Abstract
Objective: To evaluate health care use and outcomes among patients who experienced a non-medical switch of their prescribed anti-tumor-necrosis-factor biological agent (anti-TNF) for cost containment reasons.
Methods: Retrospective evaluation of Humedica electronic health records of patients ≥18 years old with anti-TNF treatment for immune conditions. Using natural language processing, stable patients who experienced a non-medical switch (for cost reasons) of their anti-TNF between 2007 and 2013 were identified (NMS cohort, n = 158) and matched to patients who did not (control cohort, n = 4804). Rates of office visits, emergency department visits, and hospitalizations at 30, 90, and 365 days following were evaluated. Medication-related adverse events, defined as subsequent medication change due to a side effect and/or efficacy-related reason were also compared.
Results: Adjusted rates of office visits were higher among the NMS cohort than the control cohort at 30 (46.4% vs. 31.7%, p < .001), 90 (71.0% vs. 57.0%, p < .001), and 365 days (87.8% vs. 76.8%, p < .001). Rates of emergency department use and hospitalization were comparable between cohorts. The NMS cohort had higher adjusted rates of medication-related adverse consequences (both increased side effects and diminished efficacy) than the control cohort at 30 (13.8% vs. 4.0%, p = .003), 90 (31.6% vs 9.6%, p < .001), and 365 days (54.7% vs. 20.3%, p < .001). Compared with controls, the NMS cohort had higher adjusted rates of subsequent medication change within 1 year (27.82% vs. 13.9%, p = .001).
Conclusion: Non-medical switching among patients prescribed anti-TNFs was associated with increased health care use, medication-related side effects, and reports of diminished efficacy.
Introduction
Switching among medications is a common practice when patients do not adequately respond to therapy or experience an adverse eventCitation1. Non-medical switching or changing medications for reasons other than efficacy, safety, or tolerability may also occur. For instance, a non-medical switch may be driven by out-of-pocket cost differentials, availability of alternative formulations (such as those that avoid infusions), or preferences for a more convenient dosing frequency. Additionally, non-medical switching may be payer-driven, such as by a pharmacy benefits manager seeking to reduce costsCitation1.
Anti-tumor necrosis factor (TNF) biological agents are used to treat immune-mediated inflammatory diseasesCitation2,Citation3. Recent studies have shown that early referral to a rheumatologist leads to more timely diagnosis of rheumatic diseaseCitation4 and that treatment with biological agents results in clinical remissionCitation5–11, reduced risk of extra-articular complicationsCitation12, and improved quality of lifeCitation2,Citation3,Citation13–17 for patients with autoimmune disorders. A small number of studies have examined the impact of non-medical switching of anti-TNF biological agents on patients and the results reported have been variable. Two of these studiesCitation18,Citation19 demonstrated that patients generally tolerate non-medical switching; however, these studies assessed a single disease condition, studied non-US populations, and were limited by data obtained from single centers. In contrast, a third studyCitation20 evaluating the effect of non-medical switching of anti-TNF biological agents found that switching from one anti-TNF biological agent to another for non-medical reasons was associated with loss of tolerance and efficacy within 1 year after the switch. In a fourth studyCitation21, non-medical switching of anti-TNF biological agents was associated with higher health care costs, although the impact of non-medical switching on clinical outcomes was not assessed. A fifth study that evaluated non-medical switching of patients with Crohn’s disease maintained on infliximab or adalimumab and who switched to certolizumab pegol found 25% of patients failed substitutionCitation22. Another recent study evaluating patient outcomes in clinical practice found that patients who switched or discontinued from an anti-TNF therapy for non-medical reasons had more frequent flares, lower rates of well controlled symptoms, and more frequent hospitalizations, emergency department visits, and outpatient visits than those who continued with their anti-TNF therapyCitation23. Finally, physicians, pharmacists, and nurses surveyed regarding treatment of Medicare Part D beneficiaries raised concerns of diminished efficacy following non-medical switchingCitation24. Additional studies assessing both clinical outcomes and health care use using real-world data are needed to understand the full effect of non-medical switching of anti-TNF biological agents.
Non-medical switching may be particularly consequential in the case of biological therapies. Implicit in non-medical switching is the assumption that agents within this therapeutic class would provide similar safety and efficacy. This expectation poses a particular challenge for available biological therapies, which differ in structure, pharmacokinetics, bioavailability, binding specificity, and immunogenicity resulting in varied responses by patients to different agentsCitation1.
To better characterize this hypothesis, the impact of non-medical switching on patients receiving anti-TNF biological therapy was evaluated using electronic medical record (EMR) data from the US to identify patients who switched anti-TNF biological agents for reasons related to cost and not due to side effects or efficacy concerns. Specifically, the effects of non-medical switching on medication-related adverse events, efficacy, health care use, and further switches in patients receiving anti-TNF biological therapy were assessed.
Methods
Data source
The present study used de-identified electronic medical record (EMR) data from Humedica, which was gathered from more than 20 medical provider organizations (medical groups, integrated delivery systems [networks of health care organizations under common ownership], hospitals) from around the US and covered the period from 2007 through 2013. This network of provider organizations had approximately 30 million patients across 38 states with broad geographic representation. The patient population included those insured by commercial insurance, Medicare, or Medicaid, as well as uninsured patients. EMR data was aggregated directly from the providers and, once aggregated, data was checked and standardized. This data included de-identified information on demographics, diagnoses, inpatient and outpatient encounters, medications, procedures, laboratory results, and vital signs. Humedica’s proprietary natural language processing (NLP)Citation25 techniques systematically searched unstructured data, such as physicians’ notes captured in the EMR, for terms related to specific medications involving “side effects“, “efficacy“, or “cost”. The NLP system used vocabulary from the Unified Medical Language System, which includes multiple medication dictionaries, such as the Logical Observation Identifiers Names and Codes, the Systemized Nomenclature of Medicine–Clinical Terms, and RxNorm, a listing of generic and branded drugs to conduct a context-sensitive search for specific terms and modifiers contained within the EMR. For the present study, a validation analysis was performed to validate the NLP algorithm. Results of this sensitivity analysis revealed a cross-validation accuracy of >95% (see Supplementary Online Appendix 1).
All data used in the analyses described in this study was de-identified and complied with Health Insurance Portability and Accountability Act (HIPAA) regulations. Because this data was completely de-identified, institutional review board approval of this study was not required.
Selection of initial cohort
Patients with at least one diagnosis of rheumatoid arthritis (714.0), Crohn’s disease (555.x), psoriasis (696.1), ulcerative colitis (556.x), psoriatic arthritis (696.0), or ankylosing spondylitis (720.0) were identified from 2007 to 2013 using International Classification of Diseases, Ninth Revision (ICD-9) codes. Of the 439,865 patients identified, 279,332 patients had physician note data extracted with NLP (). Starting with data on 279,332 patients, an initial sample was identified of patients with a non-medical switch (NMS cohort, N = 175) who were at least 18 years old, switched from one anti-TNF biological agent to another within 183 days (i.e., 6 months) of the last prescription for the prior anti-TNF biological agent, and had at least one physician note regarding “cost” associated with their initial anti-TNF biological agent and no physician notes regarding “side effect” or “efficacy”. A parallel sample was also identified of patients without a non-medical switch (control cohort, N = 13,714) who were at least 18 years old, had at least two medication records for the same anti-TNF biological agent, and had no “cost” note associated with an anti-TNF biological agent at any time.
Figure 1. Selection process. (A) NMS cohort. (B) Control cohort. Abbreviations. AS, ankylosing spondylitis; CD, Crohn’s disease; ED, emergency department; NLP, natural language processing; NMS, non-medical switch; Ps, psoriasis; PsA, psoriatic arthritis; RA, rheumatoid arthritis; TNF, tumor necrosis factor; UC, ulcerative colitis.
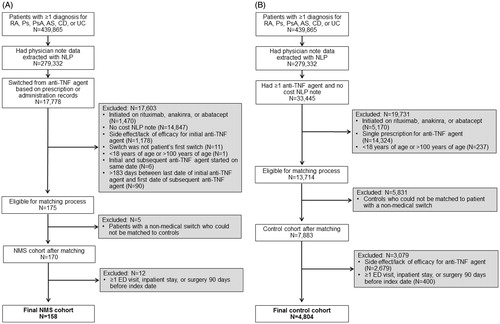
Matching process
To identify a suitable comparison group among control patients without a medication switch, an exact match between a patient with a non-medical switch and a control patient was required based on the following five matching factors: initial anti-TNF biological agent (etanercept, adalimumab, infliximab, certolizumab pegol, golimumab); clinical indication (rheumatoid arthritis, Crohn’s disease, psoriasis, ulcerative colitis, psoriatic arthritis, ankylosing spondylitis, missing); whether the patient’s EMR came from an integrated delivery system (Y/N); patient sex (M/F); and patient age category (18–34, 35–50, 51–64, 65–100 years). Patients with a non-medical switch who failed to match exactly to at least one control candidate (n = 5) and control candidates who failed to match exactly to at least one candidate with a non-medical switch (n = 5831) were excluded. A total of 170 candidates in the NMS cohort and 7883 candidates in the control cohort remained after the matching process using 91 strata (i.e. unique combinations of values for the five matching factors) ().
Assigning an index date
To calculate outcomes after a non-medical switch occurred, it was necessary to establish an index date to anchor the comparison between the NMS and control cohorts. For the NMS cohort, the index date was the date of the earliest medication record when a patient changed to a different anti-TNF biological agent. For the control cohort, the index date was imputed as the date when a switch would have occurred given the timing and duration of a control patient’s observed use of the initial drug and the average time to switch of patients who switched anti-TNF biological agents and were in the same match stratum as predicted by an interval regression model. Further details are provided in Supplementary Online Appendix 2.
Additional selection criteria
With index dates for both the NMS and control cohorts, the two samples were aligned by excluding control patients who had a “side effect” or lack of “efficacy” note associated with their initial anti-TNF biological agent between the start of their initial medication and their index date. To help ensure that the study patients were clinically stable prior to their index dates, patients who had at least one emergency department visit, inpatient stay or outpatient surgery during the 90 day period immediately before their index date were excluded. Finally, from this analytical study cohort three subsets were constructed that corresponded to having EMR coverage for 30 days, 90 days, and 365 days after the index date.
Outcomes
Health care use was assessed by evaluating the proportions of patients with separate endpoints of any ambulatory office or clinic visit, emergency department visit, or hospitalization within 30 days, 90 days, and 365 days following the index date. To evaluate the clinical impact of switching, subsequent physician notes were queried using NLP for documentation related to treatment “side effect“, lack of “efficacy“, or the combination of “side effect or lack of efficacy” within 30 days, 90 days, and 365 days following the index date. To assess the impact on prescribing, subsequent changes to another anti-TNF biological agent were identified within 30 days, 90 days, and 365 days of the index date. Whether this change was attributable to a physician-documented adverse clinical event was determined by using NLP to search for physician notes indicating “side effect” or diminished “efficacy” with the previous anti-TNF biological agent. Analyses for each time period were restricted to those patients who had EMR coverage for that period.
Covariates
Patients were matched based on their unique combinations of five factors (initial anti-TNF biological agent, clinical indication, integrated delivery network, sex, and age category). Additional patient-level covariates included race (White, African American, Asian, other/unknown), US Census region (Northeast, South, Midwest, West, unknown), insurance type (commercial, Medicare, Medicaid, other, uninsured, unknown, missing), estimated median household income and percentage college educated or higher, both at the 5 digit ZIP Code level, and calendar year of index date. (A ZIP Code is a small, contiguous geographic area represented by a single postal code).
Statistical analysis
The distributions of covariates were compared statistically between the NMS and control cohorts using nonparametric two-sample tests of equality of medians and Pearson’s χ2 tests for categorical variables. The effect of stratification was assessed by the five matching factors on covariate balance using linear regression with match strata indicator variablesCitation26.
Linear regression was used to assess the impact of having a non-medical switch versus not having a non-medical switch on each outcome for each of the 30, 90, and 365 day follow-up periods. Models were estimated for unadjusted comparisons, which included no covariates, and for adjusted comparisons, which included a vector of match strata indicator variables and the additional patient-level covariates (race, US Census region, insurance type, ZIP Code-level average household income and percentage college educated, and calendar year of index date). This approach allowed direct comparison between the unadjusted and adjusted effects of non-medical switching while also accommodating the match strata fixed effects, which could result in inconsistent coefficients in nonlinear models such as logistic regressionCitation27. Model standard errors were adjusted to be robust to heteroskedasticity. Analyses were conducted using Stata MP 13.1 (College Station, TX, USA).
Results
The NMS and control cohorts consisted of 158 patients and 4804 patients, respectively. Patients in the NMS and control cohorts were comparable in terms of sex, age, racial composition, US Census region, and ZIP Code-level educational distribution (). Insurance information was missing or unknown for a larger proportion of patients in the NMS cohort compared with controls. Patients in the NMS cohort were drawn disproportionately from more recent years. These differences were eliminated or attenuated after stratification by matching variables (Supplementary Online Appendix 2).
Table 1. Patient characteristics.
The majority of patients were prescribed anti-TNF biological agents for rheumatoid arthritis, with etanercept and adalimumab as the most commonly prescribed agents. Immune disorder prevalence differed between the cohorts such that patients with rheumatoid arthritis were more prevalent among the control group compared with the NMS group, while patients with psoriatic arthritis were more prevalent among the NMS group than the control group. Initial medication use also differed between groups. Patients in the control group had a higher rate of etanercept use, but a lower rate of golimumab use compared with those in the NMS group.
Experiencing a non-medical switch resulted in higher unadjusted proportions of patients with at least one office visit at 30 days (54.3% vs. 31.4%, p < .001), 90 days (82.1% vs. 56.7%, p < .001), and 1 year (98.0% vs. 76.5%, p < .001) (). Differences in the proportion of patients with office visits were partially attenuated with multivariable adjustment, but patients in the NMS group continued to have higher rates of office visits at 30 days (46.4% vs. 31.7%, p < .001), 90 days (71.0% vs. 57.0%, p = .001), and 365 days (87.8% vs. 76.8%), p < .001).
Table 2. Health care use.
The proportions of patients using the emergency department were comparable between the NMS group and control group at 30 days, 90 days, and 365 days (). Additionally, although the share of patients with hospitalization at 30 days was modestly lower in the NMS group than the control group (0.0% vs. 0.9%, p < .001), there was no difference at 90 days (2.1% vs. 1.9%, p = .85) or 365 days (8.1% vs. 6.5%, p = .57). These findings for emergency department use and hospitalization were largely unchanged after multivariable adjustment.
A greater proportion of patients who experienced a non-medical switch had a medication-related adverse consequence (side effect or efficacy related) within 30 days (16.3% vs. 4.0%, p < .001), 90 days (36.4% vs. 9.4%, p < .001), and 365 days (62.6% vs. 20.1%, p < .001) (). Higher rates of adverse consequences among the NMS cohort reflected higher rates of both side effect and diminished efficacy notes. Differences were attenuated, but higher rates of adverse consequences persisted among the NMS group compared with the control group after multivariable adjustment. The NMS cohort had higher adjusted rates of medication-related adverse consequences compared with the control cohort at 30 days (13.8% vs. 4.0%, p = .003), 90 days (31.6% vs 9.6%, p < .001), and 365 days (54.7% vs. 20.3%, p < .001).
Table 3. Physician documentation of adverse clinical consequences.
The NMS cohort had higher rates of subsequent medication switch compared with the control cohort at 30 days (5.2% vs. 0.8%, p < .001), 90 days (12.1% vs. 2.6%, p < .001), and 365 days (27.3% vs. 13.9%, p = .003). Higher rates of subsequent switching attributable to adverse consequences from a previous agent were more common among the NMS group compared with the control group at 365 days (14.1% vs. 4.4%, p = .005) (). Findings were similar after multivariable adjustment.
Table 4. Subsequent switching of anti-TNF biological therapy.
Discussion
In the present study, non-medical switching among patients receiving anti-TNF biological therapy was associated with increased health care use, increased prevalence of physician notes related to diminished efficacy and side effects, and higher rates of subsequent switching to alternative anti-TNF biological agents. The impact of a non-medical switch was observed as early as within the first 30 days following the switch and persisted for a year following the change. These findings suggest that a non-medical switch is a clinically consequential event that can meaningfully affect patient care and outcomes and agree with results of a recent study, which showed that patients who switch from anti-TNF biologic therapy for non-medical reasons experience significantly worse clinical outcomes and increased health care resource utilization than those who remain on therapyCitation23. Further, these results support physicians’ concerns that non-medical switching may adversely affect patient careCitation24.
The reasons underlying non-medical switching are varied. Non-medical switching may occur because patients desire alternative formulations (subcutaneous vs. infusion), less frequent dosing regimens, or other conveniences provided by an alternative agent. However, the higher rates of office visits and further therapy changes within the year following the switch suggest that this convenience may come at a price. Alternatively, non-medical switching may occur for cost-related reasons, as was likely the case for the patients in the NMS cohort in our analysis, who had physician notes mentioning cost for the initial medications. Non-medical switching for cost-related reasons may be ultimately patient-driven, as a means of reducing out-of-pocket costs, or payer-driven, such as through a pharmacy benefits manager seeking to reduce costsCitation1. Here too, higher rates of health care use and a greater need to switch to different agents suggest that potential savings in drug costs may be offset by increased health care use and worsened outcomes. For example, in the Medicare Part D population, providers reported that more than one-third of patients undergoing a non-medical switch subsequently required additional medication to treat a side effect they attributed to the non-medical switchCitation24.
A higher rate of adverse consequences, such as diminished efficacy, among patients who experience a non-medical switch is not entirely surprising. Although targeting the same receptor site, the five available anti-TNF biological agents differ in structure, pharmacokinetics, bioavailability, impact upon complement, and immunogenicityCitation1. Differences in subsequent mode of action likely underlie reported differences in the effectiveness and side effect profile of these agents. Of particular concern is the development of anti-TNF biological agent antibodies that blunt therapeutic responseCitation28,Citation29. More common among patients with previous exposure to anti-TNF biological agentsCitation30, these antibodies may account for the decreased therapeutic response with successive changes in anti-TNF biological agents among patients with rheumatoid arthritisCitation31. Non-medical switching would only serve to exacerbate this process. Another potential explanation for these consequences may be the unintentional logistic delays which invariably occur when treatments are changed, such as those related to benefit verification or prior authorization. Such delays may have resulted in discontinuations or relapses and further deterioration.
Results of the present study raise concerns regarding the continued practice of non-medical switching. Clinicians and patients considering switching agents for non-medical reasons might be assuming that prescribing agents within the same therapeutic class will result in equivalent or at least similar clinical outcomes. Clinical studies suggesting similar effectiveness among patients switching agents may be falsely reassuring in that they rely on patients who have previously failed treatment, and also do not account for real-world delaysCitation32,Citation33. By contrast, patients who experience a non-medical switch are presumably tolerating their agent prior to the switch. These patients, as such, face the potential risk of side effects or diminished efficacy inherent in switching with no expectation of added clinical benefit.
A strength of the current study is the ability to identify patients who switched anti-TNF biological agents for reasons that were related to cost and not a result of side effects or lack of efficacy, based on information in the physician’s medical notes. It is not possible to identify reasons for switching therapy in an analysis based solely on administrative claims data. In addition, the study population was both diverse and robust, drawing from a dataset with national coverage and including the five principal anti-TNF biological agents used during the period 2007–2013.
Limitations
This study has certain limitations. First, the study relied on EMR data obtained by Humedica. As such, care provided by clinicians and provider organizations not participating in Humedica cannot be evaluated, which may be particularly problematic for emergency department visits and hospital admissions that occurred at non-participating centers. It also discourages the calculation of an overall NMS rate from these data alone. Second, non-medical switching was identified based on physician notes, and thus it could not be determined whether patients actually received the prescriptions, chose not to fill them, or took their medication as prescribed. Likewise, it is possible that non-medical switching could have occurred in other patients but was not recorded in the physician notes. Further, it was not possible to independently corroborate physician documentation of “side effect” or diminished “efficacy,” nor was it possible to observe details about the patient and provider decision-making processes driving a medication switch. Third, our sample of patients who experienced a non-medical switch was limited to 158; thus, we were unable to evaluate heterogeneity of non-medical switching effects. Fourth, this evaluation focused on a specific class of therapy (anti-TNF biological agents) and may not be generalizable to other clinical agents or classes. However, physician surveys of non-medical switching suggest that concerns are not limited to any particular class of medicationCitation22. Finally, the possibility that unmeasured covariates may contribute to our findings cannot be excluded. We attempted to minimize this risk with matching and covariate adjustment. Although this study represents an improvement over existing studies of non-medical switching based on administrative data, these limitations together reinforce the gross nature of the findings and the need for additional research.
Conclusions
The present study shows that non-medical switching among stable patients prescribed anti-TNF biological agents was associated with increased health care use, increased medication-related side effects, and reports of diminished efficacy. Further, patients who experienced a non-medical switch of an anti-TNF biological agent were more likely to undergo further changes in medication within a year. As such, non-medical switching of anti-TNF biological therapy in otherwise medically stable patients often resulted in unintended consequences that undermined, in part, the underlying rationale for the switch. Accordingly, our study suggests that patients and physicians should be educated about the risks of non-medical switching of anti-TNF biological agents.
Transparency
Declaration of funding
This work was supported by AbbVie. The study sponsor participated in the interpretation of data, review, and approval of the article.
Author contributions: A.G., D.T.R., S.J.J. and M.D. were involved in the conception and design of the study. All authors provided analysis and interpretation of the data, critically revised the manuscript for intellectual content, and provided final approval of the submitted version.
Declaration of financial/other relationships
A.G. has disclosed that he has received consulting and speaker fees from AbbVie, Amgen, Celgene, Eli Lilly and Company, Genentech, Horizon, Iroko, Novartis, Pfizer and UCB; and is a shareholder of AbbVie, Amgen, BMS, GSK, Horizon, J&J and Pfizer. M.S. and M.M. have disclosed that they are employees and stockholders of AbbVie. S.J.J. and M.D. have disclosed that they are employees of Medicus Economics, which received payment from AbbVie to participate in this research. J.C. has disclosed that he is a former employee of AbbVie and stockholder of AbbVie. D.T.R. has disclosed that he has received consulting fees from Abbvie, Abgenomics, Celgene Corporation, Forward Pharma, Genentech/Roche, Janssen Pharmaceuticals, Miraca Life Sciences, Pfizer, Samsung Bioepis, Sandoz Pharmaceuticals, Shire and Takeda; and research support from AbbVie, Genentech/Roche, Janssen Pharmaceuticals, Prometheus Laboratories, Shire, Takeda and UCB Pharma.
CMRO peer reviewers on this manuscript have received an honorarium from CMRO for their review work, but have no relevant financial or other relationships to disclose.
Medical writing support was provided by Andrew Epstein PhD of Medicus Economics, LLC, Milton, Massachusetts and Joann Hettasch PhD of Arbor Communications Inc., a member of the Fishawack Group of Companies, Conshohocken, Pennsylvania and funded by AbbVie.
Appendices
Download MS Word (39.5 KB)Acknowledgements
Previous presentation: Part of this work was presented at the 10th Congress of European Crohn’s and Colitis Organisation, Barcelona, Spain, 18–21 February 2015.
References
- Reynolds A, Koenig AS, Bananis E, Singh A. When is switching warranted among biologic therapies in rheumatoid arthritis? Expert Rev Pharmacoecon Outcomes Res 2012;12:319-33
- Armuzzi A, Lionetti P, Blandizzi C, et al. Anti-TNF agents as therapeutic choice in immune-mediated inflammatory diseases: focus on adalimumab. Int J Immunopathol Pharmacol 2014;27(1Suppl):11-32
- Kuek A, Hazleman BL, Ostor AJ. Immune-mediated inflammatory diseases (IMIDs) and biologic therapy: a medical revolution. Postgrad Med J 2007;83:251-60
- Deodhar A, Mittal M, Reilly P, et al. Ankylosing spondylitis diagnosis in US patients with back pain: identifying providers involved and factors associated with rheumatology referral delay. Clin Rheumatol 2016;35:1769-76
- Kekow J, Moots RJ, Emery P, et al. Patient-reported outcomes improve with etanercept plus methotrexate in active early rheumatoid arthritis and the improvement is strongly associated with remission: the COMET trial. Ann Rheum Dis 2010;69:222-5
- Colombel JF, Sandborn WJ, Ghosh S, et al. Four-year maintenance treatment with adalimumab in patients with moderately to severely active ulcerative colitis: Data from ULTRA 1, 2, and 3. Am J Gastroenterol 2014;109:1771-80
- Rutgeerts P, Sandborn WJ, Feagan BG, et al. Infliximab for induction and maintenance therapy for ulcerative colitis. N Engl J Med 2005;353:2462-76
- Panaccione R, Colombel JF, Sandborn WJ, et al. Adalimumab maintains remission of Crohn’s disease after up to 4 years of treatment: data from CHARM and ADHERE. Aliment Pharmacol Ther 2013;38:1236-47
- Smolen JS, Han C, van der Heijde DM, et al. Radiographic changes in rheumatoid arthritis patients attaining different disease activity states with methotrexate monotherapy and infliximab plus methotrexate: the impacts of remission and tumour necrosis factor blockade. Ann Rheum Dis 2009;68:823-7
- Breedveld FC, Weisman MH, Kavanaugh AF, et al. The PREMIER study: a multicenter, randomized, double-blind clinical trial of combination therapy with adalimumab plus methotrexate versus methotrexate alone or adalimumab alone in patients with early, aggressive rheumatoid arthritis who had not had previous methotrexate treatment. Arthritis Rheum 2006;54:26-37
- Hanauer SB, Feagan BG, Lichtenstein GR, et al. Maintenance infliximab for Crohn’s disease: the ACCENT I randomised trial. Lancet 2002;359:1541-9
- Wendling D, Joshi A, Reilly P, et al. Comparing the risk of developing uveitis in patients initiating anti-tumor necrosis factor therapy for ankylosing spondylitis: an analysis of a large US claims database. Curr Med Res Opin 2014;30:2515-21
- Olivieri I, Cortesi PA, de Portu S, et al. Long-term costs and outcomes in psoriatic arthritis patients not responding to conventional therapy treated with tumour necrosis factor inhibitors: the extension of the Psoriatic Arthritis Cost Evaluation (PACE) study. Clin Exp Rheumatol 2016;34:68-75
- Staples MP, March L, Lassere M, et al. Health-related quality of life and continuation rate on first-line anti-tumour necrosis factor therapy among rheumatoid arthritis patients from the Australian Rheumatology Association Database. Rheumatology (Oxford) 2011;50:166-75
- Brandt J, Braun J. Anti-TNF-alpha agents in the treatment of psoriatic arthritis. Expert Opin Biol Ther 2006;6:99-107
- Lapadula G, Marchesoni A, Armuzzi A, et al. Adalimumab in the treatment of immune-mediated diseases. Int J Immunopathol Pharmacol 2014;27(1Suppl):33-48
- Gisondi P, Girolomoni G. Impact of TNF-alpha antagonists on the quality of life in selected skin diseases. G Ital Dermatol Venereol 2013;148:243-8
- Laas K, Peltomaa R, Kautiainen H, Leirisalo-Repo M. Clinical impact of switching from infliximab to etanercept in patients with rheumatoid arthritis. Clin Rheumatol 2008;27:927-32
- Hutchinson D, Tier J, Soper S, et al. The conversion of infliximab to adalimumab in stable RA patients [abstract]. Rheumatology 2005;44(Suppl1):i72
- Liu Y, Skup M, Chao J. Impact of non-medical switching on healthcare costs: a claims database analysis. Abstract presentation at 20th Annual meeting of ISPOR, Philadelphia, PA, May 2015. Available at: http://www.ispor.org/ScientificPresentationsDatabase/Presentation/53795 [Last accessed 1 June 2017]
- Van Assche G, Vermeire S, Ballet V, et al. Switch to adalimumab in patients with Crohn’s disease controlled by maintenance infliximab: prospective randomised SWITCH trial. Gut 2012;61:229-34
- Boktor M, Motlis A, Aravantagi A, et al. Substitution with Alternative Anti-TNFalpha Therapy (SAVANT) – outcomes of a Crohn’s disease cohort undergoing substitution therapy with certolizumab. Inflamm Bowel Dis 2016;22:1353-61
- Wolf D, Skup M, Yang H, et al. Clinical outcomes associated with switching or discontinuation from anti-TNF inhibitors for nonmedical reasons. Clin Ther 2017;39:849-62.e846
- Cote BR, Petersen EA. Impact of therapeutic switching in long-term care. Am J Manag Care 2008;14(11Suppl):SP23-8
- Bates M. Models of natural language understanding. Proc Natl Acad Sci USA 1995;92:9977-82
- Hansen BB, Bowers J. Covariate balance in simple, stratified and clustered comparative studies. Statist Sci 2008;23:219-36
- Chamberlain G. Analysis of covariance with qualitative data. Rev Econ Stud 1980;48:225-38
- Bartelds GM, Krieckaert CL, Nurmohamed MT, et al. Development of antidrug antibodies against adalimumab and association with disease activity and treatment failure during long-term follow-up. JAMA 2011;305:1460-8
- Steenholdt C, Frederiksen MT, Bendtzen K, et al. Time course and clinical implications of development of antibodies against adalimumab in patients with inflammatory bowel disease. J Clin Gastroenterol 2016;50:483-9
- Bartelds GM, Wijbrandts CA, Nurmohamed MT, et al. Anti-infliximab and anti-adalimumab antibodies in relation to response to adalimumab in infliximab switchers and anti-tumour necrosis factor naive patients: a cohort study. Ann Rheum Dis 2010;69:817-21
- Rendas-Baum R, Wallenstein GV, Koncz T, et al. Evaluating the efficacy of sequential biologic therapies for rheumatoid arthritis patients with an inadequate response to tumor necrosis factor-alpha inhibitors. Arthritis Res Ther 2011;13:R25
- Bombardieri S, Ruiz AA, Fardellone P, et al. Effectiveness of adalimumab for rheumatoid arthritis in patients with a history of TNF-antagonist therapy in clinical practice. Rheumatology (Oxford) 2007;46:1191-9
- Nikas SN, Voulgari PV, Alamanos Y, et al. Efficacy and safety of switching from infliximab to adalimumab: a comparative controlled study. Ann Rheum Dis 2006;65:257-60