Abstract
Purpose
We tested the hypothesis that gene expressions from biopsies of locally advanced head and neck squamous cell carcinoma (HNSCC) patients can supplement dose-volume parameters to predict dysphagia and xerostomia following primary radiochemotherapy (RCTx).
Material and methods
A panel of 178 genes previously related to radiochemosensitivity of HNSCC was considered for nanoString analysis based on tumour biopsies of 90 patients with locally advanced HNSCC treated by primary RCTx. Dose-volume parameters were extracted from the parotid, submandibular glands, oral cavity, larynx, buccal mucosa, and lips. Normal tissue complication probability (NTCP) models were developed for acute, late, and for the improvement of xerostomia grade ≥2 and dysphagia grade ≥3 using a cross-validation-based least absolute shrinkage and selection operator (LASSO) approach combined with stepwise logistic regression for feature selection. The final signatures were included in a logistic regression model with optimism correction. Performance was assessed by the area under the receiver operating characteristic curve (AUC).
Results
NTCP models for acute and late xerostomia and the improvement of dysphagia resulted in optimism-corrected AUC values of 0.84, 0.76, and 0.70, respectively. The minimum dose to the contralateral parotid was selected for both acute and late xerostomia and the minimum dose to the larynx was selected for dysphagia improvement. For the xerostomia endpoints, the following gene expressions were selected: RPA2 (cellular response to DNA damage), TCF3 (salivary gland cells development), GBE1 (glycogen storage and regulation), and MAPK3 (regulation of cellular processes). No gene expression features were selected for the prediction of dysphagia.
Conclusion
This hypothesis-generating study showed the potential of improving NTCP models using gene expression data for HNSCC patients. The presented models require independent validation before potential application in clinical practice.
Introduction
Radiation therapy is an effective treatment for head and neck cancers either as definitive treatment with or without chemotherapy or as an adjuvant treatment after surgical resection. The head and neck region has complex anatomy; thus, critical structures within and adjacent to the tumour may receive substantial radiation doses causing radiation-related side effects. Two of the side effects are xerostomia and dysphagia, resulting from irradiation of some parts of the salivary glands and swallowing musculature [Citation1–3]. These side effects have been toxicity endpoints of interest in several normal tissue complication probability (NTCP) models in the past years, some of which have been externally validated [Citation2,Citation4,Citation5]. In a systematic review of NTCP models for head and neck radiotherapy, none of these NTCP models included gene expressions as candidate features [Citation5]. This is despite the ample evidence of the potential of these biomarkers to predict the radiation therapy outcomes, including toxicities [Citation6,Citation7].
Several reports have observed associations between normal tissue toxicities and oncological outcomes including local control, progression-free survival and overall survival [Citation8–10]. Eade et al. [Citation9] found that the freedom from late biochemical failure rate among prostate cancer patients with acute toxicity was 0.45 times that of patients without acute toxicity. Acute toxicity was also an independent predictor of better disease-free survival among anal cancer patients [Citation10] and better tumour response among head and neck cancer patients [Citation8]. The observed mechanistic interrelationship is probably due to shared intrinsic factors that determine the susceptibility of both normal tissues and cancer cells to anti-cancer agents including radiotherapy and chemotherapy. One of the primary explanations concerns inherited mutations in pathways that deal with the incoming damage, including DNA repair pathways, which determine the ability of both normal and cancer cells to repair radiation damage. Extensive similarities were noted between the expression profiles of normal tissues and tumour cells, while a number of transcripts were expressed at significantly different levels [Citation11]. To date, genetic associations for radiotherapy-related toxicities focus on expressions derived from peripheral blood samples [Citation12–14]. This may only partially explain the normal tissue radiosensitivity as it focuses on systemic gene expressions while ignoring local gene expressions close to the organ of interest.
Therefore, this study aimed to develop NTCP models for xerostomia and dysphagia after primary radiochemotherapy of locally advanced HNSCC including gene expression data. Dose-volume metrics and gene-expression data obtained from tumour biopsies were first considered individually and then combined, in particular, to identify genes with additional prognostic value for future validation.
Material and methods
Patients
In this unicentre, retrospective study, 90 patients with locally advanced HNSCC were included. Patients were treated with primary radiochemotherapy using 3 D conformal radiotherapy between 2006 and 2014 at the Department of Radiotherapy and Radiation Oncology, University Hospital Carl Gustav Carus Dresden. Patients were treated with curative intent and received a median dose prescription of 72 Gy based concurrently with cisplatin or mitomycin-C. 85 patients (94%) received 200 cGy/fraction for 15 fractions and hyperfractionation twice daily with 140 cGy/fraction for 30 fractions and five patients (6%) received normofractionation with 35 fractions. Doses were converted into equivalent doses in 2-Gy fractions (EQD2) assuming α/β = 3 Gy. For simplicity, only one α/β value was considered due to the different evaluated endpoints, including the change from acute to late toxicity (improvement of dysphagia and xerostomia), uncertainties associated with α/β ratios and the likelihood of the selection not severely impacting model performance [Citation15]. The patient characteristics and clinical features are shown in . The considered patients were part of the retrospective HNSCC cohort of the German Cancer Consortium – Radiation Oncology Group (DKTK-ROG) [Citation16] and of a prospective observational trial [Citation17,Citation18]. Inclusion and exclusion criteria were reported previously [Citation16–18]. Briefly, the inclusion criteria were: patients were ≥18 years old, histologically proven locally advanced squamous cell carcinoma and treated curatively with primary radiochemotherapy. Patients were excluded if gene-expression data from nanoString analyses or clinical toxicity data for xerostomia or dysphagia were not available, leaving 90 patients to be analysed in this study.
Table 1. Patient characteristics (N = 90).
NanoString analysis
Haematoxylin and eosin staining was performed on formalin-fixed paraffin-embedded (FFPE) tissue biopsies of the primary tumour specimens, which were taken to histologically confirm the presence of squamous cell carcinoma. RNA extraction was performed under standardised procedures for consecutive gene expression investigations using nanoString elements technology (NanoString Technologies, Seattle, WA) as described in [Citation19,Citation20]. Expression levels of 178 genes were evaluated. These genes were selected based on hypotheses presented in the literature, relating these genes to sensitivity or resistance to radio(chemo)therapy [Citation21]. Raw counts were logarithmised and then normalised to the mean of the internal level of reference genes ACTR3, NDFIP1, RPL37A, B2M, GNB2L1, RPL11, POLR2A. Gene-expression features were removed if their median expression was below twice the median negative control. The gene expressions selected for extraction were based on hypotheses described in the literature including genes which have been reported to be linked with sensitivity or resistance to radiochemotherapy [Citation21].
Dose-volume metrics
Head and neck structures, including parotid and submandibular glands, oral cavity, buccal mucosa, lips and larynx, were delineated by KL, TM, NY and supervised by ET according to a consensus guideline [Citation22]. Available delineation performed during treatment planning was checked for consistency and modified if required. Considered dose-volume metrics were the minimal dose received by i% of the structure volume with the highest dose, Di% (D1%, D2%, D5%, D10%, D15%… D95%, D98%, D99%), the relative volume of tissue receiving more than i Gy dose, ViGy (V5Gy, V10Gy… V75Gy), mean, minimum and maximum dose (Dmean, Dmin, Dmax). For organs at risk that could not be confidently delineated due to damage resulting from the tumour, missing dose-volume metrics were imputed by assuming that the structures were homogenously irradiated to 95% of the prescription dose, i.e., received a high dose. Dose features with near-zero variance were removed from the analysis individually for every structure. If features were correlated with Spearman rho >0.80, the variable with the lowest mean absolute correlation to all other dose-volume metrics was kept, while the others were removed. The list of parameters remaining after the removal of correlated variables can be found in Supplement A.
Clinical endpoints
The endpoints were xerostomia grade ≥2 and dysphagia grade ≥3 based on the Common Terminology Criteria for Adverse Events (CTCAE) scoring system (version 4.0). Follow-up was scheduled at the end of radiotherapy, at 1, 3, 6, 9, and 12 months from the end of radiotherapy and between one to four times per year thereafter. Acute toxicity was defined as toxicities occurring from the end of treatment until the 3-month follow-up, while toxicities after this time point were categorised as late. The maximum toxicity grade in the corresponding time interval was used. Bias with respect to the available follow-up visits was not visible for late toxicities but may be relevant for dysphagia improvement, see Supplements B and C. Patients with baseline symptoms, xerostomia grade ≥2 for xerostomia endpoints and dysphagia grade ≥3 for dysphagia endpoints, were excluded for the respective endpoint. Patients with missing baseline symptoms who experienced no toxicity at the end of treatment were considered to have no baseline symptoms based on the assumption that symptoms are unlikely to improve during treatment. Xerostomia and dysphagia improvements were defined as a reduction of at least one grade from the maximum grade ≥2 and grade ≥3, respectively.
Model development
Model development for xerostomia and dysphagia based on dose-volume and gene features consisted of three main steps (): preprocessing, feature selection and performance estimation, and final model development with optimism-adjusted bootstrap analysis.
Figure 1. Steps for dose, gene, and dose-gene model development. (1) Hyperparameter tuning using 5-fold cross-validation repeated twenty times, where model parameters for the LASSO were optimised. (2) Feature selection was performed by ranking the features based on the estimated coefficient (LASSO) and frequency (stepwise). Highly ranked features were selected for the final model. (3) Final optimism-adjusted dose-gene model development utilising the features selected in step 2).
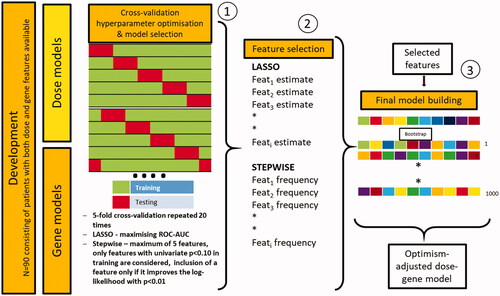
In the first step, the features were preprocessed. Yeo-Johnson- and z-transformation were applied on all continuous features, first to make the data more normal distribution-like and second to transform the mean and SD of the variables to 0 and 1, respectively, which is more favourable in the subsequent modelling steps.
In the second step, feature selection and model performance estimation for the dose and gene features was performed. Two modelling algorithms, the least absolute shrinkage and selection operator (LASSO) and stepwise logistic regression, were executed and compared to ensure a stable selection of features. The LASSO approach is a regularised form of logistic regression with added shrinkage regularisation, acting as a feature selection method. It is suitable when many features are available in the dataset that can be highly correlated [Citation23,Citation24]. Stepwise forward regression is a well-known method in statistics, which comprises starting with no features in the model, testing the addition of each feature using a model fit criterion, adding more features whose inclusion provides the most statistically significant improvement of the fit, and repeating this process until no further significant improvement is observed. Stepwise logistic regression also offers a simple final model, which can be easily interpreted. A repeated (20 times), nested 5-fold cross-validation was applied to tune (inner cross-validation) and to evaluate (outer cross-validation) the models. The tuning of hyperparameters for the LASSO model (lambda) was performed on the inner cross-validation folds of the respective development set using a grid size of 1 × 100, maximising the area under the receiver operating characteristic’s curve (AUC). Internal validation was performed using the outer fold data within the development set producing 100 AUCs for each model. The same repeated nested cross-validation was also performed using stepwise regression. Here, only features with a p-value of less than 0.1 in the training fold were considered. Additional features were included if they improved the log-likelihood with p < 0.01 and the maximum number of features was set to 5 in order to limit overfitting. Features selected in the models using LASSO and stepwise logistic regression were compared. Only features with high importance in both methods, considered to be most reliable, were included in the next step of modelling.
In the third step, one model combining relevant dosimetric and gene information was developed on the whole cohort for every endpoint by optimism-adjusted bootstrap logistic regression using the features selected in the previous step. None of the features was included if models failed to produce a mean cross-validated performance with AUC > 0.5. In this step, original features without transformations were used. The final model estimates and their standard deviation were derived from the means and standard deviations of the bootstrapped models. A nomogram was then constructed on the basis of the multivariate logistic regression model. A statistical analyses protocol (SAP) can be found in Supplement D.
Results
Patient cohort
Ninety patients with locally advanced HNSCC treated by primary radiochemotherapy were included in the analysis (). Dose distribution, gene expressions, and at least one of the endpoints were available for all patients. The median follow-up was 12 months (interquartile range (IQR) = 9–16 months). The majority of patients included were male (83%), had a tumour of UICC grade 4 (84%), T stage of 4 (60%), N stage of 2 (64%) and tumours located in the oral cavity, oropharynx, or hypopharynx (90%).
Toxicity endpoints and clinical correlates
66% and 36% of evaluable patients had grade ≥2 acute and late xerostomia, respectively. The grade ≥3 acute and late dysphagia rates for the evaluable patients were lower; 47% and 28%, respectively. The majority of patients showed at least one grade of improvement for both endpoints; 60% and 62% for xerostomia and dysphagia, respectively. The clinical features tested included age, gender, localisation, grade, UICC stage, T and N classification, and the gross tumour volume (GTV) (Supplements B and C). Age was found to be significantly related to late xerostomia (p = 0.049) and xerostomia improvement (p = 0.023). Patients with tumour grade 3 were found to have a lower probability of xerostomia improvement compared to grade 2 (p = 0.032). A larger GTV was related to a higher probability of late dysphagia (p = 0.020) and to less dysphagia improvement (p = 0.067).
NTCP models based on gene-expression features
From the gene-expression data, the genes RPA2, TCF3, and GNE1 were selected in model development to predict acute xerostomia and MAPK3 was selected for late xerostomia with an AUC of 0.68 and 0.57 for LASSO and 0.61 and 0.53 for stepwise logistic regression, respectively. No gene-expression features were selected for the other endpoints, .
Table 2. The mean value of area under the receiver operating characteristic curve (AUC) from cross-validation and features selected in dose and gene models for xerostomia and dysphagia endpoints using LASSO and stepwise selection.
NTCP models based on dose-volume metrics
The Dmin to the contralateral parotid gland was selected for acute and late xerostomia, and the Dmin to the larynx was selected for improvement in dysphagia. The AUC for acute xerostomia was good (0.72 and 0.73 for LASSO and stepwise regression, respectively). In contrast, the performance for the late xerostomia and dysphagia improvement was limited (both 0.54). No dose-volume metric was selected for the other endpoints (acute and late dysphagia and xerostomia improvement), .
NTCP models combining gene-expression and dose-volume metrics features
Features selected using the cross-validated LASSO and stepwise logistic regression were included in the final multivariable logistic regression models based on the whole cohort. This allows for straightforward model interpretation and application, but external validation is pending. The regression estimates, standard errors and P-values are reported in alongside bootstrap-based optimism-corrected AUC values. Regression curves are shown in and Supplement E. For acute xerostomia, RPA2, TCF3, and GBE1 remained significant after adjusting for the effect of the dose feature Dmin of the contralateral parotid gland, i.e., they significantly improved the model based on dose-volume metrics alone. A higher expression of TCF3 was associated with a higher probability of acute xerostomia (estimate = 1.172, p = 0.023). In contrast, lower expressions of RPA2 (estimate = −1.429, p = 0.025) and GBE1 (estimate = −0.349, p = 0.041) were associated with a higher probability of acute xerostomia. Model performance and p-values were almost unchanged when considering the Dmin of the contralateral parotid gland based on the physical dose distribution or for an α/β value of 10. The only gene expression feature selected for late xerostomia was MAPK3 (estimate = −1.283, p = 0.009), suggesting that a higher expression is associated with a lower probability for this endpoint. Furthermore, a weaker dose effect was found for late xerostomia with an increase of 0.068 per Gy of the Dmin of the contralateral parotid gland (p = 0.048). The optimism-corrected AUC values were 0.842 and 0.761 for acute and late xerostomia, respectively. On the basis of the models developed, nomograms incorporating the significant features were established to predict acute and late xerostomia and dysphagia improvement (Supplement F).
Figure 2. Univariate logistic regression curves based on the selected gene expression features for (A–C) acute and (D) late xerostomia grade ≥2. 95% confidence bands are shaded and black dots represent the actual outcome. Patients were divided into gene-expression quartiles and group averages are shown (squares) with error bars representing the standard error of the mean.
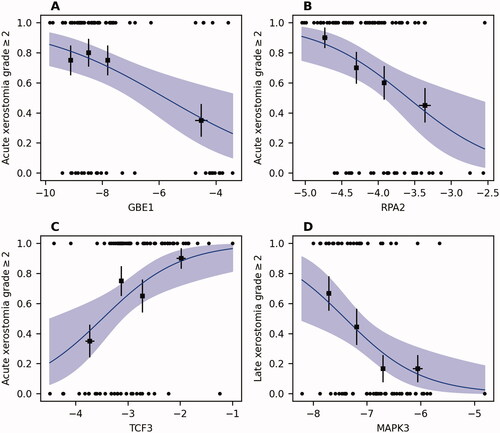
Table 3. Final models for acute and late xerostomia and dysphagia improvement.
Discussion
In this study, we examined the value of gene expression features from squamous cell carcinoma of the head and neck for NTCP modelling of acute, late, and the improvement of dysphagia and xerostomia. We developed predictive models for acute and late xerostomia, including gene expression and dose-volume features with optimism-corrected AUC values of 0.84 and 0.76, respectively. Expressions of the genes RPA2, TCF3, GBE1, and MAPK3 as well as the Dmin to the contralateral parotid gland were included in the models.
For squamous cell head and neck cancers, advances in molecular biology have generated significant amounts of data that have been used to construct risk profiles, which can be used to guide strategies for treatment individualisation to optimise outcomes [Citation25,Citation26]. Although several gene-expression profiling analyses on cancer tissue specimens have been performed to determine differentially expressed genes between patients with and without oncological endpoints like survival and distant metastases [Citation21,Citation27,Citation28], differences in expression profiles between patients with and without toxicity are mostly unknown. While some genes are differentially expressed between head and neck carcinoma and non-cancerous tissues, mechanisms that regulate the normal and cancerous tissues are conceptually similar; an extensive similarity was noted between gene expression profiles [Citation11,Citation29]. Furthermore, the cancer cells can produce both malignant progeny and generate populations of non-cancer cells in a manner similar to the development of differentiated progeny from normal stem cells [Citation29].
The present study analysed the feasibility of gene expressions from HNSCC in combination with dose-volume metrics to predict xerostomia and dysphagia following radiochemotherapy. To the best of our knowledge, this study is the first to assess the connection between gene expressions in biopsy specimens and toxicity outcomes. If successfully validated, these biomarkers may improve the optimisation of cancer treatment by combining both the prediction of oncological and toxicity outcomes. This study identified gene expressions from paraffin-embedded head and neck cancer biopsies to effectively predict acute and late xerostomia. Among these expressions, replication protein A (RPA) 32 kDa subunit (RPA2) was previously shown to have an essential role in DNA replication and the cellular response to DNA damage [Citation30]. Furthermore, the RPA complex controls DNA repair and DNA damage checkpoint activation in response to DNA damage [Citation31,Citation32]. The relevance of RPA2 in sensing DNA damage may explain the higher risk of acute xerostomia among patients with lower expression of RPA2 in the present study. Another selected expression was the transcription factor E2a (TCF3), which plays a major role in cellular development and differentiation and was suggested to be a critical regulatory factor in salivary gland cell development [Citation33,Citation34]. TCF3 enhances the cell-cycle-arrest response, suppresses apoptosis upon p53 activation, and promotes cell migration and wound repair [Citation35,Citation36]. TCF3 also acts as a cell-intrinsic inhibitor of pluripotent cell self-renewal by limiting the self-renewal factors [Citation37]. The final acute xerostomia model predicted that a high expression of TCF3 is related to a higher risk of toxicity, which may relate to its role in the inhibition of self-renewal genes. The expression of mitogen-activated protein kinase 3 (MAPK3), which coordinately regulates various cellular processes including proliferation, differentiation, cell cycle progression, motility, and survival relaying extracellular signals to intracellular responses, was found to be inversely correlated to the risk of late xerostomia [Citation38].
The gene expressions selected for extraction were performed based on hypotheses presented in the literature. Included genes have been reported to be associated with sensitivity or resistance to radiochemotherapy, including genes involved in proliferation, invasion, metastasis, hypoxia-related genes, DNA damage and repair, and genes associated with cisplatin resistance [Citation21]. The process of selecting specific gene expressions may have screened out expressions that may be important for treatment-related toxicities. It should be noted that the gene expressions presented should be interpreted as hypothesis-generating rather than definitive due to the lack of an independent validation cohort.
We considered structures potentially responsible for xerostomia, including ipsi- and contralateral parotid and submandibular glands, buccal mucosa, oral cavity, and lips. We showed that the Dmin to the contralateral parotid gland was the most predictive dose measure for acute and late xerostomia. This feature was strongly correlated to the Dmean (Spearman rho = 0.76), which has been shown in many other studies including in the QUANTEC report to be predictive of xerostomia [Citation39–41]. We could not replicate other studies, which found a strong association of dose to the oral cavity and other structures to the occurrence of xerostomia [Citation42,Citation43]. No dose features were selected as predictors for acute and late dysphagia. This is in contrast to other studies which have found significant associations between dysphagia and doses to the larynx [Citation15,Citation44], oral cavity [Citation45], and other swallowing structures [Citation1]. For dysphagia improvement, the Dmin to the larynx was selected. Interestingly, a higher dose to the larynx was associated with a higher improvement of dysphagia. It can be hypothesised that the improvement of dysphagia is among patients with laryngeal cancers linked to the resolution of side effects of the smooth swallowing muscles. However, the dose to the larynx was not found to be predictive of acute and late dysphagia.
This analysis has some limitations, including those characteristics of a single-centre study with a limited number of patients, in part due to exclusions of patients without baseline toxicity information. Despite the encouraging results, validation against external cohorts is necessary for a solid conclusion and to assess potential bias due to the small number of patients analysed. Additionally, delineations of swallowing muscles were not available, which have been strongly suggested to be important for dysphagia outcomes. This may have significantly reduced the model performance for dysphagia endpoints. For most included patients, the 12-month follow-up was available, while the 18- and 24-month follow-ups were often missing. Hence, the final long-term development of late toxicities may not have been included for some patients. While this is the first study that tests the hypothesis that gene expressions from cancer specimens may be predictive of toxicity outcomes after radio(chemo)therapy of HNSCC, an external validation cohort was not available. Thus, larger prospective studies including dose-volume information and gene expression analyses are required to validate the hypothesis. Results from the prospective HNprädBio trial (NCT02059668) will be available in 2023, which will be analysed to test the hypotheses presented in the present study.
In summary, gene expression profiles from biopsy specimens of locally advanced HNSCC were utilised to predict toxicity outcomes of head and neck cancer patients. The expressions of RPA2, TCF3, GBE1, and MAPK3 supplemented the minimum dose to the ipsilateral parotid gland and the larynx. These results have to be independently validated.
Supplemental Material
Download PDF (610.7 KB)Acknowledgements
NY received a fellowship from the Alexander von Humboldt Foundation for this project.
Disclosure statement
In the past five years, Dr. Krause received funding for her research projects by IBA (2016), Merck KGaA (2014–2018 for preclinical study; 2018–2020 for clinical study), Medipan GmbH (2014–2018). Dr. Krause, Dr. Troost and Dr. Linge are involved in an ongoing publicly funded (German Federal Ministry of Education and Research) project with the companies Medipan, Attomol GmbH, GA Generic Assays GmbH, Gesellschaft für medizinische und wissenschaftliche genetische Analysen, Lipotype GmbH and PolyAn GmbH (2019–2021). For the present manuscript, Dr. Krause, Dr. Troost and Dr. Linge confirm that none of the above-mentioned funding sources were involved.
References
- Charters EK, Bogaardt H, Freeman‐Sanderson AL, et al. Systematic review and meta-analysis of the impact of dosimetry to dysphagia and aspiration related structures. Head Neck. 2019;41(6):1984–1998.
- Brodin NP, Kabarriti R, Garg MK, et al. Systematic review of normal tissue complication models relevant to standard fractionation radiation therapy of the head and neck region published after the QUANTEC reports. Int J Radiat Oncol Biol Phys. 2018;100(2):391–407.
- Duprez F, Madani I, De Potter B, et al. Systematic review of dose-volume correlates for structures related to late swallowing disturbances after radiotherapy for head and neck cancer. Dysphagia. 2013;28(3):337–349.
- Kanayama N, Kierkels RGJ, van der Schaaf A, et al. External validation of a multifactorial normal tissue complication probability model for tube feeding dependence at 6 months after definitive radiotherapy for head and neck cancer. Radiother Oncol. 2018;129(2):403–408.
- Sharabiani M, Clementel E, Andratschke N, et al. Generalizability assessment of head and neck cancer NTCP models based on the TRIPOD criteria. Radiother Oncol. 2020;146:143–150.
- Rosenstein BS. Radiogenomics: identification of genomic predictors for radiation toxicity. Semin Radiat Oncol. 2017;27(4):300–309.
- Kerns SL, Ostrer H, Rosenstein BS. Radiogenomics: using genetics to identify cancer patients at risk for development of adverse effects following radiotherapy. Cancer Discov. 2014;4(2):155–165.
- Ikebe T, Seki K, Nakamura S, et al. Severity of oral mucositis correlates with the response of oral cancer to preoperative radiochemotherapy. Int J Oral Maxillofac Surg. 2005;34(6):642–645.
- Eade T, Choudhury A, Pollack A, et al. Acute epithelial toxicity is prognostic for improved prostate cancer response to radiation therapy: a retrospective, multicenter, cohort study. Int J Radiat Oncol Biol Phys. 2018;101(4):957–963.
- Martin D, Rödel F, von der Grün J, et al. Acute organ toxicity correlates with better clinical outcome after chemoradiotherapy in patients with anal carcinoma. Radiother Oncol. 2020;149:168–173.
- Zhang L, Zhou W, Velculescu VE, et al. Gene expression profiles in normal and cancer cells. Science. 1997;276(5316):1268–1272.
- Ghazali N, Shaw RJ, Rogers SN, et al. Genomic determinants of normal tissue toxicity after radiotherapy for head and neck malignancy: a systematic review. Oral Oncol. 2012;48(11):1090–1100.
- Lyons AJ, West CM, Risk JM, et al. Osteoradionecrosis in head-and-neck cancer has a distinct genotype-dependent cause. Int J Radiat Oncol Biol Phys. 2012;82(4):1479–1484.
- Werbrouck J, De Ruyck K, Duprez F, et al. Acute normal tissue reactions in head-and-Neck cancer patients treated with IMRT: influence of dose and association with genetic polymorphisms in DNA DSB repair genes. Int J Radiat Oncol Biol Phys. 2009;73(4):1187–1195.
- Eisbruch A, Kim HM, Feng FY, et al. Chemo-IMRT of oropharyngeal cancer aiming to reduce dysphagia: swallowing organs late complication probabilities and dosimetric correlates. Int J Radiat Oncol Biol Phys. 2011;81(3):e93–e9.
- Linge A, Lohaus F, Löck S, et al. HPV status, cancer stem cell marker expression, hypoxia gene signatures and tumour volume identify good prognosis subgroups in patients with HNSCC after primary radiochemotherapy: a multicentre retrospective study of the German Cancer Consortium Radiation Oncology Group (DKTK-ROG). Radiother Oncol. 2016;121(3):364–373.
- Löck S, Perrin R, Seidlitz A, et al. Residual tumour hypoxia in head-and-neck cancer patients undergoing primary radiochemotherapy, final results of a prospective trial on repeat FMISO-PET imaging. Radiother Oncol. 2017;124(3):533–540.
- Zips D, Zöphel K, Abolmaali N, et al. Exploratory prospective trial of hypoxia-specific PET imaging during radiochemotherapy in patients with locally advanced head-and-neck cancer. Radiother Oncol. 2012;105(1):21–28.
- Linge A, Löck S, Gudziol V, et al. Low cancer stem cell marker expression and low hypoxia identify good prognosis subgroups in HPV(-) HNSCC after postoperative radiochemotherapy: a multicenter study of the DKTK-ROG. Clin Cancer Res. 2016;22(11):2639–2649.
- Linge A, Löck S, Krenn C, et al. Independent validation of the prognostic value of cancer stem cell marker expression and hypoxia-induced gene expression for patients with locally advanced HNSCC after postoperative radiotherapy. Clin Transl Radiat Oncol. 2016;1:19–26.
- Schmidt S, Linge A, Zwanenburg A, et al. Development and validation of a gene signature for patients with head and neck carcinomas treated by postoperative radio(chemo)therapy. Clin Cancer Res. 2018;24(6):1364–1374.
- Brouwer CL, Steenbakkers RJHM, Bourhis J, et al. CT-based delineation of organs at risk in the head and neck region: DAHANCA, EORTC, GORTEC, HKNPCSG, NCIC CTG, NCRI, NRG oncology and TROG consensus guidelines. Radiother Oncol. 2015;117(1):83–90.
- Deist TM, Dankers FJWM, Valdes G, et al. Machine learning algorithms for outcome prediction in (chemo)radiotherapy: an empirical comparison of classifiers. Med Phys. 2018;45(7):3449–3459.
- Yahya N, Ebert MA, Bulsara M, et al. Statistical-learning strategies generate only modestly performing predictive models for urinary symptoms following external beam radiotherapy of the prostate: a comparison of conventional and machine-learning methods. Med Phys. 2016;43(5):2040–2052.
- Budach V, Tinhofer I. Novel prognostic clinical factors and biomarkers for outcome prediction in head and neck cancer: a systematic review. Lancet Oncol. 2019;20(6):e313–e26.
- Tonella L, Giannoccaro M, Alfieri S, et al. Gene expression signatures for head and neck cancer patient stratification: are results ready for clinical application? Curr Treat Options Oncol. 2017;18(5):32.
- Wang C, Sturgis EM, Chen X, et al. A functional variant at miRNA-122 binding site in IL-1α 3′ UTR predicts risk of recurrence in patients with oropharyngeal cancer. Oncotarget. 2016;7(23):34472–34479.
- She Y, Kong X, Ge Y, et al. Immune-related gene signature for predicting the prognosis of head and neck squamous cell carcinoma. Cancer Cell Int. 2020;20(1):22.
- Shackleton M. Normal stem cells and cancer stem cells: similar and different. Semin Cancer Biol. 2010;20(2):85–92.
- Kirkpatrick DT, Liaw H, Lee D, et al. DNA-PK-Dependent RPA2 hyperphosphorylation facilitates DNA repair and suppresses sister chromatid exchange. PLOS One. 2011;6(6):e21424.
- Dueva R, Iliakis G. Replication protein A: a multifunctional protein with roles in DNA replication, repair and beyond. NAR Cancer. 2020;2(3):zcaa022.
- Zou L, Elledge SJ. Sensing DNA damage through ATRIP recognition of RPA-ssDNA complexes. Science. 2003;300(5625):1542–1548.
- Chin W-C, Park Y-J, Koh J, et al. Identification of regulatory factors for mesenchymal stem Cell-Derived salivary epithelial cells in a Co-Culture system. PLOS One. 2014;9(11):e112158.
- Mona M, Miller R, Li H, et al. MIST1, an inductive signal for salivary amylase in mesenchymal stem cells. IJMS. 2019;20(3):767.
- Andrysik Z, Kim J, Tan AC, et al. A genetic screen identifies TCF3/E2A and TRIAP1 as Pathway-Specific regulators of the cellular response to p53 activation. Cell Rep. 2013;3(5):1346–1354.
- Miao Q, Ku AT, Nishino Y, et al. Tcf3 promotes cell migration and wound repair through regulation of lipocalin 2. Nat Commun. 2014;5:4088.
- Yi F, Pereira L, Merrill BJ. Tcf3 functions as a steady-state limiter of transcriptional programs of mouse embryonic stem cell self-renewal. Stem Cells. 2008;26(8):1951–1960.
- Cargnello M, Roux PP. Activation and function of the MAPKs and their substrates, the MAPK-Activated protein kinases. Microbiol Mol Biol Rev. 2011;75(1):50–83.
- Deasy JO, Moiseenko V, Marks L, et al. Radiotherapy dose–volume effects on salivary gland function. Int J Radiation Oncol Biol Phys. 2010;76(3):S58–S63.
- Owosho AA, Thor M, Oh JH, et al. The role of parotid gland irradiation in the development of severe hyposalivation (xerostomia) after intensity-modulated radiation therapy for head and neck cancer: temporal patterns, risk factors, and testing the QUANTEC guidelines. J Cranio-Maxillofacial Surg. 2017;45(4):595–600.
- Beetz I, Schilstra C, Visink A, et al. Role of minor salivary glands in developing patient-rated xerostomia and sticky saliva during day and night. Radiother Oncol. 2013;109(2):311–316.
- Kaae JK, Johnsen L, Hansen CR, et al. Relationship between patient and physician-rated xerostomia and dose distribution to the oral cavity and salivary glands for head and neck cancer patients after radiotherapy. Acta Oncol. 2019;58(10):1366–1372.
- Cao J, Zhang X, Jiang B, et al. Intensity-modulated proton therapy for oropharyngeal cancer reduces rates of late xerostomia. Radiother Oncol. 2021;160:32–39.
- Pauloski BR, Rademaker AW, Logemann JA, et al. Comparison of swallowing function after intensity-modulated radiation therapy and conventional radiotherapy for head and neck cancer. Head Neck. 2015;37(11):1575–1582.
- Schwartz DL, Hutcheson K, Barringer D, et al. Candidate dosimetric predictors of Long-Term swallowing dysfunction after oropharyngeal intensity-modulated radiotherapy. Int J Radiat Oncol Biol Phys. 2010;78(5):1356–1365.