Abstract
Chronic kidney disease (CKD) is a global public health issue that can lead to several complications such as, kidney failure, cerebro/cardiovascular disease, and death. There is a well-documented “awareness gap” among general practitioners (GPs) to recognize CKD. As shown by estimates stemming from the Health Search Database (HSD) of the Italian College of General Practitioners and Primary Care (SIMG), no substantial changes were observed in terms of the incident rate of CKD over the last 10 years. Namely, 10.3–9.5 per 1000 new cases of CKD were estimated in 2012 and 2021, respectively. Thus, strategies to reduce under-recognized cases are needed. Early identification of CKD might improve patient’s quality of life and clinical outcomes. In this context, patient- and population-based informatic tools may support both opportunistic and systematic screening of patients at greater risk of CKD. As such, the new effective pharmacotherapies for CKD would be proficiently administered. To this aim, these two complimentary tools have been developed and will be further implemented by GPs. The effectiveness of these instruments in identifying the condition at an early stage and reducing the burden of CKD on the national health system needs to be verified according to the new regulations on medical device (MDR: (EU) 2017/745).
Chronic kidney disease (CKD) is a global public health issue leading to several complications such as kidney failure, cerebral/cardiovascular disease, and death. In the last decades, the burden of CKD has been growing, with a global prevalence of 9.1%Citation1. Furthermore, the decrease in the disability-adjusted life year (DALY) rate for CKD is not associated to a decrease in rates of CKD mortality or prevalence, suggesting a shift to mortality due to CKD occurring at an older age. By 2030, CKD is expected to become the fifth leading cause of death worldwideCitation1. In Italy, CKD afflicts more than 6 million people, with a prevalence of about 7.5% and 6.5% among men and women, respectivelyCitation2. However, it is important to highlight that only around 10% of patients affected by CKD were aware about their diseaseCitation1,Citation3.
In the Health Search Database (HSD; Italian College of General Practitioners and Primary Care), from 2012 to 2021, some increase in the yearly prevalence of CKD was observed. Namely, the cumulative prevalence of CKD ranged from 5.2% to 13% during this period. Regarding the yearly incidence (i.e. new cases of CKD by year), some decrease was observed between 2012 and 2013 with 10.3 and 7 cases per 1000, respectively. Otherwise, values ranging from 6 to 8 per 1000 were obtained from 2014 to 2021. Of note, during the SARS-CoV-2 pandemic, the incidence rate kept values ranged from 8 to 9.5 per 1000. As such, no substantial difference in capturing new cases of CKD was revealed over the last 10 years.
There is a well-documented “awareness gap” among general practitioners (GPs) to recognize CKD in several countries, including ItalyCitation3. Indeed, only 36% of the countries confer to CKD the rule of health-care priorityCitation4. In a study involving 300 GPs, which recruited a cohort of about 500,000 patients, serum creatinine levels were requested for only 17% of the patients. Although, 16% of this patient subgroup was affected by CKD (operationally defined as eGFR <60 mL/min/1.73m2), only 12.5% of them were identified as CKD sufferers by GPsCitation5,Citation6. Along this line, it was recently reported that 77% of patients with proven Stage G3 CKD were undiagnosed by their GPs in ItalyCitation7. The fact that CKD is under-recognized by GPs depends on several reasons. Firstly, there is a lack of CKD knowledge and a poor awareness of CKD guidelines and factors which are all potentially related to the awareness gap in primary careCitation8. Furthermore, CKD is asymptomatic in the first stage. It also frequently coexists with other major conditions, mainly cardiovascular diseases, which might draw most of the GP’s attention. Nevertheless, the effect of kidney disease is not limited to the renal environment but extends to cardiovascular diseases for which it is an independent risk factorCitation9,Citation10. Furthermore, as shown in the GBD 2017 Risk Factor CollaboratorsCitation11, the DALY rate for impaired kidney function is greater than that for drug use, unsafe sanitation, low physical activity, second-hand smoke, and many other dietary risk factors. In this respect, a consensus emerged on “Early Identification and Intervention in CKD”, highlighting the need to implement of effective screening coupled with risk stratificationCitation12,Citation13.
Regarding the support for GPs in the early identification of CKD, although accurate algorithms are available to raise the suspect of CKD, no effective clinical decision support systems (CDSS) have been largely implemented in primary careCitation14–16. This is not true for other chronic conditions, for whom the use of CDSS provided a significant improve in their identification and/or managementCitation17–20. As for CKD, several country-specific prediction models have been developed and validatedCitation21. They comprise algorithms gathered through several techniques, such as traditional score development and validation as well as supervised machine learning approachesCitation22,Citation23. For the latter, Random Forest, J48 algorithm, gradient boost, support vector machine, neural network and others, have been proposed for CKD prediction. However, they likely suffer from high risk of bias, which is mainly due to the absence of external validation studies. In addition, they have a poor interpretability. In this respect, Generalized Additive Models (GAMs) could represent an adequate response for CKD forecasting, given their feasibility in learning complex associations through the combination of nonlinear functions, thereby uncovering nonlinear associations and interactions and improving the predictive accuracyCitation24. Regarding the patient-based tool, by using a dashboard-based software, the GP would be able to visualize the patient’s risk estimate combined with the list of those risk factors leading to this assessment. In Italy, through MilleDSSFootnotei,Citation25 we developed such a system by which a visual reminder appears when the GP inspects patient’s Electronic Medical Records (EHRs) including at least one of the five risk factors for CKD (i.e. arterial hypertension, diabetes, cardiovascular disease, proteinuria ≥ 300 mg, creatinine >1.5 mg/dL and >1.3 mg/dL for males and women, respectively) in those still undiagnosed with the disease. In the example reported in the hand indicates the red (high risk) section, supporting GP in his/her clinical decision makingCitation21,Citation22. Currently, we are developing and testing a similar prediction model based on GAM methodologyCitation24, which will improve the predictive accuracy of the current prediction model.
Figure 1. Examples of MilleDSS (A) and GPG (B) tools (in Italian) for opportunistic and systematic screening of CKD. (A) CDSS: Dashboard shows an example of “high risk” of CKD. (B) Governance tool: process indicators with green and red cursors indicating the absence or presence of criticisms; blu cursor shows the prevalence of patients at risk of incurring in CKD (simulated data of an individual general practitioner having in charge 1535 patients).
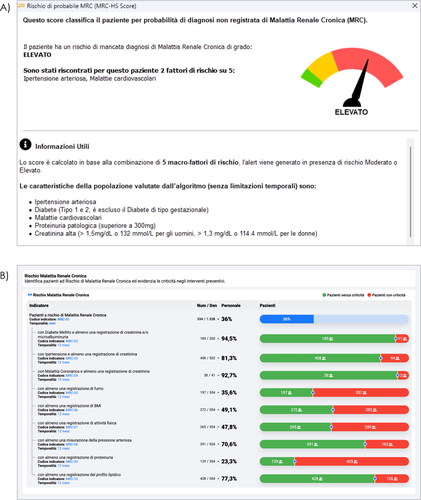
In any case, the CDSS should be complemented by other clinical governance applications, in which the GP could visualize his/her cohort of patients according to the aforementioned prediction score and other process indicatorsCitation26. By doing so, a general picture of those being at different risk levels would be complemented by specific indicators to quantify several specific aspects. For instance, among diabetes/hypertension/cardiomyopathy sufferers being tested with creatinine and/or microalbuminuria/proteinuria as well as BMI or lipid profile assessment at least once. Then, these indicators should be effectively ascertained by viewing the entire cohort at risk when the overall population is the denominator. A bar shows the related points of criticism according to “green” and “red” cursors. As in data simulation shown in , the Italian General Practice Governance (GPGFootnoteii) enables GPs to visualize such a picture of their patients. In addition to viewing the sub-groups size on or out of target (i.e. “green” or “red” cursor), clicking on the respective numbers, patient lists can be generated and ordered according to specific criteria. As such, some categories of patients might be prioritized for future visits and/or prevention, screening, or communication campaigns.
Given the crucial role of GPs in the early diagnosis of CKD, there is the need to require accessible and targeted CKD detection in primary care. Nevertheless, there are still uncertainties on the benefit/risk ratio related to screening or case finding per se. This is particularly relevant considering that cost-benefit analyses including new medications, namely sodium-glucose cotransporter-2 inhibitors (SGLT-2i), are not still available. As for screening programs, many of these showed a lower benefit than expected, and screening for CKD is not excludedCitation27–29. Indeed, given the unclear relationship between intensive screening (i.e. serum creatinine evaluations) and overt kidney disease, a potential harm exerted by treatments on asymptomatic patients cannot be excluded. As such, there is evidence that screening is unlikely cost-effective, whereas case finding appears the best approach in terms of cost and benefitCitation13,Citation30,Citation31. This is why clinical investigations should be tailored on certain risk categories, for which the concurrent risk factors would increase the development or the progression of CKD. Along this line, the GPs’ workload in screening their patients for CKD would be affordable. Namely, Italian GPs are allowed to enlist up to 2000 patients on the basis of the regional health care system. According to the data simulation report shown in , one-third of these patients might be at risk of developing CKD across different risk categories. In this context, the presence of supportive informatic tools might be pivotal in providing information that entails cost-effective screeningsCitation13,Citation30,Citation32 based on opportunistic and systematic approaches using CDSS and governance tools (i.e. MilleDSS and GPG in Italy), respectively.
In Italy, GPs are independent contractors for the national health service. They invest in their own computer-based tools, thereby participating in networks and creating an epidemiological real-time observatory. Therefore, a real-life comparator could be available given that numerous subgroups of them will benefit these “automatisms”. As such, clinical evidence will depend on the effectiveness of these instruments to reduce the burden of CKD according to the new medical device regulation (MDR: (EU) 2017/745). This is particularly relevant when new effective medications have recently become available for CKD therapyCitation33–36.
In conclusion, given the multiple facets of CKD, robust tools are needed to early and effectively identify most of the patients with CKD and promptly intervene with appropriate care.
Transparency
Declaration of funding
This article was funded by Genomedics Srl which has the ownership and copyright of MilleDSS technology.
Declaration of financial/other relationships
Lapi F. and Marconi E. provided consultancies in protocol preparation for epidemiological studies and data analyses for AstraZeneca, Mundipharma, MSD. Medea G. and Cricelli C. provided clinical consultancies for AstraZeneca, Mundipharma, MSD. Cricelli I is an employee of Genomedics Srl. Peer reviewers on this manuscript have no relevant financial or other relationships to disclose.
Author contributions
Cricelli I., Piccinocchi C., Medea G., Cricelli C. and Lapi F. conceptualized the study. Cricelli I., Marconi E. and Lapi F. wrote the manuscript. Lapi F and Cricelli C. are responsible for the integrity of the work. All aspects of the study were led by the authors.
Acknowledgements
None.
Notes
i MilleDSS is a registered trademark of Genomedics Srl - Florence, Italy.
ii GPG is a registered trademark of Genomedics Srl - Florence, Italy.
References
- Bikbov B, Purcell CA, Levey AS, et al. Global, regional, and national burden of chronic kidney disease, 1990–2017: a systematic analysis for the global burden of disease study 2017. Lancet. 2020;395(10225):709–733.
- De Nicola L, Donfrancesco C, Minutolo R, et al. Prevalence and cardiovascular risk profile of chronic kidney disease in Italy: results of the 2008–12 national health examination survey. Nephrol Dial Transplant. 2015;30(5):806–814.
- Pesce F, Pasculli D, Pasculli G, et al. “The disease awareness innovation network” for chronic kidney disease identification in general practice. J Nephrol. 2022;35(8):2057–2065.
- Kelly DM, Anders HJ, Bello AK, et al. International society of nephrology global kidney health atlas: structures, organization, and services for the management of kidney failure in Western Europe. Kidney Int Suppl. 2021;11(2):e106–e118.
- Minutolo R, Lapi F, Chiodini P, et al. Risk of ESRD and death in patients with CKD not referred to a nephrologist: a 7-year prospective study. Clin J Am Soc Nephrol. 2014;9(9):1586–1593.
- Minutolo R, De Nicola L, Mazzaglia G, et al. Detection and awareness of moderate to advanced CKD by primary care practitioners: a cross-sectional study From Italy. Am J Kidney Dis. 2008;52(3):444–453.
- Tangri N, De Nicola L. Findings and implications of the REVEAL-CKD study investigating the global prevalence of undiagnosed stage G3 chronic kidney disease. EMJ. 2022;2022:60–65.
- John Sperati C, Soman S, Agrawal V, et al. Primary care physicians’ perceptions of barriers and facilitators to management of chronic kidney disease: a mixed methods study. PLoS One. 2019;14(8):e0221325.
- Sarnak MJ, Levey AS, Schoolwerth AC, et al. Kidney disease as a risk factor for development of cardiovascular disease: a statement from the American heart association councils on kidney in cardiovascular disease, high blood pressure research, clinical cardiology, and epidemiology and prevention. Circulation. 2003;108(17):2154–2169.
- Levin A, Stevens PE, Bilous RW, Coresh J, de Francisco ALM, de Jong PE, et al. Kidney disease: improving global outcomes (KDIGO) CKD work group. KDIGO 2012 clinical practice guideline for the evaluation and management of chronic kidney disease. Vol. 3. Kidney International Supplements; 2013.
- Stanaway JD, Afshin A, Gakidou E, et al. Global, regional, and national comparative risk assessment of 84 behavioural, environmental and occupational, and metabolic risks or clusters of risks for 195 countries and territories, 1990–2017: a systematic analysis for the global burden of disease study 2017. Lancet. 2018;392(10159):1923–1994.
- Shlipak MG, Tummalapalli SL, Boulware LE, et al. The case for early identification and intervention of chronic kidney disease: conclusions from a kidney disease: improving global outcomes (KDIGO) controversies conference. Kidney Int. 2021;99(1):34–47.
- Inker LA, Astor BC, Fox CH, et al. KDOQI US commentary on the 2012 KDIGO clinical practice guideline for the evaluation and management of CKD. Am J Kidney Dis. 2014;63(5):713–735.
- Carroll JK, Pulver G, Dickinson LM, et al. Effect of 2 clinical decision support strategies on chronic kidney disease outcomes in primary care: a cluster randomized trial. JAMA Netw Open. 2018;1(6):e183377.
- Joshi P, Navaneethan SD, Estrella MM. Moving beyond tools and building bridges: lessons learned From a CKD decision support in primary care. Kidney Med. 2022;4(7):35789757.
- Samal L, D’Amore JD, Gannon MP, et al. Impact of kidney failure risk prediction clinical decision support on monitoring and referral in primary care management of CKD: a randomized pragmatic clinical trial. Kidney Med. 2022;4(7):35866010.
- Rossom RC, Crain AL, O’Connor PJ, et al. Effect of clinical decision support on cardiovascular risk among adults With bipolar disorder, schizoaffective disorder, or schizophrenia: a cluster randomized clinical trial. JAMA Netw Open. 2022;5(3):E220202.
- Gold R, Larson AE, Sperl-Hillen JM, et al. Effect of clinical decision support at community health centers on the risk of cardiovascular disease: a cluster randomized clinical trial. JAMA Netw Open. 2022;5(2):e2146519.
- Shi X, He J, Lin M, et al. Comparative effectiveness of team-based care with and without a clinical decision support system for diabetes management nagecluster randomized trial. Ann Intern Med. 2023;176(1):49–58.
- Kharbanda EO, Nordin JD, Sinaiko AR, et al. TeenBP: development and piloting of an EHR-linked clinical decision support system to improve recognition of hypertension in adolescents. eGEMs. 2015;3(2):9.
- Nelson RG, Grams ME, Ballew SH, et al. Development of risk prediction equations for incident chronic kidney disease. JAMA. 2019;322(21):2104–2114.
- Ilyas H, Ali S, Ponum M, et al. Chronic kidney disease diagnosis using decision tree algorithms. BMC Nephrol. 2021;22(1):273.
- Andaur Navarro CL, Damen JAA, Takada T, et al. Risk of bias in studies on prediction models developed using supervised machine learning techniques: systematic review. The BMJ. 2021;2021:375.
- Higdon R. Generalized additive models. In: Encyclopedia of systems biology. New York (NY): Springer New York; 2013. p. 814–815.
- Cricelli I, Marconi E, Lapi F. Clinical decision support system (CDSS) in primary care: from pragmatic use to the best approach to assess their benefit/risk profile in clinical practice. Curr Med Res Opin. 2022;38(5):827–829.
- Cricelli I, Lapi F, Montalbano C, et al. Mille general practice governance (MilleGPG): an interactive tool to address an effective quality of care through the Italian general practice network. Prim Health Care Res Dev. 2013;14(4):409–412.
- Manns B, Hemmelgarn B, Tonelli M, et al. Population based screening for chronic kidney disease: cost effectiveness study. BMJ. 2010;341(7781):1036.
- Boulware LE, Jaar BG, Tarver-Carr ME, et al. Screening for proteinuria in US adults: a cost-effectiveness analysis. JAMA. 2003;290(23):3101–3114.
- Fink HA, Ishani A, Taylor BC, et al. Screening for, monitoring, and treatment of chronic kidney disease stages 1 to 3: a systematic review for the US preventive services task force and for an American college of physicians clinical practice guideline. Ann Intern Med. 2012;156(8):570–581.
- Levey AS, Eckardt KU, Tsukamoto Y, et al. Definition and classification of chronic kidney disease: a position statement from Kidney Disease: Improving Global Outcomes (KDIGO)z. Kidney Int. 2005;67(6):2089–2100.
- Tonelli M, Dickinson JA. Early detection of CKD: implications for low-income, middle-income, and high-income countries. J Am Soc Nephrol. 2020;31(9):1931–1940.
- Sarnak MJ, Levey AS, Schoolwerth AC, et al. Kidney disease as a risk factor for development of cardiovascular disease: a statement from the American heart association councils on kidney in cardiovascular disease, high blood pressure research, clinical cardiology, and epidemiology and prevention. Hypertension. 2003;42(5):1050–1065.
- Heerspink HJL, Kosiborod M, Inzucchi SE, et al. Renoprotective effects of sodium-glucose cotransporter-2 inhibitors. Kidney Int. 2018;94(1):26–39.
- Heerspink HJL, Stefánsson BV, Correa-Rotter R, et al. Dapagliflozin in patients with chronic kidney disease. N Engl J Med. 2020;383(15):1436–1446.
- Perkovic V, Jardine MJ, Neal B, et al. Canagliflozin and renal outcomes in type 2 diabetes and nephropathy. N Engl J Med. 2019;380(24):2295–2306.
- Minutolo R, Liberti ME, Provenzano M, et al. Generalizability of DAPA-CKD trial to the real-world setting of outpatient CKD clinics in Italy. Nephrol Dial Transplant. 2022;37(12):2591–2593.