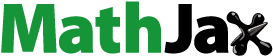
Abstract
Background: An elevated neutrophil-lymphocyte ratio (NLR) is associated with poor prognosis in advanced renal cell carcinoma (RCC). We examined whether the addition of NLR improves the risk reclassification of advanced RCC using current prognostic tools from the Memorial Sloan Kettering Cancer Center (MSKCC) and International Metastatic Renal Cell Carcinoma Database Consortium (IMDC).
Methods: Using randomised data from the COMPARZ trial of first-line pazopanib vs. sunitinib in advanced RCC, we constructed multivariable models containing MSKCC and IMDC predictor variables with and without NLR. We evaluated model discrimination using the concordance index (C-index). We computed net reclassification improvement to quantify patient reclassification into low/intermediate/poor risk groups with the addition of NLR.
Results: Of 1102 patients, NLR ≥ 5 (16%) was associated with shorter survival adjusting for MSKCC variables (adjusted HR 1.89, p < .001). Adding NLR to MSKCC variables increased the C-index by 0.01. Among patients who died before 24 months (N = 415), adding NLR reclassified 8% and 2% to a higher and lower risk category, respectively. Among those alive at 24 months (N = 636), adding NLR reclassified 4% and 1% to a higher and lower risk category, respectively. This finding translates to a net benefit of eight additional patients who die within 24 months correctly identified as poor risk per 1000 patients tested. We obtained similar results when evaluating NLR with IMDC variables.
Conclusions: NLR does not substantially improve risk reclassification over pre-existing prognostic tools. MSKCC and IMDC classifications remain the standard for guiding risk-directed therapy and trial stratification of patients with advanced RCC.
Background
An elevated neutrophil-lymphocyte ratio (NLR) is associated with poor prognosis in advanced renal cell carcinoma (RCC) [Citation1–6] and other solid tumours [Citation7–9]. This finding is consistent with the hypothesis that systemic inflammation plays a vital role in cancer development and progression [Citation10,Citation11], and is supported by similar findings for other markers of systemic inflammatory response such as C-reactive protein [Citation12–15].
The Memorial Sloan Kettering Cancer Center (MSKCC) [Citation16] and International Metastatic Renal Cell Carcinoma Database Consortium (IMDC) [Citation17] tools classify prognosis for advanced RCC patients. These tools combine patient and disease factors that predict overall survival (OS) and are widely used to guide treatment decisions and stratify patients in trials. The MSKCC risk tool includes time from diagnosis to development of metastasis, Karnofsky performance status, lactate dehydrogenase (LDH), anaemia and corrected calcium. The IMDC risk tool updated the MSKCC tool by replacing LDH with absolute neutrophil count (ANC) and platelet counts as markers of inflammation. For both tools, individuals with no adverse variables are classified as good risk, one or two risk variables as intermediate risk, and three or more risk variables as poor risk.
To consider pre-treatment NLR for routine use as a prognostic marker, it needs to meet several requirements. First, the effect of NLR on OS needs to be consistently reproducible in different patient groups from multiple settings. Second, the effect needs to add to established factors such as those in the MSKCC and IMDC tools. Finally, NLR also needs to correctly reclassify patients to risk groups from the current classification based on pre-existing tools. Specifically, correct reclassification from intermediate risk into either good or poor risk groups is of particular clinical value as it will improve risk-directed therapy and trial stratification [Citation18,Citation19].
Additionally, little is known about the impact of change in NLR from baseline after commencement of systemic therapy [Citation20]. The current MSKCC and IMDC tools rely on baseline factors and do not account for the impact of change in these factors with systemic therapy. Evaluation of the prognostic value of NLR beyond baseline in patients with advanced RCC may help guide decision-making.
To address these gaps, we used data from the COMPARZ study to assess the prognostic value of pre-treatment NLR. We quantified the degree of reclassification of patients into either better or poorer risk categories with the addition of NLR to established risk factors. We also evaluated the clinical performance of change in NLR from baseline following commencement of tyrosine kinase inhibitor (TKI) therapy.
Methods
Patients
We used data from the COMPARZ study, a randomised, non-inferiority trial of first-line TKI therapy (pazopanib vs. sunitinib) in patients with advanced or metastatic RCC with a clear-cell histologic component. Study design and eligibility criteria have been previously reported [Citation21]. All randomly assigned patients with data available for pre-treatment NLR were included in the present analysis.
Neutrophil and lymphocyte counts
White blood cell counts, ANC, lymphocyte counts, haemoglobin and platelet counts were measured in local laboratories according to their standard practices. NLR was defined as the ratio of ANC to lymphocyte count. There is no widely accepted cut-point for NLR with previous studies using a cut-point from 2.5 to 5.0 [Citation22]. We examined NLR as a continuous and categorical variable dichotomised at a cut-point of 5 based on prior work using an external cancer registry [Citation23].
Prognostic models
We developed ‘base’ MSKCC and IMDC models to predict the event at 24 months (OS) by building multivariable Cox proportional hazards models using the dichotomised risk variables included in the original MSKCC and IMDC tools. We developed ‘full’ MSKCC and IMDC models by adding NLR as a continuous (logarithmic transformed) and categorical variable (<5, ≥5).
Statistical analysis
To assess the prognostic value of pre-treatment NLR, we estimated the distributions of OS and progression-free survival (PFS) for patients classified by NLR level (<5, ≥5) using the Kaplan–Meier method. We developed univariable and multivariable Cox models adjusted for MSKCC and IMDC variables and presented the hazard ratios (HR) and associated 95% confidence intervals (CIs) for PFS and OS. We repeated these analyses for NLR as a continuous logarithmic transformed and categorical variable (<5, ≥5).
We evaluated the discrimination of each model using the concordance index (C-index). The C-index is a probability of concordance between the ranking of predicted survival for two individuals and the ranking of their observed survival, with C = 0.5 for no discrimination, for example, random predictions, and C = 1 for a perfectly discriminating model [Citation24].
We calculated the net reclassification improvement (NRI) to describe changes in risk classification by adding NLR to the MSKCC and IMDC base models. The absolute NRI represents the net proportion of patients correctly reclassified by the model. It is calculated as the sum of patients dying within 24 months who were correctly reclassified as poor risk and those surviving at 24 months who were correctly classified as good risk; minus the sum of patients incorrectly reclassified as intermediate/good risk and those incorrectly reclassified as intermediate/poor risk presented as a proportion of all patients [Citation25]. Using each model, we classified patients as good risk if their predicted risk <25%, the intermediate group 25–75%, and the poor risk group >75% [Citation25].
To quantify reclassification with the addition of NLR in clinical terms, we calculated the difference in net benefit between full and base models. The net benefit estimates the number of additional patients who have the event, in this case death within 24 months, who are correctly classified as poor risk, that is, ‘true positives’, with no additional patients incorrectly classified as poor risk, that is, ‘false positives’ per 1000 patients tested. For the purpose of comparing risk models, the advantage of the net benefit over the NRI is that it takes into account the frequency of the event and does not assume that misclassification into high (false positive) and low (false negative) risk groups carries the same weight. The formula for calculating net benefit for each model is as follows [Citation26]:
The threshold probability is the risk cutoff where the relative value of avoiding a false positive equals the relative value of finding a true positive (in this case, >25% for distinguishing intermediate and poor from good risk and >75% for the poor risk group).
We examined the pattern of change in NLR level from baseline. We also evaluated the 2-year conditional OS by NLR level (<5, ≥5) at 6-week time interval increments from randomisation and up to 24 weeks. The 2-year conditional survival is defined as the probability of a patient surviving an additional 2 years at each time-point, conditioned on the patient remaining alive at that time-point. We analysed conditional OS using a landmark analysis approach, excluding those who died before the landmark time-point [Citation27]. We measured OS times from the landmark time-points until death, or censored at the date of last follow-up, or at 2 years from the landmark time-point if the last follow-up date was beyond 2 years.
All multivariable models were stratified by treatment arm. We assessed multicollinearity using condition indices with corresponding variance proportions. As all analyses were exploratory, they were not adjusted for multiple testing. All p-values were two-sided.
Results
Patients
Of 1110 patients randomised in the COMPARZ trial, 1102 (99.3%) had pre-treatment NLR data available and were included in the analyses. Mean patient age was 61.1 years (range 18–88 years), 73% of patients were male and 83% had a prior nephrectomy (). At a median follow-up of 28.2 months, 654 (59.4%) patients had disease progression and 497 (45.1%) died. 1051 patients had data available for all MSKCC and NLR variables and 1058 patients for all IMDC and NLR variables.
Table 1. Baseline characteristics of patientsTable Footnotea overall and by neutrophil-to-lymphocyte ratio.
Distribution of NLR
The median pre-treatment NLR was 2.8 (interquartile range 2.0–4.1). 171 (15.5%) patients had pre-treatment NLR ≥ 5. Patients with elevated pre-treatment NLR were older, more likely to have a Karnofsky performance status of below 80%, anaemia, an elevated LDH and corrected calcium ().
NLR decreased during treatment mirrored the decrease in the sum of target lesions (STL) at each 6-week time-point from randomisation and up to 18 weeks. There was a mean increase in NLR after 18 weeks but STL continued to decrease (Supplementary Figure 1).
Prognostic value of pre-treatment NLR
In univariate analyses, NLR ≥ 5 was associated with significantly shorter OS and PFS. The median OS was 12.2 and 32.6 months for NLR ≥ 5 and NLR < 5, respectively (HR 2.45, 95% CI 1.99–3.02, p < .001, Supplementary Figure 2(A)). The median PFS was 4.2 and 10.9 months for NLR ≥ 5 and NLR <5, respectively (HR 1.84, 95% CI 1.51–2.26, p < .001, Supplementary Figure 2(B)). When examined as a continuous variable, each doubling of NLR was associated with poorer OS (HR 1.85, p < .001) and PFS (HR 1.44, p < .001) ().
Table 2. Hazard ratio for the association between pre-treatment NLR as a continuous, categorical variable and PFS and OS.
Impact of pre-treatment NLR on OS and PFS
The Kaplan–Meier curves for OS and PFS indicate that NLR provides prognostic information within each of the MSKCC-defined (Supplementary Figure 3) and IMDC-defined (Supplementary Figure 4) good, intermediate, and poor risk subgroups, respectively.
After adjustment for MSKCC-defined risk variables, NLR was associated with significant 1.6-fold and 1.9-fold increases in the risk of death when analysed as a continuous or a categorical variable, respectively (). Similar results were seen when NLR was adjusted for IMDC-defined risk variables ().
For prediction of OS, the base MSKCC model had a C-index value of 0.67. Addition of NLR resulted in a minor improvement in discrimination (NLR as a continuous variable: C-index = 0.70; NLR (<5, ≥5): C-index = 0.68). Similar results were seen when NLR was adjusted for IMDC-defined risk variables ().
Table 3. C-index of base and full models in all subjects.
After adjustment for MSKCC-defined risk variables, NLR was associated with a significant 1.3-fold and 1.6-fold increase in the risk of disease progression when analysed as a continuous or categorical variable, respectively (). Similar results were seen when NLR was adjusted for IMDC-defined risk variables ().
For prediction of PFS, the base MSKCC model had a C-index value of 0.62. Addition of NLR also resulted in a minor improvement in discrimination (NLR as a continuous variable: C-index = 0.63; NLR (<5, ≥5): C-index = 0.66). Similar results were seen when NLR was adjusted for IMDC-defined risk variables ().
In all multivariable models, all covariates had condition indices of <5 with corresponding variance proportions <0.3, indicating no statistical evidence of multi-collinearity. We obtained similar results when repeating analyses and confining them to patient data from the pazopanib and the sunitinib randomised arms respectively (results not shown).
Net reclassification improvement
Among the patients who died before 24 months (N = 415), addition of NLR (<5, ≥5) to the MSKCC ‘base’ variables reclassified 32 (8%) of patients to a higher risk category and 9 (2%) to a lower risk category. Among those alive at 24 months (N = 636), 24 (4%) were classified to a higher risk category and 7 (1%) to a lower risk category. The overall NRI was 0.03 (. This finding translates to a net benefit of eight additional patients who die within 24 months correctly identified as poor risk per 1000 patients tested. Alternatively, the net benefit can be expressed as 20 additional patients who survive to 24 months correctly identified as good risk per 1000 tested (Supplementary appendix).
Table 4. Reclassification of patient prognosis after addition of NLR (<5, ≥5) to (a) MSKCC and (b) IMDC factors.
Among the patients who died before 24 months, addition of NLR (<5, ≥5) to the IMDC ‘base’ variables reclassified 30 (7%) to a higher risk category and 5 (1%) to a lower risk category. Among those alive at 24 months, 14 (2%) were classified to a higher risk category and 9 (1%) to a lower risk category. The overall NRI was 0.05 (. This finding translates to a net benefit of 11 patients who die within 24 months correctly identified as poor risk per 1000 patients tested. Alternatively, the net benefit can be expressed as 20 additional patients who survive to 24 months identified as good risk per 1000 patients tested (Supplementary appendix).
We performed a sensitivity analysis by excluding ANC from the IMDC model. When NLR was added to the IMDC model without ANC, the overall NRI was 0.04. As ANC and platelet counts are markers of inflammation, and these variables are part of the IMDC model, replacement of these two variables with NLR resulted in an overall NRI of 0.01.
When NLR as a continuous variable was added to the base MSKCC and IMDC risk variables, we demonstrated consistent results (Supplementary Table 1). NRI and net benefit calculated at different time-points using categorical NLR added to the base MSKCC and IMDC risk variables also demonstrated consistent results (not shown). Bootstrap validation for all models had no impact on the overall NRI results (not shown).
Conditional OS landmark analysis
Of patients with pre-treatment NLR < 5, 84–87% remained in this group at all landmark time-points. Of patients with pre-treatment NLR ≥ 5, 10–11% changed to NLR < 5 at subsequent landmark time-points. Of patients with pre-treatment NLR < 5, 1–3% changed to NLR ≥ 5 at subsequent landmark time-points (Supplementary Table 2 and Supplementary Figure 5).
Of 934 patients with pre-treatment NLR < 5, the 2-year conditional OS remained relatively constant over the 6-month period after randomisation (). Of 168 patients with pre-treatment NLR ≥ 5, the 2-year conditional OS decreased marginally from 31% (95% CI 24–38%) to 20% (95% CI 6–39%) at Week 18 before increasing to 38% (95% CI 14–61%) at 6 months after randomisation ().
Figure 1. Probability of surviving two additional years. Probability conditioned on time survived after randomisation and stratified by (A) NLR with cut-point of 5 and (B) NLR with cut-point of 3. Error bars are 95% CI.
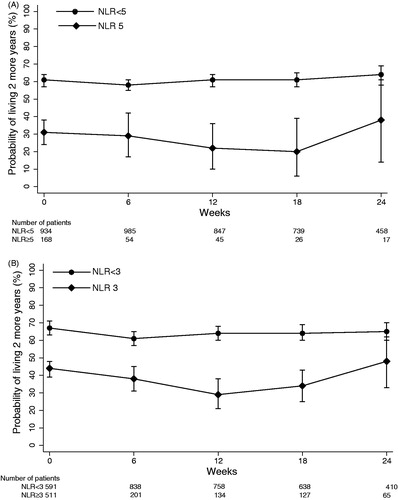
We performed a sensitivity analysis by repeating our analysis based on an NLR cut-point of 3. Of 591 patients with pre-treatment NLR < 3, the 2-year conditional OS also remained relatively constant over the 6-month period from randomisation. Of 511 patients with pre-treatment NLR ≥ 3, the 2-year conditional OS decreased from 44% (95% CI 39–48%) to 29% (95% CI 21–38%) before increasing to 48% (95% CI 33–62) at 6 months after randomisation ().
Discussion
This study confirms that a high pre-treatment NLR predicts shorter PFS and OS in patients with advanced RCC treated with first-line TKI. NLR was prognostic for OS across the good, intermediate and poor risk groups as determined by the MSKCC and IMDC classifications. However, the addition of NLR to the MSKCC and IMDC variables only resulted in modest improvements for reclassifying patients to better or worse risk categories.
Our finding that elevated NLR is associated with shorter OS and PFS is consistent with the findings of other studies in advanced RCC [Citation2,Citation3,Citation5,Citation6]. These studies assessed NLR and its association with survival outcomes but neither compared NLR to the existing risk tools nor reported on its prognostic value in re-classifying patient risk-groups when added to existing risk tools. We extend this evidence by assessing the incremental value of NLR to prognostic tools that are widely available and used in practice [Citation28,Citation29].
In our study, patients with elevated NLR were more likely to have adverse features including worse performance status and elevated LDH (both included in the current MSKCC prognostic tool). Despite being a statistically significant prognostic marker in multivariable analyses, we found only modest improvements in the C-indices when NLR was added to the MSKCC and IMDC base models. This finding suggests a new prognostic marker/s will need to have a much stronger association with survival outcomes in order to substantially improve the risk discrimination provided by the current prognostic tools.
The C-index does not provide information about how many patients may benefit from improved risk reclassification [Citation30]. Thus to consider the prognostic value of adding NLR to existing prognostic tools in clinical terms, we examined the proportion of patients correctly moving up into a poorer risk category or down into a better risk category. For clinical interpretation, patients reclassified from the intermediate to good risk group may benefit from a recommendation for upfront TKI therapy and avoid combination immunotherapy [Citation31]. For example, if a patient with newly diagnosed metastatic clear-cell RCC was categorised as IMDC intermediate risk, guidelines recommend doublet immunotherapy [Citation28,Citation29]. However, if NLR added to IMDC re-classifies this patient to good risk-group, then TKI would be recommended [Citation28,Citation29]. We estimate only 20 additional patients per 1000 would be identified as good risk when NLR is added to either the MSKCC and IMDC risk variables. Patients reclassified from the intermediate to the poor risk-group may benefit from a recommendation for upfront immunotherapy and avoid first-line TKI therapy [Citation28,Citation29,Citation31,Citation32]. However, we estimate only eight additional patients per 1000 and 11 additional patients per 1000 tested would be identified as poor risk when NLR is added to the MSKCC and IMDC risk variables, respectively. Therefore, adding NLR to the MSKCC and IMDC risk variables did not result in a substantial change in treatment recommendations for the vast majority of patients with advanced RCC. Sensitivity analyses with NLR replacing ANC alone or both ANC and platelet counts in the IMDC model also did not result in a substantial change in treatment recommendations. While we acknowledge that NLR as a marker for the systemic inflammatory process is associated with poor prognosis in cancer, our findings highlight that adding NLR to existing prognostic tools that already accounts for inflammatory markers does not provide substantial change in risk reclassification and treatment recommendations.
Our study comprehensively assessed the prognostic value of change in NLR following the initiation of TKI therapy. Only a minority of patients changed to either a higher or lower NLR level with TKI therapy. Patients with high NLR at any time-point from baseline had a lower survival probability than those with low NLR (). Our data also shows that on-treatment NLR predicts a similar 2-year survival probability as pre-treatment NLR. These data suggest that on-treatment NLR has limited utility in guiding treatment decisions while on TKI therapy.
A major strength of our study is our use of a high quality randomised trial dataset with over 1000 participants and pre-treatment NLR available for almost all patients. Our findings for the validation of the prognostic value of pre-treatment NLR are also generalisable to current clinical practice as sunitinib and pazopanib are widely used in routine clinical care. We also acknowledge the limitations of using a trial dataset. Our study sample included fewer poor prognosis patients than may be expected in routine clinical practice by virtue of the stringent selection requirement of the COMPARZ trial. Our study also had a median follow-up of 28 months in which only 45% of patients had died. Model discrimination may be higher with longer follow-up and in non-trial settings where there is a wider range of patients and prediction estimates. The choice of different cut-points for NLR could also potentially influence its prognostic value. There is currently no widely accepted cut-point in research or routine clinical use. A meta-analysis of 14 retrospective studies on the prognostic role of NLR in RCC reported NLR ranged from 2.5 to 5 [Citation22]. Our analysis using continuous NLR showed worse OS and PFS for every doubling of NLR. Although our cut-point of 5 is derived from an external cancer registry, we also performed sensitivity analyses using a cut-point of 3 based on optimal cut-point analysis with similar findings (results not presented).
Our findings also have important implications for future biomarker research. While TKI is a standard of care for first-line therapy of advanced RCC, immunotherapy is emerging as an alternative upfront systemic therapy. Recently, checkpoint inhibitors, either as combination doublet [Citation31] or monotherapy in combination with a TKI [Citation32], have shown improved survival outcomes when compared to TKI monotherapy in the intermediate and poor risk-groups. However, the MSKCC and IMDC tools have not been validated in the checkpoint inhibitor setting. It is therefore important to continue assessing the performance of MSKCC and IMDC risk tools in the checkpoint inhibitor setting as new prognostic markers, new combinations of markers, or other immune signatures are proposed, and updating the existing tools when gains are made to better guide treatment decision-making and patient stratification in clinical trials.
Additionally, with the exponential increase in discovery of promising novel prognostic biomarkers, our findings serve to highlight that while validation of the association between a prognostic biomarker and survival outcomes, is an important first step, it may not necessarily translate to a clinically valuable test. It is also essential to demonstrate the biomarker can improve the risk stratification of patients to better or poorer risk categories to inform treatment recommendations.
In conclusion, while elevated pre-treatment NLR is a significant independent predictor of shorter OS and PFS for patients with advanced RCC undergoing first-line TKI therapy, it does not substantially reclassify patients or change treatment recommendations compared to the variables used in existing prognostic tools. Change in NLR from baseline with TKI therapy predicts similar 2-year survival as pre-treatment NLR. The MSKCC and IMDC risk tools remain the standard for guiding risk-directed therapy and trial stratification of patients with advanced RCC.
Additional information
Ethics approval and consent to participate
The COMPARZ study was performed in accordance with the Declaration of Helsinki.
Consent for publication
We obtained consent for publication from the trial sponsor, Novartis, who otherwise had no role in the study. All the patients provided written informed consent.
Data availability
Data supporting the results are presented in the main manuscript and supplementary file.
Authors’ contributions
The authors meet criteria for authorship as recommended by the International Committee of Medical Journal Editors (ICMJE). AT contributed to the conception of the study, performed the data analysis, interpreted the results, wrote the primary manuscript, and revised the manuscript. DG contributed to the conception of the study, data acquisition, interpretation of the results, and manuscript revision. SL, MH, and VG contributed to the design of the study, interpretation of the results, and manuscript revision. PDS contributed to the interpretation of the results and manuscript revision. RM contributed to data acquisition, interpretation of the results, and manuscript revision. CL contributed to the conception and design of the study, data acquisition, interpretation of the results, and manuscript revision. All authors approved the final version.
Supplemental Material
Download (7.9 MB)Acknowledgments
We acknowledge Novartis for providing the individual patient data for the purposes of the analysis and the editorial support provided by Dr Sherilyn Goldstone, PhD (NHMRC Clinical Trials Centre).
Disclosure statement
RM declares a consultancy role for Pfizer, Novartis, Genentech/Roche, Exelixis, Merck, Eisai, Incyte, and Lilly. RM declares clinical trial support to employer (Memorial Sloan Kettering) from Bristol Myers Squibb, Pfizer, Eisai, Genentech/Roche. DG declares research support to his institution from Celgene, Amgen, Pfizer and research statistical support from GSK. All other authors declare no conflict of interest.
References
- Cetin B, Berk V, Kaplan M, et al. Is the pretreatment neutrophil to lymphocyte ratio an important prognostic parameter in patients with metastatic renal cell carcinoma? Clin Genitourinary Cancer. 2013;11:141–148.
- Kobayashi M, Kubo T, Komatsu K, et al. Changes in peripheral blood immune cells: their prognostic significance in metastatic renal cell carcinoma patients treated with molecular targeted therapy. Med Oncol. 2013;30:1–11.
- Ohno Y, Nakashima J, Ohori M, et al. Clinical variables for predicting metastatic renal cell carcinoma patients who might not benefit from cytoreductive nephrectomy: neutrophil-to-lymphocyte ratio and performance status. Int J Clin Oncol. 2014;19:139–145.
- Fox P, Hudson M, Brown C, et al. Markers of systemic inflammation predict survival in patients with advanced renal cell cancer. Br J Cancer. 2013;109:147–153.
- Keizman D, Ish-Shalom M, Huang P, et al. The association of pre-treatment neutrophil to lymphocyte ratio with response rate, progression free survival, and overall survival of patients treated with sunitinib for metastatic renal cell carcinoma. Eur J Cancer. 2012;48:202–208.
- Zhang G, Zhu Y, Gu W, et al. Pretreatment neutrophil‑to‑lymphocyte ratio predicts prognosis in patients with metastatic renal cell carcinoma receiving targeted therapy. Int J Clin Oncol. 2016;21:373–378.
- Dolan R, Lim J, McSorley S, et al. The role of the systemic inflammatory response in predicting outcomes in patients with operable cancer: systematic review and meta-analysis. Sci Rep. 2017;7:16717.
- Guthrie G, Charles K, Roxburgh C, et al. The systemic inflammation-based neutrophil–lymphocyte ratio: experience in patients with cancer. Crit Rev Oncol Hematol. 2013;88:218–230.
- Templeton A, McNamara M, Šeruga B, et al. Prognostic role of neutrophil-to-lymphocyte ratio in solid tumors: a systematic review and meta-analysis. J Natl Cancer Inst. 2014;106:dju124.
- Coussens L, Werb Z. Inflammation and cancer. Nature. 2002;420:860–867.
- Fitzgerald J, Nayak B, Shanmugasundaram K, et al. Nox4 mediates renal cell carcinoma cell invasion through hypoxia-induced interleukin 6- and 8- production. PLoS One. 2012;7:e30712.
- Zhou L, Cai X, Liu Q, et al. Prognostic role of C-reactive protein in urological cancers: a meta-analysis. Sci Rep. 2015;5:12733
- Négrier S, Escudier B, Gomez F, et al. Prognostic factors of survival and rapid progression in 782 patients with metastatic renal carcinomas treated by cytokines: a report from the Groupe Français d'Immunothérapie. Ann Oncol. 2002;13:1460–1468.
- Chen Z, Shao Y, Wang K, et al. Prognostic role of pretreatment serum albumin in renal cell carcinoma: a systematic review and meta-analysis. Onco Targets Ther. 2016;9:6701–6710.
- Negrier S, Perol D, Menetrier-Caux C, et al. Interleukin-6, interleukin-10, and vascular endothelial growth factor in metastatic renal cell carcinoma: prognostic value of interleukin-6—from the Groupe Français d'Immunothérapie. JCO. 2004;22:2371–2378.
- Motzer R, Bacik J, Murphy B, et al. Interferon-alfa as a comparative treatment for clinical trials of new therapies against advanced renal cell carcinoma. JCO. 2002;20:289–296.
- Heng D, Xie W, Regan M, et al. Prognostic factors for overall survival in patients with metastatic renal cell carcinoma treated with vascular endothelial growth factor–targeted agents: results from a large, multicenter study. JCO. 2009;27:5794–5799.
- Simon R, Altman DG. Statistical aspects of prognostic factor studies in oncology. Br J Cancer. 1994;69:979–985.
- McShane LM, Altman DG, Sauerbrei W, et al. REporting recommendations for tumour MARKer prognostic studies (REMARK). Br J Cancer. 2005;93:387–391.
- Templeton A, Knox J, Lin X, et al. Change in neutrophil-to-lymphocyte ratio in response to targeted therapy for metastatic renal cell carcinoma as a prognosticator and biomarker of efficacy. Eur Urol. 2016;2016:358–364.
- Motzer R, Hutson T, Cella D, et al. Pazopanib versus sunitinib in metastatic renal-cell carcinoma. N Engl J Med. 2013;368:722–731.
- Hu K, Lou L, Ye J, et al. Prognostic role of the neutrophil-lymphocyte ratio in renal cell carcinoma: a meta-analysis. BMJ Open. 2015;5:e006404
- Proctor M, Morrison D, Talwar D, et al. A comparison of inflammation-based prognostic scores in patients with cancer. A Glasgow Inflammation Outcome Study. Eur J Cancer. 2011;47:2633–2641.
- Harrell FJ. Regression modeling strategies: with applications to linear models, logistic regression and survival analysis. New York (NY): Springer; 2001.
- Pencina MJ, D' Agostino RB, D' Agostino RB, et al. Evaluating the added predictive ability of a new marker: from area under the ROC curve to reclassification and beyond. Statist Med. 2008;27:157–172.
- Vickers AJ, Elkin EB. Decision curve analysis: a novel method for evaluating prediction models. Med Decis Making. 2006;26:565–574.
- Anderson J, Cain K, Gelber R. Analysis of survival by tumor response. J Clin Oncol. 1983;1:710–719.
- Ljungberg B, Albiges L, Bensalah K. EAU guidelines: renal cell carcinoma. Proceedings of the EAU Annual Congress; Barcelona. EAU Guidelines Office, Arnhem (The Netherlands); 2019.
- Escudier B, Grünwald V, Gillessen S, et al. Renal cell carcinoma: ESMO Clinical Practice Guidelines for diagnosis, treatment and follow-up. Ann Oncol. 2019;30:706–720.
- Vickers AJ. Prediction models: revolutionary in principle, but do they do more good than harm? J Clin Oncol. 2011;29:2951–2952.
- Motzer R, Tannir N, McDermott D, et al. Nivolumab plus ipilimumab versus sunitinib in advanced renal-cell carcinoma. N Engl J Med. 2018;378:1277–1290.
- Rini B, Plimack E, Stus V, et al. Pembrolizumab plus axitinib versus sunitinib for advanced renal-cell carcinoma. N Engl J Med. 2019;380:1116.