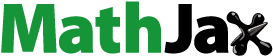
Abstract
Background
This study aimed to develop fully automated script-based radiotherapy treatment plans for cervical cancer patients, and evaluate them against clinically accepted plans, as validation before clinical implementation.
Material and methods
In this retrospective planning study, treatment plans for 25 locally advanced cervical cancer (LACC) patients with up to three dose levels were included. Fully automated plans were created using an in-house developed Python script in RayStation, and compared to clinically accepted manually made plans. Quantitatively, relevant dose statistics were compared, and average dose volume histograms (DVHs) were analyzed. Qualitatively, a blinded plan comparison was conducted between the clinical and automatic plans. The accuracy of treatment plan delivery was verified with the Delta4 Phantom+.
Results
The quantitative evaluation showed that target coverage was acceptable for all the automatic and clinical plans. The automatic plans were significantly more conformal than the clinical plans; median of 1.03 vs. 1.12. Mean doses to almost all organs at risk (OARs) were reduced in the automatic plans, with a median reduction of between 0.6 Gy and 1.9 Gy. In the blinded plan comparison, the automatic plans were the preferred plans or of equal quality as the clinical plans in 99% of the cases. In addition, plan delivery was excellent, with a mean gamma passing rate of 99.8%. Complete script-based plans were generated in 30–45 min; about four to ten times faster than manually made plans.
Conclusion
The automatic plans had acceptable target coverage, lower doses to almost all OARs, more conformal dose distributions, and were predominantly preferred by the clinicians. Based on these results, our institution has implemented the script for clinical use.
Background
External beam radiation therapy (EBRT) planning of locally advanced cervical cancer (LACC) is challenging [Citation1], especially if pathological lymph nodes are involved, due to multiple dose prescription levels and organs at risk (OARs) situated close to the target volumes. The treatment, including chemotherapy and brachytherapy, is associated with considerable normal tissue complications [Citation2–4]. However, the use of volumetric modulated arc therapy (VMAT) has resulted in improved, more conformal dose distributions [Citation5], which could potentially reduce side effects [Citation6–8]. Manual VMAT planning for LACC is complex and time-consuming [Citation1,Citation9,Citation10], and the plan quality will depend on the skills and effort of the treatment planner [Citation11], as well as the allotted planning time. A more automated process could potentially improve both quality and efficiency and guarantee less inter-planner variations [Citation12–14].
There are various types of successful automatic planning for radiotherapy described in the literature, both commercial and non-commercial [Citation9–29]. Many authors have investigated the knowledge-based planning (KBP) system RapidPlan in the Eclipse treatment planning system (TPS) (Varian Medical Systems, Palo Alto, USA) [Citation1,Citation9,Citation13,Citation24,Citation28] and the template-based planning system Auto-planning in the Pinnacle TPS (Philips Radiation Oncology Systems, Fitchburg, WI) [Citation12,Citation15,Citation16]. Lexicographic optimization was first implemented in Erasmus-iCycle [Citation14,Citation30], but is now combined with a priori multicriterial optimization (MCO) and implemented in the research version of the Monaco TPS (Elekta AB, Stockholm, Sweden), called mCycle [Citation31]. In addition, some in-house methods using scripting and deep learning (DL) are described in the literature [Citation11,Citation17–23].
This retrospective planning study evaluates fully automated script-based treatment plans generated in the RayStation TPS (RaySearch Laboratories AB, Stockholm, Sweden) for LACC patients with up to three dose levels. The automatic plans are compared to the manually generated clinical plans: Quantitatively, relevant dose statistics are compared, and average dose volume histograms (DVHs) are analyzed. Qualitatively, a blinded pairwise comparison of the two plans is conducted. In addition, the accuracy of treatment plan delivery is assessed. The ambition was clinical implementation of the automated script.
Material and methods
In this retrospective planning study, we included 25 consecutive patients with LACC treated at our institution in 2020 and 2021, excluding patients with inguinal node involvement. All patients received 45 Gy to primary and elective pelvic target volumes in 25 fractions. In addition, 17 of the patients received a simultaneously integrated boost (SIB) to 1–6 pathological lymph nodes (LNs) of either 55 Gy (n = 7) or 57.5 Gy (n = 7), or both (n = 3). All patients also received brachytherapy and chemotherapy according to national guidelines by the Norwegian Directorate of Health [Citation32]. This study includes no interventions for the patients included and was determined to be exempt from approval by the Regional Committees for Medical Research Ethics in Central Norway (2015/32).
Computed tomography (CT) simulations were done on a Brilliance CT BigBore (Philips Medical Systems, Cleveland, USA) with patients in a head-first supine position with a slice thickness of 3 mm and in-plane pixel size of 1 mm x 1 mm. The patients were scanned with comfortably filled bladders.
Gross target volume (GTV) and clinical target volume (CTV) delineations were done according to national guidelines [Citation32] and the study protocol of External beam radiochemotherapy and Magnetic Resonance Imaging (MRI)-based adaptive BRAchyterapy in locally advanced CErvical cancer (EMBRACE)-II [Citation33]. The pelvic planning target volume (PTV) and nodal PTVs (PTVns) were generated by an eight-millimeter uniform expansion from corresponding CTVs. The primary tumor PTV was created with individual anisotropic margins between 10 and 20 mm, with estimated margins for internal target volume (ITV) included. PTV volumes are reported in . The notation: target volume, TV_x, indicates a prescribed dose x to the corresponding volume. OAR delineations were performed according to national guidelines and the EMBRACE-II protocol [Citation32,Citation33]. The following OARs were delineated for the clinical treatments: rectum, bladder, bowel, femoral heads, and body. The sigmoid was only contoured in 14 patients, and the spinal cord and kidneys were delineated in seven and eight of the patients, respectively, where the target volume was nearby.
Table 1. Patient population, target volumes. PTVn is a common term for LNs to 55 and 57.5 Gy, it is the total PTVn if multiple nodes are present.
Radiotherapy treatment planning was performed in RayStation using 356 degrees 6 MV VMAT arcs. The dose calculation algorithm employed was Collapsed Cone v5.5, with a dose grid resolution of 3 mm x 3 mm x 3 mm and a maximum gantry spacing of 2 degrees.
All clinical treatment plans were generated according to local guidelines adapted from the EMBRACE-II study protocol [Citation33] in RayStation 9B. These manual VMAT plans were optimized following standard clinical practice, and templates were used for setting up initial optimization objectives. Out of the 25 clinical plans, 7 had two VMAT arcs, while the remaining 18 were created with one arc.
The automatic plans were created using an in-house developed Python script within the scripting environment of RayStation 11 A. The plan optimization strategy consisted of two phases: firstly, minimizing the dose to relevant OARs, and secondly, securing target coverage, see . In the first phase, doses to individual OARs were evaluated and optimization objective doses were adjusted accordingly after each round of optimization. This way, the OAR doses were lowered as much as possible for each patient. In the second phase, target coverage was assured by increasing the weight of the minimum dose objectives by a suitable factor based on how far the current dose distribution was from reaching the target volume coverage goal. Also, hot spot isodose volumes were created and included in the optimization, to maintain an acceptable maximum dose. Cropped OAR volumes were created for the body, bowel, rectum, bladder, and sigmoid, where PTVn was excluded with a 15 mm margin. A cropped PTV_45 was also created, excluding PTVn with a 10 mm margin. All automatic plans were created with two arcs, using the dual arc feature. The script made complete treatment plans; no manual intervention was performed after optimization. These automatic plans were generated according to the constraints summarized in Appendix A, which were based on national guidelines [Citation32] and the updated EMBRACE-II dose constraints [Citation34]. The time spent by the script to generate complete treatment plans was recorded.
Quantitatively, the automatic and clinical plans were compared by evaluating dose statistics for target volumes and given OARs. Average DVHs were calculated for relevant OARs. Conformity indices (CIs) defined as =
=
, and
=
were calculated. Here, PTV refers to PTV_45 and
refers to 90% of the prescribed dose to PTVn. The
was cropped to exclude isodose volumes ”belonging” to other lymph node targets, i.e., closer than 30 mm to another target volume with an equal or higher prescribed dose.
Qualitatively, a blinded plan comparison was conducted between the clinical and automatic plans. Two oncologists with more than ten years of experience each and three medical physicists with a minimum of five years of experience participated. Based on a visual assessment of the dose distribution and fulfillment of clinical goals, the participants were asked to select the preferred plan or state that the two plans had equal quality.
The accuracy of treatment plan delivery was assessed by measuring all plans with a Delta4 Phantom+ (ScandiDos, Uppsala, Sweden) and by using gamma analysis (3%/3 mm). All plans were delivered by an Elekta Versa HD linac (Elekta, Stockholm, Sweden), and run consecutively without moving the phantom between measurements. In addition, the number of monitor units (MUs) in the two plans was compared.
Wilcoxon signed-rank test was used to test the paired difference between the automatic and clinical plans, with a significance level of 5%. The median of the pairwise differences over all patients was calculated for each parameter. Average DVHs were calculated for the bowel, sigmoid, bladder, rectum, kidneys, and body by taking the average over all patients for each dose level. Finally, the Student T-test was used to test the paired difference between the number of MU, pass rate, and maximum gamma-index for the two plans, with a significance level of 5%.
The SQUIRE reporting guidelines [Citation35] were used and a completed checklist was provided as supplementary material.
Results
The time spent creating the clinical plans was not recorded but was typically in the range of a few hours to a whole working day. Complete automatic treatment plans were made in 30–45 min. Treatment planning without SIB was faster than with SIB as a general rule for both types of plans.
The quantitative evaluation showed that target coverage was acceptable for all the automatic and clinical plans (). For patients with pathological LNs, the maximum dose to the cropped PTV_45 exceeds the EMBRACE-II constraint ( 107%) in 6 out of 17 patients in the clinical plans, but the constraint was fulfilled in the automatic plans. The automatic plans were significantly more conformal than the clinical plans; median
of 1.03 vs. 1.12.
Table 2. Dose statistics for target volumes. CTVn and PTVn are common terms for lymph nodes to 55 and 57.5 Gy. All values are normalized to prescribed dose levels (45, 55 and 57.5 Gy). is defined as dose to 0.1 % volume and
is defined as dose to 99.9% volume. For patients with SIB, maximum doses are given in regions 10 mm outside PTVn. The
isodose was cropped to exclude isodose volumes closer than 30 mm to another target volume with an equal or higher prescribed dose. Diff. refers to the median of the pairwise differences between the automatic and clinical plans (Diff.
0 if automatic
clinical). Corresponding mean values are given in Appendix B.
Mean doses to all OARs, except the kidneys, were significantly lower in the automatic plans vs. the clinical plans. The median overall reduction ranged from 0.6 Gy for the body to 1.9 Gy for the bowel, while for the left and right kidney, as the only exception, automatic planning resulted in 1 and 0.6 Gy higher mean doses than the clinical plans, respectively. For the bowel, automatic planning reduced the median and
by 29.2
and 61.4
respectively, compared to the clinical plans. All automatic plans had a maximum dose to the bowel, rectum, bladder, and sigmoid below the EMBRACE-II limit of 105%; this was not the case for the clinical plans ().
The average DVHs in show that the automatic plans have lower doses than the clinical plans in the 10–40 Gy dose region for the bowel and bladder. Also, the doses are lower in the automatic plans for the sigmoid, rectum, and body, but the difference is not as large.
Table 3. Dose statistics for OARs. For patients with positive LNs, maximum doses are given in regions 15 mm outside PTVn. is defined as dose to 0.1
of the volume, except for body where
is dose to 0.03
of the volume. All maximum doses (%) are normalized to 45 Gy. Diff. refers to the median of the pairwise differences between the automatic and clinical plans (Diff.
0 if automatic
clinical). Corresponding mean values are given in Appendix B.
In the qualitative evaluation, the blinded plan comparison showed that the automatic plans were selected in 98/125 (78%) of cases, the clinical plans were selected in 1/125 (1%) of cases, and the two plans were judged to be of equal quality in 26/125 (21%) of cases (). Generally, the reasons for choosing the automatic plans were better conformity, lower OAR doses, especially for the bowel, and less hot spots in PTV_45 outside the positive LNs.
Figure 3. Preferred plans in the blinded comparison between the automatic and clinical plans for all 25 patients. The number of LNs and dose prescriptions level for each patient are reported.
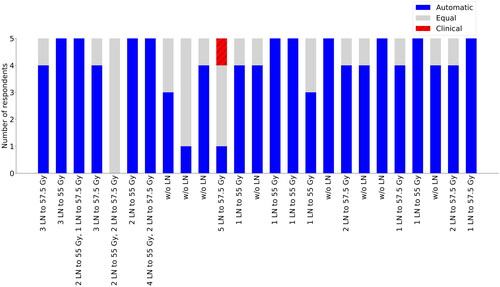
Dose distributions of two representative patients are shown in , illustrating that the automatic plans have more conformal dose distributions for all isodose levels.
Figure 4. Automatic vs. clinical plans for two different representative patients in (a), (b) and (c), (d). Isodose lines are dark yellow for 55 Gy, dark green for 49.5 Gy, orange for 47.3 Gy, yellow for 45 Gy, green for 42.75 Gy, white for 27.5 Gy, and sea green for 16.5 Gy. PTV is outlined in blue, CTV in pink and GTV in red. The bowel is dark green, the sigmoid is orange, and the rectum is brown.
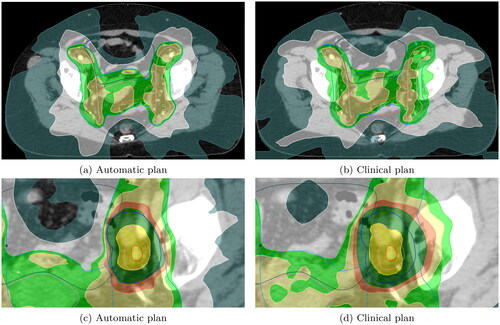
Despite the significantly higher mean number of MUs in the automatic plans, the gamma pass rate (3%/3 mm) for treatment plan delivery was nearly identical in the two types of plans ().
Table 4. Number of MU, gamma passing rate (3%/3 mm) with 20%–500% local dose, and maximum gamma-index.
Discussion
Automatic planning based on scripting for LACC patients has shown to be superior to manual planning in most aspects; the automatic plans had reduced OAR doses, better conformity, and were predominantly preferred by the clinicians.
The automatic plans created in this study satisfy the high standard of the EMBRACE-II study, as our results align closely with the updated EMBRACE-II dose constraints [Citation34]. In particular, the EMBRACE-II soft constraints for conformity of = 1.10 and
= 1.55, were similar or improved in our study, with medians of 1.03 and 1.56, respectively, for pelvic targets and pelvic targets with para-aortic nodes (). Our results are also similar to the results by Berger et al. [Citation5], which reported
of 1.02 and 1.03 for pelvic targets and pelvic targets with para-aortic nodes, respectively, in the early phase of inclusion of the EMBRACE-II study.
Our clinical practice has some minor differences from the national guidelines [Citation32] and EMBRACE-II study protocol [Citation33]; the ITV margins were included in the PTV margins. Thus, the PTV margins appear to be larger. Therefore, we apply 95% to CTV_45 instead of ITV_45. However, the PTV volumes should be comparable [Citation5]. While the EMBRACE-II study protocol has a goal of
95%, we have chosen to follow the national guidelines and used
95% for PTV_45 as a planning goal. In addition, the updated version of the EMBRACE-II dose constraints specified that maximum doses to the sigmoid, bladder, rectum, and bowel should be evaluated in regions 10–15 mm from PTVn [Citation34]. This is why these criteria are prioritized and fulfilled for the automatic plans but not necessarily for the clinical plans. Another potential issue with this study is that the sigmoid was not always a part of the optimization as it was not delineated for all patients. The number of patients with kidneys and spinal cord delineated is also low, reflecting the number of patients with target volumes close to these organs. Hence, the statistical analysis is more uncertain for these organs.
Compared to the automatic plans, the only OARs with lower mean doses in the clinical plans were the left and right kidneys. In our clinical practice, the kidneys are often highly prioritized, as they are important dose-limiting organs [Citation36]. We have chosen to relax the priority of the mean dose to kidneys in the automatic plans compared to previous clinical practice because it allowed for a significant reduction in bowel doses. However, both the hard constraint and the soft constraint (Table A1) for kidneys were met for all plans. It could be hypothesized that an even more significant reduction in bowel doses could be achieved if the kidney mean dose constraint had been further relaxed.
This is the first paper on fully automated script-based VMAT planning in RayStation for cervical cancer patients with up to three dose levels. However, there are several examples in the literature of successful automatic planning. Sharfo et al. [Citation14] created fully automated VMAT plans with one dose level using the independent system Erasmus-iCycle for cervical cancer patients. Trivellato et al. [Citation31] validated a further developed version, called mCycle, in the research version of Monaco for cervical cancer patients with one dose level. In addition, several papers have evaluated the Auto-planning module in Pinnacle for different treatment sites, for example, Wortel et al. [Citation37], Hazell et al. [Citation15], Gallio et al. [Citation16], and Hansen et al. [Citation12]. In the Auto-planning module, iterative optimization is performed based on a template that contains optimization goals for targets and OARs in addition to advanced settings. Wortel et al. [Citation37] investigated how to configure automatic planning protocols for multiple sites. It was found impossible to spare OARs optimally with a single common optimization goal for all patients, and reaching sufficient target coverage was challenging. Their solution was to use several advanced settings, such as a separate goal to the outer 2 mm of the PTV to reach target coverage. The automatic plans by Trivellato et al. and Wortel et al. were chosen over manually made plans in more than 75% and 80% of cases, respectively, in blind tests. Further, in Hazell et al. [Citation15], the automatic plans scored at least as high as the clinical plans in 94% of cases in a blinded evaluation. Not including optimization time, 4 min of effective working time was spent to create Hazell et al.’s plans, so the total planning time is unknown. In Hansen et al. [Citation12], the automatic plans were selected for treatment in a blinded comparison in 29 of 30 cases, and it was stated that the manual time spent on planning was reduced by a factor of two. Sharfo et al. [Citation14] proposed that automated planning holds a strong promise for treatment plan improvement for LACC patients with positive pelvic or para-aortic nodes. The script-based automatic planning in our study clearly demonstrates this. Another advantage of our approach is that this script is free of charge and can be used within the environment of a commercial TPS. In this study, our approach to reaching target coverage was to use a simple formula to calculate the difference between the desired PTV coverage and the current coverage to increase the PTV minimum dose objective weight. In the blinded evaluation, the clinicians preferred our automatic plans or judged them to be of equal quality to the clinical plans in 99% of cases. Experience from the clinic indicates that the manual planning time was in the range of a few hours to a whole working day. Hence, our script-based approach with a total planning time of 30–45 min reduces the planning time by a factor of four to ten compared to manual planning.
In the literature, there are only a few examples of script-based planning. Lou et al. [Citation27] have created a script in RayStation that made automated intensity-modulated radiation therapy (IMRT) or VMAT plans for nasopharyngeal carcinoma (NPC), esophageal carcinoma, and rectal cancer. The NPC cases had three dose levels, while the other two sites had one dose level. As in the present study, they found that the automatic plans were similar to or better than the manual plans, and the planning times were shorter; the average planning time was reduced by more than 43%. Further, Rigaud et al. [Citation20] investigated DL segmentation models for cervical cancer for online adaptive treatments. Scripting in RayStation was used to generate IMRT plans with one dose level to evaluate the dosimetric uncertainties of the DL models. The IMRT plans were generated using manual objective function weights, meaning that the plans were not fully automatic. DL segmentations (except nodal CTVs) were computed, and IMRT dose optimization was performed in less than 6 min. Boylan et al. [Citation18] used scripts to compare IMRT and VMAT for NPC planning. Contrary to our script, the planning time was long (7 h), the plans were not clinically accepted, and their focus was primarily on minimizing parotid dose rather than addressing other OARs. Furthermore, Xhaferllari et al. [Citation19] described an IMRT planning script for multiple sites. In contrast to our script, only the target volume doses were adjusted iteratively by Xhaferllari’s script, not the OAR doses, and the resulting plans were not clinically acceptable but had to be manually improved by a dosimetrist. The scripts used by Lou et al., Rigaud et al., Boylan et al., and Xhaferllari et al. are all similar to our script in many ways. However, in our study, all relevant OARs were addressed by setting the OAR objective dose to 0.7 of the current dose in multiple iterations, a method that results in optimal individual OAR sparing. The automatic plans created in this study were clinically acceptable and did not need manual adjustment, but it is possible if desired. Our planning script was developed specifically for LACC patients. However, it was built on the experience from similar scripts already implemented in our clinic for breast [Citation38] and prostate cancer patients. This type of automatic planning has also recently been implemented for head and neck cancer in our clinic. Therefore, automatic planning scripts can presumably be successfully implemented for most diagnoses with some adaption.
Knowledge-based planning is another method for automatic or semi-automatic treatment planning, where machine learning (ML) models trained on previous plans are used to make dose predictions for new plans [Citation1]. Several authors have used the commercial KBP software RapidPlan from Varian to produce IMRT/VMAT plans for cervical cancer patients [Citation9,Citation13,Citation28]. After some changes to the initial KBP model, Hussein et al. [Citation13] produced clinically acceptable cervical cancer plans for ten test patients. Further, Shepherd et al. [Citation9] developed treatment plans for 12 gynecologic patients with up to three dose levels, where 32 patients were used for model training. The plan optimization time was about 25 min, and 50% of the KBP plans were selected as superior to the original clinically treated plan. It should be noted that KBP is often only semi-automatic, as the plans generally require some manual fine-tuning before being clinically acceptable. Some hybrid approaches incorporate KBP or DL to obtain planning objectives for the Auto-planning module in Pinnacle. For example, Ling et al. [Citation26] used RapidPlan, Jihong et al. [Citation11] utilized a DL model, and Zhang et al. [Citation29] and Bai et al. [Citation25] used anatomical features to make predictions. The planning process took about an hour in Ling et al. and less than 10 min in the other three cases. Jihong et al. [Citation11] had a total of 140 patients included in their study. An oncologist preferred the DL automatic plans in 70% of cases. The plans of Hussein et al., Zhang et al., and Jihong et al. all had one dose level, and one could argue that it is considerably easier to generate plans with one dose level compared to those with two or three dose levels, as in the present study. KBP and DL require an extensive training set of high-quality treatment plans, and the plans the model produces will not be better than those the model was based on. This is opposed to the script-based plans in the present study, where a high-quality dose distribution with individual OARs sparing is achieved without the knowledge of previous plans. Using DL, the planning time is shorter compared with our script-based approach. There is, however, a potential to speed up the scripted planning process to be used for online adaptive treatments. As the script automatically makes plans of high and homogeneous quality, a possible future application of the script could be to create training data for a DL planning model.
Conclusion
We have developed a fully automated script that generates VMAT plans for LACC patients with up to three dose levels that are better than, or at least as good as, clinically accepted plans. The automatic plans had lower doses to almost all OARs, more conformal dose distributions, and were predominantly preferred by the clinicians. In addition, the plan delivery was excellent, with an accuracy on the same level as the clinical plans. Complete script-based treatment plans were created in 30 to 45 min, about four to ten times faster than manually made plans. Based on these results, our institution has implemented the script for clinical use.
Supplemental Material
Download MS Word (22.8 KB)Disclosure statement
No potential conflict of interest was reported by the author(s).
Data availability statement
Raw data were generated at St Olavs Hospital, Norway. Derived data supporting the findings of this study are available from the corresponding author MF on request.
References
- Yusufaly TI, Meyers SM, Mell LK, et al. Knowledge-based planning for intact cervical cancer. In: Seminars in radiation oncology. Vol. 30; Elsevier; 2020. p. 328–339. doi: 10.1016/j.semradonc.2020.05.009.
- Jensen NBK, Pötter R, Kirchheiner K, et al. Bowel morbidity following radiochemotherapy and image-guided adaptive brachytherapy for cervical cancer: physician-and patient reported outcome from the EMBRACE study. Radiother Oncol. 2018;127(3):431–439. doi: 10.1016/j.radonc.2018.05.016.
- Spampinato S, Fokdal LU, Pötter R, et al. Risk factors and dose-effects for bladder fistula, bleeding, and cystitis after radiotherapy with imaged-guided adaptive brachytherapy for cervical cancer: an EMBRACE analysis. Radiother Oncol. 2021;158:312–320. doi: 10.1016/j.radonc.2021.01.019.
- Westerveld H, Kirchheiner K, Nout RA, et al. Dose-effect relationship between vaginal dose points and vaginal stenosis in cervical cancer: an EMBRACE-I sub-study. Radiother Oncol. 2022;168:8–15. doi: 10.1016/j.radonc.2021.12.034.
- Berger T, Seppenwoolde Y, Pötter R, et al. Importance of technique, target selection, contouring, dose prescription, and dose-planning in external beam radiation therapy for cervical cancer: evolution of practice from EMBRACE-I to II. Int J Radiat Oncol Biol Phys. 2019;104(4):885–894. doi: 10.1016/j.ijrobp.2019.03.020.
- Gandhi AK, Sharma DN, Rath GK, et al. Early clinical outcomes and toxicity of intensity modulated versus conventional pelvic radiation therapy for locally advanced cervix carcinoma: a prospective randomized study. Int J Radiat Oncol Biol Phys. 2013;87(3):542–548. doi: 10.1016/j.ijrobp.2013.06.2059.
- Klopp A, Yeung A, Deshmukh S, et al. A phase III randomized trial comparing patient-reported toxicity and quality of life (QOL) during pelvic intensity modulated radiation therapy as compared to conventional radiation therapy. Int J Radiat Oncol Biol Phys. 2016;96(2):S3. doi: 10.1016/j.ijrobp.2016.06.024.
- Ray A, Sarkar B. Small bowel toxicity in pelvic radiotherapy for postoperative gynecological cancer: comparison between conformal radiotherapy and intensity modulated radiotherapy. Asia Pac J Clin Oncol. 2013;9(3):280–284. doi: 10.1111/ajco.12049.
- Shepherd M, Bromley R, Stevens M, et al. Developing knowledge-based planning for gynecological and rectal cancers: clinical validation of RapidPlan™. J Med Radiat Sci. 2020;67(3):217–224. doi: 10.1002/jmrs.396.
- Wang H, Wang R, Liu J, et al. Tree-based exploration of the optimization objectives for automatic cervical cancer IMRT treatment planning. Br J Radiol. 2021;94(1123):20210214. doi: 10.1259/bjr.20210214.
- Jihong C, Penggang B, Xiuchun Z, et al. Automated intensity modulated radiation therapy treatment planning for cervical cancer based on convolution neural network. Technol Cancer Res Treat. 2020;19:1533033820957002. doi: 10.1177/1533033820957002.
- Hansen CR, Bertelsen A, Hazell I, et al. Automatic treatment planning improves the clinical quality of head and neck cancer treatment plans. Clin Transl Radiat Oncol. 2016;1:2–8. doi: 10.1016/j.ctro.2016.08.001.
- Hussein M, South CP, Barry MA, et al. Clinical validation and benchmarking of knowledge-based IMRT and VMAT treatment planning in pelvic anatomy. Radiother Oncol. 2016;120(3):473–479. doi: 10.1016/j.radonc.2016.06.022.
- Sharfo AWM, Breedveld S, Voet PW, et al. Validation of fully automated VMAT plan generation for library-based plan-of-the-day cervical cancer radiotherapy. PLOS One. 2016;11(12):e0169202. doi: 10.1371/journal.pone.0169202.
- Hazell I, Bzdusek K, Kumar P, et al. Automatic planning of head and neck treatment plans. J Appl Clin Med Phys. 2016;17(1):272–282. doi: 10.1120/jacmp.v17i1.5901.
- Gallio E, Giglioli FR, Girardi A, et al. Evaluation of a commercial automatic treatment planning system for liver stereotactic body radiation therapy treatments. Phys Med. 2018;46:153–159. doi: 10.1016/j.ejmp.2018.01.016.
- Breedveld S, Storchi PR, Voet PW, et al. iCycle: integrated, multicriterial beam angle, and profile optimization for generation of coplanar and noncoplanar IMRT plans. Med Phys. 2012;39(2):951–963. doi: 10.1118/1.3676689.
- Boylan C, Rowbottom C. A bias-free, automated planning tool for technique comparison in radiotherapy-application to nasopharyngeal carcinoma treatments. J Appl Clin Med Phys. 2014;15(1):4530–4225. doi: 10.1120/jacmp.v15i1.4530.
- Xhaferllari I, Wong E, Bzdusek K, et al. Automated IMRT planning with regional optimization using planning scripts. J Appl Clin Med Phys. 2013;14(1):4052–4191. doi: 10.1120/jacmp.v14i1.4052.
- Rigaud B, Anderson BM, Zhiqian HY, et al. Automatic segmentation using deep learning to enable online dose optimization during adaptive radiation therapy of cervical cancer. Int J Radiat Oncol Biol Phys. 2021;109(4):1096–1110. doi: 10.1016/j.ijrobp.2020.10.038.
- McIntosh C, Welch M, McNiven A, et al. Fully automated treatment planning for head and neck radiotherapy using a voxel-based dose prediction and dose mimicking method. Phys Med Biol. 2017;62(15):5926–5944. doi: 10.1088/1361-6560/aa71f8.
- Ayala R, Ruiz G, Valdivielso T. Automatizing a nonscripting TPS for optimizing clinical workflow and reoptimizing IMRT/VMAT plans. Med Dosim. 2019;44(4):409–414. doi: 10.1016/j.meddos.2019.02.006.
- Rhee DJ, Jhingran A, Kisling K, et al. Automated radiation treatment planning for cervical cancer. In: seminars in radiation oncology. Vol. 30; Elsevier; 2020. p. 340–347. doi: 10.1016/j.semradonc.2020.05.006.
- Fogliata A, Belosi F, Clivio A, et al. On the pre-clinical validation of a commercial model-based optimisation engine: application to volumetric modulated arc therapy for patients with lung or prostate cancer. Radiother Oncol. 2014;113(3):385–391. doi: 10.1016/j.radonc.2014.11.009.
- Bai P, Weng X, Quan K, et al. A knowledge-based intensity-modulated radiation therapy treatment planning technique for locally advanced nasopharyngeal carcinoma radiotherapy. Radiat Oncol. 2020;15(1):1–10. doi: 10.1186/s13014-020-01626-z.
- Ling C, Han X, Zhai P, et al. A hybrid automated treatment planning solution for esophageal cancer. Radiat Oncol. 2019;14(1):1–7. doi: 10.1186/s13014-019-1443-5.
- Lou Z, Cheng C, Mao R, et al. A novel automated planning approach for multi-anatomical sites cancer in raystation treatment planning system. Phys Med. 2023;109:102586. doi: 10.1016/j.ejmp.2023.102586.
- Ma C, Huang F. Assessment of a knowledge-based RapidPlan model for patients with postoperative cervical cancer. Prec. Radiat. Oncol. 2017;1(3):102–107. doi: 10.1002/pro6.23.
- Zhang D, Yuan Z, Hu P, et al. Automatic treatment planning for cervical cancer radiation therapy using direct three-dimensional patient anatomy match. J Appl Clin Med Phys. 2022;23(8):e13649. doi: 10.1002/acm2.13649.
- Bijman R, Sharfo AW, Rossi L, et al. Pre-clinical validation of a novel system for fully-automated treatment planning. Radiother Oncol. 2021;158:253–261. doi: 10.1016/j.radonc.2021.03.003.
- Trivellato S, Caricato P, Pellegrini R, et al. Comprehensive dosimetric and clinical evaluation of lexicographic optimization-based planning for cervical cancer. Front Oncol. 2022;12:1041839. doi: 10.3389/fonc.2022.1041839.
- Norwegian Directorate of Health. Nasjonalt handlingsprogram med retningslinjer for diagnostikk, behandling og oppfølging av gynekologisk kreft [nettdokument], faglig oppdatert 11. 2023, https://www.helsedirektoratet.no/retningslinjer/gynekologisk-kreft-handlingsprogram.]; 2016.
- Tanderup K, Pötter R, Lindegaard J, et al. EMBRACE II study protocol v.1.0, Image guided intensity modulated External beam radiochemotherapy and MRI based adaptive BRAchytherapy in locally advanced CErvical cancer EMBRACE-II (retrieved 28.04.2023) [https://www.embracestudy.dk/UserUpload/PublicDocuments/EMBRACE\%20II\%20Protocol.pdf]; 2015.
- Pötter R, Tanderup K, Kirisits C, et al. The EMBRACE II study: the outcome and prospect of two decades of evolution within the GEC-ESTRO GYN working group and the EMBRACE studies. Clin Transl Radiat Oncol. 2018;9:48–60. doi: 10.1016/j.ctro.2018.01.001.
- Ogrinc G, Davies L, Goodman D, et al. Squire 2.0 (standards for quality improvement reporting excellence): revised publication guidelines from a detailed consensus process [https://www.equator-network.org/reporting-guidelines/squire/.]; 2015.
- Dawson LA, Kavanagh BD, Paulino AC, et al. Radiation-associated kidney injury. Int J Radiat Oncol Biol Phys. 2010;76(3 Suppl):S108–S115. doi: 10.1016/j.ijrobp.2009.02.089.
- Wortel G, Eekhout D, Lamers E, et al. Characterization of automatic treatment planning approaches in radiotherapy. Phys Imaging Radiat Oncol. 2021;19:60–65. doi: 10.1016/j.phro.2021.07.003.
- Frengen J, Vikström J, Mjaaland I, et al. Locoregional breast radiotherapy including IMN: optimizing the dose distribution using an automated non-coplanar VMAT-technique. Acta Oncol. 2023. doi: 10.1080/0284186X.2023.2264488.
Appendices
Appendix A.
Dose constraints
Table A1. Dose constraints used in this study. Adapted from national guidelines and the EMBRACE II study [Citation32, Citation34]. CTVn/PTVn is a common term for lymph nodes to 55 and 57.5 Gy. All values are normalized to the prescribed dose level (45, 55, and 57.5 Gy). = dose to 0.1
of the volume, except for body where
= dose to 0.03
of the volume. All maximum doses to OARs (%) are normalized to 45 Gy.
Appendix B.
Mean doses to targets and OARs
Table B1. Dose statistics for target volumes. CTVn/PTVn is a common term for lymph nodes to 55 and 57.5 Gy. All values are normalized to prescribed dose levels (45, 55, and 57.5 Gy). is defined as dose to 0.03
volume and
is defined as dose to 99.9% volume. The
isodose was cropped to exclude isodose volumes closer than 30 mm to another target volume with an equal or higher prescribed dose. Diff. refers to the mean pairwise differences between the automatic and clinical plans (Diff.
0 if automatic
clinical).
Table B2. Dose statistics for OARs. For patients with positive LNs, maximum doses are given in regions 15 mm outside PTVn. is defined as dose to 0.1
of the volume, except for body where
is dose to 0.03
of the volume. All maximum doses (%) are normalized to 45 Gy. Diff. refers to the mean pairwise differences between the automatic and clinical plans (Diff.
0 if automatic
clinical).