Abstract
Introduction: Prediction models using logistic regression may perform poorly in external patient cohorts. However, there is a need to standardize and validate models for clinical use. The purpose of this project was to describe a method for validation of external NTCP models used for patient selection in the randomized trial of protons versus photons in head and neck cancer radiotherapy, DAHANCA 35.
Material and methods: Organs at risk of 588 patients treated primarily with IMRT in the randomized controlled DAHANCA19 trial were retrospectively contoured according to recent international recommendations. Dose metrics were extracted using MatLab and all clinical parameters were retrieved from the DAHANCA database. The model proposed by Christianen et al. to predict physician-rated dysphagia was validated through the closed testing, where change of the model intercept, slope and individual beta’s were tested for significant prediction improvements.
Results: Six months prevalence of dysphagia in the validation cohort was 33%. The closed testing procedure for physician-rated dysphagia showed that the Christianen et al. model needed an intercept refitting for the best match for the Danish patients. The intercept update increased the risk of dysphagia for the validation cohort by 7.9 ± 2.5% point. For the raw model performance, the Brier score (mean squared residual) was 0.467, which improved significantly with a new intercept to 0.415.
Conclusions: The previously published Dutch dysphagia model needed an intercept update to match the Danish patient cohort. The implementation of a closed testing procedure on the current validation cohort allows quick and efficient validation of external NTCP models for patient selection in the future.
Introduction
Randomized clinical trials are expensive and cumbersome to process but is the gold standard of gaining understanding of the clinical benefit of new interventions. Within radiotherapy, few randomized trials have been undertaken to validate the introduction of new treatment modalities and treatment techniques [Citation1,Citation2]. However, with the rapid accessibility of proton treatment there is a possibility and unique opportunity to ensure high quality evidence for the use of protons for radiotherapy.
Comparing dose distribution between photon and proton radiotherapy treatment plan has been proposed as a method to select the patients who may have the highest theoretical gain of the new proton treatment modality [Citation3]. This selection of patients may improve the likelihood of a successful trial since patients with little or no theoretical gain will be excluded from entering the trial. The plan comparison may initially demonstrate the potential dose reduction that could be expected for a specific patient, however, it is not trivial to translate this information into a clinical gain since the dose-response relationship is not linear and may depend on dose to multiple organs and clinical factors, not apparent from the dose plan [Citation4]. One approach is to translate dose differences to clinical differences through normal tissue complication probability (NTCP) models. The prediction models using logistic regression typically originate from published models [Citation5,Citation6], which may perform poorly in external patient cohorts. Thus, before applying the models to an external patient cohort, there is a need to validate these NTCP models for clinical use. The Danish Head and Neck Cancer Group (DAHANCA) has decided that the evidence is not strong enough to recommend proton radiotherapy to head and neck cancer, even though dose planning studies suggest a benefit for selected patients [Citation3]. The uncertainties regarding dose delivery, relative biological effectiveness (RBE) and range uncertainties, and especially, the uncertainties regarding patient selection based on NTCP models created from photon treated patients, are so significant that a randomized study is planned, in the DAHANCA 35 trial.
The purpose of this project was to describe a method for validation of external NTCP models to be used for patient selection in the DAHANCA 35 trial, which is a national randomized trial of proton versus photon radiotherapy for the treatment of head–neck cancer.
Material and methods
Patients and contours
All patients included in the DAHANCA 19 trial who completed radiotherapy were included. The prospective randomized DAHANCA 19 trial investigated the benefit of adding the EGFR-inhibitor zalutumumab to radiotherapy for squamous cell carcinoma of the head and neck (H&N) and enrolled patients from 2007 to 2012 [Citation7] from six radiotherapy treatment centers. The results presented at ESTRO37 showed that zalutumumab did not improve loco-regional tumor control or survival, but resulted in more treatment related toxicity [Citation8–10].
All radiation treatment data were transferred to the national radiotherapy data storage [Citation11,Citation12] for quality assurance purposes [Citation13]. A total of 588 patients treated mainly with IMRT in DAHANCA19 had each 27 organs at risk (OARs) contoured retrospectively in the Pinnacle dose planning system according to recent international recommendations [Citation14] by a team of five experienced dose planners (radiographers) who underwent a training program and systematic evaluation, by an experienced radiation oncologist (JGE), of 30 selected cases. The dosimetric consequence of the contouring differences between the radiographers and oncologist were tested to estimate the consequences of the contour differences. The OAR structures all appear in the DAHANCA 2018 radiotherapy guidelines, see .
Table 1. Organs at risk in the DAHANCA validation cohort.
The radiotherapy plans were generated in Eclipse (four centers), Pinnacle (one center) and Oncentra masterplan (one center) according to the DAHANCA 2004 IMRT guidelines [Citation15]. The treatment plan used for the majority of fractions was used as the representative plan, which imply that no treatment adaptation information was included. Dosimetrics for the new contours were extracted using MatLab, where automated validation of the contours also was performed.
All demographic and clinical parameters were retrieved from the DAHANCA database, which is continuously being updated and curated by senior oncologists in all DAHANCA centers, covering all patients treated for H&N cancer in Denmark. The data are recorded for investigational as well as for quality assurance purposes [Citation16]. This includes recordings of normal tissue reactions which was conducted during radiation treatment and every third month thereafter for 2 years, then every sixth month until 5 years after completed radiotherapy. Normal tissue reactions were scored on an ordinal scale from 0 to 4 involving patient reported outcome such as dysphagia, hoarseness and xerostomia, and objective mucosal observations.
For the external model validation, the model proposed by Christianen et al. [Citation6], which employs the mean dose to the pharyngeal constrictor muscles and supraglottic larynx to predict physician-rated dysphagia, was selected. The DAHANCA dysphagia scale match the RTOG scale used by Christianen et al. The dysphagia endpoint was dichotomized into nonevent (grade 0–1) and event (grade 2–4) at 6 months. The baseline dysphagia was missing for all DAHANCA patient, but was imputed with week one during treatment score, alternatively the week two scores. All patients with grade 2–4 baseline dysphagia were excluded in from the model, since these were also excluded from the original model.
Closed testing procedure
The model was externally validated through the closed testing procedure [Citation17]. This procedure tests whether the model prediction significantly improves when changing the model intercept, the linear predictor slope or individual β’s. Each step is tested for a significant difference in log-likelihood using a χ2 test. The test will stop at the first step with a non-significant model prediction gain.
To confirm the closed testing procedure result, the validation cohort was split into five equally sized groups, similar to a five-fold validation. The closed testing procedure was performed on four of the five folds and model performance evaluated on the last fold and repeated five times with different validation folds each time.
A 2000 repetition bootstrap of the closed testing procedure was performed to endorse the model stopping point and determine confidence intervals.
The model performance was tested using area under the curve (AUC), and Brier score. The Brier score represents the mean squared error of the prediction. The model quality was compared using the calibration plots between the model predictions and actual clinical outcome for the validation cohort. The patients were grouped into 10 equally sized groups and the binominal uncertainty equal to one standard deviation was displayed in the error bars.
Results
The mean dose metrics for the contoured organs at risk is shown in , and the corresponding box plots are shown in the Supplementary Figures 2 and 3. The external contour was not delineated for all patients since this was not part of the recontouring process. The inner ear contour was present in 91% of the patients and missing in 9% due to planning CT scans that did not include this area. A few other structures were not delineated due to tumor invasion and consequent lack of normal tissue representation in the CT scan.
The prevalence of grade 2+ physician rate dysphagia at 6 months was 94 (33%) events out of 284 measures within one month of the 6 months endpoint.
The closed testing procedure for physician-rated dysphagia showed that the Christianen et al model needed an intercept refitting for best matching to the Danish patients. The intercept changed from –6.09 in the original model to –5.66 in the intercept-refitted model. The Brier score for the original model significantly improved by refitting the intercept from 0.467 to 0.415 (average squared residual error). The recalibration and full model revision did not significantly improve the model performance with Brier scores of 0.410 and 0.409, respectively. The AUC of the original model was 0.675, which did not change with the intercept refit. The calibration plots of the original model and the intercept refitted model are shown in . The calibration plots of the recalibrated and full revision models are shown in Supplementary Figure 1.
Figure 1. Calibration plot for the original and intercept refit models. The patients were grouped in to 10 equally sized groups (filled black circles) and the binominal uncertainty equal to one standard deviation is displayed in the error bars. The raw data are displayed as open gray circles with added noise, to illustrate the patient density.
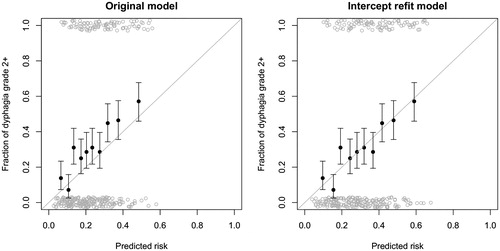
The linear predictor comprises the mean dose to the pharyngeal constrictor muscle and the supraglottic larynx (β0+β1×PCM superior Dmean β2×supraglottic larynx Dmean) is plotted in . The original model had β0 = –6.09, β1 = 0.057 and β2 = 0.037 and only the β0 changed to –5.66. The change in intercept increased the NTCP dysphagia risk on average 7.9 ± 2.5% points for the Danish patients.
Figure 2. Linear predictor plot for the original and intercept refit models. Linear predictor original model (–6.09 + 0.057 × PCM superior Dmean+0.037 × supraglottic larynx Dmean) and intercept refit model (–5.66 + 0.057 × PCM superior Dmean+0.037 × supraglottic larynx Dmean). The patients were grouped in to 10 equally sized groups (filled black circles) and the binominal uncertainty equal to one standard deviation is displayed in the error bars. The raw data are displayed as open gray circles with added noise, to illustrate the patient density.
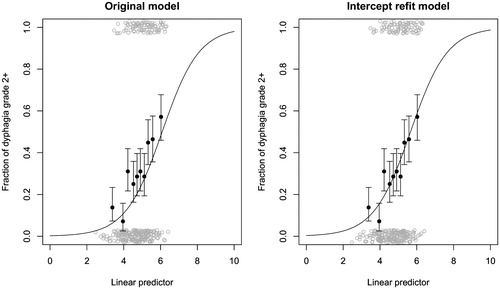
The fivefold closed testing procedure indicated the original model in one of the five folds and intercept refit in the remaining four folds. The mean intercept after refit in the five folds was –5.66 with a standard deviation of 0.07. The fivefold testing shows the robustness of the model on the validation cohort with very similar outcome for all five folds. The onefold suggesting no change of the original model indicate that the original model is a good fit for that cohort.
The bootstrap closed testing revealed a 24% original model and 69% intercept correction, indicating the same result as obtained with the closed testing procedure and the k-fold method.
Discussion
The validation through the closed testing procedure incl. the k-fold and bootstrap showed the Christianen et al. physician-rated dysphagia model to perform well on the current Danish validation cohort. Only a minor intercept adjustment was need for the model to match the Danish patients and implying that the model in principal could be used for clinical selection of patients in DAHANCA 35.
The prevalence of physician-rated grade 2+ dysphagia of 33% reported by Jensen et al. [Citation18] is in line with the previously reported data from DAHANCA 6 and 7. Which is an attractive prevalence with a nice balance of event and nonevent for modeling. Very rare or very frequent events are often difficult to predict and require very large data sets for model generation and likewise for external validation.
Validation of models is an essential part of implementing prediction models in the clinical routine. It is important to understand how the initial models were created and how potential differences between the model generation cohort and the validation cohort can influence the model performance. One issue is patient demographics which can skew the model and/or the validation. In addition, the endpoint may be interpreted differently at the different centers, which the closed testing procedure potentially would identify whereby an intercept update is suggested. However, it is important to investigate potential differences between the two cohorts, before performing the validation, since observed differences between model factors and parameters may be explained solely by selection bias and a postvalidation rationalization is rarely constructive. Differences in patient cohorts do not mean that the model cannot be used in the external cohort, but the validation process is even more critical and updates of β values will in this case be required.
When performing an external validation only part of the whole data information, i.e. data from original model development and validation data, is used. A more appealing approach is the use of combined data, since this potentially leads to a stronger and more generalizable model. However, there is a range of obstacles in this approach [Citation19], which often does not allow for data pulling. The closed testing procedure allows for most of the prior knowledge to be maintained and hence to transfer this knowledge to the clinical setting [Citation17]. The intercept and slope update preserve most of the prior information, while a refitting will lose the prior knowledge of the balance between predictors and thus only maintain the predictor selection knowledge. There is no attempt of selecting new predictors in the closed testing procedure, which would require parameter selection tools and both internal and external validation on its own.
Privacy legislation and hospital policies often prevent pooling of patient data. It has been suggested to overcome this issue by public anonymous data accessibility. However, with highly complex data, such as radiation oncology data, it is of high importance to understand the origin of the data in order to understand the outcome of the models. Distributed learning has been suggested as a tool to manage these issues [Citation20,Citation21]. Here the privacy sensitive data is stored in the local hospitals and only the prediction models move between the centers. This way the individual β values are iteratively updated and the model will utilize data information from all individual hospitals [Citation22]. A setup like this would make developing models in a robust environment possible across centers and dynamically add patients as they are treated and toxicity scored.
The ΔNTCP in a plan comparison calculation is affected by a change of the β values, but an intercept change will affect the linear part of the sigmoidal curve only little. This can be seen in , where the two lowest data points would result in a large change of ΔNTCP prediction, while the rest of the data points would have only small changes of ΔNTCP for all practical purposes. Simulating a dose reduction of 10 Gy for both organs at risk increased the ΔNTCP from the original model to the intercept updated model by 2.8 ± 1.0% point. The next step in the closed testing procedure changes the calibration slope which will affect the ΔNTCP for all patients.
NTCP models have been incorporated in many clinical decisions, but they have only been systematically used for selecting patients for specific radiotherapy modalities in recent years [Citation3,Citation23]. The use of clinical NTCP models introduces some caveats such as extrapolation, data origin, endpoint comparability etc., which need to be understood and managed in order for the clinical implementation to be safe [Citation24]. Most of these pitfalls can be handled and understood, thereby giving information on how treatment plans can improve, since dose reduction in specific organs at risk is not always the most beneficial for the specific patient. Here the NTCP values can help prioritize which organs to focus on.
It is important that the endpoint of the NTCP model is the same as will be used in the clinical trial. Different ways of assessing a specific morbidity may not yield the same results, e.g. it has been shown that physicians and patients may score the same symptom with a surprisingly low correlation [Citation25].
In conclusion, the previously published Dutch dysphagia model needed an update to match the Danish patient cohort. The closed testing procedure has been implemented, and future models are expected to be quickly and efficiently validated using the current method and validation cohort.
Supplemental Material
Download PDF (527 KB)Disclosure statement
No potential conflict of interest was reported by the authors.
Additional information
Funding
References
- Nutting CM, Morden JP, Harrington KJ, et al. Parotid-sparing intensity modulated versus conventional radiotherapy in head and neck cancer (PARSPORT): a phase 3 multicentre randomised controlled trial. Lancet Oncol. 2011;12:127–136.
- Gupta T, Kannan S, Ghosh-Laskar S, et al. Systematic review and meta-analyses of intensity-modulated radiation therapy versus conventional two-dimensional and/or or three-dimensional radiotherapy in curative-intent management of head and neck squamous cell carcinoma. PLoS One. 2018;13:e0200137.
- Langendijk JA, Lambin P, De Ruysscher D, et al. Selection of patients for radiotherapy with protons aiming at reduction of side effects: the model-based approach. Radiother Oncol. 2013;107:267–273.
- Langendijk JA, Doornaert P, Rietveld DH, et al. A predictive model for swallowing dysfunction after curative radiotherapy in head and neck cancer. Radiother Oncol. 2009;90:189–195.
- Beetz I, Schilstra C, van der Schaaf A, et al. NTCP models for patient-rated xerostomia and sticky saliva after treatment with intensity modulated radiotherapy for head and neck cancer: the role of dosimetric and clinical factors. Radiother Oncol. 2012;105:101–106.
- Christianen ME, Schilstra C, Beetz I, et al. Predictive modelling for swallowing dysfunction after primary (chemo)radiation: results of a prospective observational study. Radiother Oncol. 2012;105:107–114.
- Brondum L, Alsner J, Sorensen BS, et al. Associations between skin rash, treatment outcome, and single nucleotide polymorphisms in head and neck cancer patients receiving the EGFR-inhibitor zalutumumab: results from the DAHANCA 19 trial. Acta Oncol. 2018;57:1159–1164.
- Eriksen JG, Maare C, Johansen J, et al. OC-0271: 5-Y update of the randomized phase III trial DAHANCA19: primary (Chemo) RT ± zalutumumab in HNSCC. Radiother Oncol. 2018;127:S137–S138.
- Zukauskaite R, Hansen CR, Brink C, et al. Analysis of CT-verified loco-regional recurrences after definitive IMRT for HNSCC using site of origin estimation methods. Acta Oncol. 2017;56:1554–1561.
- Zukauskaite R, Hansen CR, Grau C, et al. Local recurrences after curative IMRT for HNSCC: effect of different GTV to high-dose CTV margins. Radiother Oncol. 2018;126:48–55.
- Westberg J, Krogh S, Brink C, et al. A DICOM based radiotherapy plan database for research collaboration and reporting. J Phys Conf Ser. 2014;489:012100.
- Brink C, Lorenzen EL, Krogh SL, et al. DBCG hypo trial validation of radiotherapy parameters from a national data bank versus manual reporting. Acta Oncol. 2018;57:107–112.
- Samsøe E, Andersen E, Hansen CR, et al. PO-0944: radiotherapy QA of the DAHANCA 19 protocol. Radiother Oncol. 2015;115:S494–S495.
- Brouwer CL, Steenbakkers RJ, Bourhis J, et al. CT-based delineation of organs at risk in the head and neck region: DAHANCA, EORTC, GORTEC, HKNPCSG, NCIC CTG, NCRI, NRG Oncology and TROG consensus guidelines. Radiother Oncol. 2015;117:83–90.
- Hansen CR, Johansen J, Kristensen CA, et al. Quality assurance of radiation therapy for head and neck cancer patients treated in DAHANCA 10 randomized trial. Acta Oncol. 2015;54:1669–1673.
- Overgaard J, Jovanovic A, Godballe C, et al. The Danish Head and Neck Cancer database. Clin Epidemiol. 2016;8:491–496.
- Vergouwe Y, Nieboer D, Oostenbrink R, et al. A closed testing procedure to select an appropriate method for updating prediction models. Statist Med. 2017;36:4529–4539.
- Mortensen HR, Overgaard J, Jensen K, et al. Factors associated with acute and late dysphagia in the DAHANCA 6 and 7 randomized trial with accelerated radiotherapy for head and neck cancer. Acta Oncol. 2013;52:1535–1542.
- Deasy JO, Bentzen SM, Jackson A, et al. Improving normal tissue complication probability models: the need to adopt a “data-pooling” culture. Int J Radiat Oncol Biol Phys. 2010;76:S151–S154.
- Lambin P, Roelofs E, Reymen B, et al. 'Rapid Learning health care in oncology' – an approach towards decision support systems enabling customised radiotherapy. Radiother Oncol. 2013;109:159–164.
- Lambin P, Zindler J, Vanneste B, et al. Modern clinical research: how rapid learning health care and cohort multiple randomised clinical trials complement traditional evidence based medicine. Acta Oncol. 2015;54:1289–1300.
- Roelofs E, Persoon L, Nijsten S, et al. Benefits of a clinical data warehouse with data mining tools to collect data for a radiotherapy trial. Radiother Oncol. 2013;108:174–179.
- Cheng Q, Roelofs E, Ramaekers BL, et al. Development and evaluation of an online three-level proton vs photon decision support prototype for head and neck cancer: comparison of dose, toxicity and cost-effectiveness. Radiother Oncol. 2016;118:281–285.
- Marks LB, Yorke ED, Jackson A, et al. Use of normal tissue complication probability models in the clinic. Int J Radiat Oncol Biol Phys. 2010;76:S10–S19.
- Jensen K, Bonde Jensen A, Grau C. The relationship between observer-based toxicity scoring and patient assessed symptom severity after treatment for head and neck cancer. A correlative cross sectional study of the DAHANCA toxicity scoring system and the EORTC quality of life questionnaires. Radiother Oncol. 2006;78:298–305.