Abstract
Objective: In rheumatoid arthritis (RA), it is of major importance to identify non-responders to tumour necrosis factor-α inhibitors (TNFi) before starting treatment, to prevent a delay in effective treatment. We developed a protein score for the response to TNFi treatment in RA and investigated its predictive value.
Method: In RA patients eligible for biological treatment included in the BiOCURA registry, 53 inflammatory proteins were measured using xMAP® technology. A supervised cluster analysis method, partial least squares (PLS), was used to select the best combination of proteins. Using logistic regression, a predictive model containing readily available clinical parameters was developed and the potential of this model with and without the protein score to predict European League Against Rheumatism (EULAR) response was assessed using the area under the receiving operating characteristics curve (AUC-ROC) and the net reclassification index (NRI).
Results: For the development step (n = 65 patient), PLS revealed 12 important proteins: CCL3 (macrophage inflammatory protein, MIP1a), CCL17 (thymus and activation-regulated chemokine), CCL19 (MIP3b), CCL22 (macrophage-derived chemokine), interleukin-4 (IL-4), IL-6, IL-7, IL-15, soluble cluster of differentiation 14 (sCD14), sCD74 (macrophage migration inhibitory factor), soluble IL-1 receptor I, and soluble tumour necrosis factor receptor II. The protein score scarcely improved the AUC-ROC (0.72 to 0.77) and the ability to improve classification and reclassification (NRI = 0.05). In validation (n = 185), the model including protein score did not improve the AUC-ROC (0.71 to 0.67) or the reclassification (NRI = −0.11).
Conclusion: No proteomic predictors were identified that were more suitable than clinical parameters in distinguishing TNFi non-responders from responders before the start of treatment. As the results of previous studies and this study are disparate, we currently have no proteomic predictors for the response to TNFi.
Rheumatoid arthritis (RA) is a chronic, disabling disease that mainly affects the synovial joints. The disease is autoimmune in origin and affects 0.5–1% of the population in industrialized countries (Citation1, Citation2). Although the introduction of therapy using tumour necrosis factor-α-inhibitors (TNFi) has dramatically improved the outlook for RA patients, a substantial proportion of patients (approximately 30–40%) fails to respond to TNFi therapy (Citation3, Citation4). As we currently cannot differentiate at the start of therapy between patients who will respond and those who will not (Citation5), TNFi treatment is administered in an empirical trial-and-error approach. This entails therapeutic inefficiency in a substantial number of patients, owing to the 3–6 month observation period before clinical response can be judged. In this time-frame, the non-responding patient may suffer from uncontrolled disease with the potential of irreversible damage and harmful side effects from treatment. Another problem is the high costs incurred with this treatment. The challenge is therefore to identify responders and non-responders to specific therapies beforehand, and to initiate optimal treatment.
TNFi targets tumour necrosis factor-α (TNF-α), which is central in the pathogenesis of RA and acts within a complex network of cells and cytokines that relate to inflammation (Citation6). It is therefore likely that proteomic approaches, in which many cytokines are measured simultaneously and are jointly investigated, are capable of classifying RA patients at baseline according to their response to TNFi treatment. We explored the baseline serum proteomic predictors that may identify responders and non-responders to TNFi, using a panel of proteins that were all specifically related to inflammation, and aimed to validate our models in a new cohort of consecutively included patients. We investigated whether a combination of proteins and clinical parameters could predict the response to TNFi treatment in RA patients more accurately than clinical parameters alone.
Method
Patients
Patients who were initiated on TNFi treatment were selected from the BiOCURA cohort (Biologicals and Outcome, Compared and predicted in Utrecht region, in Rheumatoid Arthritis). BiOCURA is an observational cohort, in which RA patients eligible for biological treatment according to regular clinical practice were enrolled and followed up until 1 year after start of treatment, in one academic hospital and seven regional hospitals in the Netherlands. To reflect clinical practice, no patients were excluded beforehand. Reinclusion after switching to a different biological treatment was possible. The study was approved by the local ethics committee of the University Medical Center Utrecht and the institutional review boards of the participating centres (see Acknowledgements). Informed consent was obtained from each patient.
Protein measurements
Trained nurses gathered all data during a separate visit, which included all clinical parameters, joint counts, and blood tests, before the first dose of the treatment was given. Blood was processed immediately after collection and serum samples were stored at −80°C until analysis.
Serum levels of 53 inflammatory proteins were measured using a multiplex immunoassay based on Luminex technology (xMAP®; Luminex, Austin, TX, USA), which was developed in house, validated, and ISO9001:2008 certified, as described previously (Citation7–Citation9). In short, non-specific heterophilic immunoglobulins, such as rheumatoid factors, were preabsorbed from all samples with Heterblock (Omega Biologicals, Bozeman, MT, USA). Next, samples were incubated with antibody-conjugated MagPlex microspheres for 1 h at room temperature with Heterblock continuous shaking, followed by 1 h incubation with biotinylated antibodies, and 10 min incubation with phycoerythrin-conjugated streptavidin diluted in high-performance ELISA (HPE) buffer (Sanquin, Amsterdam, the Netherlands). Acquisition was performed with the Bio-Rad FLEXMAP 3D® (Bio-Rad Laboratories, Hercules, CA, USA) in combination with xPONENT software version 4.2 (Luminex). Data were analysed by five-parametric curve fitting using Bio-Plex Manager software, version 6.1.1 (Bio-Rad). The selection of 53 proteins is displayed in Supplementary Table S1. The xMAP analyses for the development and validation were performed in October 2014 and June 2016, respectively.
Outcome
Disease activity was assessed using the Disease Activity Score based on 28-joint count (DAS28) (Citation10). Response to treatment was based on the European League Against Rheumatism (EULAR) response criteria (Citation11). The EULAR distinguishes among non-response, moderate response, and good response. As our main focus was to predict a good response in contrast to a moderate response, non-response and moderate response were combined and compared with good response. EULAR good response is defined as a DAS28 improvement ≥ 1.2 and an absolute DAS28 ≤ 3.2 after treatment. Patients who discontinued therapy before 3 months owing to side effects were excluded from the study because they cannot be considered merely missing at random and are not necessarily non-responders.
Analyses
All treatment courses with TNFi treatment (i.e. adalimumab, etanercept, golimumab, infliximab, or certolizumab) were included in this study, including courses for patients who had switched from one to another TNFi therapy within BiOCURA. The 276 TNFi treatment courses were divided into separate groups based on the treatment starting dates. The development of the models was performed on cases included between October 2012 and March 2014 (n = 65), whereas validation was performed on TNFi treatment cases included from June 2009 and October 2012 (n = 185). Development and validation were performed on cohorts split by treatment onset dates (i.e. non-random split), which leads to a different clinical setting for the inclusion of patients per cohort. Therefore, the validation can be considered to lie in between internal and external validation (Citation12). Details for missing data handling by multiple imputations can be found in the supplementary text. The statistical analyses of the development and validation steps are described in the subsequent paragraphs and summarized in .
Figure 1. Overview of statistical analyses. The pathways denoted by black–dotted and grey–straight lines represent the analyses performed in the development and validation steps, respectively. AUC-ROC, area under the receiving operating characteristic curve; GEE, generalized estimation equations; NPV, negative predictive value; NRI, net reclassification index; PLS, partial least squares; par., parameters; sel., selection.
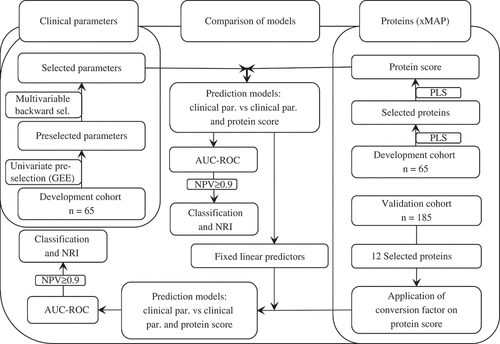
Development
In the development step, proteins were measured in 65 samples and log-transformed to approximate a normal distribution. These log-transformed values were used in a partial least squares (PLS) analysis, a supervised clustering algorithm, to select the proteins making the greatest contribution and build the protein score that predicts DAS28 at 3 months. Proteins that were not normally distributed were not entered in PLS as they not only disturb PLS, but also are unfeasible regarding (regression-based) risk prediction. The inflammation marker C-reactive protein (CRP; log-transformed value) and DAS28 at baseline were always included, because they could be influenced by previous treatment and, in turn, influence the inflammatory profile and classification of the EULAR response. The most important proteins were selected based on variable importance in projection (VIP) scores ≥ 1 in combination with the highest factor loadings, so that no more than 15 proteins were included. Subsequently, this selection of proteins, together with baseline CRP and DAS28, was included in the PLS procedure again to create the initial protein score. In this procedure, the PLS algorithm suggests the best linear fit of the proteins with the DAS28 at 3 months, considering the results of the supervised clustering, e.g. 1.5 * ln(TNF-α) + 0.7 * ln(IL-6), etc.
The inclusion of patients who were reincluded after switching to a different TNFi resulted in two-level data (i.e. data from different patients and repeated data within patients), which we accounted for by using generalized estimating equation (GEE) modelling in the preselection of clinical parameters (Citation13). This univariate analysis was carried out for all clinical baseline variables () including a health-related quality of life questionnaire [EuroQol 5 Dimensions (EQ-5D)] and Health Assessment Questionnaire (HAQ), using GEE with logit link function, and parameters were selected with a p value < 0.2. All selected clinical parameters were then entered in a (full) logistic regression model and were removed one by one using a backward selection procedure (p < 0.1), while baseline CRP and DAS28 were always included, to form the ‘clinical’ model. These clinical parameters were also used as covariates together with the protein score in the ‘combined’ model. Comparison of the clinical and combined models allows determination of the added value of measuring proteins for prediction of response to TNFi over parameters already available in clinical practice. The discriminating potential of the models was assessed using the area under the receiver operating characteristics curve (AUC-ROC). Then, for each model, a cut-off was chosen based on a negative predictive value (NPV) > 90%. If patients failed to meet this cut-off, their probability of good response was < 10%, which was considered a clinically relevant cut-off to consider an alternative (combination) treatment; all these patients were classified in the ‘low probability of response’ category. All patients who met this cut-off were assigned to the ‘normal probability of response’ category. Based on these categories, the number of patients classified in the low probability of response category was compared between the models, to determine whether the combined model is more sensitive in classifying non-responders. As an indication, a number needed to test (NNT) by the combined model before one more patient is classified into the low category of response was calculated. In addition, and also based on these categories, the net reclassification index (NRI) was calculated to determine the ability of the combined model to reclassify patients as predicted by the clinical model into a (more) correct category. For example, when a future non-responder is classified at baseline as having a normal probability of response by the clinical model, but is classified into the low-probability category in the combined model, the reclassification is ‘more correct’ and the NRI for non-responders increases, as does the total NRI (= NRI for responders + NRI for non-responders).
Table 1. Baseline characteristics of development (n = 65) and validation cohort (n = 185) before multiple imputation.
Validation
In the validation step, the selected proteins from the development step were measured with xMAP technology in an additional 185 patients. Subsequently, the two prediction models from the development step were recalibrated by entering the prediction rule (i.e. the linear predictor from the developed models, e.g. 0.8 * Protein score + 0.2 * Age + 3 * Gender, etc.) in a logistic regression model.
The AUC-ROC was calculated and compared between the clinical model and the combined model, and after setting the predefined cut-offs the added value of the protein score was analysed by comparing the classification and the NRI.
Software
The PLS analyses were performed in SAS version 9.2 (SAS institute, Cary, NC, USA), and the multiple imputation as well as the development and validation steps of the prediction models in SPSS version 21.0.0.0 (IBM Corp., Armonk, NY, USA).
Results
Baseline characteristics
Baseline characteristics for patients in the development and validation cohorts are shown in . Notable differences in the validation cohort, which was analysed a few years earlier than the development cohort, were a higher CRP (median 6.0 mg/L vs 4.0 mg/L, p = 0.011), higher DAS28 (4.5 vs 4.1, p = 0.019), and lower concomitant methotrexate use (71.9% vs 98.5%, p < 0.001). Preferences for certain TNFi treatments changed over time as a result of commercial influences, e.g. more etanercept was administered in recent years (i.e. in the development cohort).
Development of protein score
Of all 53 proteins, 38 were near normally distributed after log transformation and were used for PLS analysis. Regarding the other 15 proteins that were rejected, usually many values fell below the detection limit, hampering the use of these protein measurements in risk prediction. By selection on VIP ≥ 1 and a factor loading of > 0.135, 12 proteins were selected: CCL3 (macrophage inflammatory protein 1a, MIP1a), CCL17 (thymus and activation-regulated chemokine, TARC), CCL19 (macrophage inflammatory protein, MIP3b), CCL22 (macrophage-derived chemokine, MDC), interleukin-4 (IL-4), IL-6, IL-7, IL-15, soluble cluster of differentiation 14 (sCD14), sCD74 (macrophage migration inhibitory factor, MIF), soluble IL-1 receptor I (sIL-1-RI), and soluble tumour necrosis factor receptor II (sTNF-RII). A protein score was created by entering these proteins in PLS again (Supplementary Table S2).
Development of models
After univariate and multivariable selection, the clinical parameters CRP, DAS28, and the HAQ remained and a prediction model with and without the protein score was fitted to the data (). The clinical model predicted response with an AUC-ROC of 0.72 (0.64–0.80), whereas the combined model including the protein score resulted in an AUC-ROC of 0.77 (0.70–0.85). When the prediction models were applied to classify patients into response categories, the combined model was able to assign more patients into the category of low probability on EULAR good response, with 28.2% versus 21.5% respectively, with an NNT of 15 before one extra patient was classified into the low category (). When the redistribution of individual patients was investigated after addition of the protein score, the reclassification of non-responders was 8.2% better and that of responders 3.3% lower, resulting in an NRI of 0.05.
Table 2. Models for (baseline) prediction of response in development cohort (n = 65).
Table 3. Risk categories for probability on European League Against Rheumatism (EULAR) good response in the development cohort (n = 65).
Two models predicting response were developed: one clinical model containing the DAS28, CRP, and HAQ, and a combined model including the same clinical parameters as well as the protein score (containing a combination of the 12 best predicting proteins out of all 53 proteins). Shown are the odds ratios and statistical significance of all parameters when entered in the multivariable GEE model with response to treatment as outcome. The two models were compared for their predicting performance: the clinical model showed an AUC-ROC of 0.72 [95% confidence interval (CI) 0.59–0.86], whereas the combined model showed a higher predictive ability with an AUC-ROC of 0.77 (95% CI 0.64–0.90).
To implement a predictive tool for response to treatment requires guidance on how to use the result of this tool in clinical practice. Therefore, a cut-off was determined where patients had such a low chance of a good response to TNFi that they could be advised not to start treatment. This cut-off was defined as a probability < 10% of a good response. The proportion of patients with this low chance of response, as well as those with a normal risk, is shown for all 65 patients, as predicted by the clinical model and by the combined model. As the implementation of the better performing combined model requires expensive protein measurements, we calculated the NNT to gain some insight into the cost–benefit ratio. The NNT represents the number of patients who require protein measurements, so that one extra patient can be classified as having a low chance of response and thus can be advised not to start TNFi treatment.
Validation of models
We noticed on the xMAP analyses of the validation cohort that there was a decay in concentration for 10 out of 12 proteins (especially the interleukins), which may relate to the longer storage time at −80°C (Supplementary Table S3). In order not to involuntarily adjust the relative weight of each protein in the protein score, the protein levels in the validation cohort had to be adjusted so that the means and standard deviations (SDs) were comparable with the development step. We did so by converting the log-transformed value in the validation cohort to a Z score with mean = 0 and SD = 1, then multiplying this Z score by the SD of the corresponding protein in the development cohort, and lastly adding the mean value of the protein in the development cohort. These adjusted proteins were then put in the original protein score, without any refitting of the relative weight between proteins or refitting with respect to the outcome. The calculated prediction score from the clinical and combined models were applied in the validation cohort, which resulted in an AUC-ROC of 0.71 (0.63–0.79) and 0.67 (0.59–0.75) for the model without and with the protein score, respectively. The model including the protein score was not able to classify more patients into the low probability of response category (17.3% vs 6.1% of total) () and the NNT of −9 indicates that for every nine patients classified by the combined model, one fewer patient is classified into the low probability of response category. When the reclassification was investigated, classification of non-responders was 14.6% lower and that of responders 3.5% higher, resulting in an NRI of −0.11.
Table 4. Risk categories for European League Against Rheumatism (EULAR) good response in the validation cohort (n = 185).
A probability < 10% of a good response was used to determine which patients had such a low chance of a good response to TNFi that they could be advised not to start treatment. The proportion of patients with this low chance of response, as well as those with a normal risk, are shown for all 185 patients in the validation cohort, as predicted by the clinical model and by the combined model. The NNT represents the number of patients who require protein measurements, so that one extra patient can be classified as having a low chance of response and thus can be advised not to start TNFi treatment. The negative NNT implies that the addition of protein measurement results in fewer patients being correctly classified by the new model ().
Discussion
In this study, we aimed to develop a protein score that is predictive for the response to TNFi treatment in RA, and to investigate whether this score is of added predictive value over using clinical parameters alone. We showed that the protein score combined with clinical parameters initially improved the prediction of the TNFi treatment response compared with clinical parameters alone (including DAS28, CRP, and the HAQ), although this could not be validated in a separate cohort of consecutively included patients. The clinical model even outperformed the combined model in the validation cohort. Using DAS28, CRP, and the HAQ, which are parameters easily accessible in the clinical setting, 17% of the validation cohort could be classified in the low-risk category (< 10%). However, it is questionable whether these are truly useful and relevant parameters, as the low DAS28 already limits options for a good response considerably (it is more difficult to achieve a DAS28 change > 1.2) and a high HAQ is to some extent indicative for treatment-refractory RA. It is therefore not expected that patients with a low DAS28 and high HAQ will have a better response to any non-TNFi.
Proteomic approaches to the prediction of response have been studied before (Citation14–Citation21). An overview of these studies and reported results is presented in . Two studies found no predictors of response, of which one study did not identify any possible predictors in the discovery phase (Citation16), whereas the other study failed to validate the results of the discovery phase (Citation20). The other six studies all proposed predictors of response to therapy; however, none of these studies performed a technical replication or separate validation in new patients, and the overlap of discovered predictors between studies is restricted to CCL2 (monocyte chemoattractant protein-1, MCP-1), which has been cross-validated in two studies (Citation14, Citation15). However, CCL2 was not shown to be predictive in our study and was not identified in the studies using mass spectrometry (Citation17, Citation18), implying that it is not a consistent predictor. Several other proteins proposed as predictors in earlier studies were measured in our xMAP panel, including chemokine (C-X-C motif) ligand 4 (CXCL4), granulocyte–macrophage colony-stimulating factor (GM-CSF), IL-1α, IL-1β, IL-6, IL-8, IL-15, IL-19, IP-10, and TNF-α. However, none of these parameters was associated with response in the development step, except for IL-15, which could not be validated and was also not identified in the studies using mass spectrometry. The disparate results between all studies to date may be explained by differences in demographic characteristics, serology status, the TNFi used, concomitant treatments, and different techniques; however, the possibility of false-positive findings and the absence of biologically true predictors of response cannot be excluded.
Table 5. Non-exhaustive overview of studies with a proteomic approach to identify baseline predictive proteins regarding response to tumour necrosis factor-α inhibitor (TNFi) treatment.
Although proteomic approaches have thus far failed to provide predictors of response to treatment, they are capable of assessing current disease activity. Moreover, a multi-biomarker disease activity (MBDA) test for RA has been developed (Citation22) and validated in several external cohorts (Citation23–Citation25). Although careful clinical judgement cannot be replaced by any biomarker assay, the MBDA test could possibly be used to biochemically track changes in disease activity over time. As for the prediction of response, the abilities of the MBDA test have yet to be investigated. However, it has been shown that the baseline MBDA score is a significant prognostic factor for radiographic progression, according to one study (Citation26), but is not able to predict radiographic progression over time better than DAS28, according to another study (Citation23). In addition, one study found hardly any correlation between the MBDA score and several disease activity measurements in RA patients treated with biologicals (Citation27). In our study we were not able to test its predictive ability for therapeutic response, as the individual parameters within the MBDA were not all covered by the proteomic platform.
The results should be viewed within the perspective of the limitations of this study. First, we used GEE to adjust for the extra level in the data; however, we were unable to use this technique in validation as no universal prediction score (i.e. linear predictor) can be derived from the GEE model that can be applied in another cohort. As a sensitivity analysis, therefore, we fitted a clinical and combined model using GEE to the data in the validation cohort, containing the individual parameters instead of the prediction scores. The AUC-ROC of both models was 0.72 (0.64–0.80), again indicating that no advantage is gained by including the protein score. Another weak point of this study is the large differences in protein concentration between the development and validation cohorts, which was probably related to a longer storage time and subsequent degradation (Citation28). The means and SDs of the (log-transformed) protein concentrations were therefore rescaled to the values of the newest samples so as not to influence the protein score. However, one important assumption for this adjustment is that the actual protein concentrations of the patients in the validation cohort are likely to follow the same distribution as in the development cohort, which may not be the case considering the higher baseline CRP and DAS28 in the validation cohort. However, as none of the selected proteins significantly correlated with baseline DAS28 (all p > 0.05), and only IL-6 (r = 0.255, p = 0.042) and sCD14 (r = 0.294 p = 0.018) weakly correlated with CRP, the expected influence of baseline characteristics in the observed differences in protein concentrations between the development and validation cohorts is small. Lastly, we used serum instead of plasma for the proteomic analyses. Serum, being extracted after coagulation has taken place, has several disadvantages. The coagulation cascade is a complex biochemical process which involves activation of platelets and other cellular components, resulting in the release of various proteins. Therefore, the difference between serum and plasma in proteomic profile typically involves platelet-related markers, such as IL-6, CCL5, CXCL4, CXCL8, and vascular endothelial growth factor A (Citation29–Citation32). Of these, only IL-6 was selected as a potential marker. Therefore, it appears that plasma would not have resulted in a completely different selection of proteins, and would have provided replicable results.
Heterogeneity plays a large role in RA research. Patients differ in all aspects of the disease, e.g. genetic susceptibility, autoantibody status, erosive disease, pain perception, previous failed treatments, and efficacy and tolerability of concomitant treatments. These interpatient differences account for the difficulty in formulating subgroups using only several clinical parameters and biomarkers. In addition, even though the classification of a patient’s (universal) clinical response to therapy can be standardized, the classification is subject to heterogeneity in response patterns over time (early response, late response, loss of response, etc.), rapidly fluctuating disease activities, and subjective influences. The DAS28 also has the disadvantage that it omits the assessment of regularly affected joins, such as those in the foot. The majority of the patients with a tender/swollen joint score of zero at baseline had involvement of the feet that prompted the prescription for a TNFi, which results in misclassification of these patients in categories with low disease activity and limits the ability to detect a EULAR response. Clinical and biological heterogeneity and (mis)classification of response make the quest for biomarkers of response very challenging and will hamper future research on this topic, unless we find solutions for these issues.
As RA is a heterogeneous disease, it creates the appropriate conditions to distinguish phenotypes for personalized treatment approaches. So far, however, we do not know which phenotypes are of importance and how to characterize these. In this study, we used only proteins (and clinical characteristics) to subclassify RA patients. The fact that we and others did not find an association with response could be related to an insufficient phenotyping of RA, as this may be reflected not entirely by proteins but also by genomics, transcriptomics, miRNAomics, metabolomics, and the exposome. Theoretically, an integration of multiple high-throughput platforms will approximate RA phenomics better than one ‘omics’ can. However, the multidimensional biological matrices and methodological techniques required for such integration, such as in systems biology, are not yet available. Phenotyping by systems biology should thus be further developed, as this is better suited to exploit the full potential of the data and thereby determine the biological state of a cell population.
Conclusion
This study showed that the combination of a proteomic score and clinical parameters is not able to identify TNFi non-responders and responders before the start of treatment. As earlier studies failed to produce reproducible results, it is questionable whether proteomics are useful in the prediction of response to TNFi treatment. Future studies on this topic should at least validate results in a separate cohort, to ensure the reproducibility and validity of the proposed proteomic predictor(s).
cuppen_supplementary_material.pdf
Download PDF (239.3 KB)Acknowledgements
We thank A Concepcion, K Coeleveld, and K van der Wurff-Jacobs for bio-banking; KAL Schrijvers-te-Brake, A Sloeserwij, J Nijdeken, M van den Bosch, and B Sanaan, who facilitated in the acquisition of data; and the members of the Society for Rheumatology Research Utrecht (SRU) for including patients. We are grateful to all of the patients who participated in this study. Contributing SRU hospitals and contacts: University Medical Center Utrecht, Dr J Tekstra; Antonius, Hospital Nieuwegein/Utrecht, Dr EJ ter Borg; Diakonessen Hospital Utrecht, Dr Y Schenk; Meander Medical Center Amersfoort, Dr Linn-Rasker; Sint Maartenskliniek Woerden, Dr WH van der Laan; Hospital Sint Jansdal Harderwijk, Dr DG Kuiper-Geertsma; Tergooi Hospital Hilversum, Dr M Nabibux; Flevo Hospital Almere, Dr CM Verhoef.
Multiplex immunoassays were developed in house, and validated and performed at the Multiplex core facility of the Laboratory for Translational Immunology (ISO9001 certified) of the University Medical Center Utrecht.
This work was supported by Bristol-Myers Squibb; Center for Translational Molecular Medicine; F. Hoffmann-La Roche; ZonMW: The Netherlands Association for Health Research and Development (152001008); UCB; Foundation Friends of the University Medical Center Utrecht; Pfizer; and Schering-Plough.
Additional information
Funding
References
- Wolfe AM, Kellgren JH, Masi AT. The epidemiology of rheumatoid arthritis: a review. II. Incidence and diagnostic criteria. Bull Rheum Dis 1968;19:524–9.
- Silman AJ, Pearson JE. Epidemiology and genetics of rheumatoid arthritis. Arthritis Res 2002;4(Suppl 3):S265–72.
- Weinblatt ME, Kremer JM, Bankhurst AD, Bulpitt KJ, Fleischmann RM, Fox RI, et al. A trial of etanercept, a recombinant tumor necrosis factor receptor: fcfusion protein, in patients with rheumatoid arthritis receiving methotrexate. N Engl J Med 1999;340:253–9.
- Keystone EC, Kavanaugh AF, Sharp JT, Tannenbaum H, Hua Y, Teoh LS, et al. Radiographic, clinical, and functional outcomes of treatment with adalimumab (a human anti-tumor necrosis factor monoclonal antibody) in patients with active rheumatoid arthritis receiving concomitant methotrexate therapy: a randomized, placebo-controlled, 52-week trial. Arthritis Rheum 2004;50:1400–11.
- Cuppen BV, Welsing PM, Sprengers JJ, Bijlsma JW, Marijnissen AC, Van Laar JM, et al. Personalized biological treatment for rheumatoid arthritis: a systematic review with a focus on clinical applicability. Rheumatology (Oxford) 2016;55:826–39.
- Tracey D, Klareskog L, Sasso EH, Salfeld JG, Tak PP. Tumor necrosis factor antagonist mechanisms of action: a comprehensive review. Pharmacol Ther 2008;117:244–79.
- De Jager W, Hoppenreijs EP, Wulffraat NM, Wedderburn LR, Kuis W, Prakken BJ. Blood and synovial fluid cytokine signatures in patients with juvenile idiopathic arthritis: a cross-sectional study. Ann Rheum Dis 2007;66:589–98.
- De Jager W, Prakken BJ, Bijlsma JW, Kuis W, Rijkers GT. Improved multiplex immunoassay performance in human plasma and synovial fluid following removal of interfering heterophilic antibodies. J Immunol Methods 2005;300:124–35.
- Schipper HS, De Jager W, Van Dijk ME, Meerding J, Zelissen PM, Adan RA, et al. A multiplex immunoassay for human adipokine profiling. Clin Chem 2010;56:1320–8.
- Prevoo ML, Van ‘T Hof MA, Kuper HH, Van Leeuwen MA, Van De Putte LB, Van Riel PL. Modified disease activity scores that include twenty-eight-joint counts. Development and validation in a prospective longitudinal study of patients with rheumatoid arthritis. Arthritis Rheum 1995;38:44–8.
- Van Gestel AM, Haagsma CJ, Van Riel PL. Validation of rheumatoid arthritis improvement criteria that include simplified joint counts. Arthritis Rheum 1998;41:1845–50.
- Moons KG, Altman DG, Reitsma JB, Ioannidis JP, Macaskill P, Steyerberg EW, et al. Transparent Reporting of a multivariable prediction model for Individual Prognosis Or Diagnosis (TRIPOD): explanation and elaboration. Ann Intern Med 2015;162:W1–73.
- Hubbard AE, Ahern J, Fleischer NL, Van Der Laan M, Lippman SA, Jewell N, et al. To GEE or not to GEE: comparing population average and mixed models for estimating the associations between neighborhood risk factors and health. Epidemiology 2010;21:467–74.
- Fabre S, Dupuy AM, Dossat N, Guisset C, Cohen JD, Cristol JP, et al. Protein biochip array technology for cytokine profiling predicts etanercept responsiveness in rheumatoid arthritis. Clin Exp Immunol 2008;153:188–95.
- Hueber W, Tomooka BH, Batliwalla F, Li W, Monach PA, Tibshirani RJ, et al. Blood autoantibody and cytokine profiles predict response to anti-tumor necrosis factor therapy in rheumatoid arthritis. Arthritis Res Ther 2009;11:R76.
- Lequerre T, Jouen F, Brazier M, Clayssens S, Klemmer N, Menard JF, et al. Autoantibodies, metalloproteinases and bone markers in rheumatoid arthritis patients are unable to predict their responses to infliximab. Rheumatology (Oxford) 2007;46:446–53.
- Ortea I, Roschitzki B, Ovalles JG, Longo JL, De Lt I, Gonzalez I, et al. Discovery of serum proteomic biomarkers for prediction of response to infliximab (a monoclonal anti-TNF antibody) treatment in rheumatoid arthritis: an exploratory analysis. J Proteomics 2012;77:372–82.
- Trocme C, Marotte H, Baillet A, Pallot-Prades B, Garin J, Grange L, et al. Apolipoprotein A-I and platelet factor 4 are biomarkers for infliximab response in rheumatoid arthritis. Ann Rheum Dis 2009;68:1328–33.
- Uno K, Yoshizaki K, Iwahashi M, Yamana J, Yamana S, Tanigawa M, et al. Pretreatment prediction of individual rheumatoid arthritis patients’ response to anti-cytokine therapy using serum cytokine/chemokine/soluble receptor biomarkers. Plos One 2015;10:e0132055.
- Visvanathan S, Rahman MU, Keystone E, Genovese M, Klareskog L, Hsia E, et al. Association of serum markers with improvement in clinical response measures after treatment with golimumab in patients with active rheumatoid arthritis despite receiving methotrexate: results from the GO-FORWARD study. Arthritis Res Ther 2010;12:R211.
- Wright HL, Bucknall RC, Moots RJ, Edwards SW. Analysis of SF and plasma cytokines provides insights into the mechanisms of inflammatory arthritis and may predict response to therapy. Rheumatology (Oxford) 2012;51:451–9.
- Centola M, Cavet G, Shen Y, Ramanujan S, Knowlton N, Swan KA, et al. Development of a multi-biomarker disease activity test for rheumatoid arthritis. Plos One 2013;8:e60635.
- Bakker MF, Cavet G, Jacobs JW, Bijlsma JW, Haney DJ, Shen Y, et al. Performance of a multi-biomarker score measuring rheumatoid arthritis disease activity in the CAMERA tight control study. Ann Rheum Dis 2012;71:1692–7.
- Curtis JR, Van Der Helm-Van Mil AH, Knevel R, Huizinga TW, Haney DJ, Shen Y, et al. Validation of a novel multibiomarker test to assess rheumatoid arthritis disease activity. Arthritis Care Res (Hoboken) 2012;64:1794–803.
- Hirata S, Dirven L, Shen Y, Centola M, Cavet G, Lems WF, et al. A multi-biomarker score measures rheumatoid arthritis disease activity in the BeSt study. Rheumatology (Oxford) 2013;52:1202–7.
- Hambardzumyan K, Bolce RJ, Saevarsdottir S, Forslind K, Wallman JK, Cruickshank SE, et al. Association of a multibiomarker disease activity score at multiple time-points with radiographic progression in rheumatoid arthritis: results from the SWEFOT trial. RMD Open 2016;2:e000197.
- Fleischmann R, Connolly SE, Maldonado MA, Schiff M. Brief report: estimating disease activity using multi-biomarker disease activity scores in rheumatoid arthritis patients treated with abatacept or adalimumab. Arthritis Rheumatol 2016;68:2083–9.
- De Jager W, Bourcier K, Rijkers GT, Prakken BJ, Seyfert-Margolis V. Prerequisites for cytokine measurements in clinical trials with multiplex immunoassays. BMC Immunol 2009;10:52.
- De Jager W, Prakken B, Rijkers GT. Cytokine multiplex immunoassay: methodology and (clinical) applications. Methods Mol Biol 2009;514:119–33.
- Wong HL, Pfeiffer RM, Fears TR, Vermeulen R, Ji S, Rabkin CS. Reproducibility and correlations of multiplex cytokine levels in asymptomatic persons. Cancer Epidemiol Biomarkers Prev 2008;17:3450–6.
- Biancotto A, Feng X, Langweiler M, Young NS, McCoy JP. Effect of anticoagulants on multiplexed measurement of cytokine/chemokines in healthy subjects. Cytokine 2012;60:438–46.
- Brondum L, Sorensen BS, Eriksen JG, Mortensen LS, Lonbro S, Overgaard J, et al. An evaluation of multiplex bead-based analysis of cytokines and soluble proteins in archived lithium heparin plasma, EDTA plasma and serum samples. Scand J Clin Lab Invest 2016;76:601–11.
Supporting Information
Additional supporting information may be found in the online version of this article.
Supplementary text. Detailed section of statistical analysis.
Supplementary Table S1. Inflammatory proteins selected for analysis using xMAP technology.
Supplementary Table S2. Coefficients of selected proteins in the protein score, predicting DAS28 at 3 months.
Supplementary Table S3. Serum concentrations of selected proteins in the development and validation cohort as measured by xMAP technology.
Please note that the editors are not responsible for the content or functionality of any supplementary material supplied by the authors. Any queries should be directed to the corresponding author.