Abstract
Background
Second-generation anaplastic lymphoma kinase (ALK) gene targeted tyrosine kinase inhibitors (TKIs) alectinib and brigatinib have shown efficacy as front-line treatments for ALK-positive non–small cell lung cancer (NSCLC). No head-to-head data are currently available for brigatinib vs alectinib in the ALK-TKI–naive population.
Objective
To estimate the relative overall survival (OS) for brigatinib vs alectinib with indirect treatment comparisons (ITCs) using ALEX and ALTA-1L clinical trial data.
Methods
The latest aggregate data from the ALEX trial and final patient-level data from ALTA-1L were used. ITCs were conducted with/without treatment crossover adjustments to estimate relative OS. Bucher methods, anchored matching-adjusted indirect comparisons (MAICs) and unanchored MAICs were employed in ITCs without treatment crossover adjustments. An inverse probability of censoring weight Cox model, a marginal structure model and rank-preserving structural failure time models (with/without re-censoring) within an anchored MAIC were used in ITCs with treatment crossover adjustments. Hazard ratios (HRs) and 95% confidence intervals (CIs) were reported.
Results
HRs for brigatinib vs alectinib for relative OS generated from ITCs without treatment crossover adjustments ranged from 0.90 (95% CI: 0.59–1.38) in the unanchored MAIC to 1.20 (95% CI: 0.69–2.11) using the Bucher method. Methods employing treatment switching adjustments estimated HRs for relative OS ranging from 0.74 (95% CI: 0.38–1.45) to 1.11 (95% CI: 0.63–1.94). Results from all ITCs did not indicate statistically different survival profiles.
Conclusion
Regardless of ITC methodology, OS is comparable for brigatinib vs alectinib in patients with ALK+ NSCLC previously untreated with an ALK inhibitor.
Introduction
Lung cancer is the leading cause of cancer-related mortality worldwide each year, accounting for 18% of all cancer deathsCitation1. The most common type, non–small cell lung cancer (NSCLC), is responsible for more than 80% of cancer casesCitation2. Approximately 3–5% of patients with NSCLC have stage IIIB/IV with rearrangements in the anaplastic lymphoma kinase (ALK) gene, making these patients candidates for targeted molecular therapyCitation3–5.
Second-generation ALK-targeted tyrosine kinase inhibitors (TKIs) alectinib and brigatinib have shown efficacy as front-line treatments for ALK-positive (ALK+) NSCLC previously untreated with an ALK inhibitorCitation6,Citation7. However, no head-to-head data are currently available comparing overall survival (OS) with brigatinib versus alectinib in patients with ALK TKI-naive ALK+ NSCLC. Both drugs were investigated in international, open-label, randomized, phase 3 clinical trials relative to a common comparator arm, crizotinibCitation8,Citation9. The ALTA-1L clinical trial evaluated brigatinib versus crizotinib, and the ALEX clinical trial assessed alectinib versus crizotinib. Recently published final results for ALTA-1L reflected a median follow-up of 40.4 months (brigatinib arm); the median OS was not reached for brigatinib or crizotinib (hazard ratio [HR]: 0.81, 95% confidence interval [CI]: 0.53–1.22)Citation8. The latest results for ALEX were published in 2020, and reflected a median follow-up of 48.2 months (alectinib arm); median OS was not reached for alectinib (HR: 0.67, 95% CI: 0.46–0.98) versus 57.4 months with crizotinib (95% CI 34.6–not reached)Citation9.
In the absence of head-to-head comparisons, indirect comparison techniques using results from more than one trial must be adopted to estimate relative efficacy. There are several mathematical approaches that can utilize the results from randomized controlled trials (RCTs) for this purpose. Indirect treatment comparisons (ITCs) may be useful to payers and providers in the development of treatment pathways or treatment decisions. Bucher et al. studied the use of ITCs and proposed that treatment comparisons be based on the relative treatment effects from each study that confer intra-trial randomization benefits and not the raw or direct resultsCitation10. This method requires a common “linking” or “anchoring” treatment arm between two trials. It does not address treatment effect modifiers that may be different between studies and thereby could bias the resultsCitation11. Anchored matching-adjusted indirect comparison (MAIC) methodology, as performed with the Bucher technique, also aims to confer intra-trial randomization benefits but attempts to also control for between-study imbalances in observable treatment effect modifiers. MAIC methodology requires a common anchoring arm between the two studiesCitation11. Unanchored MAIC techniques are typically used when there is no common anchoring treatment arm between trials. It does not confer intra-study randomization benefits, and such methods must adjust for both prognostic confounder imbalance and treatment effect modifying confounder imbalance between the studies. In special circumstances that threaten the validity of the anchored MAIC assumptions, the unanchored MAIC methods can be relevant, even if there is a common linking armCitation11.
ITCs can address key differences in trial designs and baseline characteristics that may influence treatment effect and prognosis. ALTA-1L and ALEX had generally comparable patient populations in terms of age, gender and ethnicity; however, two main differences in treatment effect modifiers between ALTA-1L and ALEX were identified – one is due to the differences in random sampling and the other is due to the differences in the trial designs. First, regarding the difference in the random sampling, the proportion of patients with baseline central nervous system (CNS) metastases (bCNS-m) was different in the two trials (29% vs 30% for brigatinib vs crizotinib in ALTA-1L and 42% vs 38% for alectinib vs crizotinib in ALEX; Supplemental Table 1). The proportion of patients with bCNS-m was found to modify the treatment effect between brigatinib and crizotinib within the ALTA-1L data setCitation8,Citation9. Second, regarding the difference in the trial design: the ALTA-1L clinical trial allowed crossover from the crizotinib arm to brigatinib upon progression, while it was not specified in the ALEX protocolCitation8,Citation9. Sixty-five patients switched per protocol from crizotinib to brigatinib (47% of the total number of patients in the crizotinib arm of ALTA-1L) and an additional 15 patients received brigatinib after crizotinib as subsequent anticancer treatmentCitation8. At the data cut-off of 29 November 2019, 15.9% of the total number of patients in the crizotinib arm of ALEX received alectinib after disease progression on crizotinib (Supplemental Table 2)Citation9.
The objective of this study was to estimate the relative OS for brigatinib versus alectinib with adjustment for treatment crossover and bCNS-m using the latest results from the ALEX clinical trial and the final results from ALTA-1L. We explored the impact of differences in bCNS-m on the estimation of relative OS for brigatinib versus alectinib and assessed the impact of the potential bias caused by differences in subsequent therapies.
Methods
The latest ALEX trial aggregate results and the final patient-level data from the ALTA-1L trial were used. A comparison of study designs of the ALTA-1L and ALEX trials is shown in . The primary outcome examined in this analysis was OS, defined as the time from the first dose date to death. An ITC was conducted with and without treatment crossover adjustments.
Table 1. Characteristics of the ALTA-1L and ALEX trials.
Indirect treatment comparison without treatment crossover adjustments
Three adjustment methods were used in the ITCs without treatment crossover adjustment. The Bucher method utilizing aggregate clinical trial results was used, which assumed no difference in the treatment effect modifying variables (or that variables were balanced between trials). A subgroup analysis, stratified by patients treated with bCNS-m, was conducted to explore the impact of differences in bCNS-m. The second approach, an anchored MAIC, was conducted and adjusted for inter-trial imbalances in bCNS-m, which was identified as a significant treatment effect modifier (Supplemental Table 3). The third approach, an unanchored MAIC, was conducted using only the brigatinib arm from ALTA-1L and the alectinib arm from ALEX. This avoids the use of the common crizotinib arms where the majority of imbalance in subsequent therapies was observed. It also mitigates the concern of treatment crossover. The unanchored MAIC assumed that all effect modifiers and prognostic factors were accounted for, including sex, age, smoking history, race (Asian vs non-Asian), bCNS-m and Eastern Cooperative Oncology Group (ECOG) performance status of 2 (Supplemental Table 4). These covariates were also used in the United Kingdom’s National Institute for Health and Care Excellence’s (NICE) health technology assessment (HTA) brigatinib 2 L submission, validated at the January 2020 advisory board and used again in the brigatinib 1 L NICE HTA submissionCitation12,Citation13.
Indirect treatment comparisons with treatment crossover adjustment
To adjust for the potential time-dependent confounding effects of treatment crossover after patients discontinued crizotinib, multiple sensitivity analyses on OS were conducted by using a Cox proportional hazard model with inverse probability of censoring weight (IPCW) technique; a marginal structural model (MSM); and rank-preserving structural failure time models (RPSFTM) with and without re-censoring. The IPCW method estimates weights for patients according to their baseline demographic and disease‐related characteristics after artificially censoring at treatment switch. This method adjusts for any potential confounding created by the artificial censoringCitation14. The weighted Cox proportional hazards regression models with the estimated weights are used to obtain an estimate of the treatment effect. In the MSM, patients are also censored at treatment switch, and then weights are estimated for both censored and non-censored patientsCitation15,Citation16. Conversely, RPSFTM assumes that the treatment effect is equal across all patients relative to treatment duration and creates a distribution of survival times had treatment switching not occurred.
In the Bucher estimation comparing brigatinib versus alectinib, all three methods of treatment switching adjustment were implemented: adjusted HRs comparing brigatinib versus crizotinib were estimated by applying IPCW, MSM and RPSFTM. Bucher methods were then used to estimate the relative effect between brigatinib and alectinib. The baseline covariates included in the final model were age, initial diagnosis stage, baseline ECOG score, histopathological class at study entry, measurable intracranial central nervous system disease (yes/no), race (Asian vs non-Asian), sex and smoking history at randomization. The time-dependent covariates were intracranial disease progression, target lesion size and ECOG score.
In the anchored MAIC comparing brigatinib versus alectinib, the RPSFTM-adjusted ALTA-1L survival estimates for the treatment switchers used an acceleration factor such that they represented the predicted survival time had the patient not switched. Official treatment switchers were defined as those patients who switched from crizotinib to brigatinib following documented progressed disease or radiotherapy to the brain. MAIC methods were then applied using adjusted ALTA-1L data to estimate the HR of brigatinib versus alectinib. RPSFTM survival time adjustments can be prone to biases caused by patient-censoring issues: re-censoring corrections to these adjustments can, in theory, alleviate these biases but also can overcompensate. Thus, in line with the published literature and recommendations, results were generated with and without re-censoringCitation17.
Results
Indirect treatment comparisons without treatment crossover adjustment
When the Bucher method was applied to the overall unweighted aggregate trial results, analyses estimated a HR of 1.20 (95% CI: 0.69–2.11) (). The subgroup analysis stratified by bCNS-m found no significant differences between alectinib and brigatinib, although the point estimates showed that patients with bCNS-m experienced greater efficacy with brigatinib compared with alectinib (HR: 0.74, 95% CI: 0.30–1.82), and alternatively, patients without bCNS-m experienced lower efficacy with brigatinib compared with alectinib (HR: 1.52, 95% CI: 0.73–3.15) ().
Figure 1. Indirect treatment comparison (ITC) results for brigatinib vs alectinib for overall survival (OS) without treatment crossover adjustments. (A) Bucher, anchored MAIC and unanchored MAIC. (B) Subgroup analysis using Bucher; patients with bCMS-m and patients without bCMS-m. Abbreviations. bCMS-m, Baseline central nervous system metastases; CI, Confidence interval; HR, Hazard ratio; MAIC, Matching-adjusted indirect comparison.
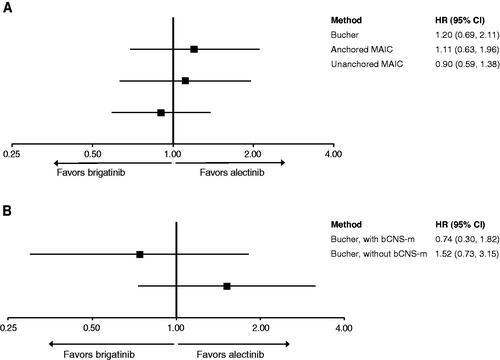
Adjusting for differences in bCNS-m in the anchored MAIC method decreased the HR in favor of brigatinib (HR: 1.11, 95% CI: 0.63–1.96) (). This finding suggests that the treatment effect of brigatinib versus crizotinib would be improved in the ALTA-1L analysis in a population with more bCNS-m, and the treatment effect of brigatinib would then also improve versus alectinib in anchored comparisons. However, the improvement of brigatinib observed after adjustment was minor.
The unanchored MAIC analysis estimated a HR of 0.90 (95% CI: 0.59–1.38) for OS (). In contrast to the anchored MAIC, the unanchored MAIC, without treatment crossover adjustment, resulted in an improvement in the HR for brigatinib versus alectinib, though the result was not significant.
Indirect treatment comparisons with treatment crossover adjustment
In the sensitivity analyses of ALTA-1L adjusting for treatment crossover in the crizotinib arm, the OS HR for brigatinib compared with crizotinib was 0.54 (95% CI: 0.31–0.92) by MSM and 0.50 (95% CI: 0.28–0.87) by IPCWCitation8. Using these adjusted HRs, the Bucher method analyses estimated OS HRs for brigatinib versus alectinib: using IPCW, a HR of 0.74 (95% CI: 0.38–1.45) was estimated; with MSM, a HR of 0.80 (95% CI: 0.41–1.54) was estimated. With RPSFTM, a HR of 1.01 (95% CI: 0.56–1.82) with re-censoring and a HR of 1.11 (95% CI: 0.63–1.94) without re-censoring were estimated (). These results indicate no significant difference in survival between brigatinib and alectinib.
Figure 2. Indirect treatment comparison (ITC) results for brigatinib vs alectinib for overall survival (OS) with treatment crossover adjustments. (A) Bucher, adjusting for treatment crossover. (B) Anchored MAIC, adjusting for treatment crossover. Abbreviations. CI, Confidence interval; HR, Hazard ratio; IPCW, Inverse probability of censoring weight; MAIC, Matching-adjusted indirect comparison; MSM, Marginal structural model; RPSFTM, Rank-preserving structural failure time models.
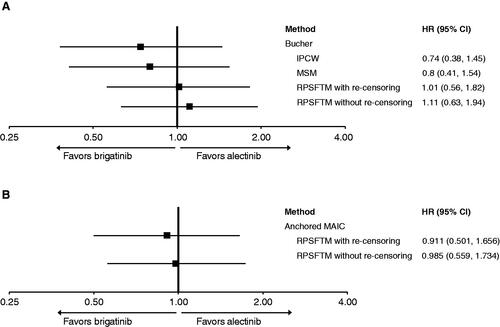
Last, the anchored MAIC method adjusting for crossover estimated HRs for brigatinib versus alectinib OS. Based on official switchers only and using RPSFTM with re-censoring, a HR of 0.91 (95% CI: 0.50–1.66) was estimated; using RPSFTM without re-censoring, a HR of 0.99 (95% CI: 0.56–1.73) was estimated. The results indicate no significant difference in survival between brigatinib and alectinib (). Following adjustment for treatment crossover, the anchored and unanchored MAICs are more similar to each other, which supports the assumptions that the models are correctly specified in and .
Discussion
Standard approaches to ITCs would result in biased estimates of relative efficacy for brigatinib versus alectinib. The assumptions underpinning these approaches require the ALTA-1L and ALEX trials to be comparable in terms of design and patient population, which our findings did not support. Analyses adjusting for these differences indicated an improvement in the HR for brigatinib versus alectinib, suggesting both factors bias against brigatinib in naive comparisons. Adjusting for bCNS-m was shown to improve the HR for brigatinib. Increasing the proportion of patients with bCNS-m by reweighting the data in the ALTA-1L clinical trial would improve the relative efficacy of brigatinib versus crizotinib and thereby improve the comparison with alectinib in the anchored analyses. Adjusting for subsequent brigatinib use in the crizotinib arm was shown to improve the HR for brigatinib in the anchored comparisons; this finding was expected because brigatinib has demonstrated efficacy in the post-crizotinib setting. Therefore, the crizotinib arm reflects a sequence of therapies (i.e. crizotinib followed by brigatinib), where the use of subsequent brigatinib will confound the resultsCitation18.
The unanchored MAIC resulted in an improvement in the HR for brigatinib versus alectinib compared with the anchored MAIC. The difference between the unanchored MAIC and the anchored MAIC indicates that the anchored MAIC has not accounted for all significant treatment effect modifiers; the unanchored MAIC has not accounted for all significant prognostic factors and/or treatment effect modifiers; or the anchored MAIC is adversely affected by differentials in treatment switching from the “linking” crizotinib arm in both trials. The list of prognostic factors has been thoroughly validated, but the assumption is ultimately untestable. Nonetheless, we believe we have accounted for the most important prognostic factors. Receipt of subsequent brigatinib in the ALTA-1L crizotinib arm is likely to improve survival outcomes and therefore bias conclusions when attempting to compare front-line therapies in isolation of subsequent treatments. Therefore, it is likely that the difference is explained by this lack of adjustment. This viewpoint is supported by the much closer level of agreement between anchored and unanchored MAIC results on progression free survival (PFS) – an endpoint unaffected by differentials in treatment switching post progression between the crizotinib arms in ALTA-1L and ALEX, HRs: 0.99 (95% CI: 0.64–1.52) for anchored compared with 1.02 (95% CI: 0.74–1.39) for unanchoredCitation19. This finding suggests that results from the unanchored MAIC act as a good proxy for its anchored counterpart when there are no obvious biases afflicting the anchored version. The unanchored MAIC OS point estimate is likely a good proxy for an anchored MAIC estimate free of trial treatment switching differentials in the “linking” crizotinib arm.
The choice of treatment switching methodology applied can have a large impact on the estimated treatment effect of brigatinib versus crizotinib, which then impacts the anchored comparisons with alectinib. Per NICE technical support document 16, the following methods were candidates for the treatment switching analysis: IPCW, RPSFTM, the two-stage method and the iterative parameter estimation (IPE) approachCitation20,Citation21. The two-stage method and IPE were not pursued in this study. The results show that the assumptions underlying the two-stage method are untenable (e.g. wide disparity in the length of time between progression diagnosis and the initiation of the switch to brigatinib treatment); therefore, this method was not employedCitation21. The IPE approach is similar to RPSFTM in theoretical terms, and these two approaches often produce similar adjusted resultsCitation21.
Because of the small number of events, immaturity of the data (median survival not yet reached), and the potential bias introduced with subsequent treatments, statistical significance was often not observed in OS outcomes despite differences in clinical efficacy; therefore, cross-trial comparison of OS is challenging. Prior studies have estimated the relative efficacy of brigatinib compared with alectinib in ALK-TKI–naive adults with locally advanced or metastatic ALK+ NSCLC using ITC network meta-analysis methodology and found no significant differences in terms of OSCitation22,Citation23. However, the impact of differences across studies regarding the existence of bCNS-m was not addressed by all studies. Given the poor CNS activity of crizotinib, the imbalance between the trials may have influenced the relative magnitude of treatment effects for brigatinib versus crizotinib and alectinib versus crizotinib.
This study has several strengths compared with previously published ITCs. The present analysis provides updated estimates for the relative efficacy of brigatinib with alectinib using the latest OS data from the ALEX trial and the final OS data from ALTA-1L. Second, for the first time, this analysis used a MAIC approach to account for imbalances in bCNS-m across the two trials. The bCNS-m were shown to be significant drivers of the treatment effect for brigatinib versus crizotinib. Third, this approach was the first attempt to adjust the confounding caused by differences in treatment crossover in the estimation of OS using sophisticated statistical methods.
Despite its strengths, this study is subject to limitations. First, while the treatment switching methods attempt to remove the bias associated with subsequent brigatinib use in the crizotinib arm, they do not attempt to adjust for any other subsequent ALK inhibitor use in either arm (Supplemental Table 5). These differences could not be accounted for without more information in the published literature. However, other subsequent therapy use was relatively balanced across treatment arms (i.e. brigatinib, crizotinib and alectinib). Therefore, any residual confounding was likely to be similar across the treatment arms. Second, IPCW and MSM assume there are no unmeasured confounding factors (i.e. all confounding variables are measured within the trial data and accounted for within the analysis). Although it is unknown whether all confounding variables have in fact been included in the analysis, randomization should, in theory, balance out both measured and unmeasured confounders. From adjusted Kaplan–Meier curves (data not shown), the IPCW outcome is based on fewer uncensored events in the crizotinib arm, while the MSM outcome is based on more uncensored events. Thus, the results from IPCW are less robust as they are based on limited information. Third, a major assumption of RPSFTM might be invalid. The assumption that the treatment effect is the same for all participants regardless of when treatment is received cannot be proved to hold. This limitation is shared with all other published RPSFTM analysis studies.
Furthermore, IPCW and MSM methods both calculate patient weights, as does the MAIC technique. Therefore, because there are potentially two sets of weights to apply and there is no published guidance on how to combine these weights, IPCW and MSM methods were not applied within the anchored MAIC methodology. Although fewer patients crossed over from the treatment arm to the control arm in ALEX than in the ALTA-1L trial (15.9% ALEX vs 58.4% ALTA-1L; Supplemental Table 2), treatment crossover in ALEX was not adjusted as this was impossible to perform without access to ALEX patient-level data. However, the fact that the unanchored comparison (unaffected by the absence of the treatment switch adjustment in ALEX) resulted in a point estimate that favored brigatinib contributes to mitigating concerns related to this issue. Last, another key difference in the design between the two trials was receipt of prior chemotherapy. However, this factor was not found to be a significant predictor of treatment effect using the ALTA-1L data. Therefore, this difference was not explored within this manuscript. Considering the stated limitations, the ideal study design would compare frontline brigatinib and alectinib in a head-to-head RCT. One RCT (NCT04318938), which is currently ongoing with an estimated completion by the end of 2025, compares brigatinib with other second-generation ALK TKIs in front- and second-line treatment. In the current absence of head-to-head evidence, ITC provides some of the only available evidence to inform clinical and economic treatment preferences or decisionsCitation24.
Conclusion
This study presents indirect estimates of relative OS efficacy for brigatinib versus alectinib. Adjustments for treatment crossover in the ALTA-1L clinical trial were applied to account for the confounding variables caused by treatment crossover, and all methods indicate that OS is comparable between the two ALK inhibitors. Brigatinib appears similar to alectinib in reducing the risk of death among overall populations as well as those stratified by bCNS-m. Adjusting for treatment crossover and bCNS-m is necessary to reduce confounding factors, and providing a more accurate estimate of relative OS as the choice of treatment switching methodology applied can have a large impact on the estimated treatment effect. Regardless of ITC methodology, OS is comparable for brigatinib versus alectinib in patients with ALK+ NSCLC previously untreated with an ALK inhibitor.
Transparency
Declaration of funding
These studies were sponsored by ARIAD Pharmaceuticals Inc., Cambridge, MA, USA, a wholly owned subsidiary of Takeda Pharmaceutical Company Limited.
Declaration of financial/other relationships
K.L.R. has disclosed that she has received consulting fees and honoraria from Amgen, AstraZeneca, Blueprint, Daiichi Sankyo, EMD Serono, Genentech, GlaxoSmithKline, Janssen, Lilly, Merck KGaA, Mirati, Seattle Genetics, Takeda and Tesaro; and research funding to institution from Genentech, Blueprint, Calithera, Daiichi Sankyo, Elevation Oncology, Janssen. H.M.L. has disclosed that he is employed by Takeda. H.C. has disclosed that she is employed by Takeda. Y.W. has disclosed that she is employed by Takeda. P.Z. has disclosed that she is employed by Takeda. S.K. has disclosed that he is employed by Model Outcomes Ltd. L.J.W. has disclosed that she is employed by Takeda. J.S. has disclosed that he is employed by Takeda. S.P. has disclosed that he has received honoraria and consulting fees from Amgen, AstraZeneca, Bayer, BeiGene, Blueprint, BMS, Boehringer Ingelheim, Daiichi Sankyo, GSK, Guardant Health, Incyte, Janssen, Lilly, Merck KGaA, MSD, Novartis, Roche, Takeda, Pfizer, Seattle Genetics, Turning Point Therapeutics and Xcovery. D.R.C. has disclosed that he has received honoraria from AstraZeneca, Takeda, Arrys/Kyn, Genoptix, G1 Therapeutics DSMB, Mersana Therapeutics, Roche/Genentech, Ignyta, Daiichi Sankyo [ILD adjudication committee], Hansoh SRC, Bio-Thera DSMB, Lycera, Revolution Med, Orion, Clovis, Celgene and Novartis; and research funding from ARIAD/Takeda. CMRO peer reviewers on this manuscript have no relevant financial or other relationships to disclose.
Author contributions
Study design: K.L.R., H.M.L., H.C., Y.W.; study investigator: K.L.R., S.P., D.R.C.; collection and assembly of data: K.L.R., H.C., S.K., Y.W.; data analysis: S.K., Y.W., H.C.; data interpretation: all authors; manuscript review and revisions: all authors; final approval of manuscript: all authors.
Supplemental Material
Download MS Word (30 KB)Acknowledgements
The authors would like to thank the patients, their families and their caregivers; the ALTA-1L and ALEX investigators and their team members at each study site; and colleagues from ARIAD Pharmaceuticals Inc., Cambridge, MA, USA, a wholly owned subsidiary of Takeda Pharmaceutical Company Limited. Professional medical writing assistance was provided by Rachel Delinger, MPH, of Peloton Advantage LLC, an OPEN Health company, Parsippany, NJ, USA, and funded by ARIAD Pharmaceuticals Inc.
Data sharing statement
The data sets generated and/or analyzed during the current study are available from the corresponding author on reasonable request to researchers who provide a methodologically sound proposal. The data will be provided after their de-identification, in compliance with data protection anonymization.
References
- Sung H, Ferlay J, Siegel RL, et al. Global cancer statistics 2020: GLOBOCAN estimates of incidence and mortality worldwide for 36 cancers in 185 countries. CA A Cancer J Clin. 2021;71(3):209–249.
- Casal-Mouriño A, Ruano-Ravina A, Lorenzo-González M, et al. Epidemiology of stage III lung cancer: frequency, diagnostic characteristics, and survival. Transl Lung Cancer Res. 2021;10(1):506–518.
- Gainor JF, Varghese AM, Ou SH, et al. ALK rearrangements are mutually exclusive with mutations in EGFR or KRAS: an analysis of 1,683 patients with non-small cell lung cancer. Clin Cancer Res. 2013;19(15):4273–4281.
- Wong DW, Leung EL, So KK, et al. The EML4-ALK fusion gene is involved in various histologic types of lung cancers from nonsmokers with wild-type EGFR and KRAS. Cancer. 2009;115(8):1723–1733.
- Koivunen JP, Mermel C, Zejnullahu K, et al. EML4-ALK fusion gene and efficacy of an ALK kinase inhibitor in lung cancer. Clin Cancer Res. 2008;14(13):4275–4283.
- Camidge DR, Kim HR, Ahn MJ, et al. Brigatinib versus crizotinib in ALK-positive non–small-cell lung cancer. N Engl J Med. 2018;379(21):2027–2039.
- Peters S, Camidge DR, Shaw AT, et al. Alectinib versus crizotinib in untreated ALK-positive non-small-cell lung cancer. N Engl J Med. 2017;377(9):829–838.
- Camidge DR, Kim HR, Ahn M-J, et al. Brigatinib versus crizotinib in ALK inhibitor–naive advanced ALK-positive NSCLC: final results of phase 3 ALTA-1L trial. J Thorac Oncol. 2021;16(12):2091–2108.
- Mok T, Camidge DR, Gadgeel SM, et al. Updated overall survival and final progression-free survival data for patients with treatment-naive advanced ALK-positive non-small-cell lung cancer in the ALEX study. Ann Oncol. 2020;31(8):1056–1064.
- Bucher HC, Guyatt GH, Griffith LE, et al. The results of direct and indirect treatment comparisons in meta-analysis of randomized controlled trials. J Clin Epidemiol. 1997;50(6):683–691.
- Workshop 21: indirect treatment comparisons: an interactive workshop on choosing the right tool for the available data [Internet] [cited 2022 Mar 25]. Available from: https://www.ispor.org/docs/default-source/presentations/91031pdf.pdf?sfvrsn=fa53447c_0
- Brigatinib for ALK-positive advanced non-small-cell lung cancer that has not been previously treated with an ALK inhibitor. Technology appraisal guidance [TA670] [Internet] [cited 2022 Mar 10]. Available from: https://www.nice.org.uk/guidance/ta670
- Brigatinib for treating ALK-positive advanced non-small-cell lung cancer after crizotinib. Technology appraisal guidance [TA571] [Internet] [cited 2022 Mar 10]. Available from: https://www.nice.org.uk/guidance/ta571/
- Robins JM, Finkelstein DM. Correcting for noncompliance and dependent censoring in an AIDS clinical trial with inverse probability of censoring weighted (IPCW) log-rank tests. Biometrics. 2000;56(3):779–788.
- Yamaguchi T, Ohashi Y. Adjusting for differential proportions of second-line treatment in cancer clinical trials. Part II: an application in a clinical trial of unresectable non-small-cell lung cancer. Stat Med. 2004;23(13):2005–2022.
- Yamaguchi T, Ohashi Y. Adjusting for differential proportions of second-line treatment in cancer clinical trials. Part I: structural nested models and marginal structural models to test and estimate treatment arm effects. Stat Med. 2004;23(13):1991–2003.
- Latimer NR, White IR, Abrams KR, et al. Causal inference for long-term survival in randomised trials with treatment switching: should re-censoring be applied when estimating counterfactual survival times? Stat Methods Med Res. 2019;28(8):2475–2493.
- Huber RM, Hansen KH, Paz Ares Rodríguez L, et al. Brigatinib in crizotinib-refractory ALK + NSCLC: 2-year follow-up on systemic and intracranial outcomes in the phase 2 ALTA trial. J Thorac Oncol. 2020;15(3):404–415.
- Reckamp KL, Lin HM, Cranmer H, et al. Indirect comparisons of brigatinib and alectinib for front-line ALK-positive non-small-cell lung cancer. Future Oncol. 2022;18(20):2499–2510.
- Faria R, Hernandez Alava M, Manca A, et al. NICE DSU technical support document 17: the use of observational data to inform estimates of treatment effectiveness in technology appraisal: methods for comparative individual patient data [Internet] [cited 2022 Mar 25]. Available from: https://nicedsu.sites.sheffield.ac.uk/tsds/observational-data-tsd
- Latimer NR, Abrams KR. NICE DSU technical support document 16: Adjusting survival time estimates in the presence of treatment switching. London (UK): National Institute for Health and Care Excellence; 2014.
- Elliott J, Bai Z, Hsieh SC, et al. ALK inhibitors for non-small cell lung cancer: a systematic review and network meta-analysis. PLoS One. 2020;15(2):e0229179.
- Wang L, Sheng Z, Zhang J, et al. Comparison of lorlatinib, alectinib and brigatinib in ALK inhibitor-naive/untreated ALK-positive advanced non-small-cell lung cancer: a systematic review and network meta-analysis. J Chemother. 2021;34(2):87–96.
- Swallow E, Patterson-Lomba O, Ayyagari R, et al. Causal inference and adjustment for reference-arm risk in indirect treatment comparison meta-analysis. J Comp Eff Res. 2020;9(10):737–750.