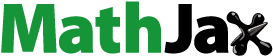
Abstract
Background
Precision cancer medicine (PCM), frequently used for the expensive and often modestly efficacious off-label treatment with medications matched to the tumour genome of end-stage cancer, challenges healthcare resources. We compared the health effects, costs and cost-effectiveness of our MetAction PCM study with corresponding data from comparator populations given best supportive care (BSC) in two external randomised controlled trials.
Methods
We designed three partitioned survival models to evaluate the healthcare costs and quality-adjusted life years (QALYs) as the main outcomes. Cost-effectiveness was calculated as the incremental cost-effectiveness ratio (ICER) of PCM relative to BSC with an annual willingness-to-pay (WTP) threshold of EUR 56,384 (NOK 605,000). One-way and probabilistic sensitivity analyses addressed uncertainty.
Results
We estimated total healthcare costs (relating to next-generation sequencing (NGS) equipment and personnel wages, molecularly matched medications to the patients with an actionable tumour target and follow-up of the responding patients) and the health outcomes for the MetAction patients versus costs (relating to estimated hospital admission) and outcomes for the BSC cases. The ICERs for incremental QALYs were twice or more as high as the WTP threshold and relatively insensitive to cost decrease of the NGS procedures, while reduction of medication prices would contribute significantly towards a cost-effective PCM strategy.
Conclusions
The models suggested that the high ICERs of PCM were driven by costs of the NGS diagnostics and molecularly matched medications, with a likelihood for the strategy to be cost-effective defying WTP constraints. Reducing drug expenses to half the list price would likely result in an ICER at the WTP threshold. This can be an incentive for a public-private partnership for sharing drug costs in PCM, exemplified by ongoing European initiatives.
ClinicalTrials.gov, identifier
NCT02142036
Background
Precision cancer medicine (PCM) is commonly defined as using information encoded by the tumour genome as the dominant factor for the prediction of therapy response, which over the past decade increasingly has been a motivation for the off-label provision of matched medications to patients with advanced cancer after all other systemic tumour-directed therapies have failed. However, it has been an unwelcome surprise to discover that stratification of patients in this particular setting has been to limited avail. Data from ambitious, prospective trials have suggested that less than 5% of patients have benefitted from genetically informed therapies [Citation1]. Moreover, a diagnostic PCM infrastructure based on next-generation sequencing (NGS) and appendant procedures within the public health service is resource-demanding [Citation2]. Therefore, until now, PCM has not been widely implemented due to the lack of evidence on clinical benefits and the potential economic burden on the healthcare system.
The PCM concept poses challenges for the collection of clinical evidence that could eventually justify its adoption [Citation3,Citation4]. The main challenge involves the realisation of well-structured clinical trials that accurately reflect treatment effects. In PCM, where patient populations are often very small (equal to one at the extreme) and the conduct of a randomised controlled trial (RCT) is seldom an option [Citation5], guidelines for how to define a control group are missing. One alternative is to compare patients receiving molecularly matched therapies with a similar group of patients who do not, but an analytic concern is the potential selection bias in the estimation of the health effects because the former patient group may systematically differ from the latter. In addition, in a cost-effectiveness framework, this particular comparison may not always include the costs of the diagnostic PCM infrastructure or any PCM-related complications. For all of these reasons, there is a need to compare with various control groups when evaluating the cost-effectiveness of PCM in its entirety.
In this report, we used data from the MetAction study for molecularly matched off-label therapies for end-stage cancer [Citation6] to estimate the cost-effectiveness of PCM. The first estimation compared MetAction patients who received matched medications with the study patients devoid of an actionable tumour target, who instead had best supportive care (BSC) for the end-stage disease. Next, we compared all MetAction cases with external BSC controls from published RCTs with similar patient populations where genetic tumour testing had not been performed. The analyses embodied the valuation of all factors that are commonly requested in a PCM cost-effectiveness assessment [Citation3].
Material and methods
The MetAction study – intervention populations
The MetAction study was approved by the Regional Committee for Medical and Health Research Ethics of South-East Norway, Norwegian Medicines Agency and the institutional review boards at Akershus University Hospital and Oslo University Hospital, and conducted in accordance with the Declaration of Helsinki. Written informed consent was required for participation. An eligible patient had treatment-refractory end-stage cancer with a life expectancy of at least 3 months. The clinical study interventions were derived from NGS-based identification of one or more gene variants that indicated drug sensitivity or resistance – a positive or negative actionable target (AT) – in a biopsy from a metastatic tumour. The full diagnostic workflow has been described previously [Citation2]. In the present cost-effectiveness study, the intervention (Supplementary Table S1) was modelled as either the entire MetAction study population (all patients whether receiving molecularly matched treatment or BSC; n = 26) or the subgroup of AT-positive cases who received molecularly matched therapy until response assessment (n = 10) [Citation6].
Comparator populations
Based on the characteristics of the MetAction study participants, the chosen BSC comparator populations (Supplementary Table S2) were from two RCTs on end-stage colorectal cancer (CRC) patients of comparable age. The RECOURSE RCT assessed the efficacy and safety of TAS-102, a chemotherapy regimen (trifluridine and tipiracil hydrochloride) [Citation7], and the CORRECT RCT assessed regorafenib, a multi-target kinase inhibitor [Citation8], both against placebo (BSC; n = 266 in RECOURSE and n = 255 in CORRECT). Furthermore, the AT-negative MetAction patients and the 3 AT-positive patients who for medical reasons could not receive molecularly matched therapy until response assessment, together referred to as AT-untreatable cases (n = 16), were used as comparator population.
Outcomes
Health effects were measured in life years (LYs; overall survival) and quality-adjusted life years (QALYs). Cost-effectiveness results were expressed by the incremental cost-effectiveness ratio (ICER) of PCM relative to BSC (here for QALY):
(1)
(1)
The analyses were conducted according to Norwegian guidelines, which recommend a healthcare perspective. Both costs and health effects were half-cycle corrected and discounted at a 4% rate. An annual willingness-to-pay (WTP) threshold of NOK 605,000, corresponding to EUR 56,384 (EUR 1 = NOK 10.73 on average for 2020), was applied to assess the cost-effectiveness of PCM. The threshold was calculated using the absolute shortfall method reported in the guidelines for the submission of documentation for a single technology assessment of pharmaceuticals by the Norwegian Medicines Agency in 2020 [Citation9]. Without considering uncertainty, if the ICER is equal to or less than the WTP, the intervention is considered cost-effective and can be implemented.
Partitioned survival models
The models (Supplementary Figure S1) were used to simulate the outcomes for cohorts of 1000 patients in a 10-year perspective. The cycle length was set to 3 weeks. The models included 3 health states for patients in the PCM intervention group (Treatment, Progression and Death) and 2 health states for the external BSC comparator groups (Progression and Death). For the MetAction population, AT-positive cases entered Treatment and AT-untreatable cases entered Progression. For each new cycle, patients in Treatment could either remain in the health state or move to Progression or Death, while patients in Progression could either stay or move to Death.
Death probabilities were calculated as:
(2)
(2)
The Progression health state was calculated as:
(3)
(3)
The health effects (LY and QALY) and cost-effectiveness (ICER) were assigned to all health states. Also, we assigned health-related quality-of-life weights to the health states Progression and Treatment [Citation10–12]; for the first 4 cycles of the latter, we assigned a lower weight to reflect the burden of the disease and treatment (Supplementary Table S3). LYs per cycle were also calculated.
Data input
To estimate BSC overall survival for the RECOURSE and CORRECT studies, we used WebPlotDigitizer [Citation13] to read off the curves, while progression-free and overall survival from the MetAction study were based on individual level data [Citation6]. To choose the optimal distribution, we followed the recommendation of using a combination of smoothened hazard plots, visual inspection and goodness of fit [Citation14] as estimated by the Akaike Information Criterion [Citation15] and the Bayesian Information Criterion [Citation16]. In the choice of optimal specification, we applied parametric (log-logistic, Weibull) and flexible spline models, the latter with different splines (Supplementary Table S4).
Costs
The following details were applied for estimations of costs:
MetAction
The full NGS procedure for all MetAction cases ( and Supplementary Table S5). Costs relating to the actual time spent by personnel within study-specific procedures as well as the acquisition of equipment and disposables were calculated. National wage rates (low and high) for the personnel and estimated costs for the theoretical usage (restricted and extended) of the Ion Torrent PGM™ were utilised as valuation. A fixed price of the Ion Oncomine™ Comprehensive Assay (the 143-gene panel used for the sequencing) with reagents and the costs for the assumed capacity of 10–20 terabytes to store the sequence data were entered. We adjusted the costs for risk of 1.04 for the possibility of sequencing a patient sample twice, as extracted from the MetAction database. In the downstream analyses, we applied the low-rate NGS procedure costs (a total of EUR 4101 per patient).
Table 1. Costs of the full next-generation sequencing procedure per patient.
Treatment with molecularly matched therapy ( and Supplementary Table S5). These costs were factored into an AT-positive patient expected to be treated for a period of 2 years. In the MetAction study, only 2 (7.7%) of the included patients were treated for as long as 2 years (both with an immune checkpoint inhibitor; intravenous medication) but achieved complete response and were still tumour-free 1 year after discontinuation when the study was completed [Citation6]. In addition, we included costs relating to visits to the outpatient clinic every 2 weeks, as valued by the national diagnosis-related group weight and unit cost. For patients receiving intravenous medication, we included the time for treatment administered by a nurse at each visit. No costs for admission from adverse events were included, based on no observed Common Terminology Criteria for Adverse Events grade 3 or higher events in the MetAction study.
Table 2. Resource use and unit costs in the intervention and comparator populations.
Follow-up ( and Supplementary Table S5). For patients in the Treatment health state, we applied a period of 5 years of follow-up, which included in total 1 visit for colonoscopy and a visit to the general practitioner every 6 months before the follow-up program was discontinued.
BSC
Costs for BSC ( and Supplementary Table S5) included hospital admission over 90 index days. On average, an AT-untreatable MetAction patient had 7.2 days in the hospital during this period [Citation2]. In the present model, we included the costs of 1 hospital admission per individual entering the Progression health state. Care outside the hospital setting was included in the end-of-life (EoL) costs.
EoL care
Costs of care relating to EoL (primary healthcare and home-/community-based care) were based on a recent study on CRC patients [Citation17] and applied to all strategies. In this, the costs were grouped into three EoL period lengths (4–6 months, 2–3 months and the last month of life) and the average costs per month were calculated ( and Supplementary Table S5).
Data analyses
The analyses were performed using Microsoft Excel with Visual Basic for Application v2016. The cost-effectiveness analysis of each comparison is represented by ICER (ΔCost/ΔLY and ΔCost/ΔQALY). We used one-way sensitivity analyses to identify the effects of the costs of the NGS procedure and the molecularly matched medications (per cycle length). To account for uncertainty in all input parameters, we ran a probabilistic sensitivity analysis with 1000 iterations for all comparisons to estimate the proportion of simulated ICERs that would lie below the WTP threshold. The distributions assigned for progression-free and overall survival were based on the estimated standard errors. Gamma distributions were used for unit costs (assuming 20% standard errors) and beta distribution for health-related quality-of-life (in which the standard errors were defined by the literature; Supplementary Table S3). The results are presented as cost-effectiveness planes (scatter plots) and by cost-effectiveness acceptability curves (in which the estimated probability that PCM is the cost-effective alternative is shown according to increasing threshold values) [Citation18].
Results
The MetAction outcome data
The study data have been published in full previously [Citation6]. In brief, 10 out of the 26 patients undertaking tumour NGS analysis were AT-positive cases who received molecularly matched therapy until response assessment. Among these, 4 patients had direct disease progression and 2 patients had disease stabilisation at 7–8 weeks before progression. Besides, 2 CRC patients (one given chemotherapy in combination with the targeted drug) had a partial response with 10.4–17.0 weeks of duration. The median time on treatment for these 8 patients was 12.9 weeks (range, 7.6–23.9). As the only subjects given an immune checkpoint inhibitor, 2 CRC patients had durable molecular or radiologic complete responses [Citation6,Citation19]. Except for these 2 cases, overall survival was similar for patients who did and did not receive study treatment [Citation6].
Deterministic base-case analyses
When comparing healthcare costs and health outcomes for the MetAction patients given molecularly matched medication (AT-positive cases) and the study patients receiving BSC (AT-untreatable cases), the incremental costs were EUR 32,027 while the QALY increment was 1.00 (). This ICER is well below the 2020 national WTP threshold of EUR 56,384 (NOK 605,000) and reflects the costs of the medications given and the improved survival (with follow-up) among the responding patients (Supplementary Table S5) in a setting where all patients had received tumour NGS analysis to determine the eligibility for molecularly based therapy in end-stage cancer.
Table 3. Cost-effectiveness of precision cancer medicine.
When comparing the MetAction patients (all cases) with patients receiving BSC in the RECOURSE and CORRECT studies (), where tumour NGS analysis had not been undertaken, the incremental costs were EUR 16,414 and EUR 16,439, respectively. In addition to the NGS-related costs, these cost differences were driven by the medications given to the AT-positive MetAction patients and their marginally higher EoL care (Supplementary Table S5). Taking account of all of the cost elements, the incremental QALYs of the MetAction strategy were 0.13 and 0.15 relative to RECOURSE and CORRECT, respectively, resulting in ICERs of EUR 126,262 and EUR 109,593 (), which are twice or more as high as the WTP threshold.
For all comparisons, the ICERs were 30–40% lower when LYs were used as the main health outcome (). The discounted incremental LY was more than 15 months when comparing the MetAction patients given molecularly matched medication with the remaining study patients not receiving tumour-directed therapy, reflecting the 2 study patients (of 26 patients enrolled) who received an immune checkpoint inhibitor and experienced a durable complete response. However, when comparing the entire MetAction population with the external BSC controls, the incremental LYs gained were only 9–11 weeks.
One-way sensitivity analyses
We found that ICERs (using QALY as the health outcome) were relatively insensitive to changes in costs of the NGS procedure; for example, a reduction to half the costs in the MetAction study would still not have resulted in ICERs at the 2020 national WTP threshold when compared to BSC in the RECOURSE and CORRECT studies (, upper panel). In stark contrast, a reduction of drug expenses to half the list price (using the mean of list prices that applied to the MetAction medications) would have led to the significant decline in ICERs of the PCM strategy compared to BSC, likely reaching the WTP threshold (, lower panel).
Figure 1. One-way sensitivity analysis. Incremental cost-effectiveness ratio (ICER) as a function of changes in total costs of the next-generation sequencing procedure (upper panel) and drug expenses per cycle length (3 weeks; lower panel) in all MetAction patients versus patients given best supportive care in the RECOURSE study (solid lines) or CORRECT study (dashed lines) or MetAction patients given molecularly targeted therapies versus those who were not (dotted lines). The thin dotted lines represent the 2020 annual willingness-to-pay threshold.
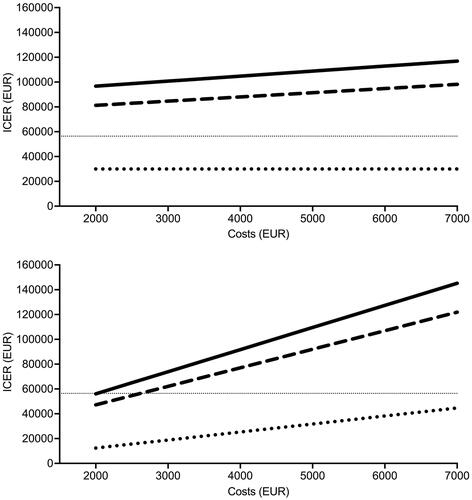
Probabilistic sensitivity analyses
For the comparison of the AT-positive MetAction patients with the AT-untreatable ones, 99% of the simulated ICERs fell below the estimated WTP threshold (, upper left panel) with a likelihood that the AT-positive cases were cost-effective converging to 100% at this specific threshold (, upper right panel). When comparing all MetAction cases with external patients receiving BSC, only 2% (versus RECOURSE; , middle left panel) and 27% (versus CORRECT; , lower left panel) of the simulated ICERs fell below the WTP threshold. For these comparisons, the >50% likelihood for the MetAction strategy to be cost-effective was at thresholds above EUR 100,000 (versus RECOURSE; , middle right panel) and EUR 90,000 (versus CORRECT; , lower right panel).
Figure 2. Probabilistic sensitivity analysis. Incremental costs as a function of incremental quality-adjusted life years (QALYs) (left panels). Cost-effectiveness acceptability as a function of increasing willingness-to-pay threshold (right panels). Upper panels: MetAction patients given molecularly targeted therapies (dashed line) versus those who were not (solid line). Middle panels: All MetAction patients (dashed line) versus patients given the best supportive care in the RECOURSE study (solid line). Lower panels: All MetAction patients (dashed line) versus patients given the best supportive care in the CORRECT study (solid line). The thin dotted lines represent the 2020 annual willingness-to-pay threshold; for the left panels, dots to the right represent simulated ICERs below this threshold.
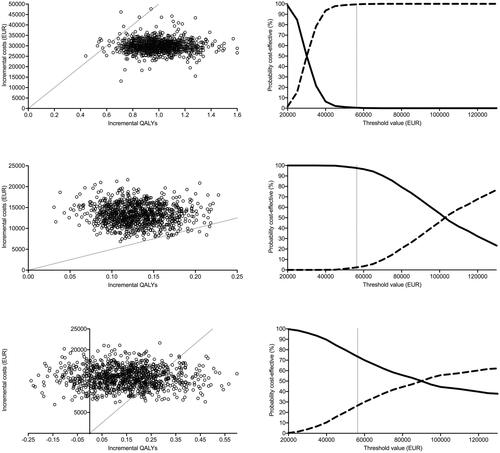
Discussion
Healthcare resources are limited and demand prioritisation among the users. The expensive and often modestly beneficial PCM for end-stage disease [Citation20] may be out of proportion to those resources if we cannot argue well for the motivation and justification of the spending. In the present analysis, using data from our MetAction PCM study and external BSC controls from published RCTs with similar patient populations, we found that the high ICERs of PCM were driven by the costs of the molecularly matched therapies that could be given, including modest costs relating to the improved survival of the responding patients. We applied the calculated low-rate NGS procedure costs (a total of EUR 4101 per patient) but found that further reduction (or an increase) of this variable would not significantly affect the ICER for the PCM strategy relative to BSC. In contrast, a price reduction of drugs would contribute significantly to a cost-effective PCM strategy. With the employed input factors, the likelihood for PCM to be cost-effective for the end-stage disease was approximately twice the annual WTP threshold. For the entire patient population that had tumour NGS analysis and the opportunity of treatment with molecularly matched medications, the survival benefit was small (gained LYs of only 9–11 weeks).
The NGS technology has become progressively more cost-effective over the time it has been in use for clinical decisions. Retail costs for most of the technical elements of the diagnostic assays have fallen. However, personnel costs are significant in the public health service of Norway and other very-high Human Development Index countries and account for a high percentage of the total expenses when the diagnostic infrastructure is first established. The continuous training of the involved personnel might contribute to improved NGS cost-effectiveness over time when it becomes implemented in routine practice. Concerning the optimal timing of NGS analysis over the course of metastatic cancer, outside a small number of indications in routine practice, the European Society for Medical Oncology recommends molecular tumour profiling only when patients are eligible for biomarker-driven clinical trials [Citation21]. Regarding molecularly targeted medicines, they are expensive to develop and therefore high-priced [Citation22], but prices commonly decline as soon as the brand-name drugs are challenged by low-cost generic versions. Health authority-negotiated pricing is another mechanism that lowers list prices [Citation23]. Our analyses indicated that such regulations will contribute substantially toward a cost-effective PCM service in public healthcare.
Overall, our analyses embodied the valuation of crucial factors of a PCM cost-effectiveness assessment [Citation3] – the type of technology (NGS), the time horizon (10 years) with the annual discount rate (4%), specification of the WTP threshold and base-case and sensitivity analyses in a high-cost public healthcare perspective. Thus, the outcome data may be used in support of resource allocations regarding PCM in the specialist health service. Precision medicine has the ultimate goal of forming biologically precise treatments for diseases that have previously been seen as intractable. Thus, formal valuations may not reflect the true clinical and societal values provided to patients. For example, discounting is a well-established practice in these types of analyses. But interventions for end-stage cancer in which patients will otherwise die within a short period of time may become less impacted because the discounting devaluates gained survival. Furthermore, the extended survival entails costs associated with lengthened management of the patients, which devaluate the intervention but is counterintuitive to how society appraises this healthcare. The actual cost-effectiveness thresholds may be arbitrary and thereby topics of debate. A systematic review with QALY as an outcome revealed variation in WTP depending on population and societal variables [Citation24]. Specifically, we calculated the WTP threshold from absolute shortfall [Citation9], measuring QALYs lost in a study population relative to the general population. The resulting threshold was within one of the severity groups defined by Norwegian priority settings and comparable with WTP in Europe elsewhere (e.g., EUR 20,000–80,000 in the Netherlands).
On the other hand, PCM trials typically apply overall response rate as the primary endpoint. This tumour response-based measure may not be a reliable surrogate for progression-free and overall survival in CRC and other tumour entities [Citation25]. PCM is in essence technology-driven and includes extraordinarily expensive medications, which raises the ethical question if it can rightfully occupy limited healthcare resources [Citation26]. This is of particular note if there is no solid evidence to support the use of high-cost, marginally beneficial cancer drugs [Citation20,Citation27] at the expense of investments into other types of cancer treatments (e.g., in surgical and radiation oncology) following reimbursement decisions made through appropriate health technology assessments.
There are evident limitations to our study. The MetAction trial had a small case number that may not have had enough power to capture a factual PCM benefit. An obstacle to proving benefit lies in the fact that actionable gene variants often are too infrequent in predefined patient cohorts for conducting a comprehensive PCM trial. Moreover, the cost-effectiveness analyses presented here employed end-stage CRC with unknown tumour genome profiles as comparator populations (because 70% of the AT-positive MetAction cases had end-stage CRC). Patients with CRC have few lines of systemic therapies in the advanced setting and are often in good performance status even at the end-stage, thus clinically amenable to off-label use of molecularly targeted medicines. However, most CRC cases are devoid of drug-sensitivity targets [Citation28,Citation29]. A cost-effectiveness analysis in patient populations with a higher incidence of tumour gene variants that confer drug sensitivity might have led to a stronger valuation of PCM. That said, the first major PCM cohort in the ongoing Dutch DRUP trial was dominated by CRC patients [Citation30]; also, drug resistance markers frequently found in CRC result in the selective withholding of therapies, which is cost-saving.
In conclusion, we have presented models comparing patients with end-stage cancer analysed by NGS for the opportunity to provide molecularly matched therapies, with comparator populations instead receiving BSC. The models suggested that the high ICERs of PCM were driven by costs relating to the NGS diagnostics, the medications and the management of patients with improved survival, with a likelihood for the strategy to be cost-effective defying WTP constraints. Our data support a public-private partnership model of sharing the costs of molecularly matched medicines in PCM, exemplified by the model used in the ongoing DRUP and IMPRESS-Norway trials [Citation31,Citation32]. The DRUP investigators emphasise that drug manufacturers must provide their products for free until the patients present meaningful clinical responses in order for the risk-sharing model to meet the purpose [Citation31]. Several cost-effectiveness analyses of PCM have been reported previously, not infrequently found to have insufficient data that populate the models [Citation4]. Thus, we believe our adherence to crucial factors for such assessment [Citation3] is a template for similar outcome reporting. Our effort has exposed a need for predefining pertinent control arms in future PCM studies.
Supplemental Material
Download MS Word (41.2 KB)Disclosure statement
Prof. A.H. Ree has received honoraria from Merck Sharp & Dohme and Bristol-Myers Squibb and research funding (fees to institution) from Bristol-Myers Squibb. Prof. K. Flatmark has received research funding (fees to institution) from Bayer Pharma. Prof. H.G. Russnes has received honoraria (fees to institution) from AstraZeneca, Pfizer, InCyte, Merck and Roche and research funding (fees to institution) from Foundation Medicine. Prof. G.M. Mælandsmo, Ms. M. Gómez Castañeda and Prof. E. Aas have declared no conflicts of interest.
Data availability statement
The dataset used and analysed in this study can be made available from the corresponding author on reasonable request and in accordance with the General Data Protection Regulation of the European Union.
Additional information
Funding
References
- Tannock IF, Hickman JA. Molecular screening to select therapy for advanced cancer? Ann Oncol. 2019;30(5):661–663.
- Ree AH, Russnes HG, Heinrich D, et al. Implementing precision cancer medicine in the public health services of Norway: the diagnostic infrastructure and a cost estimate. ESMO Open. 2017;2(2):e000158.
- Weymann D, Pataky R, Regier DA. Economic evaluations of next-generation precision oncology: a critical review. JCO Precis Oncol. 2018;2:1–23.
- Kasztura M, Richard A, Bempong N-E, et al. Cost-effectiveness of precision medicine: a scoping review. Int J Public Health. 2019;64(9):1261–1271.
- Chan KKW, Tannock IF. Should basket trials be pathways to drug registration for biomarker-defined subgroups of advanced cancers? J Clin Oncol. 2021;39(22):2426–2429.
- Ree AH, Nygaard V, Boye K, et al. Molecularly matched therapy in the context of sensitivity, resistance, and safety; patient outcomes in end-stage cancer – the MetAction study. Acta Oncol. 2020;59(7):733–740.
- Mayer RJ, Van Cutsem E, Falcone A, et al. Randomized trial of TAS-102 for refractory metastatic colorectal cancer. N Engl J Med. 2015;372(20):1909–1919.
- Grothey A, Van Cutsem E, Sobrero A, et al. Regorafenib monotherapy for previously treated metastatic colorectal cancer (CORRECT): an international, multicentre, randomised, placebo-controlled, phase 3 trial. Lancet. 2013;381(9863):303–312.
- Guidelines for the submission of documentation for single technology assessment (STA) of pharmaceuticals. [cited 2021 Dec 20]. Available from: https://legemiddelverket.no/Documents/English/Public%20funding%20and%20pricing/Documentation%20for%20STA/Guidelines%2020.05.2020.pdf.
- Färkkilä N, Torvinen S, Sintonen H, et al. Costs of colorectal cancer in different states of the disease. Acta Oncol. 2015;54(4):454–462.
- Ratcliffe J, Longworth L, Young T, et al. Assessing health-related quality of life pre- and post-liver transplantation: a prospective multicenter study. Liver Transpl. 2002;8(3):263–270.
- Bjørnelv GMW, Dueland S, Line P-D, et al. Cost-effectiveness of liver transplantation in patients with colorectal metastases confined to the liver. Br J Surg. 2019;106(1):132–141.
- WebPlotDigitizer. [cited 2021 Dec 20]. Available from: https://automeris.io/WebPlotDigitizer/.
- Latimer NR. Survival analysis for economic evaluations alongside clinical trials—extrapolation with patient-level data: inconsistencies, limitations, and a practical guide. Med Decis Making. 2013;33(6):743–754.
- Akaike H. A new look at the statistical model identification. IEEE Trans Automat Contr. 1974;19(6):716–723.
- Schwarz G. Estimating the dimension of a model. Ann Statistics. 1978;6:461–464.
- Bjørnelv GMW, Edwin B, Fretland ÅA, et al. Till death do us part: the effect of marital status on health care utilization and costs at end-of-life. A register study on all colorectal cancer decedents in Norway between 2009 and 2013. BMC Health Serv Res. 2020;20(1):115.
- Barton GR, Briggs AH, Fenwick EAL. Optimal cost-effectiveness decisions: the role of the cost-effectiveness acceptability curve (CEAC), the cost-effectiveness acceptability frontier (CEAF), and the expected value of perfection information (EVPI). Value Health. 2008;11(5):886–897.
- Ree AH, Nygaard V, Russnes HG, et al. Responsiveness to PD-1 blockade in end-stage colon cancer with gene locus 9p24.1 copy-number gain. Cancer Immunol Res. 2019;7(5):701–706.
- Marquart J, Chen EY, Prasad V. Estimation of the percentage of US patients with cancer who benefit from genome-driven oncology. JAMA Oncol. 2018;4(8):1093–1098.
- Mosele F, Remon J, Mateo J, et al. Recommendations for the use of next-generation sequencing (NGS) for patients with metastatic cancers: a report from the ESMO Precision Medicine Working Group. Ann Oncol. 2020;31(11):1491–1505.
- Cutler DM. Early returns from the era of precision medicine. JAMA. 2020;323(2):109–110.
- Vokinger KN, Hwang TJ, Grischott T, et al. Prices and clinical benefit of cancer drugs in the USA and Europe: a cost-benefit analysis. Lancet Oncol. 2020;21(5):664–670.
- Kouakou CRC, Poder TG. Willingness to pay for a quality-adjusted life year: a systematic review with meta-regression. Eur J Health Econ. 2022;23(2):277–299.
- Cooper K, Tappenden P, Cantrell A, et al. A systematic review of meta-analyses assessing the validity of tumour response endpoints as surrogates for progression-free or overall survival in cancer. Br J Cancer. 2020;123(11):1686–1696.
- Fleck LM. Precision medicine and the fragmentation of solidarity (and justice). Med Health Care Philos. 2022;25(2):191–206.
- Aggarwal A, Fojo T, Chamberlain C, et al. Do patient access schemes for high-cost cancer drugs deliver value to society?–lessons from the NHS Cancer Drugs Fund. Ann Oncol. 2017;28(8):1738–1750.
- Sepulveda AR, Hamilton SR, Allegra CJ, et al. Molecular biomarkers for the evaluation of colorectal cancer: guideline from the American Society for Clinical Pathology, College of American Pathologists, Association for Molecular Pathology, and the American Society of Clinical Oncology. J Clin Oncol. 2017;35(13):1453–1486.
- Martini G, Dienstmann R, Ros J, et al. Molecular subtypes and the evolution of treatment management in metastatic colorectal cancer. Ther Adv Med Oncol. 2020;12:1758835920936089.
- van der Velden DL, Hoes LR, van der Wijngaart H, et al. The Drug Rediscovery protocol facilitates the expanded use of existing anticancer drugs. Nature. 2019;574(7776):127–131.
- van Waalwijk van Doorn-Khosrovani SB, Pisters-van Roy A, van Saase L, et al. Personalised reimbursement: a risk-sharing model for biomarker-driven treatment of rare subgroups of cancer patients. Ann Oncol. 2019;30(5):663–665.
- Taskén K, Russnes HEG, Aas E, et al. A national precision cancer medicine implementation initiative for Norway. Nat Med. 2022;28(5):885–887.