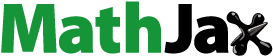
ABSTRACT
We examine household food security resilience to conflict and shock in Uganda and Malawi using data from the Household Living Standards Measurement Survey. We collect data on resilience components such as education, asset ownership, dwelling characteristics, water access, and sanitation facilities. Principal component analysis is used to generate factor weights for constructing the Resilience Capacity Index, which measures overall household resilience capacity and individual resilience components. The resilience capacity is then used in regression models to assess its mitigating effects on food security during conflicts and shocks. Our results indicate that resilience capacity significantly reduces the adverse effects of conflict and shock on food security. In Malawi, household head education contributes most to food security, while in Uganda, water access is the most critical component. In Malawi, water access resilience diminishes the impact of conflict, sanitation resilience reduces the impacts of flooding, and dwelling characteristics lessen the effects of drought, although the effect size is small. In Uganda, asset ownership resilience mitigates the impacts of drought, while sanitation resilience reduces the adverse effects of conflict. These findings highlight the importance of different resilience components in enhancing food security under various adverse conditions.
1. Introduction
The challenge of residing in environments marked by continuous exposure to traumatic and tense situations, such as violent conflicts, has garnered increased attention from global researchers and policymakers (Eagle and Kaminer Citation2013; Stevens et al. Citation2013; Pat-Horenczyk and Schiff Citation2019; Smith and Frankenberger Citation2017). Violent outbreaks have been linked to reduced innovation (Cook Citation2014), slower growth rates (De Groot et al. Citation2022; Young and Bologna Citation2016), and insecure property rights (North et al. Citation2013). Particularly in less developed countries, violence can severely hinder development by disrupting production and impeding beneficial exchanges for lower-income and vulnerable populations (George, Adelaja, and Weatherspoon Citation2020).
While the direct and spillover effects of violent conflicts on food security have been extensively studied and established (see Martin-Shields and Stojetz, Citation2019; Muriuki, Hudson, and Fuad Citation2023a and Muriuki et al. Citation2023b), an overlooked aspect is the examination of household resilience and strategies to mitigate the impact of violence and other shocks on food security. For instance, when households face losses in crops and livestock due to various shocks, they become vulnerable to food insecurity. Without mitigation strategies or safety nets in place, acute food insecurity is likely to ensue (Barrett Citation2010).
Resilience, as defined by the UK Department for International Development (DFID, Citation2011), is crucial for countries, communities, and households to manage change while maintaining or transforming living standards in the face of shocks or stresses. Recovering from conflicts requires resilience, involving prevention, anticipation, preparation, response, and recovery (FAO Citation2017). Building household resilience is pivotal for improving food security in areas experiencing recurrent violent conflicts (Kurtz and McMahon Citation2015).
Measuring resilience is inherently challenging, but various studies have attempted to do so in the past. The FAO, for example, has integrated resilience into its food security framework, emphasising strategies that strengthen diversity, local institutions, and household knowledge and adaptability (Pingali, Alinovi, and Sutton Citation2005). Options to monitor food security include access to basic public services, asset ownership, income-generating programmes, resistance capacity, and formal and informal safety nets (Browne, Ortmann, and Hendriks Citation2014b). Despite the importance of understanding the relationship between food security and resilience, little research exists on this topic, and existing evidence in the literature is sometimes counterintuitive. For example, while prior studies have suggested that food aid improves food security, recent research indicates that it may exacerbate cases of violent conflicts (Zürcher Citation2017; Ledford et al. Citation2022).
This study delves into alternative policy measures to address increased conflicts in sub-Saharan Africa, focusing on the household level. The analysis explores household resilience to conflict and shocks, examining their components and their role as effective adaptive strategies in Uganda and Malawi. The study provides valuable information on mitigation strategies for building regional stability in the two countries, taking a more granular approach.
This analysis contributes to the literature by utilising principal component analysis to construct a composite Resilience Capacity Index (RCI) that considers different dimensions of resilience capacity, including household assets, amenities, and members’ education (Browne, Ortmann, and Hendriks Citation2014). The study's implications include the feasibility of measuring resilience from publicly available data, providing valuable insights for policymakers and government agencies to design effective programmes for enhancing food security and facilitating household recovery from conflicts in Uganda and Malawi. Understanding the contribution of each resilience component to bolstering food security can inform targeted and swift policy interventions. The study's results are relevant to ongoing debates about mitigating strategies, advocating for a more granular approach rather than broad regional perspectives in building regional stability in Uganda and Malawi.
Previous literature highlights the importance of using assets to smooth consumption for risk management, as they impact the ability to prevent, mitigate, or cope with shocks. Greater access to productive assets and wealth is associated with a decreased likelihood of reduced food consumption in Uganda (FAO Citation2017). Ownership and use of tangible assets are emphasised as indicators of food security (Maxwell and Smith Citation1992; Løvendal and Knowles Citation2005), and a decline in asset status can signal vulnerability (Swift Citation2006). Positive relationships between food consumption, food security, and household resilience have been found in Kenya (Alinovi et al., Citation2010), indicating that resilience capacity plays a role in increasing food consumption. Research beyond Africa also suggests that violence affects household resilience capacity. Conflict-exposed households in Gaza have lower access to basic services, a higher social safety net, and lower adaptive capacity (Brück, d’Errico, and Pietrelli Citation2019). Additionally, households with high resilience levels in Nicaragua experience better food security in the future (Ciani and Romano Citation2014).
Despite these insights, much of the existing literature does not thoroughly examine how resilience directly mitigates the effects of conflict and shock on food security. Moreover, studies that do so often rely on data collected shortly after conflicts end, possibly missing the overall impacts. While previous literature provides valuable insights into household resilience and strategies for mitigating violence and shocks, it also identifies limitations. This study addresses these gaps by examining alternative policies for mitigating conflict in households and determining the impact of each component on food security in Uganda and Malawi. The analysis makes a significant contribution to existing research, considering the commonality of food insecurity across regions and the complex repercussions of violence.
2. Model
Montgomery et al. (Citation2000) argue that there is no standard methodology for constructing asset–, living conditions – and education-based indices, and their construction differs mostly in the types of assets and other variables included in the index, as well as the weights assigned to the individual variables or indicators. This study utilises a well-established index construction method proposed by Browne, Ortmann, and Hendriks (Citation2014). The method involves employing factor weights from principal component analysis to construct Resilience Capacity Indices (RCI) for different dimensions of resilience capacity (including household asset ownership, dwelling characteristics, and education attainment of household head). These individual resilience capacity indices are then combined to construct a composite index.
2.1 Principal component analysis
Principal Component Analysis (PCA) is a statistical technique for analysing datasets with a large number of features by reducing the dimensionality of the dataset. The primary utility of using PCA is to reduce the dimensionality of the data without compromising the information in the variation the variables explain (Vyas and Kumaranayake Citation2006). Previous studies have used PCA on large sets of indicators and variables as proxies to measure socioeconomic status, such as durable asset ownership, access to utilities/infrastructure like water and sanitation facilities, and housing characteristics like floor, wall, and roof materials, education level of head of household, etc. As an example, PCA based on linear variables are classified by socioeconomic status in papers by Kolenikov and Angeles (Citation2009) and Linting et al. (Citation2007).
PCA works by creating uncorrelated indices or components from a set of correlated variables, where each component is a linear combination of the initial variables. If
through
are a set of variables, then the following results will be obtained:
where
represents the weight for the
principal component and the
variable.
In the case of standardised data, the covariance matrix, or the eigenvectors of the correlation matrix, determine the weights for each principal component. Each principal component's variance (λ) is given by its eigenvalue under the constraint that the sum of the squared weights () must equal one. Accordingly,
represents the proportion of the total variation in the original data set accounted for by each principal component, where the sum of the eigenvalues equals the number of variables in the original data set.
It is important to note that the second component () is completely uncorrelated with the first component (
) and explains additional but less variation than the first component, subject to the same constraints as the first component. Each component adds an additional dimension to the data, while explaining a smaller and smaller proportion of the variation of the original variables. As the degree of correlation among the original variables in the data increases, fewer components are required to capture the same information.
2.2 Variable selection for Resilience Capacity Index construction
A variety of variables have been used to measure socioeconomic status of households, including ownership of land, farm animals, type of housing, literacy level of household head, and demographics (see Filmer and Pritchett Citation2001). There are no best practices for selecting variables to proxy living standards, as variables are selected on an “ad hoc” basis in many studies depending on availability (Montgomery et al. Citation2000). Ideally, PCA works best when asset variables are correlated, but also when variable distribution varies from household to household. According to McKenzie (Citation2003), PCA gives more weight to assets that are more unequally distributed among households. An asset owned by all households or one owned by no households (i.e., zero standard deviation) would not show any variation between households, therefore being zero weighted, and being of little use when analysing the household social economic status. Variables with low standard deviations will be low weighted in the PCA. Therefore, asset indices, PCA is widely used to generate the weights for the variables. This is because we typically have a lot of variables that convey the same information in socioeconomic studies.
In order to investigate all variables, we first conduct and report descriptive analyses, examining means and standard deviation to inform variable selection and highlight data issues. McKenzie (Citation2003) emphasised the importance of ensuring the range of asset variables used in PCA-based asset indices is broad enough to avoid problems of clumping and truncation. Clustering is the process of grouping households into a small number of distinct groups. On the other hand, by truncating social economic status, a more even distribution is created, but over a narrow range, which makes identifying socioeconomic groups difficult.
2.3 Application of Principal Component Analysis
Our original data are in categorical format; for example, questions related to drinking water access are grouped into answer categories such as “spring”, “covered spring”, “rainwater”, “well”, etc. However, data in categorical form are not suitable for PCA because the categories have no hierarchical relationship between them, and so PCA converts them to a quantitative scale which does not have any meaning (Vyas and Kumaranayake Citation2006). To circumvent this, we convert all the qualitative categorical variables to binary variables. For example, the single categorical variable for drinking water access is now converted to four binary variables: (i) spring; (ii) covered spring; (iii) rainwater; (iv) well; each taking a value of 1 or 0 depending on how the household derives its drinking water. Converting to binary variables does not change the relationship between variables, does not add any new information or correlation in the dataset (Vyas and Kumaranayake Citation2006) and allows PCA to determine which variable can distinguish between the households.
Factor weights (eigenvectors) from PCA can be derived from either correlation matrix or covariance matrix. We do not use standardised data since the variables are not expressed in the same units, so the analysis is run using the correlation matrix. To obtain the weights, we first need to define the number of principal components. Prior studies have shown that the first principal component is a measure of economic status (Houweling, Kunst, and Mackenbach Citation2003). Some studies also considered using additional principal components to characterise socioeconomic index but concluded that only the first principal component was necessary (McKenzie Citation2003). Therefore, in this study, we only use the first principal component as weights.
Using the first principal component factor weights, the index of resilience is then calculated based on Equation (1) below (Browne, Ortmann, and Hendriks Citation2014b):
(1)
(1) where
is the Resilience Capacity Index (RCI) for household
,
is the weight generated by PCA for the first variable,
is the
household value for the first variable (1 if the household owns that asset or has access to that facility) and
and
are means and standard deviations respectively of the first variable over all the households. In order to make resilience capacity indices easier to interpret, we standardise all indices between 0 and 100. The issue of adjusting for household size was raised by McKenzie (Citation2003). As in the study by Filmer and Pritchett (Citation2001), McKenzie (Citation2003) does not adjust for household size, arguing the benefits of indicators used are available at household level. Following the two precedents, we also do not adjust for household size.
Using the formula above, we measure five different component resilience capacity indices: (i) education index, comprising the variables indicating the highest level of education attainment by the household head; (ii) durable asset index, comprising variables indicating durable asset ownership; (iii) water access index, comprising variables indicating drinking water access; (iv) dwelling characteristics index, comprising variables indicating dwelling construction materials; and (v) sanitation facilities index, comprising variables indicating sanitation facilities. In addition to these variables, we also construct a composite resilience index, which comprises all variables used to construct the five categorical indices. A full list of the variables used to construct the component resilience capacity indices and the composite index is presented in Appendix for Malawi and for Uganda.
We address two key research questions in this paper using the resilience indices: (i) what is the relationship between household’s food security and their resilience capacity in response to conflict or shocks; and (ii) does resilience capacity, measured by resilience indices, mitigate the negative effect of violent conflicts and shocks on food security. Another related question we also address through the second question is which types of individual components of the composite resilience capacity contribute the most in bolstering households’ food security in the face of conflict and shock. To address the first question, we conduct regression analyses including conflict exposure and resilience capacity as independent variables while controlling other households’ sociodemographic characteristics. To understand this relationship, the OLS is presented in Equation (2) below:
(2)
(2) where
is the household food security measured by the food consumption score,
indicates whether the household was exposed to a shock (violent conflict, drought or flood),
is one of the five index or the composite index of resilience capacity measured using factor weights from the PCA, and
is a set of covariates including household characteristics such as age and gender of household head, income of household, distance of household from nearest population centre, etc.
To address the question of whether (and which types of) resilience capacity mitigate the negative impacts of violent conflicts on food security, we include in the regression the interaction term between the resilience indices and a binary variable denoting incidences of conflicts or shocks (Equation (3)). The key variable of interest is this interaction term (); a positive and statistically significant coefficient on the interaction term is interpreted as the resilience index mitigating the negative effect of the conflict or shock, which otherwise is expected to have a negative effect on food security.
(3)
(3)
3. Data
We construct resilience indices for Uganda (2011) and Malawi (2010, 2013, 2016, 2019) from household survey data from the Household Living Standards Measurement Survey – Integrated Surveys on Agriculture (LSMS-ISA). For Uganda, we construct resilience index using education level of household head, assets ownership, and dwelling characteristics (type of roof, floor, wall, etc.). The resilience index for Malawi is constructed using the education level of the household head, dwelling characteristics, sanitation characteristics, and water access facility. Since resilience scores are constructed using different variables, they are not comparable and thus the regressions are run separately for each country.
The dependent variable in the study is food security measured by food consumption score (FCS). The FCS measures dietary diversity taking into account both diversity and frequency of food intake. The FCS is constructed using a list of household-level food items and food groups from the World Food Program's method (WFP Citation2008). The household is asked about the frequency of consumption over the past week for each food group. The method then aggregates the food items into eight basic food groups and sums up the consumption frequencies in each of the food groups. Any food group score greater than seven is truncated. These truncated food group scores are multiplied with their corresponding weights and the weighted food group scores are added to obtain the final FCS as a measure of food security.
The LSMS also has information on whether the household was exposed to any kind of shock, such as conflict, drought or flood. These variables are a typical binary variable with “1” indicating the household experienced conflict, drought or flood in the past 12 months and “0” otherwise. We use these variables to define shocks experienced by the household and examine their resilience in the face of such shocks. A full list, description, and summary statistics for all variables in each country are provided in Table 1.
4. Results
4.1 Interpretation of Principal Component Analysis results
The PCA output is a table of factor weights (alternatively, factor scores or simply weights) for each variable. Generally, higher scores indicate higher resilience capacity and negative scores lower resilience capacity. Table 1 shows the factor weights for Uganda; the first row shows a negative, albeit small, factor weight (-0.072) associated with radio ownership, implying, all things being equal, lower resilience capacity ranking than non-owners. This may seem counterintuitive at first, but this may be because radio ownership correlates more with lower quality housing/sanitation. Findings like these may occur when indices are constructed for combined urban and rural locations, where assets represent wealth in some parts of the country but not others (Vyas and Kumaranayake Citation2006). However, relative to other durable assets, weights for radio ownership are small in absolute terms.
We construct separate indices for both Malawi and Uganda. Descriptive analysis and factor weights are presented in Appendix for Malawi and for Uganda.
For Uganda, the factor weights are positive for all durable assets (e.g., refrigerator, mobile, computer, bike), as is the usage of higher quality usage of water (e.g., pipe water to dwelling or yard) and sanitation facility (e.g., ventilated improved pit, latrine w/ slab, toilets connected to septic), housing characteristic (e.g., bricked walls, tiled floor), and education of household head (university diploma). Similarly, low quality water supply, sanitation facility, walls and flooring have negative weights.
For Malawi, we observe similar results. The factor scores are positive for electricity access (0.306). The scores are also higher for higher quality water source (e.g., piped water to dwelling or yard, as opposed to open well or bore), sanitation facility (e.g., toiler to sewer or septic tank, slab latrine in contrast to pit latrine), housing characteristics (e.g., bricked wall, iron roof, concrete floor), and education of household head (any degree beyond primary schooling).
Interpreting the weights from our example, a household in Uganda with more durable assets, piped drinking water to residence, sanitation facility that leads to a sewer or septic, finished floor coverings and higher education attainment would attain a higher SES score, and subsequently a higher resilience score. The finding is similar for Malawi, except it includes electricity access, along with drinking water piped to dwelling or yard, housing characteristics such as floor coverings, constructed roof and walls, and access to infrastructure facilities such as sanitation, would lead to a higher SES and resilience capacity score.
We then use the factor scores from the first principal component as weights in constructing resilience capacity indices for each household using Equation (1). We first construct five component indices based on five dimensions of resilience: (i) asset ownership (using all variables under “Asset ownership” in and ); (ii) water supply (using all variables under “Source of water supply” in and ); (iii) dwelling characteristics (using all variables under “Type of roof material”, “Type of wall material”, and “Type of floor material” in and ); (iv) sanitation facility (using all variables under “Type of sanitation facility”) in and ; and (v) education attainment by household head (using all variables under “Education of household head” in and ). Finally, a composite index is constructed using all variables in and .
Table 1. Variable definitions.
Table 2. Composite resilience capacity regression results – Malawi and Uganda.
In Equation (1), for household ,
is the weight generated by PCA for the first variable,
is the
household value for the first variable (1 if the household owns that asset or has access to that facility) and
and
are means and standard deviations respectively of the first variable over all the households.
As a simple illustration, to construct the asset ownership index for Uganda, would take a value of – 0.072;
takes a value of 1 if household i owns a radio and 0 otherwise;
takes a value of 0.679; and
takes a value of 0.469;
takes a value of 0.216;
takes a value of 1 if household i owns a refrigerator and 0 otherwise;
takes a value of 0.451; and
takes a value of 0.358; and so on until n = 7 (radio, refrigerator, mobile, computer, bike, other vehicle, electricity). Similarly, for the composite index for Uganda, n = 49 (Radio, Refrigerator, … , Secondary School, University Diploma).
4.2 Relationship between food security and resilience capacity
presents the variable definitions and descriptive statistics for the major shocks and normalised resilience capacity indices from individual countries. The normalised resilience capacity index measures how resilient households are, with zero being the least resilient and 100 being the most resilient; the index includes the resilience index pillars used in this study: asset index, education level of the household head index, asset ownership index, dwelling characteristics index, sanitation characteristics index, and water access index.
Food security values from households from Uganda are on average relatively higher (49.87) compared to households from Malawi (49.86), indicating they are generally more food secure. Households in Malawi are also exposed to substantially more incidences of drought and flood relative to Uganda.
4.2.1 Composite resilience capacity
We first present the regression results from Equation (2) in Malawi and Uganda in . The key variable of interest is the composite resilience capacity, which as a result of combining all dimensions of resilience, measures the overall resilience. The results indicate that conflict exposure indeed has a negative and significant influence on household food security in Uganda, although the relationship is not significant in Malawi. It is however important to note that results for each country are not comparable since the composite indices are composed of different variables depending on the availability of data. In terms of magnitude, every incidence of conflict is associated with a decline in food security by -7.348 units in Uganda, which is equivalent to a 14.73% reduction in the average food security score. In the case of resilience capacity measured by the composite index, the regression coefficients have the expected signs and are statistically significant. In terms of magnitude, an additional unit of resilience is associated with an increase in food consumption score by 1.302 in Malawi (equivalent to 2.611% of average food consumption score) and 2.349 in Uganda (equivalent to 4.710%). The results indicate that resilience capacity serves to increase the number of days of adequate food and reduce hunger.
Several other variables controlled for also have the expected direction of effect as determinants of food security. Those that are statistically significant are: age and gender of household head and distance to nearest population centre in Malawi, and household income in both Malawi and Uganda.
4.2.2 Component-wise resilience capacity
The composite resilience capacity, however, may not provide clear policy implications, especially when it comes to mitigating strategies after violent conflicts because it captures overall resilience. To address something as abstract as resilience, more precise insights are needed regarding the specific dimensions of resilience and their relationship with food security. We therefore present the regression estimates from Equation (2) with the individual component pillars of resilience capacity in . Our dependent variable again is food consumption score, and the variables of interest are the individual component indices. Only the results for the main variables of interest are reported. The results reveal that resilience capacity for education of the household head has the biggest positive-magnitude relation with food security in Malawi, followed by water access while dwelling characteristics have the largest negative relation. The findings from Uganda yield mixed results. Surprisingly, there is a negative but statistically insignificant relationship between food security and factors such as water access, dwelling characteristics, and the resilience capacity for sanitation facilities. The negative results in general may be because the indices are constructed for combined urban and rural locations, where assets represents wealth in some parts of the country but not others. For example, ownership of radios in urban areas may not be as much of an advantage as it may be in rural areas. Similarly, a particular education level represents an advantage in some parts of the country but not others. For example, a high school level education may be a disadvantage in certain urban locations because it was not be high enough to allow an individual to pursue a formal profession, but at the same time, the individual may be deemed overqualified for a lot of jobs available.
Table 3. Component-wise resilience capacity regression results – Malawi and Uganda.
4.3 Does resilience capacity reduce the negative impact of shocks on food security?
The previous analysis indicates that exposure to shocks or stresses undermines a household's food security, whereas having greater resilience-building capacity enhances food security. Resilience refers to a household's ability to withstand and recover from adverse events and shocks while still maintaining their well-being. In other words, resilience allows households to deal with hardship in a way that protects their food situation rather than compromising it, even when faced with difficult circumstances. The analysis suggests resilience is important for households to maintain food security in the context of shock exposure.
The next critical question we ask is whether and which type of resilience capacity reduces the negative impacts of shocks on food security. To answer this, we use Equation (3) and include in the regressions the interaction terms between resilience capacity and violent conflicts and non-violent shocks. If the sign on the interaction term is positive (negative), we can argue that the index component has a mitigating (facilitating) effect on violent conflict and vice versa. These results are reported in .
Table 4. Does resilience capacity reduce the negative impact of conflicts and shocks on food security.
We first look at the results for Malawi. When the interaction term between violent conflict and resilience capacity for water access is included, the coefficient is positive (0.035) and statistically significant. The implied empirical relationship between food security measured by food consumption score (FCS), resilience capacity index (RCI) and conflict (Conflict) is:
where D represents the contribution to the equation of the constant term and the remaining independent variables controlled for.
The estimated impact of conflict exposure on food security is thus:
As a result, water access as a mitigating mechanism reduces the impact of conflicts on food security from -1.206 to (-1.206+0.035) -1.171; this is equivalent to a (0.035/1.206 = 0.029) 2.9% reduction in the effect of conflict on average food consumption score. Contrary to our expectations, education, water access and sanitation facilities have a negative relation with household’s capacity to recover from droughts, indicating that these resilience capacities exacerbate the negative effect of droughts in Malawi. That the resilience capacity-mediated relationship between drought and food security is negative may allude to the strong effects of drought on food security and the inability of the common measures of resilience to mitigate their impacts, which is especially relevant because the sample is from countries in sub-Saharan Africa. However, the results also point to the effective role of dwelling characteristics to lessen the impacts of drought on food security. Regarding floods, the resilience capacities of water access and sanitation facilities play a role in mitigating the harmful impacts. This can be related to the fact that during floods, access to safe drinking water and proper sanitation can prevent serious health risks and the spread of disease.
For Uganda, some of the estimates of the interaction term lacks statistical power, which may be driven by the relatively small sample size. Regardless, we observe a positive sign on the asset-based resilience capacity mitigating the effects of conflict while sanitation-based resilience capacity mitigates the effects of drought. Intuitively, having more durable assets can positively affect a household's ability to recover from conflict in several ways. For example, the sale or use of assets as collateral provides emergency funds. Assets also retain value better than currency during instability. Durable goods generally hold their value better than local currency during conflict-induced inflation or currency depreciation, letting households store wealth. Having better access to sanitation facilities can also positively affect a household's ability to recover from drought. For example, improved sanitation reduces the spread of waterborne diseases during drought when water access/quality declines. This keeps households healthier to cope with hardship. Better sanitation can also result in fewer medical costs. Less spending on treatment of sanitation-related illnesses like diarrhoea frees up limited funds for food, tools to restart livelihoods after drought ends.
To summarise: the results in Malawi, especially those for water access in face of conflict, dwelling characteristics in face of drought, and sanitation facilities in face of flood suggest that indeed households’ resilience capacity works to enable their resilience, as measured by their ability to maintain their food security. The results in Uganda are a little less convincing; nevertheless, durable asset ownership in face of conflict and sanitation facilities in face of drought also bolster their resilience and allow them to maintain their food security.
5. Discussion of results
Comprehending the household's resilience to conflict and other shocks is crucial when devising effective and targeted adaptive strategies. Resilience has now become a central element in the planning and implementation of interventions across various domains, such as climate change adaptation, humanitarian activities, disaster risk reduction, social protection, food security, and nutrition. This integration is evident in the strategies adopted by international organisations like UNDP, FAO, and donors, including USAID (Parsons et al. Citation2016; Béné Citation2020). Additional insights from d’Errico, Romano, and Pietrelli (Citation2018) emphasise that resilience to food insecurity denotes a household's capacity to uphold a certain level of well-being, such as being food secure, despite encountering shocks and stresses.
To assess overall resilience, we first consolidate all variables to construct a composite resilience index. The regression findings reveal a negative and significant impact of conflict exposure on household food security in Uganda, although this relationship lacks statistical significance in Malawi. Conversely, the positive and significant coefficient associated with the resilience capacity index indicates that resilience preserves food security in households. Notably, these results align with prior research findings (see Sharifi Citation2016; d’Errico, Romano, and Pietrelli Citation2018; Smith and Frankenberger Citation2018; Béné Citation2020; Murendo, Kairezi, and Mazvimavi Citation2020). For instance, d’Errico, Romano, and Pietrelli (Citation2018) show that the relationship between resilience and food security is positive in Tanzania. The collective evidence underscores the critical role of resilience in mitigating the adverse effects of conflict on food security in diverse contexts.
In Malawi, an increase in rainfall has demonstrated a positive correlation with food security. Nevertheless, prior investigations have presented diverse findings regarding the connection between climate variables and food security. Notably, inconsistent rainfall remains a significant vulnerability factor for economies heavily dependent on agriculture (Demeke, Keil, and Zeller Citation2011). Given that 84 percent of rural households in Malawi rely on agriculture for their livelihoods, weather variability poses a substantial threat to food security (NSO Citation2018). Conversely, rising temperatures in Malawi are linked to a decrease in food security. In contrast, and contrary to our expectations, elevated rainfall levels in Uganda are associated with diminished food security. These results align with earlier studies that have demonstrated mixed outcomes. For instance, Lesk, Rowhani, and Ramankutty (Citation2016) revealed that sustained drought and extreme heat can lead to a reduction in crop yields by up to 10 percent. While Hsiang, Burke, and Miguel (Citation2013) suggested that warmer temperatures and lower rainfall contribute to violent conflicts, Muriuki et al. (2023) examined the broader implications of conflict on food security. Salehyan and Hendrix (Citation2014) further illustrated that water abundance may pose a greater risk of conflict than water scarcity. To address these challenges, policy recommendations should concentrate on adapting agricultural systems through transformative practices, enterprise diversification, and crop species diversification (Di Falco, Veronesi, and Yesuf Citation2011; Kankwamba, Kadzamira, and Pauw Citation2018). Additionally, alternative mitigation strategies should encompass risk transfer mechanisms, such as rainfall-indexed agricultural insurance (HLPE Citation2012; Barrett and Constas Citation2014).
The age of the household head is negatively correlated with food security, aligning with d’Errico, Romano, and Pietrelli (Citation2018) and Beyene et al. (Citation2023) findings. This may be due to older household heads having limited access to diverse food sources. Additionally, male-headed households exhibit greater resilience than their female-headed counterparts, corroborating previous vulnerability studies (Quisumbing Citation2003; Kumar and Quisumbing Citation2013). Distance from the nearest market is also a significant factor, with increasing distance associated with decreased food security.
While the composite resilience capacity may not offer clear policy implications for post-conflict mitigation strategies, disaggregating the index into specific pillars reveals more nuanced insights. Education of the household head exhibits the strongest positive correlation with food security in Malawi, supporting FAO's (Citation2017) assertion that less educated households are more vulnerable to shocks. Our findings align with Alinovi et al. (Citation2010), who also found a positive and significant relationship between education level and food security. However, Beyene et al. (Citation2023) did not observe a significant difference in educational levels across resilience levels to food insecurity.
Dwelling characteristics, particularly improved roof, walls, and floors, have the most substantial negative impact among the resilience indices. Water access and sanitation indices also contribute to food security in Malawi, suggesting that having a stable water source and a decent latrine can enhance resilience even in the face of adversity. These findings align with Dercon et al.'s (Citation2008) conclusion that access to water and sanitation services is fundamental to household resilience capacity.
The results from Uganda present a combination of outcomes. Unexpectedly, there exists a negative but statistically insignificant relation between food security and elements such as water access, dwelling conditions, and sanitation facilities resilience capacity. Notably, there is a negative and statistically significant coefficient linked to the durable asset resilience capacity. An explanatory insight can be drawn from an FAO (Citation2017) study, which suggests that households may curtail their consumption of dietary foods to safeguard their assets through asset smoothing. Consequently, households with greater asset ownership may merely be indicative of a strategy to smooth food consumption for asset preservation.
To determine whether resilience capacity can buffer against the adverse consequences of shocks on food security, we introduce interaction terms between the resilience capacity and both violent conflicts and non-violent shocks into our regression models. A positive (negative) sign on the interaction term indicates that the index component mitigates (exacerbates) the impact of violent conflicts and vice versa. Our initial analysis focuses on Malawi. When we include the interaction term between violent conflict and resilience capacity for water access, the positive coefficient (0.035) is statistically significant. This implies that water access as a mitigating factor reduced the impact of conflicts on food security from -1.206 to -1.171 for every unit increase in the resilience index. This translates to a 2.9 percent reduction in the negative impact of conflict on food security. These results are supported by previous studies. That has shown that access to water has been a trigger of conflict (Gleick and Iceland Citation2018). For example, in 2017, a German government report warned that up to 6.6 million migrants were waiting to cross into Europe from African and Middle East prolonged migration crises influenced partly due to water (Iceland Citation2017). Therefore, the issue of water access and security is receiving more attention because of the growing evidence that natural resource degradation is a root cause of conflict, migration, and acute food insecurity (Vishwanath Citation2015; Von Lossow Citation2016; Balakrishnan Citation2017; Regan and Kim Citation2020).
The interaction between household dwelling characteristics and drought shows a positive sign (0.07), indicating that housing amenities marginally protect households from the negative impacts of drought on food security. However, the effect size is small, reducing the impact from -6.77 to -6.70, equivalent to approximately 1.03% reduction in the negative impact because of resilience capacity. Furthermore, results show that when the resilience capacity for sanitation is interacted with flooding, it mitigates the impacts of flooding by 0.82%, reducing the impact from -7.84 to -7.78 units for every unit increase in sanitation resilience capacity.
Though the coefficient for drought is not statistically significant, the interaction with sanitation resilience capacity is positive (0.06) and statistically significant, suggesting that the household's sanitation characteristics reduces the negative effects of droughts on food security by 7.65 percent in Uganda. Based on the results of asset ownership, resilience capacity for assets reduces the negative effects of violent conflicts from 123 to 121.83 equivalent to 0.95 percent and enhances the effects of droughts on food security in Uganda from 122.5 to 123.73 equivalent to 1 percent increase. These findings could be explained by the non-productivity of the assets since their capital returns decrease rapidly over time (Hallegatte Citation2014), thereby not being able to smooth assets during shocks.
6. Conclusions and recommendations
It is essential to understand the components that households possess to protect, provide and promote resilience (Hendriks Citation2011). Knowing which component of a household contributes to building resilience and which negatively affect resilience are critical for policymakers in identifying the mediating or protective effect of resilience capacities in the presence of different shock exposures. To fill that literature gap, this study develops a measure of resilience, composed of individual components, for measuring household resilience levels on food security in Uganda and Malawi. Using the resilience measures of different household components, we test how each is related to food security.
To do this, we utilise the World Bank Living Standards Measurement Study data for two sub-Saharan African countries – Malawi and Uganda. The findings show that resilience capacity for education of the household head contributes the most in bolstering households’ food security in the face of the violent conflicts in Malawi while water access contributes the most in Uganda. Resilience capacity for water access also reduces the negative impacts of violent conflicts on food security in Malawi. Similarly, dwelling characteristics and sanitation facilities diminished the drought and flood impacts on food security respectively; durable assets significantly reduced the negative effects of violent conflicts on food security in Uganda.
This study has interesting implications for the two countries. First, the resilience capacity utilised in this study shows that it could be measured and can detect the negative impacts following a conflict outbreak or other non-violent disaster in Malawi and Uganda. These findings can therefore be a valuable tool to provide policy insights as to which strategies should be adopted to not only enhance food security but also reverse the impact of shocks and facilitate the recovery of households from conflicts in the two countries.
Second, understanding the contribution of each pillar and subsequently knowing the category with the most impact on food security in these sub-Saharan African countries, will help inform policymakers especially during interventions, often when these interventions have to be very targeted and made swiftly. For example, some studies have claimed food aid is the most effective coping tool, but some recent studies have shown that food aid does not necessarily enhance food security, rather, it sometimes exacerbates cases of violent conflicts (Ledford et al. Citation2022). Therefore, having a clear understanding of factors that contribute to building resilience is a prerequisite for developing a preventive response plan.
Disclosure statement
No potential conflict of interest was reported by the author(s).
References
- Alinovi, L., Mane, E. and Romano, D., 2010. Measuring household resilience to food insecurity: Application to Palestinian households. Agricultural survey methods, pp.341–68.
- Alinovi, L., D’errico, M., Mane, E. and Romano, D., 2010. Livelihoods strategies and household resilience to food insecurity: An empirical analysis to Kenya. European report on development, 1(1), pp.1–52.
- Balakrishnan, V.S. 2017. Cholera in Yemen. The Lancet Infectious Diseases 17, no. 7: 700–1.
- Barrett, C.B. 2010. Measuring food insecurity. Science 327, no. 5967: 825–8.
- Barrett, C.B., and M.A. Constas. 2014. Toward a theory of resilience for international development applications. Proceedings of the National Academy of Sciences 111, no. 40: 14625–30.
- Béné, C. 2020. Are we messing with people's resilience? Analysing the impact of external interventions on community intrinsic resilience. International Journal of Disaster Risk Reduction 44: 101431.
- Beyene, F., M. Senapathy, E. Bojago, and T. Tadiwos. 2023. Rural household resilience to food insecurity and its determinants: Damot Pulasa district, Southern Ethiopia. Journal of Agriculture and Food Research 11: 100500.
- Browne, M., G.F. Ortmann, and S.L. Hendriks. 2014. Household food security monitoring and evaluation using a resilience indicator: An application of categorical principal component analysis and simple sum of assets in five African countries. Agrekon 53, no. 2: 25–46.
- Brück, T., M. d’Errico, and R. Pietrelli. 2019. The effects of violent conflict on household resilience and food security: Evidence from the 2014 Gaza conflict. World Development 119: 203–23.
- Ciani, F., and D. Romano. 2014. Testing for household resilience to food insecurity: Evidence from Nicaragua (No. 1053-2016-85948).
- Cook, L.D. 2014. Violence and economic activity: Evidence from African American patents, 1870–1940. Journal of Economic Growth 19: 221–57.
- d’Errico, M., D. Romano, and R. Pietrelli. 2018. Household resilience to food insecurity: Evidence from Tanzania and Uganda. Food Security 10, no. 4: 1033–54.
- De Groot, O.J., C. Bozzoli, A. Alamir, and T. Brück. 2022. The global economic burden of violent conflict. Journal of Peace Research 59, no. 2: 259–76.
- Demeke, A.B., A. Keil, and M. Zeller. 2011. Using panel data to estimate the effect of rainfall shocks on smallholders food security and vulnerability in rural Ethiopia. Climatic Change 108, no. 1-2: 185–206.
- Dercon, S., Bold, T. and Calvo, C., 2008. Insurance for the Poor?. In Social protection for the poor and poorest: Concepts, policies and politics (pp. 47–63). London: Palgrave Macmillan UK.
- DFID. 2011. Defining disaster resilience: A DFID approach paper. Department for International Development. Available at: https://www.gov.uk/government/publications/defining-disaster-resilience-a-dfid-approach-paper (Accessed: 14 June 2024)
- Di Falco, S., M. Veronesi, and M. Yesuf. 2011. Does adaptation to climate change provide food security? A micro-perspective from Ethiopia. American Journal of Agricultural Economics 93, no. 3: 829–46.
- Eagle, G. and Kaminer, D., 2013. Continuous traumatic stress: Expanding the lexicon of traumatic stress. Peace and Conflict: Journal of Peace Psychology 19, no. 2: 85–99.
- FAO, I. F. A. D., & UNICEF, W. 2017. The state of food security and nutrition in the world 2017. Building resilience for peace and food security. Rome: FAO.
- Filmer, D., and L.H. Pritchett. 2001. Estimating wealth effects without expenditure data—or tears: An application to educational enrollments in states of India. Demography 38, no. 1: 115–32.
- George, J., A. Adelaja, and D. Weatherspoon. 2020. Armed conflicts and food insecurity: Evidence from Boko Haram's attacks. American Journal of Agricultural Economics 102, no. 1: 114–31.
- Gleick, P., and C. Iceland. 2018. Water, security and conflict. Washington, DC: World Resources Institute.
- Hallegatte, S., 2014. Economic resilience: definition and measurement. World Bank Policy Research Working Paper (6852), 1–44.
- Hendriks, S.L. 2011. Overview: Trade for nutrition. Plenary technical paper presented at the first commemoration of the Africa food security and nutrition Day, MidRand, 27-28.
- HLPE, C. 2012. Social protection for food security. A Report by the High Level Panel of Experts on Food Security and Nutrition of the Committee on World Food Security.
- Houweling, T.A., A.E. Kunst, and J.P. Mackenbach. 2003. Measuring health inequality among children in developing countries: Does the choice of the indicator of economic status matter? International Journal for Equity in Health 2, no. 1: 1–12.
- Hsiang, S.M., M. Burke, and E. Miguel. 2013. Quantifying the influence of climate on human conflict. Science 341, no. 6151: 1235367.
- Iceland, C. 2017. Water stress is helping drive conflict and migration. Washington, DC: World Resources Institute.
- Kankwamba, H., M. Kadzamira, and K. Pauw. 2018. How diversified is cropping in Malawi? Patterns, determinants and policy implications. Food Security 10, no. 2: 323–38.
- Kolenikov, S., and G. Angeles. 2009. Socioeconomic status measurement with discrete proxy variables: Is principal component analysis a reliable answer? Review of Income and Wealth 55, no. 1: 128–65.
- Kumar, N., and A.R. Quisumbing. 2013. Gendered impacts of the 2007–2008 food price crisis: Evidence using panel data from rural Ethiopia. Food Policy 38: 11–22.
- Kurtz, J., and K. McMahon. 2015. Pathways from peace to resilience: Evidence from the greater horn of Africa on the links between conflict management and resilience to food security shocks. Washington, DC: Mercy Corps.
- Ledford, L., D. Hudson, C. Carpio, J. Pavlik, and R. Post. 2022. Food security and conflict in Sub-saharan Africa working paper, Tech Tech University.
- Lesk, C., P. Rowhani, and N. Ramankutty. 2016. Influence of extreme weather disasters on global crop production. Nature 529, no. 7584: 84–87.
- Linting, M., J.J. Meulman, P.J. Groenen, and A.J. van der Koojj. 2007. Nonlinear principal components analysis: Introduction and application. Psychological Methods 12, no. 3: 336.
- Løvendal, C.R. and Knowles, M., 2005. Tomorrow’s hunger: A framework for analyzing vulnerability to food insecurity. Agriculture and Development Economics Division ESA Working Paper, No. 05-07. Rome: Food and Agriculture Organization of the United Nations.
- Martin-Shields, C.P. and Stojetz, W., 2019. Food security and conflict: Empirical challenges and future opportunities for research and policy making on food security and conflict. World development, 119, pp.150–64.
- Maxwell, S., and M. Smith. 1992. Household food security: A conceptual review. Household Food Security: Concepts, Indicators, Measurements 1: 1–72.
- McKenzie, D.J. 2003. Measure inequality with asset indicators (No. 042). BREAD Working Paper.
- Montgomery, M.R., M. Gragnolati, K.A. Burke, and E. Paredes. 2000. Measuring living standards with proxy variables. Demography 37, no. 2: 155–74.
- Murendo, C., G. Kairezi, and K. Mazvimavi. 2020. Resilience capacities and household nutrition in the presence of shocks. Evidence from Malawi. World Development Perspectives 20: 100241.
- Muriuki, J., D. Hudson, and S. Fuad. 2023a. The impact of conflict on food security: Evidence from household data in Ethiopia and Malawi. Agriculture & Food Security 12, no. 1: 41.
- Muriuki, J., D. Hudson, S. Fuad, R.J. March, and D.J. Lacombe. 2023b. Spillover effect of violent conflicts on food insecurity in sub-Saharan Africa. Food Policy 115: 102417.
- North, D.C., Wallis, J.J., Webb, S.B., and Weingast, B.R. eds., 2013. In the shadow of violence: Politics, economics, and the problems of development. Cambridge: Cambridge University Press.
- NSO (National Statistical Office). 2018. Malawi population and housing census- main report. Zomba: National Statical Office.
- Parsons, M., S. Glavac, P. Hastings, G. Marshall, J. McGregor, J. McNeill, … R. Stayner. 2016. Top-down assessment of disaster resilience: A conceptual framework using coping and adaptive capacities. International Journal of Disaster Risk Reduction 19: 1–11.
- Pat-Horenczyk, R., and M. Schiff. 2019. Continuous traumatic stress and the life cycle: Exposure to repeated political violence in Israel. Current Psychiatry Reports 21, no. 8: 1–9.
- Pingali, P., L. Alinovi, and J. Sutton. 2005. Food security in complex emergencies: Enhancing food system resilience. Disasters 29: S5–S24.
- Quisumbing, A.R. 2003. Food aid and child nutrition in rural Ethiopia. World Development 31: 1309–24.
- Regan, P.M., and H. Kim. 2020. Water scarcity, climate adaptation, and armed conflict: Insights from Africa. Regional Environmental Change 20: 1–14.
- Salehyan, I., and C.S. Hendrix. 2014. Climate shocks and political violence. Global Environmental Change 28: 239–50.
- Sharifi, A. 2016. A critical review of selected tools for assessing community resilience. Ecological Indicators 69: 629–47.
- Smith, L.C., and T.R. Frankenberger. 2018. Does resilience capacity reduce the negative impact of shocks on household food security? Evidence from the 2014 floods in Northern Bangladesh. World Development 102: 358–76.
- Stevens, G., G. Eagle, D. Kaminer, and C. Higson-Smith. 2013. Continuous traumatic stress: Conceptual conversations in contexts of global conflict, violence and trauma. Peace and Conflict: Journal of Peace Psychology 19, no. 2: 75.
- Swift, J. 2006. Why are rural people vulnerable to famine?
- Vishwanath, A., 2015. The water wars waged by the Islamic state. Global Affairs. Washington, DC: Stratfor.
- Von Lossow, T. 2016. The rebirth of water as a weapon: IS in Syria and Iraq. The International Spectator 51, no. 3: 82–99.
- Vyas, S., and L. Kumaranayake. 2006. Constructing socio-economic status indices: How to use principal components analysis. Health Policy and Planning 21, no. 6: 459–68.
- World Food Programme. 2008. Food consumption analysis: Calculation and use of the food consumption score in food security analysis. WFP: Rome, Italy.
- Young, A.T., and J. Bologna. 2016. Crises and government: Some empirical evidence. Contemporary Economic Policy 34, no. 2: 234–49.
- Zürcher, C. 2017. What do we (not) know about development aid and violence? A systematic review. World Development 98: 506–22.
Appendix
Table A1. Results from principal components analysis in Malawi.
Table A2. Results from principal components analysis in Uganda.