Background
During the last decade, major improvements in metastatic melanoma treatment have led to the widespread use of immune checkpoint inhibitors (ICIs) and targeted therapies [Citation1,Citation2]. Although anti–PD-1–based immunotherapy has improved treatment outcomes of patients with metastatic melanoma, all patients do not benefit from it. The tumor microenvironment (TME) is a constantly evolving composition of blood vessels, immune cells, signalling molecules, fibroblasts, stromal cells, and extracellular matrix surrounding the tumor. The TME differs between tumor types, and between patients within the same tumor type and even within similar tumor genotypes. The TME plays a relevant role in immune surveillance and resistance to immunotherapy [Citation3–5].
Melanoma tumor heterogeneity is present between the patients (interpatient heterogeneity), within a tumor (intra-tumoral heterogeneity) and among tumors within a patient at various sites of the body concurrently or sequentially during the course of the disease (intertumoral or intra-patient heterogeneity) [Citation6,Citation7]. However, the BRAF mutation, which is one of the key driver mutations in melanoma, is thought to be stable and to occur early in the course of melanoma development [Citation8].
BRAF mutation is a predictive marker since only BRAF-mutated tumors respond to BRAF inhibitor therapy and mutational status thus affecting treatment selection critically [Citation9–11]. Furthermore, patients with BRAF-mutant metastatic melanoma treated with ipilimumab and nivolumab seem to have a survival benefit compared to patients with wild-type tumors [Citation1], and that treatment-naïve BRAF-mutant melanoma has a distinct immune profile compared with BRAF-wild type melanoma in the TME [Citation12].
TME and immune surveillance seem to play an important role in melanoma pathogenesis. Tumor heterogeneity may affect treatment response and hasten the development of acquired resistance. Specific immune gene expression signatures and individual genes associated with the response to immunotherapy [Citation13–16]. An area less studied is the immune signature gene heterogeneity among samples within a patient. Regulatory T-cell (Treg)-mediated T-cell suppression is an important mechanism for the tumor cells to escape the host’s activated immune system. A central mechanism contributing to impaired T-cell function against tumors or pathogens is T-cell exhaustion. Infection-induced exhaustion is usually reversible, whereas tumor-induced dysfunction of T-cells is only reversible at early malignant stages and irreversible at later stages [Citation17].
In this pilot study we aimed to characterize immunological profiles by gene-expression analysis and to explore if any mutational heterogeneity could be detected between sequential intrapatient primary and metastatic melanoma tumors.
Material and methods
Patient samples
We searched our electronic patient record database for metastatic melanoma patients with available formalin-fixed, paraffin-embedded primary tumors and sequential metastatic samples. We found 9 metastatic melanoma patients with matching primary and metastatic tumor samples. Of these 8 were immunotherapy-naïve patients.
Patient G presented with metastatic disease soon after the primary tumor resection and underwent a biopsy of a subcutaneous metastasis two months after treatment initiation. All patients were treated at Helsinki University Hospital Comprehensive Cancer Center between 2016 and 2017 and biopsies were taken during standard diagnostic or treatment procedures. No additional samples were taken for this study. Patient characteristics are described in . The mutational status of the tumor samples was determined by next-generation sequencing as part of the routine clinical analysis covering ten cancer genes, including BRAF, NRAS, and KIT, at the HUS Diagnostic Center (HUSLAB) at Helsinki University Hospital. The study was approved by the Helsinki Biobank and Institutional Review Board of Helsinki University Hospital. Data was anonymized before statistical analyses and handled in a manner that met general data protection regulations.
Table 1. Patient characteristics. Previous treatments indicate systemic therapy for metastatic disease. .
Digital gene expression profiling and statistical analysis
For RNA isolation, three 20 µm sections from formalin-fixed paraffin-embedded (FFPE) blocks were processed with RecoverAll™ Total Nucleic Acid Isolation Kit for FFPE (Life Technologies, Thermo Fisher Inc., Waltham, MA) according to manufacturer’s instructions. Thereafter, a total of 100 ng of RNA was hybridized with the 770-gene nCounter PanCancer IO 360™ Panel codeset (NanoString Technologies, Seattle, WA) and analyzed with the nCounter Digital Analyzer system (NanoString Technologies). The quality of the data was confirmed by using the default quality control settings in nSolver 3.0 software (NanoString Technologies). Thereafter, the data were normalized using the geNorm algorithm implemented in nSolver Advanced Analysis plug-in, and log2 was transformed for subsequent analyses, which were performed by R version 4.0.2. Genes expressed in <10% of the samples, based on negative control geometric mean thresholding, were filtered out from the analyses. This resulted in the final dataset of 729 genes. For differential gene expression analysis, R package “limma” was used [Citation18].
Results
Patient samples
We analyzed 18 melanoma tumor samples from nine patients for this study, of which nine were primary melanomas, and nine metastases. The patient characteristics of these patients are presented in .
Gene expression analysis
In order to study the differences in primary and metastasis samples, we first performed pairwise correlation analyses for each patient’s primary and metastatic samples. In general, the correlation of the matched primary and metastatic samples was relatively strong (Pearson R > 0.7 for all patients) (). Then, we proceeded with hierarchical clustering, which enables the identification of differences and similarities throughout all patients and all samples. The clustering analysis based on all genes showed heterogeneity between patients and samples, and the primary and metastatic samples could not be split into their own groups (). Instead, for three patients (D, H, and L), the primary and metastatic sample pairs clustered closely together in the same subcluster, whereas for six patients the primary and metastatic samples resided in distinct clusters (). Moreover, BRAF and NRAS-mutated tumors clustered irrespective of each other (). The time from primary to metastasis did not associate with the gene expression profile. For instance, patient L, which had the longest time from primary to metastasis (74 months), had the strongest correlation (R = 0.91) and there were only a few differentially expressed genes between the primary and the metastatic samples ( and ).
Figure 1. Correlation of paired primary and metastatic tumor samples. Gene expression was assessed using the 770-gene NanoString PanCancer IO 360™ Panel. The scatter plots visualize correlation of the paired primary (x-axis) and metastatic (y-axis) melanoma patient samples. Selected genes with log2 fold change >3 are highlighted in the plots. R denotes Pearson correlation coefficient.
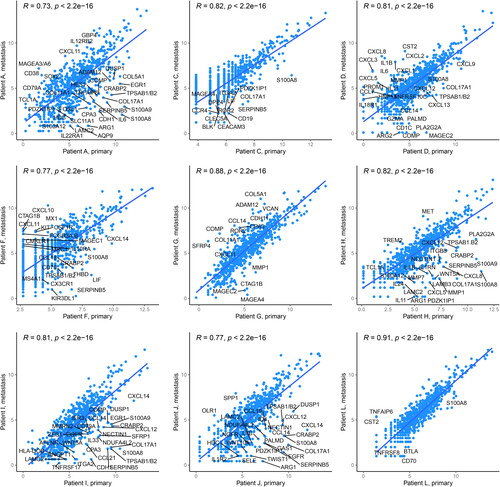
Figure 2. Gene expression profiles in the primary and metastatic melanoma patient samples. (A) Unsupervised hierarchical clustering of the NanoString PanCancer IO 360™ Panel genes in paired primary (n = 9) and metastatic (n = 9) melanoma samples. The heatmap was generated using Euclidean distance with ward.D linkage. The rows in the heatmap denote genes and columns samples. The data were z-score normalized, scaled row-wise, and blue color denotes lower expression and red color higher expression. The upper panel shows annotation of the samples. (B) The heatmap visualizes differentially expressed genes between paired primary and metastatic melanoma samples (p < 0.01, moderated t-test by paired “limma” analysis). prim: primary; met: metastasis.
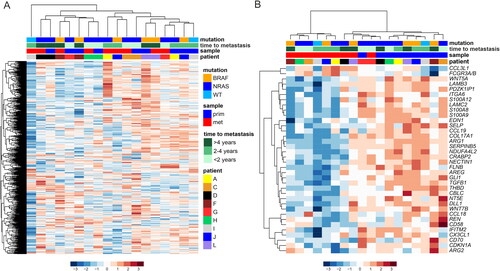
Despite the heterogeneity between the patients, we were able to identify 36 genes (p < 0.01), which were differentially expressed across all paired primary and metastatic melanoma samples (). The majority of the identified genes had lower expression in the metastases as compared to the primary tumors. For instance, in the primary tumor samples, we found higher expression of genes for the Wnt-signaling pathway (WNT5A, WNT7B), S100 calcium binding proteins (S100A8, S100A9, S100A12), as well as extracellular matrix (COL17A1, LAMC2, LAMB3, TGFB1, ITGA6). Two genes, C-C Motif Chemokine Ligand 3 Like 1 (CCL3L1) and Fc Gamma Receptor IIIa/b (FCGR3A/B) had higher expression in the metastatic samples.
Discussion
In this pilot study we analyzed differences between primary tumors and metastases of nine patients with metastatic melanoma at the gene expression level. We found that several immune-related genes have higher expression in primary tumor samples compared to the metastatic samples, respectively. Data on these immunologically relevant genes and their association to progression of melanoma are limited. Furthermore, their relevance and interplay in the TME of primary melanomas compared to metastases is still unclear. Wnt Family Member 5 A (WNT5A) is a IL-6 mediated cell motility regulator [Citation19], Laminin Subunit Gamma 2 (LAMC2) expression correlates with worse survival and risk of metastases [Citation20], and S100 calcium-binding protein A8/9 (S100A8/9) correlates with the metastatic potential of primary melanomas, worse survival and impaired response to therapy with pembrolizumab [Citation21]. High expression of S100 calcium-binding protein A12 (S100A12) is associated with worse survival in patients with metastatic melanoma treated with targeted therapy [Citation22]. Endothelin 1 (EDN1) controls UV-induced melanocytes and plays a role in the maintenance of dermal melanocytes, which loss could explain the cell’s metastatic potential in melanoma metastases [Citation23]. Furthermore, EDN1 supports phenotype heterogeneity and thus promotes the development of therapy-resistant melanoma cells [Citation24]. Our finding that collagen Type XVII Alpha 1 Chain (COL17A1) expression was higher in primary tumors than in metastases has also been shown by Xie et al. previously [Citation25]. High expression of Cellular Retinoic Acid Binding Protein 2 (CRABP2) has also been found to associate with poor survival in melanoma patients [Citation26]. GLI Family Zinc Finger 1 (GLI1), an activator if the Hedgehog signaling pathway, affects melanoma invasion and cell migration [Citation27]. To our knowledge, no correlations with survival have been found for Arginase 1 (ARG1) or Serpin Family Member 5 (SERPINB5) between primary melanomas and melanoma metastases. However, in colorectal cancer, ARG1 has been found to correlate with impaired overall survival [Citation28], and in pancreatic cancer, SERPINB5 overexpression promotes metastases and is also associated with poor survival [Citation29]. In many cancer types, 5′-Nucleotidase Ecto (NT5E) associates with the infiltration of cancer-associated fibroblasts and worse prognosis [Citation30].
Integrins play a role in cell adhesion and their loss of expression is associated with higher capacity of invasion [Citation31, Citation32], which supports our observation of the loss of expression of Integrin Subunit Alpha 6 (ITGA6) in metastatic samples. In metastases, we found low expression of CD58 and CD70. CD58 loss is associated with immune evasion and resistance to therapy with ICI [Citation33]. Loss of CD70 is in accordance with previous findings from Pich et al. who reported that CD70 expression associates with lower metastatic potential and is found more often in primary tumor samples than in metastatic samples [Citation34]. Our observation that primary melanomas are more likely to be immunologically hot may indicate that they are more responsive to ICIs. As shown by Rozeman et al. in the neoadjuvant setting, patients with high tumor mutational burden and high IFN-gamma signature treated with ipilimumab-nivolumab had a 100% pathological response rate (pRR) compared to patients with low signatures (pRR 39%) [Citation35]. The recent study by Patel et al. showed that neoadjuvant is more effective than adjuvant immunotherapy supporting the assumption of ICIs being more beneficial early in the course of the disease [Citation36].
Our results support the findings made by others of immunologically cold tumors being more often present in the metastatic setting [Citation13,Citation37,Citation38]. Successful treatment of metastatic and immunologically colder tumors demands for a combinatorial strategy in contrast to the warmer ones encountered more often in the adjuvant setting.
Transforming an immunologically cold tumor into one responding to ICI requires release of tumor antigens with an interference such as oncolytic viral therapy, radiation therapy, other local treatment, or cytotoxic systemic treatments causing tumor cell disruption [Citation39,Citation40]. In cancer therapy, exhaustion of CD8+ T cells may explain ICI treatment failure. Identifying factors influencing T-cell exhaustion and Treg-mediated T-cell suppression enabling tumor cells’ escape of host’s activated immune system would be helpful. [Citation17].
As we found many immunologically active genes differing between the primary tumors and their counterpart metastases, primary tumor samples may be unrepresentative of the metastatic TME. Therefore, it is advisable to examine the TME from a metastatic biopsy taken as close as possible to treatment begin to predict potential immunotherapy responsiveness. Several clinical trials mandate a fresh biopsy from a metastasis not older than three months, which seems reasonable. PD-L1 is a marker for response to anti-PD-1 therapy in some malignancies. In melanoma, however, when selecting patients benefiting from immunotherapy, PD-L1 has proven unselective and thus an unreliable marker. [Citation41].
A predictive marker to support clinical decisions, such as which patients need an escalated, toxic, and expensive front-line combination immunotherapy and which ones succeed long-term with monotherapy, is furthermore lacking.
In conclusion, TME seems to change during disease progression, and therefore tumor tissue sampling should be done just prior to treatment of metastatic disease to find predictive factors that could be used for treatment planning. Since the number of patients in this pilot study is very limited, the results should be interpreted with much caution and should be seen as hypothesis generating. Due to the low number of patients, it was impossible to evaluate correlations to treatment response. To confirm these observations and to evaluate possible correlations to treatment response, we plan to examine larger patient series in a prospective setting. The aim is to collect tumor biopsies prior to immunotherapy, as well as after treatment onset, to detect changes of clinical importance in the TME and to find potential predictive markers.
Acknowledgements
We thank Anne Aarnio for excellent technical assistance and the DNA Sequencing and Genomics Laboratory at the Institute of Biotechnology, University of Helsinki for the NanoString analyzes.
Disclosure statement
LK has received consulting, advisory or speakers’ bureau honoraria from Roche and Amgen, Bristol-Myers Squibb, and Roche. SL has received consulting, advisory or speakers’ bureau honoraria from Gilead, Incyte, Novartis, Orion, Roche and Sofi. MH has received consulting, speakers’ bureau, or advisory honoraria from Bristol-Myers Squibb, Merck Sharp & Dohme, Pierre Fabre, Novartis, Roche, and MedEngine. SM has received consulting or advisory honoraria from Amgen, Bristol-Myers Squibb, Merck Sharp & Dohme, Novartis, Roche, Astra Zeneca, Servier, Pierre Fabre, and Sanofi; speakers’ bureau honoraria from Bristol-Myers Squibb, Merck Sharp & Dohme, and Sanofi. All remaining authors have declared no conflicts of interest.
Data availability statement
The data supporting the findings of this study are available from the corresponding author, upon reasonable request.
Additional information
Funding
References
- Wolchok JD, Chiarion-Sileni V, Gonzalez R, et al. Long-term outcomes with nivolumab plus ipilimumab or nivolumab alone versus ipilimumab in patients with advanced melanoma. J Clin Oncol. 2022;40(2):127–137. doi:10.1200/JCO.21.02229.
- Hauschild A, Ascierto PA, Schadendorf D, et al. Long-term outcomes in patients with BRAF V600-mutant metastatic melanoma receiving dabrafenib monotherapy: analysis from phase 2 and 3 clinical trials. Eur J Cancer. 2020;125:114–120. doi:10.1016/j.ejca.2019.10.033.
- Sahu A, Kose K, Kraehenbuehl L, et al. In vivo tumor immune microenvironment phenotypes correlate with inflammation and vasculature to predict immunotherapy response. Nat Commun. 2022;13(1):5312. doi:10.1038/s41467-022-32738-7.
- Attrill GH, Lee H, Tasker AT, et al. Detailed spatial immunophenotyping of primary melanomas reveals immune cell subpopulations associated with patient outcome. Front Immunol. 2022;13:979993. doi:10.3389/fimmu.2022.979993.
- Garg M, Couturier D-L, Nsengimana J, et al. Tumour gene expression signature in primary melanoma predicts long-term outcomes. Nat Commun. 2021;12(1):1137. doi:10.1038/s41467-021-21207-2.
- Grzywa TM, Paskal W, Włodarski PK. Intratumor and intertumor heterogeneity in melanoma. Transl Oncol. 2017;10(6):956–975. doi:10.1016/j.tranon.2017.09.007.
- Lawrence MS, Stojanov P, Polak P, et al. Mutational heterogeneity in cancer and the search for new cancer-associated genes. Nature. 2013;499(7457):214–218. doi:10.1038/nature12213.
- Curtin JA, Fridlyand J, Kageshita T, et al. Distinct sets of genetic alterations in melanoma. N Engl J Med. 2005;353(20):2135–2147. doi:10.1056/NEJMoa050092.
- Ito T, Tanaka Y, Murata M, et al. BRAF heterogeneity in melanoma. Curr Treat Options Oncol. 2021;22(3):20. doi:10.1007/s11864-021-00818-3.
- Pellegrini C, Cardelli L, Padova M, et al. Intra-patient heterogeneity of BRAF and NRAS molecular alterations in primary melanoma and metastases. Acta Derm Venereol. 2020;100(1):adv00040. doi:10.2340/00015555-3382.
- Valachis A, Ullenhag GJ. Discrepancy in BRAF status among patients with metastatic malignant melanoma: a meta-analysis. Eur J Cancer. 2017;81:106–115. doi:10.1016/j.ejca.2017.05.015.
- Wang M, Zadeh S, Pizzolla A, et al. Characterization of the treatment-naive immune microenvironment in melanoma with BRAF mutation. J Immunother Cancer. 2022;10:e004095. doi:10.1136/jitc-2021-004095.
- Ayers M, Lunceford J, Nebozhyn M, et al. IFN-γ–related mRNA profile predicts clinical response to PD-1 blockade. J Clin Invest. 2017;127(8):2930–2940. doi:10.1172/JCI91190.
- Xiong D, Wang Y, You M. A gene expression signature of TREM2hi macrophages and γδ T cells predicts immunotherapy response. Nat Commun. 2020;11(1):5084. doi:10.1038/s41467-020-18546-x.
- Cristescu R, Mogg R, Ayers M, et al. Pan-tumor genomic biomarkers for PD-1 checkpoint blockade–based immunotherapy. Science. 2018;362:eaar3593. doi:10.1126/science.aar3593.
- Jiang P, Gu S, Pan D, et al. Signatures of T cell dysfunction and exclusion predict cancer immunotherapy response. Nat Med. 2018;24(10):1550–1558. doi:10.1038/s41591-018-0136-1.
- Dyck L, Mills KHG. Immune checkpoints and their inhibition in cancer and infectious diseases. Eur J Immunol. 2017;47(5):765–779. doi:10.1002/eji.201646875.
- Ritchie ME, Phipson B, Wu D, et al. Limma powers differential expression analyses for RNA-sequencing and microarray studies. Nucleic Acids Res. 2015;43(7):e47. doi:10.1093/nar/gkv007.
- Linnskog R, Jönsson G, Axelsson L, et al. Interleukin-6 drives melanoma cell motility through p38α-MAPK-dependent up-regulation of WNT5A expression. Mol Oncol. 2014;8(8):1365–1378. doi:10.1016/j.molonc.2014.05.008.
- Fu T, Liu J-X, Xie J, et al. LAMC2 as a prognostic biomarker in human cancer: a systematic review and meta-analysis. BMJ Open. 2022;12(11):e063682. doi:10.1136/bmjopen-2022-063682.
- Wagner NB, Weide B, Gries M, et al. Tumor microenvironment-derived S100A8/A9 is a novel prognostic biomarker for advanced melanoma patients and during immunotherapy with anti-PD-1 antibodies. J Immunother Cancer. 2019;7:343.
- Berciano-Guerrero M-A, Lavado-Valenzuela R, Moya A, et al. Genes involved in immune reinduction may constitute biomarkers of response for metastatic melanoma patients treated with targeted therapy. Biomedicines. 2022;10:284. doi:10.3390/biomedicines10020284.
- Chiriboga L, Meehan S, Osman I, et al. Endothelin-1 in the tumor microenvironment correlates with melanoma invasion. Melanoma Res. 2016;26(3):236–244. doi:10.1097/CMR.0000000000000235.
- Smith MP, Rowling EJ, Miskolczi Z, et al. Targeting endothelin receptor signalling overcomes heterogeneity driven therapy failure. EMBO Mol Med. 2017;9(8):1011–1029. doi:10.15252/emmm.201607156.
- Xie R, Li B, Jia L, et al. Identification of core genes and pathways in melanoma metastasis via bioinformatics analysis. Int J Mol Sci. 2022;23:794. doi:10.3390/ijms23020794.
- Yan J, Wu X, Yu J, et al. Prognostic role of tumor mutation burden combined With immune infiltrates in skin cutaneous melanoma based on multi-omics analysis. Front Oncol. 2020;10:570654. doi:10.3389/fonc.2020.570654.
- Giuntini G, Coppola F, Falsini A, et al. Role of the hedgehog pathway and CAXII in controlling melanoma cell migration and invasion in hypoxia. Cancers. 2022;14:4776. doi:10.3390/cancers14194776.
- Ma Z, Lian J, Yang M, et al. Overexpression of arginase-1 is an indicator of poor prognosis in patients with colorectal cancer. Pathol Res Pract. 2019;215(6):152383. doi:10.1016/j.prp.2019.03.012.
- Tian C, Öhlund D, Rickelt S, et al. Cancer cell–derived matrisome proteins promote metastasis in pancreatic ductal adenocarcinoma. Cancer Res. 2020;80(7):1461–1474. doi:10.1158/0008-5472.CAN-19-2578.
- Xue X, Liu Y, Chen X, et al. Pan-cancer analysis identifies NT5E as a novel prognostic biomarker on cancer-associated fibroblasts associated with unique tumor microenvironment. Front Pharmacol. 2022;13:1064032. doi:10.3389/fphar.2022.1064032.
- Vizkeleti L, Kiss T, Koroknai V, et al. Altered integrin expression patterns shown by microarray in human cutaneous melanoma. Melanoma Res. 2017;27(3):180–188. doi:10.1097/CMR.0000000000000322.
- Nurzat Y, Su W, Min P, et al. Identification of therapeutic targets and prognostic biomarkers Among integrin subunits in the skin cutaneous melanoma microenvironment. Front Oncol. 2021;11:751875. doi:10.3389/fonc.2021.751875.
- Frangieh CJ, Melms JC, Thakore PI, et al. Multimodal pooled Perturb-CITE-seq screens in patient models define mechanisms of cancer immune evasion. Nat Genet. 2021;53(3):332–341. doi:10.1038/s41588-021-00779-1.
- Pich C, Sarrabayrouse G, Teiti I, et al. Melanoma-expressed CD70 is involved in invasion and metastasis. Br J Cancer. 2016;114(1):63–70. doi:10.1038/bjc.2015.412.
- Rozeman EA, Hoefsmit EP, Reijers ILM, et al. Survival and biomarker analyses from the OpACIN-neo and OpACIN neoadjuvant immunotherapy trials in stage III melanoma. Nat Med. 2021;27(2):256–263. doi:10.1038/s41591-020-01211-7.
- Patel SP, Othus M, Chen Y, et al. Neoadjuvant–adjuvant or adjuvant-only pembrolizumab in advanced melanoma. N Engl J Med. 2023;388(9):813–823. doi:10.1056/NEJMoa2211437.
- Liu D, Lin J-R, Robitschek EJ, et al. Evolution of delayed resistance to immunotherapy in a melanoma responder. Nat Med. 2021;27(6):985–992. doi:10.1038/s41591-021-01331-8.
- Cerezo-Wallis D, Contreras-Alcalde M, Troulé K, et al. Midkine rewires the melanoma microenvironment toward a tolerogenic and immune-resistant state. Nat Med. 2020;26(12):1865–1877. doi:10.1038/s41591-020-1073-3.
- Chen DS, Mellman I. Oncology meets immunology: the cancer-immunity cycle. Immunity. 2013;39(1):1–10. doi:10.1016/j.immuni.2013.07.012.
- Wang Q, Wu X. Primary and acquired resistance to PD-1/PD-L1 blockade in cancer treatment. Int Immunopharmacol. 2017;46:210–219. doi:10.1016/j.intimp.2017.03.015.
- Lu S, Stein JE, Rimm DL, et al. Comparison of biomarker modalities for predicting response to PD-1/PD-L1 checkpoint blockade: a systematic review and meta-analysis. JAMA Oncol. 2019;5(8):1195–1204. doi:10.1001/jamaoncol.2019.1549.