Peripheral early-stage non-small cell lung cancer in medically inoperable patients is a guideline-recommended indication for stereotactic body radiotherapy (SBRT) [Citation1]. However, peripheral lesions present varying tumor geometries and overlap with organs at risk (OARs) such as the thoracic wall (TW); treatment planning is prone to variation, leading to inconsistencies in treatment plan (TP) quality [Citation2,Citation3]; and implementing a lung SBRT program is resource intensive [Citation4]. Automated solutions have been devised to help address these problems, including knowledge-based planning (KBP) [Citation5–10].
One commercial KBP solution utilizes a model based on previous TPs to generate dose-volume histogram (DVH) prediction ranges which position optimization objectives for the OARs of prospective patients. Pre-clinical evaluation has yielded clinically acceptable results for a number of disease sites [Citation11–13]. However, detailed investigations for lung SBRT are lacking. We therefore investigated the performance of this KBP solution for 3 and 5 fraction lung SBRT using volumetric modulated arc therapy (VMAT); whether TPs from these fractionation schemes could be combined into a single model; and how the models performed when the planning target volume (PTV) overlapped with OARs including the TW.
Material and methods
Lung SBRT is treated using flattening filter-free beams and VMAT [Citation14]. Risk-adapted fractionation schemes are applied, for example 3 × 18 Gy for peripheral tumors <3 cm in diameter, without broad TW contact and 5 × 11 Gy for tumors >3 cm or with broad TW contact. Both schemes allow inhomogeneous PTV doses up to 140% of the prescribed dose. When there is PTV-TW overlap, two target structures, PTVinOAR/PTVoutOAR, are created for the part of the PTV with/without TW overlap. Maximum dose (Dmax) is restricted to ≤140% of the prescribed dose in PTVoutOAR and ≤110% in PTVinOAR. A 1–2 cm wide TW structure is delineated to control TW dose outside the PTV. Optimization objectives limit the volume receiving 30–60 Gy (V30-60), which may be predictive for TW toxicity [Citation15]. A 5-mm wide ring structure 5 mm from the PTV, and a normal tissue objective (NTO), are used for dose fall off. To limit irradiation of the contralateral lung, a dose objective on the V5 [Citation16] as well as a partial arc/avoidance sector are used. A maximum dose objective is used for the spinal cord, and a 3-mm planning-at-risk volume (Table S1). Finally, all TPs undergo a continue previous optimization (CPO) [Citation17,Citation18], as described in Appendix 1 of the supplementary materials.
RapidPlanTM is a commercial KBP solution constituting a model derived from a library of TPs. Models use regression analysis to determine correlations between dosimetric and geometric features, with the coefficient of determination (R2) indicative of the quality and variance of regression models [Citation13]. The model generates DVH prediction ranges for OARs of prospective patients. Optimization objectives are then placed along the lower boundary of these prediction ranges to guide the VMAT optimization [Citation12].
Clinical plans (CPs) of 100 patients were used to populate three models: Model5 × 11/Model3 × 18 comprised CPs of 50 patients treated with 5 × 11 Gy/3 × 18 Gy; ModelCOMBI comprised all 100 plans, and is therefore more heterogeneous in terms of dosimetry, fractionation, arc configuration, and geometry (Table S2).
An evaluation group (EG) comprised 20 further CPs (10 each of 5 × 11 Gy/3 × 18 Gy). CPs were optimized using PRO v10.0.28 (PRO10) and dose was calculated using AcurosXB v11.0.31. Two KBPs were created for each evaluation patient using the Photon Optimizer v13.6.15 (PO13). The first used Model3 × 18 or Model5 × 11 depending on the CP fractionation; the second used ModelCOMBI. Both used the CP arc setup. To limit user interaction, KBPs utilized DVH predictions for all of the OAR objectives, except for the dose-volume objective limiting the contralateral lung V5 (Table S3), and an automatic NTO. Dose was calculated using AcurosXB v13.6.15 with a 2.5 mm calculation grid. Both CPs and KBPs were normalized such that 95% of the PTV received the prescribed dose. To account for differences between optimizers, all CPs in the EG were re-optimized using PO13 (CPPO), using the same arc configuration, end-clinical objectives, and priorities.
Model quality was evaluated using statistical metrics/regression plots reported by RapidPlan and Model Analytics (https://modelanalytics.varian.com), facilitating outlier identification [Citation19]. Furthermore, KBPs generated by Model3 × 18/5 × 11 and ModelCOMBI were compared with their respective CPs using OAR and PTV dose metrics. Where appropriate two-sided Student’s t-tests, or for skewed data the Kruskal-Wallis test, were performed with p < 0.05 considered significant.
Results
Model3 × 18, Model5 × 11 and ModelCOMBI had 34, 19 and 73 structures identified as outliers, respectively, by at least one model metric. Of these, 2/34, 0/19 and 4/73 were visually confirmed and removed [Citation19]. Two PTVinOAR structures with the smallest volumes (0.2 cm3) were removed as their respective DVHs were insufficiently sampled during the extraction phase of model creation [Citation13] leading to inaccurate DVH representation in the model configuration window. Regression analysis of models showed good correlation between geometric and dosimetric features; average R2 was 0.75, 0.80 and 0.76 for Model3 × 18, Model5 × 11 and ModelCOMBI, respectively (Figure S1). R2 for the TW and contralateral lung in Model3 × 18/Model5 × 11/ModelCOMBI was 0.88/0.88/0.91 and 0.74/0.81/0.73, respectively. Figure S1 shows the majority of 5 × 11 Gy and 3 × 18 Gy patients are located in different regions of the regression plot consistent with differences in their TW dosimetry/geometry. The ring structure had the lowest R2: 0.59/0.59/0.60 in Model3 × 18/Model5 × 11/ModelCOMBI.
and compare individual results for all 20 patients from CP, CPPO, Model3 × 18/5 × 11 and ModelCOMBI, while and summarize averaged results. Re-optimization of CPs using PO13 led to similar plan quality, however differences existed in certain patients, mainly for the PTVinOAR, TW V50 and ring. Using ModelCOMBI produced, on average, similar plan quality to the respective individual Model3 × 18/5 × 11.
Figure 1. Dosimetric results per patient. Patients 1–10 are 5 × 11 Gy cases while 11–20 are 3 × 18 Gy.
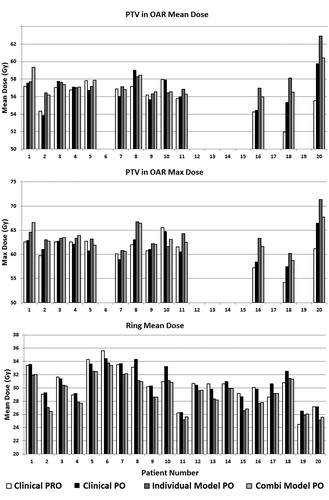
Table 1. Averaged RapidPlan results for 5 × 11 Gy patients of EG using Model5 × 11 and ModelCOMBI compared with respective clinical plans and clinical plans re-optimized using P013 [Clinical(PO)].
Table 2. Averaged RapidPlan results for 3 × 18 Gy patients of EG using Model3 × 18 and ModelCOMBI compared with respective clinical plans and clinical plans re-optimized using P013 [Clinical(PO)].
KBPs of nine patients exhibited increases in both Dmean and Dmax to the PTVinOAR over CPPO (). This was associated with a decrease in ring Dmean. For some of these patients, the differences were more pronounced when compared to the CP. In 8/9 cases the KBP PTVinOAR Dmean was ≤110% of the prescribed dose and following review by a physician and physicist, these plans were considered clinically acceptable. For the remaining patient (20), CPPO showed a relatively large increase in PTVinOAR Dmean/max when compared to CP, (PRO10). The dose increased further when using Model3 × 18, while ModelCOMBI performed similarly to CPPO. The KBP ring Dmean for this patient was the lowest among evaluation cases.
Discussion
We evaluated a commercial KBP solution for risk-adapted peripheral lung SBRT VMAT treatment planning. On average, two dose-fractionation-specific models generated plans of comparable quality to their respective clinical plan. Additionally, a larger, combined model, could be successfully created using plans with different fractionation and arc setup (partial arcs or avoidance sectors), but with similar total dose and planning goals. In some cases, the combined model led to dosimetric improvements compared to the fraction-specific model, likely because of increased geometric heterogeneity. In total, 39/40 KBPs (98%) would have been considered clinically acceptable (one plan, for Patient 20, would have been rejected due to a high PTVinOAR Dmean/max with Model3 × 18).
Regression plots (Figure S1) and R2 values suggest models generally provided good correlation between dosimetric and geometric features. However, the ring was modeled less well with the lowest R2 amongst the OARs, and ring Dmean decreased for all patients when using KBPs over CPPO. Reasons for this are still uncertain. We currently use a ring structure and NTO with standard settings. NTO modifications, for example with model-predicted settings based on PTV geometry, might remove the necessity for a ring structure.
PTVinOAR dose increased in a number of cases, particularly for those with a small volume (PTVinOAR for Patients 2, 16, 18 and 20 was <0.7 cm3). Several contributing factors were identified. First, the model does not predict PTVinOAR doses, as it is not an OAR. Furthermore, when the ring structure extends into the TW and the ring prediction is too low compared to the TW (Patient 20), TW sparing is compromised resulting in higher PTVinOAR doses. Second, model composition may have been an issue with only nine patients with chest wall overlapping/touching the PTV in Model3 × 18. Finally, some cases exhibited large differences between CP and CPPO plans. The CPO is needed to correct dose in low density regions resulting from the initial optimization, which attributes more dose to higher density regions (e.g. PTVinOAR). However, we found that the CPO in PO13 stopped prematurely and that PO13 seemed to inherently minimize MUs to a greater extent than PRO10 (Appendix 2 of the supplementary materials). This has been communicated to the manufacturer. Early evaluation of a preliminary solution provided by the manufacturer appeared to resolve this issue although this is not yet clinically available.
In conclusion, KBPs were, on average, of comparable quality to their respective CPs for 3 and 5 fraction peripheral lung SBRT treatments, however, differences were noted for individual patients. All plans therefore needed to be critically reviewed. Plan quality generated by ModelCOMBI showed that TPs with differing fractionation schemes, but similar total prescription doses and planning strategies, could be used to populate a combined model. Deviations from CPs seemed to be largely attributable to the inherent mechanics of the PO13 optimizer, which are different to PRO10. This requires further investigation by the manufacturer. Finally, caution should be exercised when dealing with structures poorly represented in a knowledge-based model.
IONC_1273544_Supplementary_Materials.docx
Download MS Word (646.1 KB)Disclosure statement
The department has a research collaboration with Varian Medical Systems, and Dahele, Slotman, and Verbakel have received honoraria/travel support from Varian Medical Systems.
Additional information
Funding
References
- Lagerwaard FJ, Haasbeek CJA, Smit EF, et al. Outcomes of risk-adapted fractionated stereotactic radiotherapy for stage I non-small-cell lung cancer. Int J Radiat Oncol Biol Phys. 2008;70:685–692.
- Nelms BE, Robinson G, Markham J, et al. Variation in external beam treatment plan quality: an inter-institutional study of planners and planning systems. Pract Radiat Oncol. 2012;2:296–305.
- Das IJ, Cheng CW, Chopra KL, et al. Intensity-modulated radiation therapy dose prescription, recording, and delivery: patterns of variability among institutions and treatment planning systems. J Natl Cancer Inst. 2008;100:300–307.
- Khader J, Al-Mousa A, Hijla FA, et al. Requirements and implementation of a lung sbrt program in a developing country: benefits of international cooperation. Int J Radiat Oncol Biol Phys. 2016;95:1236–1238.
- Wu B, Pang D, Simari P, et al. Using overlap volume histogram and IMRT plan data to guide and automate VMAT planning: a head-and-neck case study. Med Phys. 2013;40:021714.
- Lian J, Yuan L, Ge Y, et al. Modeling the dosimetry of organ-at-risk in head and neck IMRT planning: an intertechnique and interinstitutional study. Med Phys. 2013;40:121704.
- Zhu X, Ge Y, Li T, et al. A planning quality evaluation tool for prostate adaptive IMRT based on machine learning. Med Phys. 2011;38:719.
- Moore KL, Brame RS, Low DA, et al. Experience-based quality control of clinical intensity-modulated radiotherapy planning. Int J Radiat Oncol Biol Phys. 2011;81:545–551.
- Zarepisheh M, Long T, Li N, et al. A DVH-guided IMRT optimization algorithm for automatic treatment planning and adaptive radiotherapy replanning. Med Phys. 2014;41:061711.
- Good D, Lo J, Lee WR, et al. A knowledge-based approach to improving and homogenizing intensity modulated radiation therapy planning quality among treatment centers: an example application to prostate cancer planning. Int J Radiat Oncol Biol Phys. 2013;87:176–181.
- Fogliata A, Nicolini G, Clivio A, et al. A broad scope knowledge based model for optimization of VMAT in esophageal cancer: validation and assessment of plan quality among different treatment centers. Radiat Oncol. 2015;10:220.
- Tol JP, Delaney AR, Dahele M, et al. Evaluation of a knowledge-based planning solution for head and neck cancer. Int J Radiat Oncol Biol Phys. 2015;91:612–620.
- Fogliata A, Belosi F, Clivio A, et al. On the pre-clinical validation of a commercial model-based optimisation engine: application to volumetric modulated arc therapy for patients with lung or prostate cancer. Radiother Oncol. 2014;113:385–391.
- Ong CL, Verbakel WFAR, Dahele M, et al. Fast arc delivery for stereotactic body radiotherapy of vertebral and lung tumors. Int J Radiat Oncol Biol Phys. 2012;83:e137–e143.
- Stephans KL, Djemil T, Tendulkar RD, et al. Prediction of chest wall toxicity from lung stereotactic body radiotherapy (SBRT). Int J Radiat Oncol Biol Phys. 2012;82:974–980.
- Ong CL, Palma D, Verbakel WFAR, et al. Treatment of large stage I–II lung tumors using stereotactic body radiotherapy (SBRT): Planning considerations and early toxicity. Radiother Oncol. 2010;97:431–436.
- Tol JP, Dahele M, Doornaert P, et al. Toward optimal organ at risk sparing in complex volumetric modulated arc therapy: an exponential trade-off with target volume dose homogeneity. Med Phys. 2014;41:021722.
- Ong CL, Verbakel WFAR, Cuijpers JP, et al. Stereotactic radiotherapy for peripheral lung tumors: a comparison of volumetric modulated arc therapy with 3 other delivery techniques. Radiother Oncol. 2010;97:437–442.
- Delaney AR, Tol JP, Dahele M, et al. Effect of dosimetric outliers on the performance of a commercial knowledge-based planning solution. Int J Radiat Oncol Biol Phys. 2016;94:469–477.