2O3– (m/z 60, 76, 77, 88, and 104) in are probably suppressed by this effect, resulting in low signal intensities compared to the expected abundance of silicate (>70 wt%) in the mineral populations (Abrecht and Schaltegger Citation1988).
FeO2– (m/z 88) and FeO3– (m/z 104) signals in the rock, sand and sediment spectra show the same trend as the iron content measured by ICP-OES (see ), suggesting iron oxide rather than silicon oxide to be the major source of both signals (Gallavardin, Lohmann, and Cziczo Citation2008). The signal at m/z 56 was not used for evaluation, because of a possible interference with CaO+, especially for the sediment particles. Ti2O+ and Ti2O2+ could be observed at the same m/z values as Fe2+ and Fe2O+ (112 and 128). In rock, both signals most likely represent iron, due to low Ti+ (m/z 48) and TiO+ (m/z 64) signals compared to intense FeO2– and FeO3– signals. In the river sediment we expect an influence of titanium and iron, due to strong Ti+ and TiO+ signals. Both signals were absent in the sand spectra and even Ca+ at m/z 40 was lower than in the river sediment particles, although the mass fraction of calcium in the sand particles is the highest within all mineral populations () (Schmidt et al. Citation2017). The higher Ca+ signal in the sediment particles is supposed to be a result of a better desorption/ionization yield for calcium in the respective mineral matrix due to an enrichment of chemically persistent TiO2, which is known to support desorption/ionization due to its high UV absorption (Castro et al. Citation2008). Reilly et al. showed that the sensitivity for difficult-to-ionize species in matrices that are even more difficult to ionize, is greatly increased (Reilly et al. Citation2000). Probably this effect also contributes to a significant increase of the calcium signal due to a more complex mineral matrix in the sediment particles.
Other ion species indicating the influence of anthropogenic factors are NO2– (m/z 46), NO3– (m/z 62) and carbon cluster ions from C2– (m/z 24) to C15– (m/z 180), mainly found in the river sediment and snow water populations in , respectively. The carbon contents of the four populations were determined from the sum of all signal intensities at m/z 36, 48, 72, and 84 in the negative-ion spectra. Wherever possible, ambiguous m/z values were not used to determine the carbon content, hence the low number of signals. The higher carbon content of the river sediment and snow water populations reflects an anthropogenic influence that was not found for the rock and sand populations. The total nitrate content was calculated by the sum of the negatively charged ions at m/z 46 and 62. Significantly higher nitrate content was found in the snow water particles in , formed under atmospheric conditions. In the snow layer, no continuous replacement of the water-soluble nitrate salts takes place, in contrast to river sediment particles.
In summary, it is very difficult to differentiate between different mineral samples based on relative intensities of the respective ion species on the basis of LDI MS, as these depend very much on the ionization mechanism and the mineral matrix. Therefore, a statement about the identity of the mineral particles, especially on the basis of abundance in combination with the signal intensities of individual ions, even if they are very low, is more suitable. During our statistical evaluation, we focused more on characteristic signals with high abundance and possibly lower intensity in each population, than on common ones which are less abundant but highly intense.
3.2. Identification of descriptive ions and artificial aging
Statistical evaluation of online-measured single particles is a common method for the characterization of different particle populations within a large dataset (Hinz et al. Citation2005; Sierau et al. Citation2014). Here we focus on mineral particles because this was the main particle type measured during the field campaign CLACE-1 with the LAMPAS 2 instrument. To discover different sources of mineral particles in the online data set from CLACE-1, we first identified signals in the lab-measured rock, sand and sediment particles that are descriptive for each population and used only these signals for classification.
3.2.1. Descriptive ions
Signals that were increased or decreased in a model population relative to another model population could be characteristic for the identification of a specific mineral matrix. shows descriptive ions for each model population. Because of the natural variability and the ambiguity of a number of m/z values, there is a high degree of uncertainty even between mineral particles (Gallavardin, Lohmann, and Cziczo Citation2008). Signals at m/z 80, 96, and 97 were attributed to SO3–, SO4–, and HSO4– and were found predominately in rock particles. Signals at m/z 79, 95, 96 and 97 may also belong to mineral phosphate (PO3–, PO4–, HPO4–, H2PO4–) but correlations based on Pearson’s correlation coefficient ρ between m/z 79 and 97 as well as 95 and 96 are poor (ρ = −0.4 and ρ = 0.4).
Table 2. Comparison between rock, sand, and river sediment population with all ions shown to be descriptive for the respective population (Hinz et al. Citation2005; Citation2006; Schmidt et al. Citation2017; Shen et al. Citation2019).
The high intensity of m/z 97 in the rock stone particles is very likely caused by the mineral sulfate content as can be seen from the ICP-OES results (see ), while the same signal in the Jungfraujoch sum spectrum may also be a result of atmospheric aging.
For atmospheric particles it is known that the uptake of SO2 or H2SO4 in the outer liquid layer of the particle, which is an irreversible process, leads to an enrichment of SO42– in the particles (Song and Carmichael Citation1999). Our results indicate that the mineralogical sulfate content in atmospheric particles can become dominant, if a large proportion of igneous rock contributes to this population.
There were no characteristic signals found within the positively charged ions and only very few within the negatively charged ions for the sand particle population. Among some very minor signals, only NaNO3–, C3N–, HSiO2–, H2PO3–/HSO3–, C5H7O–, C7–, and P2O– were found to be significant for this population. This complicates the identification of sand particles within populations of greater diversity such as online-measured particles from different natural and anthropogenic sources.
Rock and river sediment samples shared similar characteristic positive ions while sand and river sediment samples shared similar negative ions. The largest differences between characteristic rock and river sediment signals were found for sulfur oxides and calcium. As the calcium signal is found to be very low in the rock stone particles, the characteristic signals at m/z 56, 57, 112, and 128 should represent iron, iron oxides or titanium oxides rather than calcium oxides. A unique feature of the rock stone is the signal at m/z 213 in the positive-ion spectra. The isotopologue pattern of this signal is similar to that of K3SO4+ or K3HPO4+ but the mass resolving power of the mass spectrometer is not sufficient for an exact determination. However, correlations between 213 and PO3– at m/z 79 (ρ = −0.2) as well as 213 and SO4– at m/z 96 (ρ = 0.7) suggest the formation of K3SO4+. Often it is claimed that the signal at 213 is an indicator for atmospheric aging or biomass burning, but here we found this signal almost exclusively in the unaltered rock particles with a high share of 24% (Sierau et al. Citation2014; Zauscher et al. Citation2013). Due to the high content of sulfate in the rock samples, the formation of K3SO4+ in the laser plume, from easily accessible ionic components, is very likely. The signal is not significantly enhanced in our atmospheric mineral samples. This example shows that the identification of ion signals, even with only nominal mass resolution, is possible if both polarities are measured simultaneously from one particle.
3.2.2. Characteristic signals indicating the aging of particles
In a second step, signals related to atmospheric aging were identified. Here the rock and sand particles served as a reference standard since no direct aging effects are expected for these populations. Details on the identification of ions related to atmospheric aging and consecutive theoretical aging of sand, rock and sediment spectra can be found in Sect. 2.3 and as detailed workflow in the SI (Section 2).
Ions that were found to play a significant role in atmospheric aging were only found within the negatively charged ions, for example H– and H2–/He– as a result of a higher water content and acidity in the atmospheric particles after uptake of acidic trace gases. Cl–, NO2–, or NO3– are other typical ions found in aged particles. Nitrogen-containing ions, especially the higher oxidized species are the quantitatively most important indicators for aged mineral aerosol particles in polluted air (Hinz et al. Citation2005; Laskin et al. Citation2005). The growth rate of nitrate compounds, particularly for particles >2 µm is fast compared to other species such as chloride (Song and Carmichael Citation1999). The detected nitrate can either be formed by the direct uptake of ammonium nitrate, formed by neutralization of gaseous HNO3 with NH3 (Brauer, Koutrakis, and Spengler Citation1989), or by the conversion of gaseous precursors like NO2 or N2O5 (Russell, Cass, and Seinfeld Citation1986). Alkaline oxides such as K2O, Na2O, or CaO, present in mineral particles, easily form water-soluble alkaline nitrate species. This reaction can also take place with lowly soluble CaCO3. Calcium carbonate reacts to more soluble Ca(NO3)2 at the presence of HNO3 (Laskin et al. Citation2005). The chloride species in aged aerosol particles are expected to be formed mainly at marine boundary layers, where the evaporation of volatile HCl from sea salt particles creates a high HCl concentration environment. In the case of our measurements we expect only a small amount of sea salt particles, but a high amount of mineral dust, which in turn is able to form hygroscopic CaCl2 under these conditions (Tobo et al. Citation2010).
The reactions above lead to strong differences in hygroscopicity, solubility and light scattering as well as absorption behavior of the reaction products Ca(NO3)2 or CaCl2 compared to CaCO3. Henning et al. found CaCO3 to be the major compound responsible for high nitrate content in particles > 1 µm after exposure to anthropogenic influences (Henning et al. Citation2003).
Some species are not expected to be formed during particle transport in the atmosphere but instead are chemically persistent and insoluble, such as Mg+ from MgCO3, Ti+, or TiO+ from TiO2 and Al+ from alumosilicates. These ions are more likely enriched in the particles while more soluble components like potassium and sodium salts are washed out. Changes in the signal patterns of these ions were not significant enough, so they were not identified as products of atmospheric aging. As a result, the influence of atmospheric processes is mainly reflected in the spectra of negatively charged ions, particularly by the ratio of nitrate compared to other compounds like for example phosphate, while the signal patterns of positively charged ions differ only slightly between fresh and aged populations. The signals identified above were used for recalculation of the signal patterns in rock and sand spectra in order to prevent those spectra to be classified as distinct groups, statistically separated from the Jungfraujoch atmospheric aerosol spectra. Prior studies with laboratory-aged particles showed very similar changes in the signal patterns, as we got after our theoretical aging approach (Hinz et al. Citation2006).
3.3. Statistical results from lab and field measurements
The complete data set (3432 spectra) used for the statistical evaluation consists of 2505 atmospheric single-particle spectra from the Jungfraujoch, 660 single-particle spectra from dispersed, ground river sediment, 265 single-particle spectra from dispersed, ground snow residue and the artificially aged as well as unaltered sum spectra of rock and sand populations.
The descriptive signals identified in were used for clustering, together with additional carbon chain fragments from C2– to C7– and C1+ to C12+, which were included in the signal list to count for organic and carbonaceous particles in positive- and negative-ion spectra. The resulting dendrograms are shown in . Dendrograms including heatmap visualization are shown in Figure S4. Column clustering of the chosen m/z values in shows groups of ions, with high correlations. For example, m/z (+) 56, 54, 112, 128, and m/z (-) 88, 104 were grouped together.
The respective ions arise from iron and iron oxides and are well separated from titanium and titanium oxide at m/z (+) 48 and 64. Correlations between different negative and positive ions, belonging to the same compound, enabled the separation of spectra with similar nominal-mass signal patterns, thus making the Pearson-based clustering well suited for classification of low-mass-resolution bipolar mass spectra.
For the generation of spectra patterns and to determine the quantitative composition of the Jungfraujoch single-particles, snow residues and sediments (), a mean spectrum from all single-particle spectra contained within a chosen cluster (branch of the joining tree) was calculated. Changing the threshold in the joining tree of the dendrogram between 0.86 (), 0.50 (), and 0.17 (Figure S5) allowed to identify 3 main clusters, 11 sub-clusters and 24 clusters (source apportionment), respectively. The artificially aged sum spectra were used as reference spectra in this classification to find online measured single-particle spectra that are most similar to aged sand and rock spectra.
Figure 3. Spectra patterns derived from a HCA-clustering method based on a Pearson correlation distance metric for atmospheric single-particle spectra from Jungfraujoch, lab-measured snow water residues and river sediment. The patterns were assigned to different particle classes. The number of single particle spectra used to form the spectra patterns is given in brackets. Three main patterns were assigned to mineral particles (3177) (a), carbonaceous particles (160) (b), and organic particles (95) (c). Refining those main clusters allowed to identify 11 sub-spectra patterns (d) to (n). Sub spectra patterns for mineral particles were Mg/Al-rich (322) (d), iron-rich (1340) (g), TiO2-rich (738) (j), phosphate-rich (293) (m), calcium sediment (267) (k), and other (215) (n). For the carbonaceous main cluster only one carbon/secondary organic aerosol (SOA) (92) (e) and one soot sub-cluster (68) (h) were found. Organic particles were separated in organic NOx/SOA (70) (f), CN-rich organic (20) (i), and hydrocarbon like organic (HOA)/SOA (5) (l).
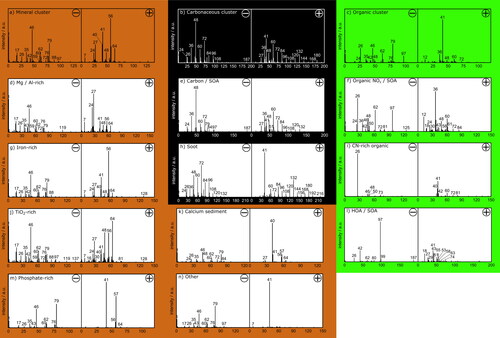
A second clustering was carried out by using the unaltered instead of artificially aged rock and sand sum spectra. These were used here as a reference to identify “fresh” Jungfraujoch single-particle spectra that most closely resemble the rock and sand spectra patterns. To separate the very similar rock and sand sum spectra (see Figure S6), the distance threshold was lowered to 0.22, which resulted in 21 clusters. To pay more attention to aging-related features in this classification, the negatively charged ions with masses 46, 62, 63, and 79 (NO2–, NO3–, PO2–, and PO3–) were additionally used for HCA. It was observed that fresh mineral particles, besides their metal and metal oxide signals, were mainly characterized by their PO3– to NO2– signal intensity ratios. Higher values of PO3– indicate less-aged particles (Hinz et al. Citation2006). Some Jungfraujoch spectra appeared in the “fresh” as well as in the aged clusters, as these could not be clearly assigned due to slight aging characteristics. We decided to count these spectra as “fresh.”
3.3.1. Particle clusters from JFJ, snow, and sediment samples
In a first step, a rough separation of particles, depending on the major characteristic ions was conducted. This resulted in a separation of three well defined main clusters (). These show carbonaceous, organic and mineral populations. The mineral particles make up 92.6% of the total particle population of all samples with a vacuum aerodynamic diameter between 400 and 5000 nm and an average of 1272 nm. Carbonaceous and organic particles are on average smaller with 1142 and 1136 nm, respectively.
From the three main clusters, a more refined classification into 11 sub-clusters was done. The sub-cluster patterns are shown in ). Due to the removal of the sodium and potassium signals at m/z 23 and 39, the remaining potassium isotopic signal at m/z 41 is dominant in many spectra (see ). The signal at m/z 41 was not removed because it can be an indicator for hydrocarbons (C3H5+) as well. All clusters exhibited clearly distinguishable ion patterns which enabled a separate consideration of each individual cluster origin.
The carbonaceous cluster () is divided into two sub-clusters, a soot cluster in , showing only carbon chain fragments from C2– to C11– and from C5+ to C18+ and a carbon/secondary organic aerosol (SOA) cluster in with shorter carbon-chain fragments and additional Al+/C2H3+, C4H2+, C4H3+ ions. Especially C4H2+ and C4H3+ are suitable diagnostic fragments for aromatic rings. The observed ions indicate the presence of polyaromatic hydrocarbons, which, due to their high fragmentation, mostly cannot be observed as complete molecular ions (Schmidt et al. Citation2017; Silva and Prather Citation2000). Schmidt et al. observed similar ions for a class of particles, which they assigned to cigarette smoke (Schmidt et al. Citation2017). In our case we found a higher degree of internal mixing for this particle cluster, including more and longer carbon-chain fragments. The negatively charged ion at m/z 187 (C8H11O5–) is referred to as a marker of α-pinene and indicates the absorption of SOA on soot or hydrocarbon particles followed by oxidation, resulting in the formation of 2-hydroxyterpenylic acid (Kahnt et al. Citation2014).
The smallest group of particles (), containing only 95 spectra, was assigned to organic material. The sub-cluster in represents oxidized organic material, mainly characterized by positively charged C+, C3+, C5+/C3H10N+, and C3H8N+ (m/z 58) ions. The signal at m/z 43, referring to C2H3O+, a marker of SOA single particle spectra, is elevated likewise (Dall'Osto and Harrison Citation2012; Qin et al. Citation2012; Schmidt et al. Citation2017). CN–, NO3–, HSO4–, and C3N– (m/z 50) dominated the negatively charged ions besides less intense signals of carbon-chain fragments. Signals at m/z 125, 97, and 81 may be associated with the fragmentation of 2-hydroxyterpenylic acid. The fragment at m/z 125 is likely produced by loss of CO2 and H2O from m/z 187. The fragment at m/z 97 was caused by loss of CO from m/z 125 and m/z 81 by loss of CO2 from m/z 125. The fragmentation patterns found are in very good agreement with results obtained by electrospray ionization tandem MS (ESI-MS2) of extracted aerosol filter samples (Kahnt et al. Citation2014). The signal at m/z 97 can also arise from HSO4– as described before, while m/z 125 can also be assigned to HN2O6– (Silva and Prather Citation2000). The formation of 2-hydroxyterpenylic acid involves NO as reactant species and β-pinene is oxidized by NO3, which explains the higher abundance of NO2–, NO3–, and NO+ (at m/z 30) signals in this cluster (Kahnt et al. Citation2014; Zheng et al. Citation2005). C3N– and in particular C3H8N+ and C3H10N+ indicate a choline-like core structure, as for example in phosphatidylcholines. This could be an indication of a plant-based origin of the particle class. The latter two are known choline fragments in ESI-MS2 (Chary et al. Citation2012). The CN-rich cluster in shows very high abundance of CN–, which is generally associated with organic single particles (Bi et al. Citation2011). Besides that, there are hardly any characteristic signals in this class. SOA-like particles including hydrocarbon-like organic (HOA) species without any additional carbon chain fragments are summarized in . Major fragment ions in this sub-cluster were m/z 97 and 99, the latter due to a loss of two CO2 groups from m/z 187. Positively charged ions in this cluster refer to hydrocarbon chain fragments at m/z 29, 43, 57… (CnH2n+1+) and m/z 27, 41, 55, 69… (CnH2n-1+) typically found for those particle type (Cross et al. Citation2009).
The numerically largest cluster with 3177 spectra in contains 6 different mineral sub-clusters shown in . These vary mainly in their metal and metal oxide intensities. Additionally, different degrees of atmospheric aging, represented by the negatively charged ions at m/z 35 (Cl–), 46 (NO2–), and 62 (NO3–), are obvious. While the mineral sub-clusters (d), (g), and (j) show a moderate amount of calcium, cluster 3 (k) almost exclusively consists of calcium and shows, together with cluster 3 m), significantly enhanced intensities at m/z 57 within the positively charged ions. This signal is mainly caused by the presence of CaOH+ rather than Fe+ or C4H9+. The phosphate-rich cluster in is an exception with respect to age-related signals. This sub-cluster is characterized by low nitrate and chloride intensities compared to phosphate. Particles in this cluster cannot be identified as fresh or aged, either because the phosphate was converted into easily soluble components which subsequently dissolved in snow, rain or river water, leaving undissolved phosphate in the mineral matrix, or because the particles were only slightly aged from the beginning (Tobo et al. Citation2010). As mentioned before, phosphate signals are boosted by matrix effects, so only small amounts of phosphate are needed to produce sufficiently high signals once compounds that are easier to ionize are no longer present. As a result, these particles are mainly found in the river sediment population. summarizes particles which were difficult to assign to a distinct group. Due to the lack of characteristic signals in the spectra patterns, this cluster is labeled as “other.” Particles assigned to this cluster typically show only very low intensities of ions in general, except for potassium, sodium, phosphate and silicon. However, these signals were mainly associated with mineral particles. Therefore, particles in this cluster were manually assigned to the group of mineral particles due to a higher consistency of the negatively charged ions with the mineral cluster patterns. Large proportions of particles from the snow water residues (20.8%) and river sediment (10.5%) were classified as “other” particles (see ).
Figure 4. Distribution of particles over different clusters within the populations of dispersed, ground river sediment, dispersed, ground snow water residues and atmospheric aerosol particles online measured at Jungfraujoch. The categorization is based on the eleven clusters identified in . The top row summarizes the three main groups but counting the “other” cluster to the mineral main cluster.
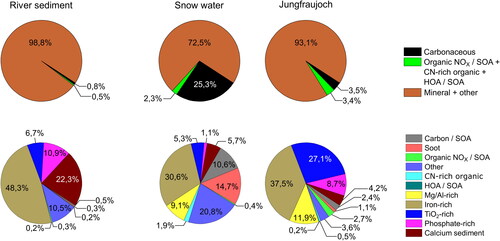
The identified particle classes provide a good overview of the particle composition and internal mixing degree of the atmospheric particle population at the Jungfraujoch site. In this study, the different internally mixed particle types are clearly dominated by mineral particles with strong aging-related signals.
3.3.2. Composition of JFJ, snow, and river sediment samples
The composition of the Jungfraujoch, snow water and sediment populations can be illustrated by the proportion of spectra in the previously formed clusters. shows the distribution of particles based on the clusters identified in . The first row of pie charts gives an overview of the distribution for the three main clusters within one population.
Comparison of the composition of snow water residues and Jungfraujoch aerosol particles is difficult. First, because snow water residues are an accumulation of particles over a longer period of time, whereas the Jungfraujoch data represent only a snapshot during the measurement campaign. On the other hand, the particles of the snow water residues were subsequently altered by dissolution, partitioning and agglomeration. The effect of accumulation is particularly evident in the high proportion of carbonaceous particles with a share of over 25% within the snow water spectra, especially in comparison to the atmospheric particle population on the Jungfraujoch. This proportion corresponds to the typical composition of the Jungfraujoch aerosol, which was measured, for example, during the CLACE-6 using a Single Particle Laser Ablation Time-of-Flight Mass Spectrometer (SPLAT), but is significantly lower than the proportion we see in our online measurements (Kamphus et al. Citation2010). One reason for this is the nozzle inlet system used in the LAMPAS 2, whose lower detection limit is around 500 nm and which can therefore detect the smaller carbon particles to a limited extent, compared to the aerodynamic lens used in the SPLAT instrument. We assume that these small carbon particles will agglomerate in the snow water and thus enter the measurable size range of the LAMPAS device. On the other hand, the strong Sahara sandstorm activity during the measuring period is of course responsible for the low proportion of carbon particles measured online.
The iron-rich, Mg/Al-rich, calcium sediment and organic NOx/SOA clusters exhibit very similar proportions within the Jungfraujoch and snow water populations. Greater differences exist for TiO2-rich and phosphate-rich clusters. The dissolution of nitrate and other easily soluble compounds in the snow water and river sediment particles is expected to be the main cause for the high proportion of “other” particles in this populations. Due to the lack of further characteristic signals in this class, the original particle composition before dissolving in water is no longer accessible.
More than 70% of the river sediment particles were grouped within the iron-rich and calcium-sediment clusters. The high proportion of 22% calcium-sediment suggests that softer minerals like calcite accumulate within these particle populations due to their higher erosion. The iron-rich cluster in represents the largest group of particles within all three populations. The aged rock and sand particle sum spectra belong to this large cluster.
Deeper clustering into 24 clusters was able to separate these very similar particle types, whereas the most important distinguishing feature was the calcium content, as already confirmed by the ICP-MS measurements. These showed that the sand particles consist of 22% calcium, which corresponds to 45% CaCO3, as long as CaCO3 was the only source of calcium. So, this particle type consisted of roughly 50% igneous rock and 50% calcite, while the rock stone from the Jungfraujoch site was poor in calcium and resembled a more igneous-rock-like composition. The deeper clustering enables a more accurate identification of these types of mineral particles within the Jungfraujoch atmospheric aerosol population. As a result, 18% of the online-measured particles from the Jungfraujoch site were more igneous-rock-like while only 3% resembled the Morocco-sand-like signal pattern. The remaining 16% of the iron-rich particles in the Jungfraujoch population showed higher titanium ratios than the rock and sand particles. More than 23% of the river sediment particles belonged to the Morocco sand-like cluster.
3.3.3. Source apportionment analysis and atmospheric aging of particles
For the source apportionment of the different particle classes, we divided our complete single-particle measurement data from CLACE-1 into three episodes, from 17 March at 9 AM to 18 March at 10 PM, from 21 March at 11 PM to 23 March at midnight and from 24 March at 5 AM to 24 March at 7 PM. The temperature during the whole measurement campaign ranged from −24.3 to −7.7 °C. shows the total particle composition based on 8 selected particle classes in two-hour intervals. The number of particles was normalized to the respective interval. A total of 8 classes were selected. From the cluster analysis of the aged particles with 24 classes, 6 classes were used, which represent aged sand particles (calcium rich iron), aged rock particles (calcium poor iron), river sediment particles (calcium sediment), titanium oxide particles (TiO2-rich), carbonaceous particles (Carbonaceous), and organic particles (organic NOx/SOA + CN-rich organic + HOA/SOA). From a classification with unaged sand and rock sum spectra, the less-aged sand (less-aged calcium rich) and rock particle (less-aged igneous rock) classes were included.
Figure 5. Relative number of particles per cluster in 2-h intervals (a), from 8 out of a 24/21 clusters. Particle groups were created based on two different classifications. First, the 24 clusters with artificially aged particles and second, a classification into 21 clusters with the unaltered sum spectra from rock and sand particles. The second classification was used for comparison to identify fresh mineral particles within the populations from Jungfraujoch and snow water. Particle sizes determined by LAMPAS 2 during the measurement period as mean of two hours of recording (black dots) and numbers of detected particles in one hour intervals shown as red bars (b). 180 h (c) and 72 h back trajectories (d, e), computed by HYSPLIT for the three measurement periods. The colors correspond to the trajectories simulated every two hours as shown in the time-altitude diagram below.
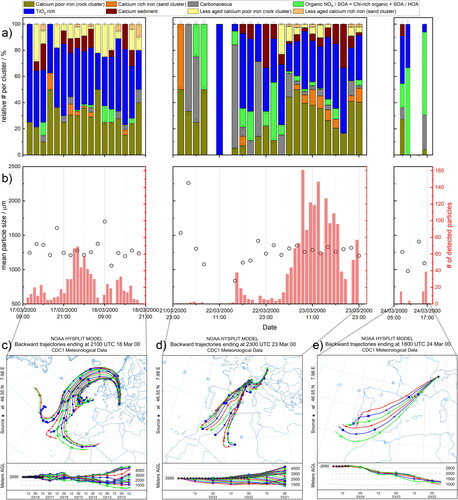
The presented results show only a small section of the particles actually present at the measurement location. Especially during some intervals, as for example on 21 March between 11 PM and 3 PM and on 24 March around noon, either the number of particles measured was too low for a statical evaluation or the LAMPAS 2 instrument was in a maintenance phase. The lower number of particles detected on these days could also be a result of the limited sampling efficiency of the LAMPAS 2 inlet system, which is in the range of 10−3 for particles with 800 nm diameter (Trimborn, Hinz, and Spengler Citation2000). The results for these periods should therefore be interpreted carefully. The statistical significance of the remaining measurement days is higher and more reliable, especially for the mineral particles examined here. The data points in show the mean particle sizes recorded by the LAMPAS 2 device for two-hour intervals of data recording. In addition, the sum of measured particles in one-hour intervals is shown as red columns. All findings are supported by either 180-h () or 72-h back trajectories ().
In our measurement period, two Sahara sandstorm events were recorded, reaching from 16 March in the evening until 18 March and on 23 March and 24 March. In a previous study by Hinz et al., based on the same online data set, two episodes were observed during this period of time in which the number of super-micron particles (particle diameter >1 µm) measured by an optical particle counter (OPC) increased significantly (Hinz et al. Citation2005). Both events had an above-average influence on the particle composition. Their effect on various particle classes is examined in more detail below.
At times with no or less desert-sand influence, TiO2-rich, carbonaceous, organic, aged-rock, and calcium-sediment particles prevailed. The calcium-sediment-like and TiO2-rich particles were found on all measurement days, except 22 March until 3 PM, where the total number of detected particles was the lowest during the complete measurement period. On 22 and 24 March, the carbonaceous and organic particles contributed most to the total particle count, suggesting the influence of local traffic and industrial emissions (Hinz et al. Citation2005). Especially on 22 March, this was accompanied by a strong reduction in particle size over the course of the day, as can be seen in . The increase of the fraction of organic particles on 24 March is far above all other clusters and most probably originated from natural organic sources. On both days, the trajectories mainly showed the influence of continental particle sources, since the wind came from the east on 22 March, while on 23 March () in the evening and 24 March (), mainly air masses from lower altitudes on the Spanish mainland reached the measuring station. Particularly within these periods, on 22 March around 11 PM and 23 March at 7 PM, the proportion of calcium-sediment particles was highest. Typically, the bottom rock in the alpine region consists of calcium-sediment mineral which is comparably soft, and erosion due to wind and weather is more likely than for other minerals. Such particles are found in atmospheric aerosols more frequently if their source is close to the measurement site. Those particles make up 4.1% of the total number of particles measured. On 23 March in the evening, the calcium sediment particles most likely originated from the Helvetic nappes, which surrounds the Aar Massif from the southwest to the north on the edges of the Alps, while on 22 March, air masses crossed the Dolomites. Both geological formations consist of calcareous sedimentary rocks and are located exactly in the directions of the air currents on the respective days. Due to the lower altitude, most of these regions are no longer snow-covered in spring, which makes it easy to blow up the eroded sediment. It can be assumed that the particle populations measured on 22 and 24 March reflect the typical composition of the aerosol at the Jungfraujoch site. This is supported by measurements of Cozic et al., showing a similar trend with organic matter as the main component in the fine mode for the measurement period in February, March, July and August 2005 at the Jungfraujoch (Cozic et al. Citation2008).
On all other measurement days, the effect of mineral dust transport from desert storm events prevailed. In total, higher ratios of sand and rock (aged and less aged), aged TiO2 and calcium sediment particles were observed during 17/18 March (period 1) and 23 March (period 2) compared to 21/22 March and 24 March, suggesting the strong influence of desert sand for both periods. Especially the 4 sand and rock clusters were found to correlate reasonably well with the two Sahara desert storm events in the year 2000. Their share on the total population was about 25%. The main differences between the two events were the direction of the wind that transported the Sahara sand particles to the measuring station and their residence time in the atmosphere. When the first particles during these events arrived (March 17 at 1 PM and 7 PM, March 18 at 7 AM and March 23 at 3 AM), an elevated contribution of less-aged calcium-poor iron particles (rock) to the total population was observed, while the percentage of aged calcium-poor iron particles (rock) and less-aged calcium-rich iron particles (sand) increased with a two- to four-hour delay. Especially the less-aged particle types occurred exclusively on 17/18 March and 23 March and accounted for 4.2% of the total number of particles measured. This indicates that the fresh particles stemmed predominately from desert sand, transported to the measurement site without undergoing extensive alterations in the atmosphere. Another two to four hours later, the first aged sand particles (calcium rich iron) were detected (17 March at 5 PM and 11 PM and on 23 March at 7 AM), while the proportion of aged and less-aged rock particles slowly decreased (23 March between 1 and 9 PM).
For the first event, the back trajectories in show the influence of air, reaching the measurement site from northern directions. For those particles, an increase in size on 17 March at 7 PM and 18 March from 5 to 9 AM, due to coating effects, was observed (). This effect is most likely due to increased air pollution in urban areas north of the Alps and longer transportation times of up to 180 h, as no such increase in size was observed for the second Sahara dust event, where transportation times were in the range of 72 h (). Due to longer transportation times for the first event, a stronger removal of particles by sedimentation and scavenging was obtained, resulting in lower total particle numbers. This is especially true for the aged sand particles. Increased mixing of different particle sources, as some of the air masses came from high altitudes above the Atlantic, was also expected.
The development from fresh to aged particles becomes particularly clear during the second event on 23 March between 3 AM and 19 PM, which was almost exclusively influenced by Sahara sand, transported from southwest directions, as can be seen in . We saw a strong increase of TiO2-rich and calcium sediment particle proportions when the source of the trajectories changed from Africa to the European mainland during evening of 23 March. The TiO2-rich particles were most likely exposed to a higher level of industrial or traffic pollution, which occurs mainly in lower altitude. They can be hardly addressed to a certain particle source but are typically transported in the atmosphere over a longer period of time, resulting in high nitrate and chloride fractions. This type of particles can be considered as strongly aged. The low amount of TiO2 in most minerals (<1 wt%), together with its chemical stability and high UV absorption, promotes intense Ti+ and TiO+ signals in this particle class. Especially the TiO2-rich and aged rock particles (calcium-poor iron) can also originate from local sources, where they were eroded and processed over a longer period of time and then transported to the measurement site by up winds. These particles, due to their strong aging features, can no longer be identified as coming from local sources.
A combination of different phenomena contributed to the observed effects of the successive mineral cluster appearance during both sandstorm events. The iron-rich particles of the resuspended Saharan sand consisted mainly of silicates and calcium carbonate. Especially the calcium cations react with the inorganic acids present in the atmosphere and thus significantly cause the aging of the particles. Model calculations show that due to the large number of emitted particles, a complete neutralization of the gaseous acids in the area of the dust outflow is probable (Abdelkader et al. Citation2017). Due to the hygroscopic properties of the salts formed, the aged particles were washed out of the atmosphere much more efficiently, while less-aged particles remained. Especially the silicate-rich particles are very stable, both chemically and physically, and were the first to arrive at the measuring station. Typically a few hours later during the sandstorm events, however, the proportion of aged sand and rock particles increased, compared to other particle classes. A similar effect was observed by Kandler et al. during single-particle measurements of sand storm events in the Moroccan desert (Kandler et al. 2009). The proportion of less-aged calcium-rich particles (sand cluster) was very low due to their fast conversion to aged particles, followed by scavenging during atmospheric transport.
In addition to the study by Hinz et al. in 2005, we were now able to correlate different stages of particle aging with particle sources and transport times. Anthropogenic influences were found predominately for particles transported in higher altitude over longer distances, as on 17 and 18 March, or for particles transported at lower altitudes, even if their transport is comparably fast. In the latter case it is very likely that a high amount of CaCO3 in the original particles is responsible for the high proportion of age-related signals, even if they were only shortly exposed to anthropogenic influences (Henning et al. Citation2003).
4. Conclusion
With the LAMPAS 3 instrument, four different groups of mineral particles (rock, sand, sediment and snow water residues) were measured in the lab and compared to data from a measurement campaign at the Jungfraujoch in the Swiss Alps between 17 March, 2000 and 24 March, 2000. From the laboratory measurements, typical representative bipolar mineral particle spectra patterns were generated. Patterns from rock and sand particles provided information about fresh mineral particles, only slightly influenced by further anthropogenic or natural factors. The Rhine river sediment and snow water residue samples showed enhanced modifications and alterations due to mechanical erosion, mixing and solvation. The snow water residues were directly compared to the field-measured atmospherically aged particles from the Jungfraujoch. Source apportionment of mineral particles within the Jungfraujoch population was realized by using a HCA clustering approach with fresh as well as artificially aged sum spectra from sand and rock by only using a very limited number of descriptive ions, found for each population. A distinction into different particle classes as well as different stages of particle aging was possible. Due to the fast conversion of CaCO3 to Ca(NO3)2 in the presence of HNO3 in the atmosphere, particle spectra containing low nitrate signals at m/z 46 and 62 are expected to arise either from local sources or from remote locations only if they are chemically persistent or if their transport is fast. A determination of the percentage of particles arising from local and remote locations at different time points during the measurement period was achieved. It was found that the composition of the Jungfraujoch particles measured during the investigation period strongly diverged from the snow-water distribution due to temporal Sahara sandstorm events. At the beginning of these events, elevated fractions of fresh mineral particles were found that correlated nicely with the lab-measured Sahara-desert sand and rock particle populations. With increasing duration of the sandstorm events, a change from fresh to aged particles was observed, while during the periods between the sandstorm events only aged mineral and calcium sediment particles were found.
The fresh and lowly soluble mineral particles during the sandstorm events as well as the carbonaceous particles on the snow layer at the top regions of the Alps enhance the surface warming effect in this region. For the carbonaceous particles this is particularly important throughout the year, whereas the fresh particles only play a greater role at the beginning of the sandstorm events. Although the sandstorm events are very short, the mass concentrations of mineral dust are expected to be many times higher than the continuous sedimentation of BC particles over the year. Therefore, the reflectivity of snow, especially in the visible wavelength range, is much more influenced by mineral dust than by BC. Yasunari et al. could show a decrease in albedo of 20 to 40% by mineral dust versus 15 to 20% by BC (Yasunari et al. Citation2011). Especially in spring, where less fresh snowfall is expected, this results in faster snow melting. After sedimentation of the fresh particles, the increased amount of hygroscopic, aged particles in the air additionally supports light scattering and cloud formation. Titanium oxide and calcium sediment-like particles were observed during all measurement days in very similar ratios. While the first ones are thought to result from remote sources due to their high extent of atmospheric aging, the latter ones more likely stem from local sources, like the typical bottom rock of the Alpine region.
Supplemental Material
Download PDF (1.3 MB)Additional information
Funding
References
- Abdelkader, M., S. Metzger, B. Steil, K. Klingmüller, H. Tost, A. Pozzer, G. L. Stenchikov, L. Barrie, and J. Lelieveld. 2017. Sensitivity of transatlantic dust transport to chemical aging and related atmospheric processes. Atmos. Chem. Phys. 17 (6):3799–821. doi:https://doi.org/10.5194/acp-17-3799-2017.
- Abrecht, J., and U. Schaltegger. 1988. Aplitic intrusions in the central aar massif basement - geology, petrography and rb-sr data. Eclogae Geol. Helv. 81:227–39.
- Albrecht, B. A. 1989. Aerosols, cloud microphysics, and fractional cloudiness. Science 245 (4923):1227–30. doi:https://doi.org/10.1126/science.245.4923.1227.
- Allen, R. J., W. Landuyt, and S. T. Rumbold. 2016. An increase in aerosol burden and radiative effects in a warmer world. Nature Clim. Change 6 (3):269–74. doi:https://doi.org/10.1038/nclimate2827.
- Andreae, M. O., and A. Gelencser. 2006. Black carbon or brown carbon? The nature of light-absorbing carbonaceous aerosols. Atmos. Chem. Phys. 6 (10):3131–48. doi:https://doi.org/10.5194/acp-6-3131-2006.
- Azar, A. T., S. A. El-Said, and A. E. Hassanien. 2013. Fuzzy and hard clustering analysis for thyroid disease. Comput Methods Programs Biomed. 111 (1):1–16. doi:https://doi.org/10.1016/j.cmpb.2013.01.002.
- Bi, X. H., G. H. Zhang, L. Li, X. M. Wang, M. Li, G. Y. Sheng, J. M. Fu, and Z. Zhou. 2011. Mixing state of biomass burning particles by single particle aerosol mass spectrometer in the urban area of prd, china. Atmos. Environ. 45 (20):3447–53. doi:https://doi.org/10.1016/j.atmosenv.2011.03.034.
- Brauer, M., P. Koutrakis, and J. D. Spengler. 1989. Personal exposures to acidic aerosols and gases. Environ. Sci. Technol. 23 (11):1408–12. doi:https://doi.org/10.1021/es00069a013.
- Cai, Y., A. Zelenyuk, and D. Imre. 2006. A high resolution study of the effect of morphology on the mass spectra of single psl particles with na-containing layers and nodules. Aerosol Sci. Technol. 40 (12):1111–22. doi:https://doi.org/10.1080/02786820601001677.
- Castro, A. L., P. J. A. Madeira, M. R. Nunes, F. M. Costa, and M. H. Florêncio. 2008. Titanium dioxide anatase as matrix for matrix-assisted laser desorption/ionization analysis of small molecules. Rapid Commun Mass Spectrom 22 (23):3761–6. doi:https://doi.org/10.1002/rcm.3795.
- Charlson, R. J., J. Langner, H. Rodhe, C. B. Leovy, and S. G. Warren. 1991. Perturbation of the northern-hemisphere radiative balance by backscattering from anthropogenic sulfate aerosols. Tellus Series a-Dynamic Meteorology and Oceanography 43 (4):152–63. doi:https://doi.org/10.1034/j.1600-0870.1991.00013.x.
- Chary, V. N., C. D. Kumar, M. Vairamani, and S. Prabhakar. 2012. Characterization of amino acid-derived betaines by electrospray ionization tandem mass spectrometry. J Mass Spectrom. 47 (1):79–88. doi:https://doi.org/10.1002/jms.2029.
- Cozic, J., B. Verheggen, E. Weingartner, J. Crosier, K. N. Bower, M. Flynn, H. Coe, S. Henning, M. Steinbacher, S. Henne, et al. 2008. Chemical composition of free tropospheric aerosol for pm1 and coarse mode at the high alpine site jungfraujoch. Atmos. Chem. Phys. 8 (2):407–23. doi:https://doi.org/10.5194/acp-8-407-2008.
- Cross, E. S., T. B. Onasch, M. Canagaratna, J. T. Jayne, J. Kimmel, X. Y. Yu, M. L. Alexander, D. R. Worsnop, and P. Davidovits. 2009. Single particle characterization using a light scattering module coupled to a time-of-flight aerosol mass spectrometer. Atmos. Chem. Phys. 9 (20):7769–93. doi:https://doi.org/10.5194/acp-9-7769-2009.
- Dall'Osto, M., and R. M. Harrison. 2012. Urban organic aerosols measured by single particle mass spectrometry in the megacity of london. Atmos. Chem. Phys. 12 (9):4127–42. doi:https://doi.org/10.5194/acp-12-4127-2012.
- El Asri, S., A. Laghzizil, A. Alaoui, A. Saoiabi, R. M’Hamdi, K. El Abbassi, and A. Hakam. 2009. Structure and thermal behaviors of moroccan phosphate rock (bengurir). J. Therm. Anal. Calorim. 95 (1):15–9. doi:https://doi.org/10.1007/s10973-008-9114-z.
- Gallavardin, S., U. Lohmann, and D. Cziczo. 2008. Analysis and differentiation of mineral dust by single particle laser mass spectrometry. Int. J. Mass Spectrom. 274 (1-3):56–63. doi:https://doi.org/10.1016/j.ijms.2008.04.031.
- Gautam, R., N. C. Hsu, W. K. M. Lau, and T. J. Yasunari. 2013. Satellite observations of desert dust-induced himalayan snow darkening. Geophys. Res. Lett. 40 (5):988–93. doi:https://doi.org/10.1002/grl.50226.
- Hansen, J., and L. Nazarenko. 2004. Soot climate forcing via snow and ice albedos. Proc Natl Acad Sci U S A ... 101 (2):423–8. doi:https://doi.org/10.1073/pnas.2237157100.
- Haywood, J. M., and K. P. Shine. 1995. The effect of anthropogenic sulfate and soot aerosol on the clear-sky planetary radiation budget. Geophys. Res. Lett. 22 (5):603–6. doi:https://doi.org/10.1029/95GL00075.
- Henning, S., E. Weingartner, M. Schwikowski, H. W. Gaggeler, R. Gehrig, K. P. Hinz, A. Trimborn, B. Spengler, and U. Baltensperger. 2003. Seasonal variation of water-soluble ions of the aerosol at the high-alpine site jungfraujoch (3580 m asl). J. Geophys. Res 108 (D1):10. doi:https://doi.org/10.1029/2002JD002439.
- Hinz, K. P., N. Erdmann, C. Gruning, and B. Spengler. 2006. Comparative parallel characterization of particle populations with two mass spectrometric systems lampas 2 and spass. Int. J. Mass Spectrom. 258 (1-3):151–66. doi:https://doi.org/10.1016/j.ijms.2006.09.008.
- Hinz, K.-P., E. Gelhausen, K.-C. Schäfer, Z. Takats, and B. Spengler. 2011. Characterization of surgical aerosols by the compact single-particle mass spectrometer lampas 3. Anal. Bioanal. Chem. 401 (10):3165–72. doi:https://doi.org/10.1007/s00216-011-5465-6.
- Hinz, K. P., M. Greweling, F. Drews, and B. Spengler. 1999. Data processing in on-line laser mass spectrometry of inorganic, organic, or biological airborne particles. J. Am. Soc. Mass Spectrom. 10 (7):648–60. doi:https://doi.org/10.1016/S1044-0305(99)00028-8.
- Hinz, K. P., R. Kaufmann, and B. Spengler. 1996. Simultaneous detection of positive and negative ions from single airborne particles by real-time laser mass spectrometry. Aerosol Sci. Technol. 24 (4):233–42. doi:https://doi.org/10.1080/02786829608965368.
- Hinz, K. P., and B. Spengler. 2007. Instrumentation, data evaluation and quantification in on-line aerosol mass spectrometry. J Mass Spectrom. 42 (7):843–60. doi:https://doi.org/10.1002/jms.1262.
- Hinz, K. P., A. Trimborn, E. Weingartner, S. Henning, U. Baltensperger, and B. Spengler. 2005. Aerosol single particle composition at the jungfraujoch. J. Aerosol Sci. 36 (1):123–45. doi:https://doi.org/10.1016/j.jaerosci.2004.08.001.
- Isaksen, I. S. A., C. Granier, G. Myhre, T. K. Berntsen, S. B. Dalsoren, M. Gauss, Z. Klimont, R. Benestad, P. Bousquet, W. Collins, et al. 2009. Atmospheric composition change: Climate-chemistry interactions. Atmos. Environ. 43 (33):5138–92. doi:https://doi.org/10.1016/j.atmosenv.2009.08.003.
- Kahnt, A., Y. Iinuma, F. Blockhuys, A. Mutzel, R. Vermeylen, T. E. Kleindienst, M. Jaoui, J. H. Offenberg, M. Lewandowski, O. Boge, et al. 2014. 2-hydroxyterpenylic acid: An oxygenated marker compound for α-pinene secondary organic aerosol in ambient fine aerosol . Environ. Sci. Technol. 48 (9):4901–8. doi:https://doi.org/10.1021/es500377d.
- Kamphus, M., M. Ettner-Mahl, T. Klimach, F. Drewnick, L. Keller, D. J. Cziczo, S. Mertes, S. Borrmann, and J. Curtius. 2010. Chemical composition of ambient aerosol, ice residues and cloud droplet residues in mixed-phase clouds: Single particle analysis during the cloud and aerosol characterization experiment (clace 6). Atmos. Chem. Phys. 10 (16):8077–95. doi:https://doi.org/10.5194/acp-10-8077-2010.
- Kandler, K.,. L. Schutz, C. Deutscher, M. Ebert, H. Hofmann, S. Jackel, R. Jaenicke, P. Knippertz, K. Lieke, A. Massling, et al. 2009. Size distribution, mass concentration, chemical and mineralogical composition and derived optical parameters of the boundary layer aerosol at tinfou, morocco, during samum 2006. Tellus Series B-Chemical and Physical Meteorology 61 (1):32–50. doi:https://doi.org/10.1111/j.1600-0889.2008.00385.x.
- Koch, D., S. Menon, A. Del Genio, R. Ruedy, I. Alienov, and G. A. Schmidt. 2009. Distinguishing aerosol impacts on climate over the past century. Journal of Climate 22 (10):2659–77. doi:https://doi.org/10.1175/2008JCLI2573.1.
- Laskin, A., M. J. Iedema, A. Ichkovich, E. R. Graber, I. Taraniuk, and Y. Rudich. 2005. Direct observation of completely processed calcium carbonate dust particles. Faraday Discuss. 130:453–68. doi:https://doi.org/10.1039/b417366j.
- Liou, K. N., Y. Takano, C. He, P. Yang, L. R. Leung, Y. Gu, and W. L. Lee. 2014. Stochastic parameterization for light absorption by internally mixed bc/dust in snow grains for application to climate models. J. Geophys. Res. Atmos. 119 (12):7616–32. doi:https://doi.org/10.1002/2014JD021665.
- Matschullat, J., W. Maenhaut, F. Zimmermann, and J. Fiebig. 2000. Aerosol and bulk deposition trends in the 1990's, eastern erzgebirge, central europe. Atmospheric Environment 34:3213–21. doi:https://doi.org/10.1016/S1352-2310(99)00516-6.
- Ming, J., H. Cachier, C. Xiao, D. Qin, S. Kang, S. Hou, and J. Xu. 2008. Black carbon record based on a shallow himalayan ice core and its climatic implications. Atmos. Chem. Phys. 8 (5):1343–52. doi:https://doi.org/10.5194/acp-8-1343-2008.
- Murphy, D. M., D. J. Cziczo, K. D. Froyd, P. K. Hudson, B. M. Matthew, A. M. Middlebrook, R. E. Peltier, A. Sullivan, D. S. Thomson, and R. J. Weber. 2006. Single-particle mass spectrometry of tropospheric aerosol particles. Journal of Geophysical Research-Atmospheres 111 (D23):1–15. doi:https://doi.org/10.1029/2006jd007340.
- Nabat, P., S. Somot, M. Mallet, F. Sevault, M. Chiacchio, and M. Wild. 2015. Direct and semi-direct aerosol radiative effect on the mediterranean climate variability using a coupled regional climate system model. Clim. Dyn. 44 (3–4):1127–55. doi:https://doi.org/10.1007/s00382-014-2205-6.
- Qin, X. Y., K. A. Pratt, L. G. Shields, S. M. Toner, and K. A. Prather. 2012. Seasonal comparisons of single-particle chemical mixing state in riverside, ca. Atmos. Environ. 59:587–96. doi:https://doi.org/10.1016/j.atmosenv.2012.05.032.
- Ramanathan, V., and G. Carmichael. 2008. Global and regional climate changes due to black carbon. Nature Geosci. 1 (4):221–7. doi:https://doi.org/10.1038/ngeo156.
- Reilly, P. T. A., A. C. Lazar, R. A. Gieray, W. B. Whitten, and J. M. Ramsey. 2000. The elucidation of charge-transfer-induced matrix effects in environmental aerosols via real-time aerosol mass spectral analysis of individual airborne particles. Aerosol Sci. Technol. 33 (1–2):135–52. doi:https://doi.org/10.1080/027868200410895.
- Rolph, G., A. Stein, and B. Stunder. 2017. Real-time environmental applications and display system: Ready. Environmental Modelling & Software 95:210–28. doi:https://doi.org/10.1016/j.envsoft.2017.06.025.
- Russell, A. G., G. R. Cass, and J. H. Seinfeld. 1986. On some aspects of nighttime atmospheric chemistry. Environ. Sci. Technol. 20 (11):1167–72. doi:https://doi.org/10.1021/es00153a013.
- Schmidt, S.,. J. Schneider, T. Klimach, S. Mertes, L. P. Schenk, P. Kupiszewski, J. Curtius, and S. Borrmann. 2017. Online single particle analysis of ice particle residuals from mountain-top mixed-phase clouds using laboratory derived particle type assignment. Atmos. Chem. Phys. 17 (1):575–94. doi:https://doi.org/10.5194/acp-17-575-2017.
- Shen, X., H. Saathoff, W. Huang, C. Mohr, R. Ramisetty, and T. Leisner. 2019. Understanding atmospheric aerosol particles with improved particle identification and quantification by single-particle mass spectrometry. Atmos. Meas. Tech. 12 (4):2219–40. doi:https://doi.org/10.5194/amt-12-2219-2019.
- Shindell, D. T., G. Faluvegi, D. M. Koch, G. A. Schmidt, N. Unger, and S. E. Bauer. 2009. Improved attribution of climate forcing to emissions. Science 326 (5953):716–8. doi:https://doi.org/10.1126/science.1174760.
- Sierau, B., R. Y. W. Chang, C. Leck, J. Paatero, and U. Lohmann. 2014. Single-particle characterization of the high-arctic summertime aerosol. Atmos. Chem. Phys. 14 (14):7409–30. doi:https://doi.org/10.5194/acp-14-7409-2014.
- Silva, P. J., and K. A. Prather. 2000. Interpretation of mass spectra from organic compounds in aerosol time-of-flight mass spectrometry. Anal. Chem. 72 (15):3553–62. doi:https://doi.org/10.1021/ac9910132.
- Sokolik, I. N., and O. B. Toon. 1999. Incorporation of mineralogical composition into models of the radiative properties of mineral aerosol from uv to ir wavelengths. J. Geophys. Res. 104 (D8):9423–44. doi:https://doi.org/10.1029/1998JD200048.
- Song, C. H., and G. R. Carmichael. 1999. The aging process of naturally emitted aerosol (sea-salt and mineral aerosol) during long range transport. Atmos. Environ. 33 (14):2203–18. doi:https://doi.org/10.1016/S1352-2310(98)00301-X.
- Stein, A. F., R. R. Draxler, G. D. Rolph, B. J. B. Stunder, M. D. Cohen, and F. Ngan. 2015. Noaa's hysplit atmospheric transport and dispersion modeling system. Bulletin of the American Meteorological Society 96 (12):2059–77. doi:https://doi.org/10.1175/BAMS-D-14-00110.1.
- Tegen, I., A. A. Lacis, and I. Fung. 1996. The influence on climate forcing of mineral aerosols from disturbed soils. Nature 380 (6573):419–22. doi:https://doi.org/10.1038/380419a0.
- Tobo, Y., D. Z. Zhang, A. Matsuki, and Y. Iwasaka. 2010. Asian dust particles converted into aqueous droplets under remote marine atmospheric conditions. Pnas. 107 (42):17905–10. doi:https://doi.org/10.1073/pnas.1008235107.
- Trimborn, A., K. P. Hinz, and B. Spengler. 2000. Online analysis of atmospheric particles with a transportable laser mass spectrometer. Aerosol Sci. Technol. 33 (1–2):191–201. doi:https://doi.org/10.1080/027868200410921.
- Twomey, S. 1977. The influence of pollution on the shortwave albedo of clouds. J. Atmos. Sci. 34 (7):1149–52. doi:https://doi.org/10.1175/1520-0469(1977)034 < 1149:TIOPOT.
- Tyanova, S., T. Temu, P. Sinitcyn, A. Carlson, M. Y. Hein, T. Geiger, M. Mann, and J. Cox. 2016. The perseus computational platform for comprehensive analysis of (prote)omics data. Nat. Methods. 13 (9):731–40. doi:https://doi.org/10.1038/nmeth.3901.
- Ulbrich, I. M., M. R. Canagaratna, Q. Zhang, D. R. Worsnop, and J. L. Jimenez. 2009. Interpretation of organic components from positive matrix factorization of aerosol mass spectrometric data. Atmos. Chem. Phys. 9 (9):2891–918. doi:https://doi.org/10.5194/acp-9-2891-2009.
- Ward, J. H. 1963. Hierarchical grouping to optimize an objective function. J. Am. Stat. Assoc. 58 (301):236–44. doi:https://doi.org/10.2307/2282967.
- Yasunari, T. J., R. D. Koster, K.-M. Lau, T. Aoki, Y. C. Sud, T. Yamazaki, H. Motoyoshi, and Y. Kodama. 2011. Influence of dust and black carbon on the snow albedo in the nasa goddard earth observing system version 5 land surface model. J. Geophys. Res 116 (D2):1–15. doi:https://doi.org/10.1029/2010JD014861.
- Zauscher, M. D., Y. Wang, M. J. K. Moore, C. J. Gaston, and K. A. Prather. 2013. Air quality impact and physicochemical aging of biomass burning aerosols during the 2007 san diego wildfires. Environ. Sci. Technol. 47 (14):7633–43. doi:https://doi.org/10.1021/es4004137.
- Zheng, M., L. G. Salmon, J. J. Schauer, L. M. Zeng, C. S. Kiang, Y. H. Zhang, and G. R. Cass. 2005. Seasonal trends in pm2.5 source contributions in beijing, china. Atmos. Environ. 39 (22):3967–76. doi:https://doi.org/10.1016/j.atmosenv.2005.03.036.