Abstract
Context: Reviews of the literature on symptoms in oncology patients undergoing curative treatment, as well as patients receiving palliative care, suggest that they experience multiple, co-occurring symptoms and side effects.
Objectives: The purposes of this study were to determine if subgroups of oncology patients could be identified based on symptom occurrence rates and if these subgroups differed on a number of demographic and clinical characteristics, as well as on quality of life (QoL) outcomes.
Methods: Latent class analysis (LCA) was used to identify subgroups (i.e. latent classes) of patients with distinct symptom experiences based on the occurrence rates for the 13 most common symptoms from the Memorial Symptom Assessment Scale.
Results: In total, 534 patients with breast, head and neck, colorectal, or ovarian cancer participated. Four latent classes of patients were identified based on probability of symptom occurrence: all low class [i.e. low probability for all symptoms (n = 152)], all high class (n = 149), high psychological class (n = 121), and low psychological class (n = 112). Patients in the all high class were significantly younger compared with patients in the all low class. Furthermore, compared to the other three classes, patients in the all high class had lower functional status and higher comorbidity scores, and reported poorer QoL scores. Patients in the high and low psychological classes had a moderate probability of reporting physical symptoms. Patients in the low psychological class reported a higher number of symptoms, a lower functional status, and poorer physical and total QoL scores.
Conclusion: Distinct subgroups of oncology patients can be identified based on symptom occurrence rates. Patient characteristics that are associated with these subgroups can be used to identify patients who are at greater risk for multiple co-occurring symptoms and diminished QoL, so that these patients can be offered appropriate symptom management interventions.
Reviews of the literature on symptoms in patients undergoing active treatment for cancer [Citation1], as well as in patients with advanced disease receiving palliative care [Citation2], suggest that patients experience multiple, co-occurring symptoms and side effects. Findings from these reviews suggest that, while the relationships between specific symptoms and patient-reported outcomes are complex, the occurrence of multiple symptoms is associated with decreased functional status and quality of life (QoL) [Citation1,Citation2]. However, less is known about specific demographic and clinical characteristics that distinguish among patients with a higher versus a lower symptom burden.
An emerging area in symptom management research is the identification of subgroups of oncology patients who are at greater risk for more severe symptoms from the disease itself or its treatment. To date, 13 studies were found that used a variety of analytic approaches to classify oncology patients based on their symptom experience [Citation3–15]. Across these studies, two [Citation3,Citation4,Citation9] to five [Citation13] groups of patients were identified. In all 13 studies, subgroups of patients who reported a low and high number of symptoms were found. In the studies that reported three [Citation7,Citation8,Citation10,Citation12,Citation14,Citation15], four [Citation5,Citation6,Citation11], or five [Citation13] subgroups, the distinguishing characteristics of the subgroups of patients with moderate symptoms were highly variable in terms of the number and types of symptoms, as well as in the specific demographic and clinical characteristics that distinguished among these subgroups.
In the earliest studies [Citation3–6], cluster analysis was used to create the patient groups, while in subsequent studies [Citation7–15], latent class analysis (LCA) was used to identify subgroups of patients with distinct symptom profiles. However, the number of symptoms used in these LCAs varied from four [Citation7,Citation12–14] to 25 [Citation10]. All except one [Citation8] of the 13 studies evaluated for differences in demographic and clinical characteristics among the latent classes. While some studies found no differences in demographic characteristics [Citation3,Citation4,Citation6,Citation12], others found that allocation to the ‘high’ symptom class was associated with: being female [Citation7,Citation10,Citation11,Citation13,Citation15], being younger [Citation5,Citation7,Citation10,Citation11,Citation14,Citation15], having less education [Citation10,Citation14], being unmarried [Citation5, Citation10], and living alone [Citation14]. In terms of clinical characteristics, while some studies found no differences in clinical characteristics [Citation4,Citation6,Citation13], others found that patients in the ‘high’ class had a higher number of comorbid conditions [Citation7,Citation12,Citation14,Citation15], more extensive disease [Citation3,Citation9,Citation11,Citation14], and poorer functional status [Citation3,Citation5–7,Citation10,Citation12–15]. The identification of subgroups of oncology patients and their associated demographic and clinical characteristics will allow clinicians to identify more vulnerable patients who are at higher risk for poorer outcomes.
Nine [Citation3–6,Citation10–13,Citation15] of the 13 previous studies evaluated for differences in QoL outcomes. In every study, patients in the ‘high’ symptom class had poorer QoL outcomes. While progress is being made in the identification of subgroups of oncology patients with a higher symptom burden, the relatively small sample sizes in several studies [Citation3,Citation4,Citation9], and the use of single symptom inventories [Citation5–7,Citation12–14] versus multiple symptom inventories [Citation3,Citation4,Citation8–11,Citation15], make it difficult to draw definitive conclusions about the relationships among multiple symptoms, associated characteristics, and patient-reported outcomes. Therefore, the purposes of this study were to determine if subgroups (i.e. latent classes) of oncology patients could be identified based on their distinct experiences with multiple co-occurring symptoms and if these classes differed on a number of demographic and clinical characteristics, as well as on QoL outcomes.
Methods
Patients and settings
This longitudinal study evaluated symptoms and QoL outcomes in oncology patients. Patients (n = 534) were included if they: were adults (≥18 years of age); were able to read, write and understand Norwegian; and were scheduled to receive radiotherapy (RT) for breast or head and neck cancer or chemotherapy (CTX) for colorectal or ovarian cancer. Patients were excluded if they were scheduled to receive RT for brain metastases or had a disease that affected their cognitive ability. Patients were recruited prior to initiation of treatment at Oslo University Hospital (OUH). The study was approved by the Regional Committee for Medical and Health Research Ethics, the Norwegian Directorate of Health, the privacy ombudsman at the hospital, and the institutional review board. Patient recruitment procedures are summarized in .
Study procedures
At the time of their first outpatient appointment with their physician (i.e. approximately one week prior to RT or CTX), patients were introduced to a member of the research team and provided with information about the study. After obtaining written informed consent, patients completed a number of self-report questionnaires at enrollment and again at approximately one, two, three, and six months after the initiation of treatment. In addition, colorectal and ovarian cancer patients completed three additional questionnaires to evaluate symptoms related to CTX. Only data from the enrollment assessment (prior to initiation of treatment) were used in the analyses.
Instruments
Demographic and clinical characteristics
Patients provided information on gender, marital status, living situation, and level of education. Study nurses obtained information on age, the presence of metastases, number of metastatic sites, intention of the treatment (i.e. curative or palliative), and number and types of previous treatments from the patients’ medical record.
Functional status
Patients rated their functional status using the Karnofsky Performance Status (KPS) scale [Citation16], which can range from 0 (death) to 100 (normal; no complaints; no evidence of disease). The KPS scale has well established validity and reliability [Citation17]. As patients in this study were outpatients, the 40 (disabled, need special help and care) to 100 point range of the KPS scale was used.
Comorbidities
The Self-Administered Comorbidity Questionnaire-19 (SCQ-19) [Citation18] evaluated the number of, treatments for, and functional impact of 16 common and three optional medical conditions. Using the SCQ-19, patients were asked to indicate whether they had the comorbid condition, if they received treatment for it, and if it limited their activities. The total SCQ-19 score can range from 0 to 57. A higher total score indicates a more severe comorbidity profile. The SCQ-19 has well established validity and reliability in patients with chronic medical conditions [Citation18]. In this study, both the total number of comorbidities (0–19) and SCQ total score were used in the statistical analyses.
Multiple symptoms
The Memorial Symptom Assessment Scale (MSAS) [Citation19] contains a list of 32 physical and psychological symptoms that occur as a result of cancer or its treatment. Using the MSAS, patients were asked to indicate whether they had a specific symptom during the past week (i.e. occurrence). If they experienced a symptom, they were asked to rate its frequency, severity, and distress on 4–5-point Likert scales. If patients rated the frequency, severity, or distress of the symptoms as >0 despite reporting ‘no’ on symptom occurrence, occurrence was coded to indicate that the patient had the symptom. All missing items were interpreted and coded to indicate that the patient did not have the symptom. The occurrence rates for the 32 symptoms were summed and used to quantify the total number of symptoms each patient experienced. The reliability and validity of the MSAS are well established [Citation19]. The MSAS was translated into Norwegian according to established guidelines [Citation20].
Quality of life
In order to assess both disease-specific and generic aspects of QoL, the Multidimensional Quality of Life Scale-Cancer (MQOLS-CA) [Citation21] and the Medical Outcomes Study Short Form-12 (SF-12) [Citation22] were used. The MQOLS-CA consists of 33 items that evaluate five dimensions of QoL (i.e. psychological wellbeing, physical wellbeing, nutrition, symptom distress, interpersonal wellbeing) [Citation21]. Each item is rated on a 0–10 numeric rating scale (NRS). A total QoL score is calculated as the mean of the 33 items. Higher scores indicate a better QoL. Only the total QoL score was used in the analyses. The MQOLS-CA has well established construct validity and test-retest reliability [Citation21,Citation23].
The SF-12 (version 1) consists of 12 questions about physical and mental health as well as overall health status [Citation22]. The questionnaire is scored into two components, namely a physical component summary (PCS) and a mental component summary (MCS). The PCS and MCS scores are calculated using norm-based methods from the 1998 general US population, with a mean of 50 (SD 10) and interpreted as being greater than or less than the mean scores in the general US population [Citation24]. Scores for the general Norwegian population [i.e. mean PCS, 50.3 (SD 8.8); mean MCS, 50.6 (SD 9.9)] are similar to the US scores [Citation25]. The SF-12 has well established validity and reliability [Citation22,Citation25].
Data analysis
Descriptive statistics and frequency distributions were generated on the sample characteristics and symptom data at enrollment using SPSS version 23 (SPSS, Armonk, NY, USA: IBM Corp). LCA was performed using Mplus version 7 (Los Angeles, CA, USA: Muthén & Muthén) [Citation26]. LCA is a method for identifying unmeasured class membership among participants using observed variables, and was used to identify subgroups of patients (i.e. latent classes) with distinct symptom experiences [Citation27,Citation28]. For this analysis, the LCA was performed using patients’ ratings of symptom occurrence [Citation4,Citation10,Citation11].
To have a sufficient number of patients with each symptom to perform the LCA, the 13 MSAS symptoms that occurred in ≥30% of the patients were used to identify the latent classes. The final number of latent classes was identified by evaluating the Bayesian Information Criterion (BIC) and entropy. The model that fits the data best had the lowest BIC [Citation29]. In addition, well fitting models produce entropy values of ≥0.80 [Citation30]. Finally, well fitting models ‘make sense’ conceptually and the estimated classes differ on variables not used in the generation of the model (e.g. demographic and clinical characteristics) [Citation29]. Estimation was performed with robust maximum likelihood and the expectation-maximization algorithms [Citation27].
After the latent classes were identified, differences in demographic and clinical characteristics, occurrence rates for each comorbidity, as well as QoL outcomes among the latent classes were evaluated using analyses of variance, Kruskal-Wallis tests, and χ2-tests of independence using SPSS. Pairwise post hoc contrasts were done using the Bonferroni procedure. In addition, differences in occurrence rates of each symptom among the latent classes were tested using logistic regression, followed by Bonferroni post hoc contrasts in Stata (Stata, College Station, TX, USA: StataCorp LP). A p value of <0.05 was considered statistically significant.
Results
Overall sample
As illustrated in , 534 patients completed the enrollment questionnaire (response rate 87% among those who signed the consent form). The sample comprised 188 patients with breast cancer, 133 with head and neck cancer, 120 with colorectal cancer, and 93 with ovarian cancer. The total sample comprised 69% women, and their mean age was 60 (SD 10.4; range 23–88) years. The majority (73%) was treated with curative intent.
Results of the LCA
Thirteen symptoms (i.e. lack of energy, feeling drowsy, pain, difficulty sleeping, dry mouth, problems with sexual interest/activity, feeling bloated, sweats, worrying, feeling sad, difficulty concentrating, feeling nervous, feeling irritable) occurred in ≥30% of the patients and were included in the LCA. Using LCA, four classes of patients were identified based on the probability of occurrence of these symptoms (). Fit indices for the models with two through five classes are shown in . The four-class solution was selected because its BIC was the lowest [the log-likelihood (LL) and Akaike Information Criterion (AIC) are provided for the interested reader].
Figure 2. Probability of symptom occurrence for each of the latent classes for the 13 symptoms on the Memorial Symptom Assessment Scale that occurred in ≥30% of the patients.
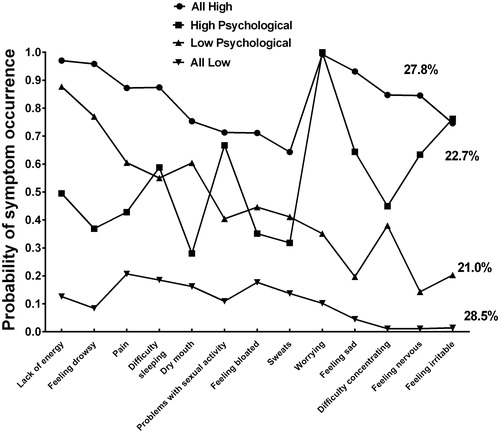
Table 1. Latent class solutions and fit indices for two- through five classes using occurrence rates for 13 symptoms on the Memorial Symptom Assessment Scale.
As shown in , the largest class (n = 152, 28.5%) reported a relatively low probability of occurrence for all 13 MSAS symptoms (i.e. all low), that ranged from 0.01 (difficulty concentrating and feeling nervous) to 0.21 (pain). The second largest class (n = 149, 27.8%) reported a relatively high probability of occurrence for all 13 symptoms (i.e. all high), that ranged from 0.64 (sweats) to 0.99 (worrying). The third largest class (n = 121, 22.7%) reported a low to moderate probability of occurrence for the physical symptoms, that ranged from 0.28 (dry mouth) to 0.50 (lack of energy), and a higher probability of occurrence for the psychological symptoms, that ranged from 0.24 (feeling irritable) to 1.00 (worrying) (i.e. high psychological). The smallest class of patients (n = 112, 21.0%) reported a moderate to high probability of occurrence for the physical symptoms, that ranged from 0.40 (problems with sexual interest) to 0.88 (lack of energy), and a lower probability of occurrence for the psychological symptoms, that ranged from 0.14 (feeling nervous) to 0.38 (difficulty concentrating) (i.e. low psychological). Differences in the actual occurrence rates for the 13 symptoms among the four classes are presented in .
Table 2. Differences in the occurrence rates for the 13 most common symptoms among the four latent classes (n = 534).
Differences in demographic and clinical characteristics among the latent classes
Differences in demographic and clinical characteristics among the four classes are summarized in and , respectively. Age was the only demographic characteristic that differed among the classes. Patients in the all high class were significantly younger than patients in the all low class ().
Table 3. Differences in demographic characteristics among the four latent classes (n = 534).
Table 4. Differences in clinical characteristics among the four latent classes (n = 534).
Compared to the all low class, patients in the low psychological and all high classes had a lower functional status, a higher number of comorbidities, and higher SCQ scores (). In addition, compared to the high psychological class, patients in the all high class had lower functional status, a higher number of comorbidities, and a higher SCQ score. Significant differences in the occurrence of several comorbidities (i.e. neck/back pain, headache, depression) were found among the classes (). Post hoc contrasts demonstrated the following pattern in terms of differences in total number of MSAS symptoms reported: all low < high psychological < low psychological < all high ().
Differences in QoL scores among the latent classes
summarizes the differences in QoL scores among the latent classes using a generic (i.e. SF-12) and a disease-specific (i.e. MQOLS-CA) measure. Compared to patients in the all low and the high psychological classes, patients in the all high and low psychological classes had lower PCS scores. For the MCS, post hoc contrasts demonstrated the expected pattern (i.e. all low > low psychological > high psychological > all high). Post hoc contrasts for differences in the MQOLS-CA total QoL scores followed a similar pattern as the PCS scores (i.e. all low > high psychological > low psychological > all high).
Table 5. Differences in quality of life scores among the four latent classes (n = 534).
Discussion
Identification of latent classes
Findings from this study add to the growing body of evidence that subgroups of oncology patients with distinct symptom experiences can be identified using statistical approaches like LCA. While direct comparisons across studies are difficult because of differences in the number of symptoms evaluated and patients’ disease and treatment characteristics, a consistent finding across all of the studies is the identification of three [Citation7,Citation8,Citation10,Citation12,Citation14,Citation15] to four [Citation5,Citation6,Citation11] classes of which one has a relatively high and the other a relatively low symptom burden. However, some studies [Citation5–7,Citation12,Citation14] used only four symptoms (i.e. pain, fatigue, sleep disturbance, depression) to create the patient subgroups.
In three previous studies, Miaskowski and colleagues [Citation10,Citation11,Citation15] used LCA to identify classes of oncology patients with distinct symptom experiences using MSAS symptom occurrence rates, comparable to our study. The percentages of patients in the all low and all high classes varied from 25% to 36% and from 14% to 31%, respectively. Differences across studies in the percentages of patients in the two extreme phenotype classes may be related to differences in patients’ demographic and clinical characteristics as well as in the number of symptoms used in the LCAs. In the current study, over 25% of the patients were in the all high class, and, on average, they reported 18 symptoms at the initiation of CTX or RT. This finding is consistent with previous reports where the mean number of symptoms was approximately 20 in the all high class [Citation10,Citation11,Citation15]. Given the number of studies that identified a group of oncology patients with an extremely high number of co-occurring symptoms, clinicians need to perform comprehensive symptom assessments on a regular basis to identify this particularly high-risk group.
Consistent with a previous report [Citation11], in the current study, two distinct classes were identified based on the probability of experiencing psychological symptoms, (i.e. high psychological, low psychological). These two groups had a low to moderate versus a moderate to high probability of reporting physical symptoms, respectively. Interestingly, within each class, the occurrence rates for the physical and psychological symptoms seem to vary in opposite directions. Patients in the low psychological class reported a higher mean number of symptoms () and a higher percentage of these patients reported lack of energy, feeling drowsy, pain, and dry mouth (). Furthermore, they reported poorer functional status () and poorer PCS and MQOLS-CA total QoL scores (), which may be related to their physical symptoms. In the previous LCA study that found low and high psychological classes [Citation11], the low psychological class had poorer functional status. However, no differences were found between the two groups in total number of symptoms or any of their QoL outcomes. Differences in study findings may be related to differences in the number of symptoms used in the LCAs (i.e. 13 vs. 25) and differences in patients’ demographic and clinical characteristics.
Characteristics associated with a higher symptom burden
In the current study, age was the only demographic characteristic that differed between the all high and all low classes. Consistent with previous studies [Citation5,Citation7,Citation10,Citation11,Citation14,Citation15], patients in the all high class were significantly younger than patients in the all low class. While a potential explanation for these age differences is that younger patients may receive more aggressive treatments, no differences were found between these two classes in disease or treatment characteristics (). Although some studies found that women reported a higher symptom burden [Citation7,Citation10,Citation11,Citation13,Citation15], our findings are consistent with studies that did not find any gender differences [Citation3,Citation4,Citation6,Citation14]. However, the majority (69%) of our sample was female because two of the diagnostic groups were patients with breast and ovarian cancer.
The only clinical characteristics that differed among the four classes were comorbidities and functional status. Consistent with previous studies [Citation7,Citation10,Citation12,Citation14,Citation15], compared to the all low class, patients in the all high class reported a higher number of comorbidities, as well as a higher comorbidity score. While the most common comorbidities across the entire sample were neck/back pain and osteoarthritis, compared to the all low and the high psychological classes, a higher percentage of patients in the all high class reported neck/back pain, headache, and depression (). These comorbidities may be associated with decrements in functional status independent of the patients’ cancer diagnosis.
In terms of differences in functional status between the extreme phenotype groups, our findings are consistent with previous reports [Citation3,Citation5–7,Citation9–15]. As previously mentioned, the low psychological class reported lower KPS scores than the high psychological class. These findings suggest that patients’ symptom experience has a significant impact on their functional status.
Of note, and consistent with several previous studies [Citation6,Citation10,Citation12,Citation13], no significant differences were found among the classes in terms of disease and treatment characteristics (). However, in some studies, stage I breast cancer versus stage II [Citation4], presence of distant metastases [Citation3,Citation11], more extensive disease [Citation9], receipt of CTX [Citation9], and higher stage of disease [Citation14] were associated with allocation to the higher symptom classes. These inconsistent findings may be related to variability in patients’ disease and treatment characteristics, instruments used to assess symptoms, and the timing of the symptom assessments.
Differences in QoL among the classes
Consistent with previous reports, compared to the all low class, patients in the all high class had worse scores on both the generic [Citation4,Citation10,Citation15] and disease-specific [Citation3,Citation6,Citation10,Citation11,Citation13,Citation15] QoL measures. In terms of the generic measure of QoL, the MCS scores among the four classes differed in the expected direction. The difference in MCS scores between the low psychological (48.5) and the high psychological (44.7) classes represent a small effect size according to the categorization of Cohen [Citation31] (Cohen’s d = 0.37) in the psychological dimension of QoL. Except for the all low class, patients in all of the other classes reported both PCS and MCS scores below 50, which indicate poorer QoL scores compared to the general population. Interestingly, patients in the all low class reported a mean PCS just above 50 (50.9) and a mean MCS score of 54.7, which suggests a slightly better QoL compared to the general population.
The statistically significant differences in PCS, MCS, and MQOLS-CA total QoL scores between the all low and all high classes in the current study represent large effect sizes according to the categorization of Cohen (d ≥ 0.8) [Citation31] that are clinically meaningful [Citation32]. Taken together, these findings support that a higher symptom burden is associated with clinically meaningful decrements in QoL.
Limitations
The current study has some limitations. First, the sample was heterogeneous in terms of cancer diagnoses and receipt of cancer treatments prior to inclusion. These differences may explain some of the inconsistent findings. Second, the majority of the patients was female (69%) and white, which may have influenced the results. Third, only symptoms that were reported by ≥30% of the sample were included in the analyses, which may omit or favor symptoms that are of importance for one group of patients. In addition, a larger sample may have produced different results. Finally, the stability of the subgroups over time was not evaluated.
Clinical and research implications
The main finding from this study is the identification of four distinct classes based on symptom occurrence rates, and that several demographic and clinical characteristics were associated with subgroup allocation. A higher symptom burden was associated with poorer QoL. Clinicians should assess symptoms prior to cancer treatment, with a specific focus on the most vulnerable patients (i.e. younger age, poorer functional status, and more comorbidities), so that appropriate symptom management strategies can be initiated.
Another important finding in the current study is identification of the low and high psychological classes where findings on several physical and psychological symptoms, as well as QoL outcomes, were opposite. Further research is needed that confirms the presence of the two classes and the distinguishing demographic and clinical characteristics, as well as the contradicting relationship between physical and psychological outcomes. In addition, studies that identify the underlying mechanisms that contribute to subgroup membership are needed. Additional research is warranted to develop and test symptom management interventions for the various subgroups.
Acknowledgments
The authors would like to thank the patients who participated in the study, and the nurses who facilitated the enrollment of patients.
Disclosure statement
No potential conflict of interest was reported by the authors.
Additional information
Funding
References
- Esther Kim JE, Dodd MJ, Aouizerat BE, et al. A review of the prevalence and impact of multiple symptoms in oncology patients. J Pain Symptom Manage. 2009;37:715–736.
- Gilbertson-White S, Aouizerat BE, Jahan T, et al. A review of the literature on multiple symptoms, their predictors, and associated outcomes in patients with advanced cancer. Pall Supp Care. 2011;9:81–102.
- Ferreira KA, Kimura M, Teixeira MJ, et al. Impact of cancer-related symptom synergisms on health-related quality of life and performance status. J Pain Symptom Manage. 2008;35:604–616.
- Gwede CK, Small BJ, Munster PN, et al. Exploring the differential experience of breast cancer treatment-related symptoms: a cluster analytic approach. Support Care Cancer. 2008;16:925–933.
- Miaskowski C, Cooper BA, Paul SM, et al. Subgroups of patients with cancer with different symptom experiences and quality-of-life outcomes: a cluster analysis. Oncol Nurs Forum. 2006;33:E79–E89.
- Pud D, Ben Ami S, Cooper BA, et al. The symptom experience of oncology outpatients has a different impact on quality-of-life outcomes. J Pain Symptom Manage. 2008;35:162–170.
- Illi J, Miaskowski C, Cooper B, et al. Association between pro- and anti-inflammatory cytokine genes and a symptom cluster of pain, fatigue, sleep disturbance, and depression. Cytokine. 2012;58:437–447.
- Reese JB, Blackford A, Sussman J, et al. Cancer patients' function, symptoms and supportive care needs: a latent class analysis across cultures. Qual Life Res. 2015;24:135–146.
- Snyder CF, Garrett-Mayer E, Blackford AL, et al. Concordance of cancer patients' function, symptoms, and supportive care needs. Qual Life Res. 2009;18:991–998.
- Miaskowski C, Cooper BA, Melisko M, et al. Disease and treatment characteristics do not predict symptom occurrence profiles in oncology outpatients receiving chemotherapy. Cancer. 2014;120:2371–2378.
- Miaskowski C, Dunn L, Ritchie C, et al. Latent class analysis reveals distinct subgroups of patients based on symptom occurrence and demographic and clinical characteristics. J Pain Symptom Manage. 2015;50:28–37.
- Langford DJ, Paul SM, Cooper B, et al. Comparison of subgroups of breast cancer patients on pain and co-occurring symptoms following chemotherapy. Support Care Cancer. 2016;24:605–614.
- Dodd MJ, Cho MH, Cooper BA, et al. Identification of latent classes in patients who are receiving biotherapy based on symptom experience and its effect on functional status and quality of life. Oncol Nurs Forum. 2011;38:33–42.
- Doong SH, Dhruva A, Dunn LB, et al. Associations between cytokine genes and a symptom cluster of pain, fatigue, sleep disturbance, and depression in patients prior to breast cancer surgery. Biol Res Nurs. 2015;17:237–247.
- Miaskowski C, Cooper BA, Aouizerat B, et al. The symptom phenotype of oncology outpatients remains relatively stable from prior to through 1 week following chemotherapy. Eur J Cancer Care. 2016. doi: 10.1111/ecc.12437.
- Karnofsky D. Performance scale. New York: Plenum Press; 1977.
- Schag CC, Heinrich RL, Ganz PA. Karnofsky performance status revisited: reliability, validity, and guidelines. J Clin Oncol. 1984;2:187–193.
- Sangha O, Stucki G, Liang MH, et al. The Self-Administered Comorbidity Questionnaire: a new method to assess comorbidity for clinical and health services research. Arthritis Rheum. 2003;49:156–163.
- Portenoy RK, Thaler HT, Kornblith AB, et al. The Memorial Symptom Assessment Scale: an instrument for the evaluation of symptom prevalence, characteristics and distress. Eur J Cancer. 1994;36:1326–130A.
- Hofso K, Rustoen T, Cooper BA, et al. Changes over time in occurrence, severity, and distress of common symptoms during and after radiation therapy for breast cancer. J Pain Symptom Manage. 2013;45:980–1006.
- Ferrell BR, Wisdom C, Wenzl C. Quality of life as an outcome variable in the management of cancer pain. Cancer. 1989;63:2321–2327.
- Ware J Jr, Kosinski M, Keller SD. A 12-Item Short-Form Health Survey: construction of scales and preliminary tests of reliability and validity. Med Care. 1996;34:220–233.
- Padilla GV. Validity of health-related quality of life subscales. Prog Cardiovasc Nurs. 1992;7:13–20.
- Ware JE, Kosinski M, Keller SD. How to score the SF-12 physical and mental summary scales. Boston, MA: New England Medical Center, Health Institute; 1995.
- Gandek B, Ware JE, Aaronson NK, et al. Cross-validation of item selection and scoring for the SF-12 Health Survey in nine countries: results from the IQOLA Project. International Quality of Life Assessment. J Clin Epidemiol. 1998;51:1171–1178.
- Muthén LK, Muthén BO. Mplus User’s Guide. 7th ed. Los Angeles, CA: Muthén & Muthén; 2012.
- Muthen B, Shedden K. Finite mixture modeling with mixture outcomes using the EM algorithm. Biometrics. 1999;55:463–469.
- Vermunt JK, Magdison J. Latent class cluster analyses. New York: Cambridge University Press; 2002.
- Nylund KL, Asparouhov T, Muthen BO. Deciding on the number of classes in latent class analysis and growth mixture modeling: a Monte Carlo simulation study. Struct Equ Model. 2007;14:535–569.
- Celeux GS. An entropy criterion for assessing the number of clusters in a mixture model. J Classification. 1996;13:195–212.
- Cohen J. Statistical power analysis for the behavioral sciences. 2 ed. New Jersey: Lawrence Erlbaum Associates; 1988.
- Sloan JA, Frost MH, Berzon R, et al. The clinical significance of quality of life assessments in oncology: a summary for clinicians. Support Care Cancer. 2006;14:988–998.