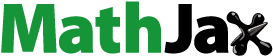
Abstract
Background
Manual volumetric modulated arc therapy (VMAT) treatment planning for high-risk prostate cancer receiving whole pelvic radiotherapy (WPRT) with four integrated dose levels is complex and time consuming. We have investigated if the radiotherapy planning process and plan quality can be improved using a well-tuned model developed through a commercial system for knowledge-based planning (KBP).
Material and methods
Treatment plans from 69 patients treated for high-risk prostate cancer with manually planned VMAT were used to develop an initial KBP model (RapidPlan, RP). Prescribed doses were 50, 60, 67.5, and 72.5 Gy in 25 fractions to the pelvic lymph nodes, prostate and seminal vesicles, prostate gland, and prostate tumour(s), respectively. This RP model was in clinical use from July 2019 to February 2020, producing another set of 69 clinically delivered treatment plans for a new patient group, which were used to develop a second RP model. Both models were validated on an independent group of 40 patients. Plan quality was compared by D98% and the Paddick conformity index for targets, mean dose (Dmean) and generalised equivalent uniform dose (gEUD) for bladder, bowel bag and rectum, and number of monitor units (MU).
Results
Target coverage and conformity was similar between manually created and RP treatment plans. Compared to the manually created treatment plans, the final RP model reduced average Dmean and gEUD with 2.7 Gy and 1.3 Gy for bladder, 1.2 Gy and 0.9 Gy for bowel bag, and 2.7 Gy and 0.8 Gy for rectum, respectively (p < .05). For rectum, the interpatient variation (i.e., 95% confidence interval) of DVHs was reduced by 23%.
Conclusion
KBP improved plan quality and consistency among treatment plans for high-risk prostate cancer. Model tuning using KBP-based clinical plans further improved model outcome.
Introduction
Prostate cancer is the cancer with the highest incidence in Norwegian men [Citation1]. Radiotherapy is one of the standard curative therapeutic options for prostate cancer. Development in radiation treatment techniques has made dose escalation to the prostate gland possible, increasing the probability of tumour control without increasing doses to the organs at risk (OARs) [Citation2–5].
Manual dose planning of volumetric modulated arc therapy (VMAT) treatments with multiple overlapping planning target volumes (PTVs) with distinct prescribed doses normally requires a number of auxiliary structures and many objectives to be balanced in the optimisation. Multiple iterations of plan optimisation are often necessary and time consuming, and variability in plan quality is known to be associated with the experience of the planner [Citation6,Citation7]. Knowledge-based planning (KBP), utilising a library of clinical treatment plans to estimate the best achievable dose distribution for new patients, can offer efficient and consistent plan generation for several treatment sites and fractionation regimens [Citation8–12].
The quality of the KBP model output relies on the mean quality of the training set, hence an optimal procedure for selection of high-quality treatment plans and optimal tuning of the model is essential and under investigation [Citation9–15]. The commercial KBP software RapidPlan(RP, Varian Medical Systems, Inc., Palo Alto, CA, USA) complements the optimisation template with the possibility of line objectives for OARs generated from the dose–volume histogram (DVH) estimated by the RP model. These objectives are located under the lower bound of the DVH estimate range and can simplify the manually set (often by trial and error) optimisation template.
Our radiation therapy clinic has a long tradition of dose-escalation and whole pelvic radiation therapy (WPRT) [Citation5,Citation16,Citation17]. More than 40% of our patients treated for prostate cancer receive WPRT with simultaneous integrated boost (SIB), requiring complex treatment plans with four target dose levels. KBP has previously been used in treatment of WPRT of prostate cancer, but limited to two target dose levels [Citation14,Citation18–20]. In the current study, we investigate the effect of using KBP clinically for plans with an increased plan complexity where different dose levels are administered simultaneously to the prostate tumour, prostate gland, seminal vesicles, and elective lymph nodes.
A potential for model improvement by repeated model building using the same patient group has previously been shown by Fogliata et al. [Citation15]. In the current study, we investigate if a similar effect of model improvement could be obtained simply by using clinical plans (CPs) created with the first model version. This method would be intriguing as it could be performed along with clinical practice without much extra workload.
Material and methods
Patient material
This quality improvement study used image and dose data from 159 patients with high-risk prostate cancer treated with WPRT with SIB at Haukeland University Hospital (HUH), Bergen, Norway, in the period 2018–2020. The Data Protection Official at HUH has been informed about the project, and procedures for data handling are in line with the General Data Protection Regulation (GDPR, article 89 (1)) and according to department routines for quality improvement projects.
An initial KBP model was trained using 70 (later limited to 69) patients treated between January 2018 and June 2019 with manually created plans (RPm_v1). An updated KBP model (RPm_v2) was trained using 69 clinical treatment plans created between July 2019 and February 2020 with RPm_v1 as a planning tool. A separate group of 40 patients, treated with manually created plans in the first period, was used for validation of both models.
Definition of high-risk prostate cancer was clinical stage ≥ T3a, Gleason score 8–10 or s-prostate-specific antigen (s-PSA) > 20 ng/mL. All patients were treated according to our standard clinical procedure with prescribed CTV doses of 50, 60, 67.5, and 72.5 Gy in 25 fractions to the pelvic lymph nodes, prostate and seminal vesicles, prostate gland, and prostate tumour(s), respectively. Patients with pathologically enlarged regional lymph nodes at the time of diagnosis or pelvic bone oligometastases received an extra SIB up to 60 Gy in these local areas, in addition to the standard prescribed doses.
Clinical practice
All patients had a planning CT scan acquired with knee and ankle fixation in supine position on a Phillips Big Bore CT scanner (Philips Healthcare, Cleveland, Highland Heights, OH, USA), with 2 mm slice thickness and intravesical contrast (density replaced by HU = 0 during treatment planning). Diagnostic multiparametric MRI images (acquired at HUH or the patient’s local hospital) were analysed by an experienced radiologist, and used in combination with biopsies and examination findings to guide the oncologists when defining the active cancer subject to the individual boost volumes. The radiation treatment was executed with daily image guidance, matched with respect to three fiducial gold markers in the prostate.
Our protocol involved five CTVs: CTV_72.5 defined the MRI guided boost volume in the prostate gland, CTV_67.5 included the CTV_72.5 volume and the prostate gland and tumour extensions outside the prostate gland, CTV_60 included CTV_67.5 and the proximal 2 cm of the seminal vesicles (all of the seminal vesicles were included in T3b and T4 tumours), in addition to pathologically enlarged or suspicious lymph nodes or oligometastases, CTV_prost_50 included CTV_60 and the seminal vesicles, and CTVe_pelvic_50 included the pelvic lymph nodes. The PTV_72.5 was identical to the CTV_72.5, while PTV_67.5, PTV_60 and a combined PTV_50 volume were generated adding an isotropic margin of 2, 5, 7, and 10 mm to CTV_67.5, CTV_60, CTV_prost_50, and CTVe_pelvic_50, respectively. The considered OARs were bladder, bowel bag (defined as the intestinal cavity with L4 as cranial limit) and rectum (sigmoid as cranial limit), contoured according to the RTOG guidelines [Citation21]. A description of the auxiliary structures and general limitation of dose to normal tissue can be found in the appendix.
All treatments were delivered as 6 MV VMAT in two full arcs using a CLINAC-IX 2300 with the Millennium 120 MLC system (Varian Medical Systems, Inc., Palo Alto, CA, USA). The position of the isocenter was normally at the centre of mass of the PTV_50. For long target volumes, it was adjusted so the central MLC region with thin leaves covered the complex caudal part of the target. To maintain the degree of freedom for the MLC, the field was limited to 16 cm in x direction. All plans had a standard collimator inclination angle of 20° and restricted plan complexity by an objective of maximum 700 monitor units (MU), and were normalised to the PTV_72.5 median dose. All plans were optimised with the Photon Optimiser and the dose was calculated with the Anisotropic Analytical Algorithm. The plans included in the first model had been created in Eclipse version 13.6 (41/69 plans) or 15.6 (28/69 plans) (Varian Medical Systems, Inc., Palo Alto, CA, USA). For comparison reasons, the manually optimised CPs created with version 13.6 in the validation set (13/40 plans) were reoptimised to version 15.6. All RP models and treatment plans were created in Eclipse version 15.6. A thorough explanation of RPs functionality is given elsewhere [Citation22].
RP model creation
The following steps were performed during the model creation:
Structures with optimisation objectives were added to the model.
Eligible patients were identified, and their treatment plans included.
The model was trained and verified by inspecting all potential outliers identified through the available statistics in the Model Configuration workspace and the Model Analytics platform (Aria version 15.6, Varian Medical Systems, Inc., Palo Alto, CA, USA). If delineation followed the guidelines and the optimisation objectives and dose distribution appeared ideal, the plan and structure were kept in the model.
The coefficient of determination (R2) and the average chi square (χ2) are metrics from the goodness of fit statistics in the model training log, offering a quantified measure of the model quality (details in Appendix). In combination, R2 and χ2 can indicate whether the model is overfitting data, reducing the estimation capability.
Creation of the first RP model
A total of 70 plans were added to the first RP model (RPm_v1), but one plan was removed during the verification process as the plan quality was considered suboptimal. In addition, the dose distribution for the bladder in one plan was considered suboptimal and the match to the model was removed.
Creation of the second RP model
Between July 2019 and February 2020, the RPm_v1 model was the standard planning tool for this patient group in our clinic. The plan generated by RPm_v1 was used as a starting point in the optimisation; kept unchanged if optimal, and manually adjusted by experienced planners if room for improvement was recognised. Establishing a second RP model (RPm_v2), the first 69 clinical treatment plans created using RPm_v1 were included. No plans or structures were removed during model verification.
Validation
A small selection (< 10) of patients in each model was used for testing and tuning the objectives and priorities (closed-loop). An independent patient group, consisting of 40 patients with manually created clinical treatment plans not included in the models, was used for open-loop validation of both models. For each of these patients, we thus had three treatment plans: a CP created manually, a plan created with RPm_v1 (RP_v1) and a plan created with RPm_v2 (RP_v2). Dosimetric endpoints for target volumes were D98% and the Paddick conformity index [Citation23]. For OARs, we compared mean dose (Dmean) and generalised equivalent uniform dose (gEUD):
where
denotes the ith bin of the differential DVH, and a is the volume–effect parameter [Citation24,Citation25]. The value of a was set to 8 for bladder, 4 for bowel bag, and 12 for rectum [Citation26–28]. For the OARs, we also calculated population averaged DVHs with confidence limits. The degree of DVH variance reduction with RP relative to manually created treatment plans was found by comparing the area encompassed by the 95% confidence interval (CI) around the average DVH. In addition, the number of MU was compared.
Statistical analysis
All OAR dose–volume parameters were normally distributed (Shapiro–Wilk), with the exception of gEUD for bowel bag. Normally distributed parameters were tested for significance with the paired samples t-test (IBM SPSS Statistics, IBM Corp., Armonk, NY, USA), where a p-value of < .05 was considered statistically significant. The non-normal distributions, i.e., gEUD for bowel bag and data on MU, were tested for significance by the related-samples Wilcoxon’s signed rank test.
Results
The setup of model structures and objectives is presented in and was identical for both RP models. Altogether 14 target objectives, including objectives for three auxiliary structures ensuring dose homogeneity in the non-overlapping parts of the target volumes, and three OAR line objectives were utilised. Goodness of fit statistics were closer to unity in RPm_v2 than in RPm_v1 (Table A1).
Table 1. Optimisation template.
An example of the dose distribution obtained with RPm_v2 compared to manual planning is shown in . Target coverage was similar between manually and model created treatments, with D98% > 95% of prescribed dose for all targets in all plans () and comparable conformity indexes (Fig. A1). Conformity was high with average index above 0.76 for PTV_50, PTV_60 and PTV_67.5, but low (average conformity index < 0.44) in PTV_72.5 as expected due to the 95% (68.9 Gy) isodose extending outside the volume caused by the accepted dose range (95–107%, i.e., 64.1–72.2 Gy) of the enclosing PTV_67.5 volume.
Figure 1. Dose distributions obtained with manual planning (CP; a, c) and the final version of the RapidPlan model (RP_v2; b, d) for the same validation patient. Dose range from 45 Gy (blue) to 73 Gy (red). PTVs are contoured in red, rectum in green, bladder in yellow, and bowel bag in magenta.
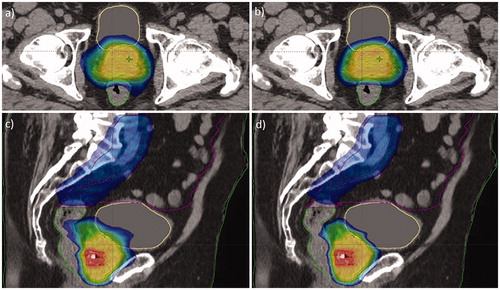
Figure 2. Population averaged DVH for all OARs and PTVs. Solid, dashed dotted, and dashed lines are used for manual , RP_v1, and RP_v2, respectively.
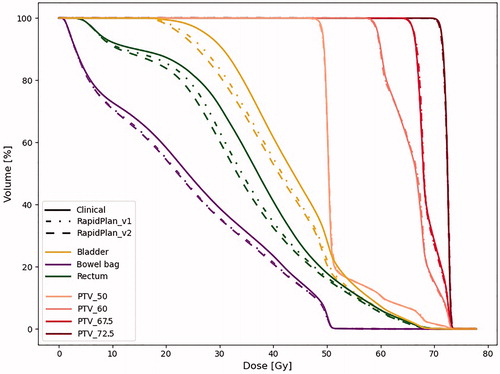
For all OARs, with the exception of gEUD for bowel bag, average Dmean and gEUD were significantly reduced when moving from manual planning to RPm_v1, and then further reduced using RPm_v2 (). For seven out of the 40 patients the reduction in Dmean with RPm_v2 compared to manual planning was larger than 3 Gy for both rectum and bladder, with maximum reductions of more than 6 Gy for both OARs. For rectum, the largest benefit was seen for doses below 50 Gy (). This was reflected in a larger gain in Dmean compared to gEUD, which puts more weight on higher dose levels (). Also for bowel and bladder the largest gain was seen for low and intermediate dose levels ().
Table 2. OAR dose–volume parameters (Dmean and gEUD) obtained in the validation group with manually created clinical plans (CP) and RapidPlan created plans (version 1 and version 2).
Substantial interpatient variation in the DVHs for the OARs was seen in the manually planned CPs, reflecting differences between treatment planning experts and patient geometries (, Fig. A2). With RPm_v2 this interpatient DVH variation (measured as the 95% CI area) was reduced by 23% for the rectum compared to manual planning, while it remained similar for the bladder and bowel.
Figure 3. Population averaged DVHs with 95% CI for bladder (a, b) and rectum (c, d). Solid lines are used for manually planned CP, dashed dotted lines for RP_v1, and dashed lines for RP_v2.
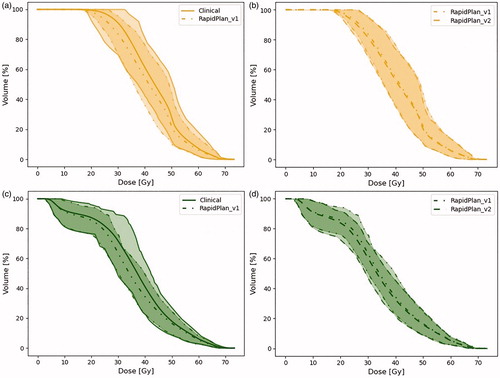
The number of MU was similar for all techniques (median number of MU; 702, 701, and 703 for CP, RP_v1, and RP_v2, respectively). The number of auxiliary structures regarding PTVs and OARs was reduced from seven when planning manually to three using RP. The three auxiliary structures concerning normal tissue sparing were redundant due to the change in protocol from automatic normal tissue objective (NTO) to manual NTO, set by default in the RP models.
Estimated planning time with RP in our clinic was 55 min; one round of VMAT optimisation without interaction took 30 min, and the remaining 25 min included preparations for planning and evaluation of the treatment plan. Planning time for the manually planned CPs was not recorded, but manual planning usually involved 2–3 rounds of repeated optimisation and evaluation which translates to an overall longer planning time compared to RP.
Discussion
In the current study, we have implemented and refined a KBP model for WPRT of high-risk prostate cancer with four integrated target dose levels. Despite the higher model complexity in our study than in previous studies due to the number of dose levels, improved quality with RP compared to the manually created plans was achieved, in accordance with other studies on KBP [Citation14,Citation18–20]. The largest gain in OAR sparing was obtained when moving from manually created CPs to the initial RP model (RPm_v1); however, a smaller but significant further improvement from RP_v1 was seen for rectum and bladder with the refined KBP model (RPm_v2). In individual patients, a substantial and potentially clinically relevant reduction in Dmean of several Gy was observed for both bladder and rectum using RPm_v2 compared to manual planning. Furthermore, RP planning showed more interpatient consistency in rectum DVHs than manual planning.
OAR dose sparing with RP was mostly in the intermediate and low dose regions, and it is therefore uncertain whether the observed change will lead to overall reduced side effects [Citation29–31]. The bladder neck and prostatic urethra are included in the target volume; therefore, doses to these parts remain the same with RP compared to manual planning. Especially, the prostatic urethra receives high doses with our current practice if close to the 2.9 Gy/fraction boost volume and is therefore more likely to cause genitourinary side effects than bladder intermediate doses [Citation31]. For rectum, the dose–response relationship for intermediate doses is unclear, but a positive effect of intermediate dose reduction on the risk of, e.g., stool frequency cannot be excluded [Citation32].
All OAR optimisation objectives and priorities in the models in this study solely consist of values generated by the RP model, with the intention of not limiting the model by using fixed values. Notably, the OAR sparing achieved with RP was not at the expense of target conformity, which we would expect if the optimisation algorithm was stressed to its limits. In other words, the derived solutions were feasible and improved our clinical results, but we cannot know if the final RP model produced the optimal solution for these patients or if further improvements would be achievable through adjusted optimisation criteria.
Several studies have investigated the training and refinement of RP models; Hussein et al. performed systematic refinement with several iterations of model parameter adjustments [Citation10], Li et al. filtered treatment plans based on optimal OAR sparing and identified the best objective priority set after a comprehensive analysis of a selection of possible priorities [Citation13], and Chatterjee et al. investigated a tiered approach where an intermediate model, consisting of the plans with the best spared OARs of the original model, was used as basis to replan all original plans for a final model [Citation11]. Our approach when creating the first RP model was standard, as earlier described in literature [Citation22], while the further improvement has been rather pragmatic, though highly feasible in combination with clinical practice, demanding a minimum of additional planning effort and no extra workload for the oncologists. Creating a new RP model with clinically accepted plans (either created by a preliminary RP model alone or with additional final manual adjustments), the inspection of treatment plans in the training set is performed thoroughly as a part of the clinical practice, where the RP optimisation workspace and estimated DVHs facilitate the detection of both dose and contouring outliers.
Populating a second model with a group of reoptimised treatment plans used to train the initial model will probably improve the model quality, but not necessarily improve the clinical plan quality, as experienced by Fogliata et al. and Lin et al. [Citation15,Citation33]. In our case, not only the expected DVH area (estimated by the model before optimisation) was tightened, but also the CI of the mean DVHs for the actual clinical treatment plans.
A limitation of our study is that it only considers bladder, bowel bag, and rectum as relevant OARs with dose limiting criteria, as this is in line with our clinical practice. Based on experience from our treatment planning traditions, dose to the penile bulb and femoral heads seldom reaches our clinic’s complication threshold (based on QUANTEC [Citation29]) and these structures are therefore not delineated by default. However, further automation of the generation of planning objectives and priorities could, over a period of time, alter the clinic’s ‘normal’ dose distribution, provoking the need for contouring, monitoring, and possibly adding planning objectives to additional OARs. In addition, a recent study by Murray et al. [Citation34] indicates a lower tolerance of dose to the penile bulb than previously estimated, suggesting that it should be considered during optimisation.
A weakness of our approach on model improvement is that new models might contain treatment plans from older software versions, as the models depend on previous work in the clinic. In that concern, all version 13.6 plans in RPm_v1 were reoptimised in retrospect with version 15.6 to evaluate the effect of Eclipse version. The reoptimisation did not result in a significant change, with the exception of a decrease of 0.2 Gy in average Dmean and gEUD for rectum and an increase in average gEUD of 0.2 Gy for bladder, respectively (data not shown).
Another factor that could impact the model improvement is the different patient sets utilised when building the models. In our case, a large group consisting of 69 high risk prostate cancer patients was used in each model to minimise this factor, where all patients had the same prescribed doses, followed the same drinking regime and were contoured following the same guidelines. However, with RPm_v1 as planning tool, the importance of focus on delineation guidelines was more noticeable. Small deviations in OAR contouring (possibly accepted in the first model) could more easily be recognised and corrected early in the process due to the statistics from RapidPlan, conceivably leading to more consistent plan quality. Though these improvements were experienced in the clinic, the difference when comparing the descriptive statistics in the RapidPlan Model Configuration was not prominent, suggesting a minor impact on the model improvement.
Varian recommends a minimum of 20–25 treatment plans in the training set for very specific cases, while generic models should contain a set of 50–100 plans. A possible next step could be to optimise the patients in RPm_v1 with RPm_v2, and then combine the reoptimised plans with the plans in RPm_v2 in a new model. In that case, we anticipate the dosimetric change to be of a smaller impact than the change from RPm_v1 to RPm_v2. However, a potential advantage could be improved handling of outlier patients.
In conclusion, KBP planning was highly feasible and improved both plan quality and consistency for WPRT of high-risk prostate cancer with four integrated target dose levels. Model tuning using KBP-based clinical treatment plans refined the model with modest but significant improvements. KBP has made the treatment planning process more efficient than manual planning and is routinely used in our clinic.
Supplemental Material
Download MS Word (148 KB)Acknowledgments
The authors want to thank Sara Pilskog (https://orcid.org/0000-0002-3475-7939) for fruitful discussions and guidance.
Disclosure statement
The authors report no conflicts of interest.
Additional information
Funding
References
- Cancer Registry of Norway. Cancer in Norway 2018 – cancer incidence, mortality, survival and prevalence in Norway. Oslo: Cancer Registry of Norway; 2019.
- Bauman G, Haider M, Van der Heide UA, et al. Boosting imaging defined dominant prostatic tumors: a systematic review. Radiother Oncol. 2013;107(3):274–281.
- Spratt DE, Pei X, Yamada J, et al. Long-term survival and toxicity in patients treated with high-dose intensity modulated radiation therapy for localized prostate cancer. Int J Radiat Oncol Biol Phys. 2013;85(3):686–692.
- Dearnaley DP, Jovic G, Syndikus I, et al. Escalated-dose versus control-dose conformal radiotherapy for prostate cancer: long-term results from the MRC RT01 randomised controlled trial. Lancet Oncol. 2014;15(4):464–473.
- Ekanger C, Helle SI, Heinrich D, et al. Ten-year results from a phase II study on image guided, intensity modulated radiation therapy with simultaneous integrated boost in high-risk prostate cancer. Adv Radiat Oncol. 2019;5(3):396–403.
- Das IJ, Cheng C-W, Chopra KL, et al. Intensity-modulated radiation therapy dose prescription, recording, and delivery: patterns of variability among institutions and treatment planning systems. J Natl Cancer Inst. 2008;100(5):300–307.
- Nelms BE, Robinson G, Markham J, et al. Variation in external beam treatment plan quality: an inter-institutional study of planners and planning systems. Pract Radiat Oncol. 2012;2(4):296–305.
- Fogliata A, Wang P-M, Belosi F, et al. Assessment of a model based optimization engine for volumetric modulated arc therapy for patients with advanced hepatocellular cancer. Radiat Oncol. 2014;9:236.
- Tol JP, Delaney AR, Dahele M, et al. Evaluation of a knowledge-based planning solution for head and neck cancer. Int J Radiat Oncol Biol Phys. 2015;91(3):612–620.
- Hussein M, South CP, Barry MA, et al. Clinical validation and benchmarking of knowledge-based IMRT and VMAT treatment planning in pelvic anatomy. Radiother Oncol. 2016;120(3):473–479.
- Chatterjee A, Serban M, Abdulkarim B, et al. Performance of knowledge-based radiation therapy planning for the glioblastoma disease site. Int J Radiat Oncol Biol Phys. 2017;99(4):1021–1028.
- Castriconi R, Fiorino C, Passoni P, et al. Knowledge-based automatic optimization of adaptive early-regression-guided VMAT for rectal cancer. Phys Med. 2020;70:58–64.
- Li N, Carmona R, Sirak I, et al. Highly efficient training, refinement, and validation of a knowledge-based planning quality-control system for radiation therapy clinical trials. Int J Radiat Oncol Biol Phys. 2017;97(1):164–172.
- Castriconi R, Fiorino C, Broggi S, et al. Comprehensive intra-institution stepping validation of knowledge-based models for automatic plan optimization. Phys Med. 2019;57:231–237.
- Fogliata A, Cozzi L, Reggiori G, et al. RapidPlan knowledge based planning: iterative learning process and model ability to steer planning strategies. Radiat Oncol. 2019;14(1):187.
- Muren LP, Wasbø E, Helle SI, et al. Intensity-modulated radiotherapy of pelvic lymph nodes in locally advanced prostate cancer: planning procedures and early experiences. Int J Radiat Oncol Biol Phys. 2008;71(4):1034–1041.
- Karlsdottir Á, Muren LP, Wentzel-Larsen T, et al. Outcome in intermediate or high risk prostate cancer patients receiving radiation dose and hormone therapy. Acta Oncol. 2009;48(6):874–881.
- Schubert C, Waletzko O, Weiss C, et al. Intercenter validation of a knowledge based model for automated planning of volumetric modulated arc therapy for prostate cancer. The experience of the German RapidPlan Consortium. PLoS One. 2017;12(5):e0178034.
- Bossart E, Duffy M, Simpson G, et al. Assessment of specific versus combined purpose knowledge based models in prostate radiotherapy. J Appl Clin Med Phys. 2018;19(6):209–216.
- Chatterjee A, Serban M, Faria S, et al. Novel knowledge-based treatment planning model for hypofractionated radiotherapy of prostate cancer patients. Phys Med. 2020;69:36–43.
- Gay HA, Barthold HJ, O'Meara E, et al. Pelvic normal tissue contouring guidelines for radiation therapy: a Radiation Therapy Oncology Group Consensus Panel Atlas. Int J Radiat Oncol Biol Phys. 2012;83(3):e353–e362.
- Fogliata A, Belosi F, Clivio A, et al. On the pre-clinical validation of a commercial model-based optimisation engine: application to volumetric modulated arc therapy for patients with lung or prostate cancer. Radiother Oncol. 2014;113(3):385–391.
- Paddick I. A simple scoring ratio to index the conformity of radiosurgical treatment plans. J Neurosurg. 2000;93(Suppl. 3):219–222.
- Niemierko A. Reporting and analyzing dose distributions: a concept of equivalent uniform dose. Med Phys. 1997;24(1):103–110.
- Niemierko A. A generalized concept of equivalent uniform dose (EUD). Med Phys. 1999;26:1100.
- Roeske JC, Bonta D, Mell LK, et al. A dosimetric analysis of acute gastrointestinal toxicity in women receiving intensity-modulated whole-pelvic radiation therapy. Radiother Oncol. 2003;69(2):201–207.
- Söhn M, Yan D, Liang J, et al. Incidence of late rectal bleeding in high-dose conformal radiotherapy of prostate cancer using equivalent uniform dose-based and dose–volume-based normal tissue complication probability models. Int J Radiat Oncol Biol Phys. 2007;67(4):1066–1073.
- Allen Li X, Alber M, Deasy JO, et al. The use and QA of biologically related models for treatment planning: short report of the TG-166 of the therapy physics committee of the AAPM: TG-166 report. Med Phys. 2012;39(3):1386–1409.
- Marks LB, Yorke ED, Jackson A, et al. Use of normal tissue complication probability models in the clinic. Int J Radiat Oncol Biol Phys. 2010;76(3 Suppl.):S10–S19.
- Michalski JM, Gay H, Jackson A, et al. Radiation dose–volume effects in radiation-induced rectal injury. Int J Radiat Oncol Biol Phys. 2010;76(3 Suppl.):S123–S129.
- Viswanathan AN, Yorke ED, Marks LB, et al. Radiation dose–volume effects of the urinary bladder. Int J Radiat Oncol Biol Phys. 2010;76(3 Suppl.):S116–S122.
- Schaake W, van der Schaaf A, van Dijk LV, et al. Normal tissue complication probability (NTCP) models for late rectal bleeding, stool frequency and fecal incontinence after radiotherapy in prostate cancer patients. Radiother Oncol. 2016;119(3):381–387.
- Lin YH, Hong LX, Hunt MA, et al. Use of a constrained hierarchical optimization dataset enhances knowledge-based planning as a quality assurance tool for prostate bed irradiation. Med Phys. 2018;45(10):4364–4369.
- Murray J, Gulliford S, Griffin C, et al. Evaluation of erectile potency and radiation dose to the penile bulb using image guided radiotherapy in the CHHiP trial. Clin Transl Radiat Oncol. 2020;21:77–84.