Abstract
Objective: The accuracy of prediction of ovulation by cycle apps and published calendar methods was determined by comparing to true probability of ovulation.
Methods: A total of 949 volunteers collected urine samples for one entire menstrual cycle. Luteinizing hormone was measured to assign surge day, enabling probability of ovulation to be determined across different cycle lengths. Cycle-tracking apps were downloaded. As none provided their methodology, four published calendar-based methods were also examined: standard days, rhythm, alternative rhythm and simple calendar method. The volunteer ovulation data was applied to the app/calendar methods to determine their accuracy.
Results: Mean cycle length was 28 days (range: 23–35); 34% of women believed they had a 28-day cycle, but only 15% did. No LH surge was seen for 99 women. Most likely day of ovulation for a 28-day cycle was day 16 (21%). Accuracy of ovulation prediction was no better than 21% by the apps. The standard days and rhythm methods were most likely to predict ovulation (70% and 89%, respectively) but had very low accuracy.
Conclusions: Ovulation day varies considerably for any given menstrual cycle length, thus it is not possible for calendar/app methods that use cycle-length information alone to accurately predict the day of ovulation.
National Clinical Trial Code: NCT01577147. Registry website: www.clinicaltrials.gov.
Introduction
The demographic of women seeking to conceive has changed greatly over the past few decades, with women in more developed countries increasingly delaying pregnancy. Busier lifestyle commitments often coincide with less frequent sexual intercourse so timed intercourse is often used to assist with conceptionCitation1,Citation2. Women planning pregnancy expect to conceive quickly, “within 3–6 months”Citation3, leading to anxiety if they fail to do soCitation4. The fertile window is the time in a woman’s cycle where unprotected intercourse can lead to pregnancy, and typically lasts 6 days ending on the day of ovulationCitation5, but does vary between womenCitation6. So correct timing of intercourse is important, with it being especially important to engage in intercourse prior to ovulation. Evidence also suggests that women who are aware of their ovulatory pattern are more likely to conceive over 12 monthsCitation6,Citation7. The majority of women actively trying to conceive, however, have significant knowledge gaps with regard to pregnancy and fertilityCitation8,Citation9.
Although the average length of a woman’s menstrual cycle is 28 days, there is considerable intra- and inter-individual variation in cycle length, as well as changes in length with timeCitation10,Citation11. It has been demonstrated that cycle length varies by ≥7 days in more than 46% of women aged 18–40 years, and by ≥14 days in 20% of women in the same age rangeCitation12. Similarly, the length and timing of the fertile window is variable between womenCitation6.
There is also both inter- and intra-individual variation in the day of ovulationCitation13. Measurement of urinary luteinizing hormone (LH) is an excellent way to determine ovulation day, but it is of great importance to select the appropriate assay. Intact LH surges prior to ovulation and is excreted quickly, whereas assays measuring beta LH also detect LH breakdown products that are excreted more slowly. Therefore, beta LH assays can often provide profiles with more than one surge and have peak levels occurring at a considerable time after ovulationCitation14–18. As peak levels can occur post ovulation, even with an intact LH assay, it is more appropriate to measure the LH surge: there are a wide variety of methods for determining the surgeCitation19, with the most accurate method providing 1-day prediction of ovulation with maximal error of ±1 dayCitation14,Citation15.
There is a growing number of fertility applications (apps), although few of them disclose the algorithms that they useCitation2,Citation20. Most predictions of the fertile window given by fertility apps are generated from user data, such as date of last menstrual period and cycle lengthCitation21, or the assumption of a 28-day cycle, with ovulation on day 14Citation22. Owing to the variability of menstrual cycle length, doubt has been cast over the reliability of calendar-based fertility appsCitation12. This study explored the variability in the timing of ovulation, via measurement of the LH surge, during women’s cycles and used this information to assess prediction accuracy of calendar apps and calendar-based methods for women seeking to become pregnant.
Methods
This study consisted of three separate strands: a cohort study to determine actual ovulation day for any given cycle length in women trying to conceive, an assessment of the accuracy of apps, in comparison to actual ovulation day, and an assessment of calendar-based methods in comparison to actual ovulation day. An outline of the study flow is shown in .
This home-based observational study (NCT01577147) involved 949 volunteers aged 18 years or older, who were seeking to conceive naturally. The study was approved by the SPD Ethics Committee and all procedures were conducted in accordance with relevant regulations and guidelines.
Participants were recruited from across the UK via Internet advertising. In order to recruit a study cohort which represents the population of women using apps, there was no restriction on the length of time participants had been trying to conceive prior to study entry. Those who were eligible were enrolled onto the study once they had provided written consent to the inclusion of information pertaining to themselves. Demographic data was self-reported and participants acknowledged that their data would be fully anonymized within the final manuscript.
Volunteers collected a daily early morning urine sample for an entire menstrual cycle into labeled pots containing sodium azide preservative. Samples were posted to the laboratory in batches, and analyte stability has previously been validated under these conditionsCitation23. Volunteers also completed a menstrual cycle diary. Menstrual cycle length was defined as the time between the day on which bleeding commenced to the day before bleeding within the next cycle commenced. Spotting was recorded separately in the menstrual cycle diary. Samples were stored under previously validated conditions; 4 °C until analysis or at −80 °C if the analysis could not be performed within 2 weeks of receiptCitation24. Urinary LH was measured using an AutoDELFIA™ (Perkin Elmer, Waltham, MA, USA) assay with in-house antibodies for intact LH, which has been validated against ultrasound methods and been shown to predict ovulation by 1 day (±1 day)Citation14,Citation15. Human chorionic gonadotropin (hCG) (AutoDELFIA™) was also measured to exclude volunteers already pregnant from participating in the study. Volunteers who became pregnant during the study were not included as they did not have a cycle length. Missing urine samples were only significant if they occurred at LH surge onset, and no cycles were omitted because surge samples were missing.
All cycle-tracking calendar apps (iOS and Android) available during the period 4th–13th October 2017 were downloaded and examined to determine whether information was provided on how the apps calculate the fertile phase and on their accuracy. As none of the apps published their method of calculation, a simulated cycle was inputted to examine accuracy. The data inputted were: last menstrual period, 1st October 2017; period length, 5 days; cycle length, 28 days; luteal phase, 14 days; regular cycle; no hormonal contraception (not all apps required all the information).
As the method of fertile-phase calculation by the examined apps was not available, methods published in the literature that use calendar information alone were examined. These predictive methods were: the standard days method, which predicts days 8–19 of the cycle as fertile daysCitation25; the rhythm method, which predicts fertile days using a formula and is based on data from the menstrual records of the past six cycles (the fertile period is predicted as starting on day [x − 18] and ending on day [y – 11], where x is the shortest and y is the longest number of days in a woman’s menstrual cycle record)Citation12,Citation26; the alternative rhythm method, which predicts fertile days from menstrual records using a different formula (the fertile days start on day (½x − 5) and last for (y – x + 8) days, where x is the shortest and y is the longest number of days in a woman’s menstrual cycle recordCitation27; and the simple calendar method, which subtracts 14 and 15 days from the last cycle length to give the peak fertility daysCitation22.
For statistical analyses, a probability table () and probability curve () were created to record the likelihood of ovulation on any given day for the range of cycle lengths, based on percentage of the population observed to ovulate on any given day. Statistical analyses were performed using the SAS 9.3 software by a qualified statistician.
Figure 2. Probability curve of the likelihood of ovulation on any given day of a 28-day cycle based on the percentage of the population observed to ovulate on any given day, where ovulation is day of LH surge +1 day.
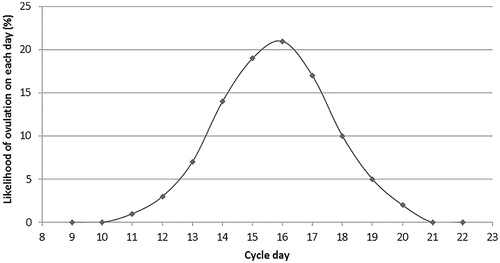
Table 1. Probability of ovulation on any given day of the cycle according to cycle length.
The accuracy of calendar apps was determined by comparison of the prediction provided following input of standard data with the probability of ovulation obtained from examination of the volunteers’ cycles. Where a single day is predicted by the app, accuracy is the probability of ovulation on that day. Where several days are predicted by the app, accuracy is the cumulative probability of the ovulation day being contained within this window. The accuracy assessment does not adjust for incorrect predictions (because there are no clinical repercussions for an incorrect result), so the analysis represents best-case scenario. Accuracy of the calendar-based methods was determined retrospectively also as “best-case scenario”, in that the calculation used the pertinent cycle characteristics supplied in the baseline demographics.
Data availability
Raw data were generated at SPD Development Company Ltd. Derived data supporting the findings of this study are available from the corresponding author S.J. on request.
Results
Participants had a mean age of 32 years (range: 18–50), and a mean body mass index (BMI) of 26.67 kg/m2 (range: 14.8–63.8). On average, the participants had been trying to conceive for 15 months (range: 1–162), the number of previous live births was one (range: 0–10), and the number of reported miscarriages or stillbirths was one. Of the total number of participants, 3% (n = 23) had self-reported endometriosis and 6.8% (n = 52) had self-reported polycystic ovary syndrome. Women reported a median of 3 days’ difference between their shortest and longest cycle lengths for the last 6 months (range 0–56 days), with 27.4% reporting a difference of five or more days and 11.2% reporting 0 days’ difference between their shortest and longest cycles.
For the final study population (n = 768), a number of participants were excluded due to menstrual cycle lengths being shorter than 23 days or longer that 35 days (n = 81); in addition, one volunteer was excluded due to hCG being present at low levels (0.5–5 mIU/ml) for the entire cycle, which is indicative of perimenopauseCitation28. A further 99 volunteers were not included in the analysis due to having no LH surge in the cycle. The mean menstrual cycle length was 28 days (range: 17–35), with 15% of volunteers having a 28-day cycle and more than 70% having a cycle length of 25–30 days. Interestingly, self-reported menstrual cycle characteristics prior to study start indicated that 34% of the participants thought that their cycle length was 28 days (range 20–47).
Surprisingly, we found that the most likely day of ovulation, defined as the day following the LH surge, was day 16 (21%) for a 28-day cycle. Day 14, which is typically estimated to be the most likely day of ovulation for a woman with a 28-day cycle using the simple calendar methodCitation22 was associated with a 14% likelihood of ovulation (; ). The probability of ovulating was spread across a range of 11–20 days in a 28-day cycle (; ). A similar broad spread of probable ovulation days was observed in cycle lengths that were either shorter or longer than 28 days (). This means that, to include the day of ovulation, an app/calendar method using cycle length alone would have to identify at least 10 potentially fertile days. For a 90% probability of providing a window that includes the ovulation day, an 8-day window is required.
Spread of day of ovulation across age quartiles and quartiles of time trying to conceive were examined to see whether luteal phase length varied by age or length of time trying. This analysis revealed that the luteal phase length was not associated with the age of the participants () or with the time trying to conceive ().
Figure 3. Length of luteal phase (time from ovulation day (LH surge +1 day) to end of cycle) was determined for participants in the age ranges: 18–29, 30–32, 33–36, and 37–50 years. Bars indicate the proportion of participants with a luteal phase of a given length. Lines indicate the normal length of luteal phase.
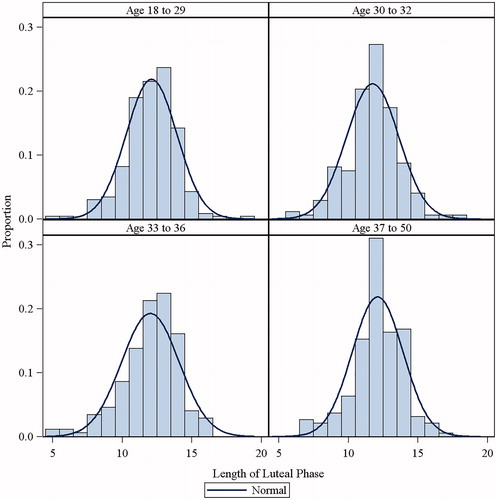
Figure 4. Length of luteal phase (time from ovulation day (LH surge +1 day) to end of cycle) was determined for participants who had been trying to conceive for: 1–4 months, 5–8 months, 9–18 months, and 19–162 months. Bars indicate the proportion of participants with a luteal phase of a given length. Lines indicate the normal length of luteal phase.
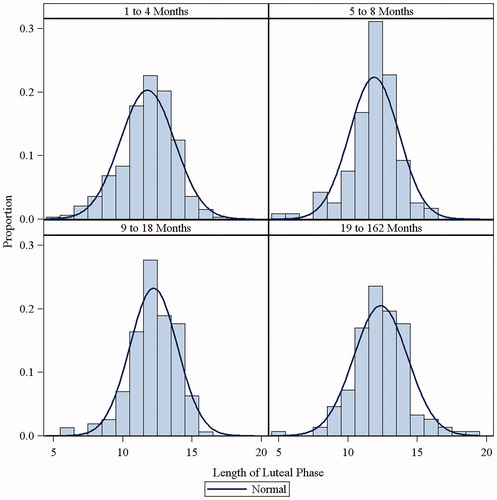
Of the 73 calendar apps downloaded for cycle tracking (10 iOS and Android, 37 iOS only, 26 Android only; Supplemental Appendix), none provided any information on how they were able to predict the day of ovulation. Only one app provided information on the accuracy of prediction, indicating it was 60%. A prediction of day of ovulation was provided by 55 apps using input information of cycle length, cycle variability and last menstrual period. When a simulated 28-day cycle was inputted into the apps, the majority reported day 15 to be the day of ovulation (), which has a 19% probability of being the true day of ovulation for a 28-day cycle. The highest probability possible for the prediction of day 16 was 21%. This analysis assumed that the user’s next cycle would be 28 days when, in reality, it is not possible to predict future cycle length and there is considerable intra-individual variation in cycle length. Therefore, these results represent optimal performance.
Table 2. Prediction of day of ovulation by apps.
Most apps (n = 64, ) provided the user with a window for their fertile days. These estimates varied considerably between apps, varying from 4 to 12 days in length. The most commonly generated fertile window was 10–16 days (n = 16), which had a 65% probability of including the day of ovulation for a 28-day cycle. Those with high probabilities of prediction provided users with the longest window.
Table 3. Prediction of the fertile window by apps.
As none of the apps published their method for fertile-phase prediction, published methods were examined for accuracy. A high degree of variation in prediction accuracy of the fertile window and day of ovulation between different predictive methods was observed, with the alternative rhythm and simple calendar method having the poorest predictive value () as they included fertile days in their predictions less than 52% and 70% of the time, respectively.
Figure 5. Graphical representation of the proportion of published calendar methods that identify ovulation day or fertile window on any given day. Proportion of calendar methods that correctly identified the day of ovulation is indicated by light grey bars. Proportion of calendar methods that correctly identified any day in the fertile window is indicated by dark grey bars.
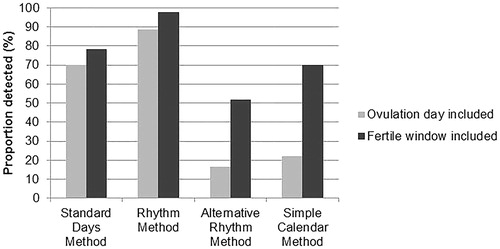
All four methods had an ovulation-day prediction accuracy lower than 90% (). The standard days method and the rhythm method showed the highest predictive ability for ovulation day, although 30% and 11% of the participants, respectively, had menstrual cycle characteristics outside the permitted range to enable calculation. Both the alternative rhythm method and the simple calendar method showed poor predictive ability. The simple calendar method included day of ovulation in its prediction less than 22% of the time, whereas the alternative rhythm method included ovulation day less than 17% of the time. However, the more accurate methods were only able to provide better prediction because they provided the user with a broad fertile window in which to target intercourse: rhythm method, 11 days (range 8–64); standard days method, always 12 days; alternative rhythm method, 4 days (range 0–63 days); simple calendar method, always 2 days.
Discussion
In the current study, a high degree of variability was detected among participants with respect to both menstrual cycle length and day of ovulation for any given cycle length (). Even in participants with 28-day cyclesCitation10,Citation11, there was a wide spread of ovulation days, with the modal value being at day 16 (; ). This is later than seen in other studies, and may be related to the definition of “first day of menstrual cycle” used within this study, i.e. the day on which bleeding commences. This definition was chosen as it makes it directly comparable to apps, which also apply this definition. Researchers defining day 1 as the first full day of bleeding would likely ascertain ovulation day to be up to 1 day earlier, which would still be later than the textbook definition. The spread is also greater than that observed in other studies, probably because this study investigated a seeking-to-conceive population rather than a population of healthy fertile women. Thus, this dataset is much more relevant to examine the performance of methods that are used to predict the fertile phase when trying to achieve pregnancy, especially given that women usually turn to methods which assist with timed intercourse following several months of unsuccessful conceptionCitation29.
Although calendar apps designed to define optimal fertility are becoming an increasingly popular method for timing sexual intercourse, as found in this study, they do not provide information on how the predictions are calculated or on the accuracy of those predictions.
Most calendar apps attempted to provide users with their day of ovulation, but maximal probability of this being correct was 21%. Therefore, it appears that predictions are based on the assumption that ovulation occurs on a set day of the menstrual cycle, for any given cycle length.
Intercourse before ovulation is important, and therefore predicting the day of ovulation in advance is of utility to those trying to conceive. This can be achieved by identifying the wider fertile window. Nearly all calendar apps provided a fertile window to users. Apps that showed extremely long fertile windows had a high likelihood of including day of ovulation, e.g. for the 12-day fertile window, days 10–21 had 100% probability of including the day of ovulation. However, providing such a long fertile window is of little benefit to women trying to conceive as it does not enable the targeting of acts of sexual intercourse. The window that provided the most realistic number of days to users included days 15–19, which had a 72% probability of being correct and included the day of ovulation. The analysis reported here provides best-case results because it assumed that the next cycle would be 28 days long, whereas in reality it is not possible to predict future cycle length owing to intra-individual cycle variabilityCitation13.
The calendar-based methods successfully predicted the fertile window in 53–98% of cases, and successfully predicted the day of ovulation in 16–89% of cases. Indeed, it would only be possible to predict ovulation accurately by giving users an 8–10-day window in which to time sexual intercourse, which provides users with poor accuracy and is similar to the recommended advice of intercourse every 2 days, which practitioners of this advice have reported to be difficult to follow and unrealisticCitation24.
Our findings regarding accuracy of apps/calendar methods are in agreement with both Setton et al.Citation21, who found less than 8% of various websites and Android/iOS apps to accurately predict precise fertile windows, and Moglia et al.Citation30, who found only 19% of English iOS apps which assumed ovulation to occur 13–15 days before the next cycle to accurately detect optimal fertility periodsCitation5,Citation21; however, these studies were based on textbook menstrual cycle definitions, rather than contemporary cycle data. Our study goes a step further, in that it applies the true variability of day of ovulation that is seen in a seeking-to-conceive population.
One limitation of the current study is that only one cycle was investigated, thus the variation in ovulation days between menstrual cycles in individual women could not be determined. Only 27.4% of women in this study varied by 5 days or more, which is substantially less variability than observed in large cohort studies where around half of women have cycles that vary by 7 days or moreCitation12,Citation13, indicating that even women seeking to conceive have poor appreciation of their menstrual cycle characteristics. Predictions of next period date by app-based methods may improve over time, if using an adaptive algorithm, which would not be demonstrated during the investigation of just one cycle. However, improvements in the accuracy of cycle length predictions would not correspond with greater accuracy in predicting the day of ovulation. This is because, as our data shows, it is not possible to predict ovulation day accurately, even if true cycle length is known; a method of external validation is needed.
Conclusions
In conclusion, the true day of ovulation varies considerably for any given cycle length; therefore, calendar/app methods are not able to provide women with an accurate prediction of the day of ovulation. Women should be advised not to rely on such methods to enable the optimal timing of sexual intercourse to achieve pregnancy.
Transparency
Declaration of funding
The study was funded by SPD Development Company Limited, a fully owned subsidiary of SPD Swiss Precision Diagnostics GmbH, the manufacturers of Clearblue pregnancy and fertility tests.
Author contributions: SJ study design, planning, conduct, manuscript writing, final approval of manuscript version to be published. LM study design, data analysis. MZ study design, manuscript writing. All authors agree to be accountable for all aspects of their work.
Declaration of financial/other relationships
S.J. and L.M. have disclosed that they are employees of SPD Development Company Ltd. M.Z. has disclosed that he is an advisory board member for SPD Development Company Ltd.
A peer reviewer on this manuscript was involved with the development and testing of the Standard Days Method for pregnancy prevention, which is one of the calendar-based methods included in this study. They are also currently studying the efficacy of the Dynamic Optimal Timing (Dot) app for pregnancy prevention. CMRO peer reviewers on this manuscript have received an honorarium from CMRO for their review work, and have no other relevant financial relationships to disclose.
Supplementary_Table.docx
Download MS Word (21.2 KB)Acknowledgements
Dionysia Lymperatou (an employee of SPD Development Company Ltd.) downloaded all the cycle apps and inputted the cycle data to obtain the app predictions. Dr Kathryn Charlwood, integrated medhealth communications (funded by SPD Development Company Ltd.), provided medical writing assistance in the form of manuscript journal styling.
References
- Call V, Sprecher S, Schwartz P. The incidence and frequency of marital sex in a national sample. J Marriage Fam 1995;57:639-52
- Godbert S, Miro F, Shreeves C, et al. Comparison between the different methods developed for determining the onset of the LH surge in urine during the human menstrual cycle. Arch Gynecol Obstet 2015;292:1153-61
- Johnson S, Pion C. Multi-national survey of women’s knowledge and attitudes towards fertility and pregnancy. Poster presented at the American Society for Reproductive Medicine meeting; October 12th–17th, 2013, Boston, MA, USA
- Clay R. Does stress hinder conception? Monitor Psychol 2006;37:46
- Wilcox AJ, Dunson D, Baird DD. The timing of the “fertile window” in the menstrual cycle: day specific estimates from a prospective study. BMJ 2000;321:1259-62
- Keulers MJ, Hamilton CJ, Franx A, et al. The length of the fertile window is associated with the chance of spontaneously conceiving an ongoing pregnancy in subfertile couples. Hum Reprod 2007;22:1652-6
- Hilgers TW, Daly KD, Prebil AM, et al. Cumulative pregnancy rates in patients with apparently normal fertility and fertility-focused intercourse. J Reprod Med 1992;37:864-6
- Zinaman M, Johnson S, Ellis J, et al. Accuracy of perception of ovulation day in women trying to conceive. Curr Med Res Opin 2012;28:749-54
- Kudesia R, Chernyak E, and McAvey B. Low fertility awareness in United States reproductive-aged women and medical trainees: creation and validation of the Fertility & Infertility Treatment Knowledge Score (FIT-KS). Fertil Steril 2017;108:711-17
- Weller L, Weller A. Menstrual variability and the measurement of menstrual synchrony. Psychoneuroendocrinology 1997;22:115-28
- Park SJ, Goldsmith LT, Skurnick JH, et al. Characteristics of the urinary luteinizing hormone surge in young ovulatory women. Fertil Steril 2007;88:684-90
- Creinin MD, Keverline S, Meyn LA. How regular is regular? An analysis of menstrual cycle regularity. Contraception 2004;70:289-92
- Johnson SR, Miro F, Barrett S, et al. Levels of urinary human chorionic gonadotrophin (hCG) following conception and variability of menstrual cycle length in a cohort of women attempting to conceive. Curr Med Res Opin 2009;25:741-8
- Roos J, Johnson S, Weddell S, et al. Monitoring the menstrual cycle: comparison of urinary and serum reproductive hormones referenced to true ovulation. Eur J Contracept Reprod Health Care 2015;20:438-50
- Johnson S, Weddell S, Godbert S, et al. Development of the first urinary reproductive hormone ranges referenced to independently determined ovulation day. Clin Chem Lab Med 2015;53:1099-108
- Echocard R, Boehringer H, Rabilloud M, et al. Chronological aspects of ultrasound, hormonal, and other indirect indices of ovulation. BJOG 2001;108:822-9
- Leiva RA, Bouchard TP, Abdullah SH, et al. Urinary lutenizing hormone tests: which concentration threshold best predicts ovulation? Front Public Health 2017;5:1-17
- Direito A, Bailly S, Mariani A, et al. Relationships between the luteinizing hormone surge and other characteristics of the menstrual cycle in normally ovulating women. Fertil Steril 2013;99:279-85
- Godbert S, Miro F, Shreeves C, et al. Comparison between the different methods developed for determining the onset of the LH surge in urine during the human menstrual cycle. Arch Gynecol Obstet 2015;292:1153-61
- Institute for Human Data Science. Patient adoption of mHealth: use, evidence and remaining barriers to mainstream acceptance. Parsippany, NJ: IMS Institute for Healthcare Informatics: 2015 [cited May 17th 2017]. Available from: https://www.iqvia.com/-/media/iqvia/pdfs/institute-reports/patient-adoption-of-mhealth.pdf
- Setton R, Tierney C, Tsai T. The accuracy of web sites and cellular phone applications in predicting the fertile window. Obstet Gynecol 2016;128:58-63
- Howards PP, Schisterman EF, Wactawski-Wende J, et al. Timing clinic visits to phases of the menstrual cycle by using a fertility monitor: the BioCycle Study. Am J Epidemiol 2009;169:105-12
- Miro F, Parker SW, Aspinall LJ, et al. Sequential classification of endocrine stages during reproductive aging in women: the FREEDOM study. Menopause 2005;12:281-90
- Tiplady S, Jones G, Campbell M, et al. Home ovulation tests and stress in women trying to conceive: a randomized controlled trial. Hum Reprod 2013;28:138-51
- Arevalo M, Jennings V, Sinai I. Efficacy of a new method of family planning: the Standard Days Method. Contraception 2002;65:333-8
- Family Planning Website: A global handbook for providers, Calendar Rhythm Method. 2017. Available at: https://www.fphandbook.org/calendar-rhythm-method [Last accessed August 2017]
- James WH. Cycle day of ovulation. J Biosoc Sci 1972;4:371-8
- Gronowski AM, Fantz CR, Parvin CA, et al. Use of serum FSH to identify perimenopausal women with pituitary hCG. Clin Chem 2008;54:652-6
- Johnson S, Foster L, Humberstone F. Women’s views on fertility and conception in five countries. Fertil Steril 2012;98:409
- Moglia ML, Nguyen HV, Chyjek K, et al. Evaluation of smartphone menstrual cycle tracking applications using an adapted APPLICATIONS scoring system. Obstet Gynecol 2016;127:1153-60