ABSTRACT
Empowering students to actively shape their learning is essential. Various student involvement models, such as design-based research, participatory design, and co-creation, emphasise students’ growing role in shaping educational activities. Engaging students in course design, as seen in student co-creation, can enhance agency, improve the student experience, and boost outcomes. Considering this, we propose a systematic approach combining multi-criteria decision-making with constructive alignment theory to involve students as co-creators in course design. This approach aims to engage students in the course design process and co-create intended learning outcomes, which can be regarded as the primary participatory phase in developing a co-created course. By involving students, our approach aims to gain insight into their needs, prioritise their views, and guide the formulation of appropriate course specifications throughout the course design process. The approach is applied to a new multi-disciplinary engineering course, and the results are summarised following its corresponding steps. Students’ feedback indicates that the approach positively influenced their motivation, engagement with course objectives, collaboration with teachers, and overall achievement of intended learning outcomes. This study’s significance lies in its contribution to higher education, offering a more integrated and systematic approach to support co-creation between educators and learners in academic course design.
1. Introduction
Contemporary educational literature highlights how students are becoming increasingly involved in the creation of educational activities for both teaching and learning purposes, which is evident in various approaches such as design-based research, participatory design, co-creation, co-design, student voice, student-staff partnership, student engagement, and student empowerment (Anderson and Shattuck Citation2012; Bovill et al. Citation2016; Seale Citation2009). Students are becoming central actors in a co-creation approach since the aim is to increase the active engagement of students in the educational (design) process and improve teaching and learning by welcoming students’ views (Blair and Valdez Noel Citation2014; Bovill et al. Citation2016). It falls under the broader concept of ‘students as partners’ or ‘partnership’ in higher education (Bovill Citation2020b, 1024). This collaborative effort can serve various purposes, including evaluating course content, redesigning courses, researching teaching and learning, designing assessment tasks, and participating in grading processes (Bovill et al. Citation2016). Co-creation can range from limited influence on decision-making to deep partnerships with teachers, allowing for a more profound level of student engagement (Bovill and Bulley Citation2011).
Given the value of involving students as co-creators in the course design (Bovill et al. Citation2016; Bovill, Cook-Sather, and Felten Citation2011), this article aims to propose a novel systematic approach that integrates the multi-criteria decision-making (MCDM) method into Constructive alignment theory (CAT) for designing a course with students can be considered as co-creators. CAT is commonly used for course design and begins with the end in mind (i.e. what students should know and be able to demonstrate at the end of the course) (Biggs Citation1996). CAT posits that when intended learning outcomes (ILOs), teaching and learning activities (TLAs), and assessment tasks (ATs) are intentionally aligned, the outcomes of learning are improved (Blumberg Citation2009). As constructive alignment means when teaching and assessment are in harmony with the ILOs, identifying clear ILOs is important to enhance the quality of learning students’ experience (Edström et al. Citation2003). The ILOs serve as the foundation for the rest of the course design process. Taking this into account, with the proposed approach, we want to involve students’ views in the course design process for co-creating the ILOs, which can be considered a first participatory step in constructing a co-created course. We acknowledge that these students’ views then play a major role in the subsequent planning of the TLAs and ATs and thus lead to a good alignment of all these elements in the design process. Here, we also make an assumption guiding our study that this co-creation of ILOs helps to gain an explicit understanding of student needs (i.e. which elements in their learning they believe are important for them to concentrate on at that point in time), and this might result in enhanced learner outcomes since the content can be structured in a student-centered way. Thus, the ILOs can be prioritised based on the students’ views, which are then constructively aligned with TLAs and ATs throughout the course design process. The reason for prioritising the identification of ILOs in the CAT process is that it serves as the initial and crucial step that sets the stage for aligning TLAs and ATs in the subsequent phases. The proposed approach is implemented for a new multi-disciplinary course design developed for a manufacturing engineering-related Master of Science programme. The results are summarised according to the corresponding steps of the proposed approach. Furthermore, insights obtained from interviews with students who participated in this study underscore the evidence supporting a positive impact on student’s motivation and their engagement with course objectives resulting from applying the proposed approach. In summary, the approach provides the opportunity to capture students’ views through their involvement in the course design process in a more integrated manner. It contributes to a more systematic way of designing co-created academic courses in higher education.
In addition, inviting students to be partners with academic staff in pedagogical planning and design can also pose some key challenges from two perspectives (e.g. students and academic staff). For example, students often doubt that they will be taken into account seriously in this process and also might need time to develop the language and confidence to articulate their pedagogical ideas (Bovill, Cook-Sather, and Felten Citation2011) on the other hand, academic staff might feel uncomfortable with the necessary change in power relations as a more democratic pedagogical planning process requires, and also they might show resistance on new approaches since it can be viewed as time-consuming if they already feel overloaded with work (Bovill, Cook-Sather, and Felten Citation2011). Drawing upon the potential and challenges presented above, we believe that our approach can also support academic staff to take small steps in promoting student participation in pedagogical planning and design in a way, trying out something more contained, structured, and manageable for them and also the students. To sum up, following the systematic approach outlined in this article and taking gradual steps can assist both students and staff in effectively navigating the co-creation of course design.
The rest of the article is organised as follows. In the theoretical background, relevant state-of-the-art knowledge for the proposed approach is presented. The proposed approach section describes each step taken throughout the integration of the MCDM method into CAT. Thereafter, results are provided by exemplifying the implementation of the proposed approach in a new course design. The article concludes by discussing the limitations and further improvements of the proposed approach.
2. Theoretical background
2.1. Course design using CAT
CAT focuses on the mechanisms for aligning all the components of learning, i.e. the systems of teaching, learning, and assessing (Biggs and Tang Citation2011). It is a conscious effort to provide learners with specified goals and ILOs, well-designed TLAs that are appropriate for the task, and well-designed ATs for providing feedback to learners (Biggs and Tang Citation2011). For a comprehensive overview of CAT, the reader is referred to Biggs and Tang (Citation2011). An example of applying CAT in a degree project course in engineering education was presented by Malmqvist, Knutson Wedel, and Enelund (Citation2011). Wang et al. (Citation2013) explored the relationship between instructors’ adoption of Biggs’ CAT in a course design and students’ learning approaches. The authors demonstrated that a constructively aligned teaching and learning environment encouraged students to employ a more deep approach by taking increased responsibilities to construct their knowledge, as also emphasised in Biggs (Citation1996). Students are affected by the decisions taken in the design of their education, and their views therefore need to be considered in a more structured way throughout the course design process (Vanfretti and Farrokhabadi Citation2015).
2.2. Student involvement in the design of teaching and learning activities
Bovill et al. (Citation2016) highlight in modern educational literature that students are increasingly participating in the design of educational activities for teaching and learning purposes. This is reflected in approaches to student participation (or involvement) such as design-based research, participatory design, co-creation, co-design, student voice, student-staff partnership, student engagement, and student empowerment (Anderson and Shattuck Citation2012; Bovill et al. Citation2016; Seale Citation2009). It should be noted that similarities and differences between these approaches to involving students in the design of pedagogical practices have still remained uncertain, and terminology has not been separated in terms of definitions (Martens et al. Citation2019). In a study done by Martens et al. (Citation2019), they identified the most frequently used terms related to student participation in the design of teaching and learning, which are design-based research, participatory design, student voice, and co-creation in order to comb out the terminology with a certain focus on relevant definitions, aims, involvement of students, and outcomes. Whilst there is an overlapping nature of many terms, and the similarity is reflected in a shared perception of valuing students’ input as stakeholders in the educational design process, the difference between the approaches has been observed in the degree of student participation in the design process (i.e. students play as central actors) and the focus on educational theory.
As with design-based research, researchers and educational practitioners collaborate to improve the design of the learning environment and to advance the theoretical understanding of education (Anderson and Shattuck Citation2012). This approach provides an iterative design process in which other stakeholders, such as students and educational designers, are involved, however, students’ roles can often be limited by only giving input within the design process (McKenney and Reeves Citation2018).
In participatory design, students collaborate with educators as design partners to shape their learning experience and develop innovations that are tailored to the learners and context (Könings and McKenney Citation2017). The main advantage is that both students and educators are encouraged to reflect on teaching and learning methods by sharing their points of view in the design process (Cober et al. Citation2015). A case study presented by Könings, Seidel, and van Merriënboer (Citation2014) highlights the positive change in the level of student engagement, intellectual stimulation, and, ultimately student experience as a result of the participatory design of the learning environment. However, this is not simple and thus requires much more effort in how involvement in such design processes needs to proceed (Könings, Bovill, and Woolner Citation2017). Based on the context and the stages of the process, it can be decided what level of participation might be reasonable (Bovill and Bulley Citation2011), and the main challenge is, therefore to find a way for a realistic and relevant participation level during the different phases of the design process.
Co-creation has been described by Ryan and Tilbury (Citation2013) as a new pedagogical idea that emphasises empowering students to actively collaborate with teachers. There has been increasing interest in research and practice about ‘students as partners’, ‘partnership’, and ‘co-creation of learning and teaching’, which are terms that are often used interchangeably in higher education (Bovill Citation2020b, 1024). In the co-creation of learning and teaching, students and teachers work collaboratively with one another by aiming to reinforce the active engagement of students in the educational (design) process by welcoming students’ perspectives (Bovill et al. Citation2016; Martens et al. Citation2019). This collaboration may be developed for different purposes, e.g. evaluating course content and learning and teaching processes; (re)designing the content of courses; researching learning and teaching; designing assessments tasks; and grading their own and others’ work (Bovill et al. Citation2016). Several frameworks have been also proposed to conceptualise existing partnership and co-creation roles (i.e. students’ roles range from being involved with limited influence on decision-making to working in a partnership with teachers) (Bovill Citation2020b). For instance, Bovill et al. (Citation2016, 197) recommend one way to do that is ‘co-creation is occupying the space in between student engagement and partnership, to suggest a meaningful collaboration between students and staff, with students becoming more active participants in the learning process, constructing understanding and resources with academic staff.’ In co-creation, the involvement of students can go up to being at the highest level, which means students are having more influence on decision-making in the design process and thereby it refers to a much deeper level of student engagement (Bovill and Bulley Citation2011). Therefore, co-creation can be a more appropriate approach for enhancing active student engagement, student experience, and the effectiveness of the learning environment.
Involving students in key pedagogical decisions about the learning content and instructional methods is posited to make them more motivated and engaged during their learning (Brooman, Darwent, and Pimor Citation2015). Student engagement is considered crucial to student success in higher education, with engagement it can be understood as a significant interest in, active taking up of, and commitment to learning (Kuh et al. Citation2011). In this context, an implicit assumption is that students will become more engaged if they are able to participate in key decisions about the context and content of their learning, as highlighted in the student engagement agenda (Seale Citation2009). When students take authentic responsibility for the educational (design) process, their roles are shifted from being passive to active, which means they simply act out what is required of them to learn and analyse consciously what constitutes and enhances that learning, and this can make students more likely to adopt deep approaches to learning (Cook-Sather Citation2014). In some definitions of engaged learning, students take an active role in the learning process (Wolf-Wendel, Ward, and Kinzie Citation2009), with more recent calls, students have been encouraged to become co-creators of learning (Bovill, Cook-Sather, and Felten Citation2011; Davis and Sumara Citation2002). One example of student engagement is demonstrated in a study done by Cook-Sather (Citation2011), in which undergraduate students were involved as consultants in the development of curricula and pedagogic approaches. With this study, the author presented that not only did explicit consultation with students have a positive impact on the practice of lecturers, but also led to a higher level of self-confidence and learner agency amongst the students. ‘Like engagement, student voice is a theory and set of practices that position students as active agents in analyses and revisions of education’ (Bovill, Cook-Sather, and Felten Citation2011, 2). For an overview of students’ voice work in higher education and its definitions, the reader is referred to Seale (Citation2009).
Moreover, incorporating students’ views through different forms of student participation in pedagogical planning (e.g. co-creators of teaching approaches, course design, and curricula) may create more empowered education systems (Bovill, Cook-Sather, and Felten Citation2011). In the context of curricula design, there has been a growing body of literature that emphasises the importance of the empowerment of teachers in the design process (Cober et al. Citation2015) to take small steps in collaborative pedagogical planning, as well as evidence that student participation in learning design leads to increase satisfaction with the learning experience (Cook-Sather Citation2014; Könings, Seidel, and van Merriënboer Citation2014). Additionally, Edström et al. (Citation2003) showed that addressing students’ participation can help to identify the shortcomings in course designs. In summary, having the opportunity to involve students’ views in developing pedagogical approaches and designing curricula/courses generally points out the benefits for students and academic staff like experiencing an enhanced engagement, motivation, and enthusiasm, as well as gaining a deeper understanding of learning (Bovil et al., 2011).
2.3. MCDM in higher education
MCDM is widely used to develop and implement decision support tools and approaches to solve complex decision problems containing multiple criteria, goals, or objectives of conflicting nature (Zopounidis and Doumpos Citation2002). According to a literature review study done by Yüksel, Kayadelen, and Antmen (Citation2023), MCDM has been widely applied in higher education, particularly for improving e-learning and prioritising performance indicators and evaluation. An MCDM method was used by Tsinidou, Gerogiannis, and Fitsilis (Citation2010) to identify the quality determinants for education services provided by higher education institutions. Similarly, Thanassoulis et al. (Citation2017) proposed an MCDM approach to evaluate higher education teaching performance. Liu et al. (Citation2013) applied an integrated MCDM approach in industrial design curricula planning by identifying the most important professional competencies required of industrial design professionals in industry and academia. Kamvysi et al. (Citation2014) proposed an integrated MCDM approach to prioritise students’ requirements for a course design in higher education. Vanfretti and Farrokhabadi (Citation2015) presented the implementation of the CAT in a power system analysis course through a consensus-based course design process. This involved both the instructor and graduate-level students to develop the CAT framework by including different perceptions.
The main benefits of MCDM are presenting a systematic approach for helping decision-makers in screening, evaluating, prioritising, ranking, or selecting a set of alternatives (also referred to as ‘candidates’ or ‘actions’) under multiple criteria with respect to their preferences (Triantaphyllou Citation2000). MCDM enables decision-makers to make quick, accurate, and reliable decisions by providing structured decision-making, transparency, and objectivity to communicate the rationale behind decisions (Zavadskas, Turskis, and Kildienė Citation2014). Considering the benefits above, this study proposes a novel systematic approach based on integrating an MCDM method into CAT to evaluate ILOs by collecting the students’ views and prioritising their requirements in a course design process. There are numerous MCDM methods in the literature, and each of them has its specific characteristics and applicability (Velasquez and Hester Citation2013; Zavadskas, Turskis, and Kildienė Citation2014). In this study, the Technique for Order Preference by Similarity to an Ideal Solution (TOPSIS) method is chosen due to several key advantages: simplicity to structure the problems to be solved, comprehensibility to conduct analyses, and good computational efficiency with the ability to measure the relative performance for alternatives in a simple mathematical form (Tzeng and Huang Citation2011). TOPSIS is a practical and widely applied method for solving various MCDM problems (Shih, Shyur, and Lee Citation2007). To the best of the authors’ knowledge, this is the first study that combines the implementation of the CAT and MCDM for the involvement of students in a course design process as co-creators.
3. The proposed approach
This study proposes a combined approach based on CAT and MCDM for a course design. The proposed approach can be implemented in different stages within a course design process, such as in the early design (pre-design) and late design (post-design). In a pre-design implementation, the students evaluate the ILOs in an early stage of the design process. The other course elements, such as TLAs and ATs, are designed and aligned according to the prioritised ILOs based on the student’s requirements. In this context, the students could be actively involved in the design process of a course from the beginning. On the other hand, in a post-design implementation, the students evaluate the ILOs in the late stage of the design process. The prioritised ILOs are used as inputs for improving the course by revising already designed TLAs and ATs. In this study, the proposed approach is implemented in the post-design stage of a newly designed course. Therefore, shows the proposed approach with a post-design implementation.
Figure 1. An overview of the proposed approach combining CAT and MCDM with a post-design implementation.
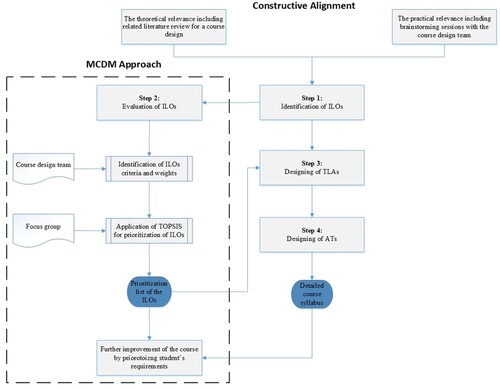
As is seen in , this approach consists of the following four main steps:
Step 1: Identification of ILOs.
Step 2: Evaluation of ILOs. This includes two sub-steps:
o Step 2.1 – Identification of criteria and weights of ILOs.
o Step 2.2 – Application of TOPSIS.
Step 3: Designing of TLAs.
Step 4: Designing of ATs.
In Step 1 (identification of ILOs), the ILOs are identified in terms of theoretical and practical relevance. The theoretical relevance includes related literature that covers constructive alignment and Bloom’s taxonomy used for the identification of the course ILOs (Bloom Citation1956; Krathwohl Citation2002). The practical relevance includes brainstorming sessions with the course design team to analyse the identified ILOs in terms of their clarity and levels in Bloom’s taxonomy.
In Step 2 (evaluation of the identified ILOs), the identified ILOs are prioritised using the TOPSIS technique under some criteria by focus group including students. This step includes two sub-steps, which are explained in the following paragraphs.
In Step 2.1 (identification of the ILOs’ criteria and their weights), the course design team identifies a subset of essential criteria utilised to prioritise the ILOs of course. These are theory-based knowledge, practical-based knowledge, key transferable skills, and generic academic skills. Theory-based knowledge provides fundamental knowledge regarding the concepts and expertise about their mechanism (Allan Citation1996). Practical knowledge guarantees that you can do something instead of simply knowing how to do it, and it is gained through hands-on exercises (Allan Citation1996; Kamvysi et al. Citation2014). Analytical, synthetic, and critical thinking skills are covered under the umbrella of generic academic skills (Allan Citation1996; Kamvysi et al. Citation2014). Key transferable skills comprise communication skills, IT skills, problem-solving, and decision-making skills, planning-organizational and time management skills, team leadership and negotiation skills, and information gathering-research skills (Allan Citation1996; Kamvysi et al. Citation2014). After identifying the ILOs criteria, they are evaluated by a focus group to calculate their weights that will be utilised in the application of the TOPSIS method for prioritisation of the ILOs. A matrix is designed to collect data using a linguistic scale from the focus group to evaluate the ILOs criteria as given in Appendix A. The evaluation matrixes of the ILOs criteria obtained from the focus group are aggregated by applying the geometric mean method (GMM) that is commonly used to aggregate assessments of individuals within a group. GMM reduces the bias effect of extreme values (very low or very high) (Shih, Shyur, and Lee Citation2007) and is selected to obtain the aggregated matrix. Afterward, this matrix is normalised to calculate the weights of the ILOs criteria using a widely used normalisation technique in MCDM (Shih, Shyur, and Lee Citation2007).
In Step 2.2 (application of TOPSIS method for prioritisation of ILOs), the data is first collected again from focus groups using the designed matrix for the evaluation of the ILOs of the course as given in Appendix B. Afterwards, the TOPSIS method includes some specific steps that are applied to generate a priority ranking of the identified ILOs. The underlying principle is to select an alternative closest to the positive ideal solution and farthest from the negative ideal solution (Hwang and Yoon Citation1981). The TOPSIS method begins with constructing the evaluation matrix of the ILOs based on each ILO criteria identified in Step 2.1. Next, the matrix is normalised with a proper normalisation procedure, and the values are multiplied by ILOs criteria weights calculated in Step 2.2. Subsequently, the positive-ideal and negative-ideal solutions are calculated, and the distance of each ILOs to these solutions is calculated with a distance measure. This is done using the Euclidian distance metric, commonly used in the classical TOPSIS method to calculate distances (Erpolat Taşabat Citation2019; Olson Citation2004). Finally, the ILOs of the course are ranked based on their relative closeness to the ideal solution. For an in-depth description of the TOPSIS method, including detailed mathematical formulations, see Dymova, Sevastjanov, and Tikhonenko (Citation2013).
In Step 3 (designing of TLAs), the TLAs of the course are designed to motivate and encourage the students to reach the levels of understanding specified in the ILOs. In this step, the following five principles recommended by Meyers and Nulty (Citation2009, 567) are considered to maximise the quality of learning outcomes. These principles provide students with teaching and learning materials, tasks, and experiences which:
are authentic, real-world, and relevant;
are constructive, sequential, and interlinked;
require students to use and engage with progressively higher-order cognitive processes;
are aligned with each other, and the desired learning outcomes; and
provide challenge, interest, and motivation to learn.
What activities are appropriate for students to reach the identified ILOs should be answered by considering these five principles. It is also important to consider applying active learning methods to engage students directly in thinking and problem-solving activities, particularly in engineering education (Christie and De Graaff Citation2017). Furthermore, active learning is considered experiential when students take on roles that simulate professional engineering practice, such as design-implement projects, simulations, and case studies (Crawley et al. Citation2014).
In Step 4 (designing of ATs), the ATs of the course are aligned with all other course components. Biggs (Citation1996) suggested several types of assessment methods related to the kind of learning assessed, comparing the levels of understanding of the ILOs. Therefore, different assessment tasks are selected, e.g. group and individual assessment components with a focus on problem-based learning. Problem-based learning is an effective way of integrating learning activities and assessment tasks while concurrently preparing the students for problems they will face in their professional careers (Skoogh, Johansson, and Williams Citation2012).
It is important to clarify that the TLAs for the course are devised by the course design team, and students are not directly involved in their co-creation. As outlined in the introduction section, the proposed approach seeks to engage students in the course design process by incorporating their insights into the co-creation of the ILOs, which represents the initial collaborative step in shaping a co-created course. By prioritising ILOs based on students’ perspectives and aligning them constructively with TLAs and ATs throughout the course design process, this approach aims to facilitate a successful co-creation of the course. In addition to this, steps 1 and 2 in the proposed approach play a critical role in guiding steps 3 and 4. The initial step of defining ILOs (step 1) is particularly crucial in CAT as it establishes the course's purpose and direction. Step 2, which involves evaluating the identified ILOs, allows for the prioritisation of learning outcomes based on students’ perspectives. This prioritised list then guides the selection of appropriate TLAs (step 3) and ATs (step 4) and ensures that they are aligned with the desired learning outcomes. By incorporating step 2 into the CAT process, the proposed approach guarantees that TLAs and ATs that correspond to the most significant ILOs are adequately addressed in course design.
4. Implementation of the proposed approach in a new course design
In this section, the proposed approach is implemented in the post-design stage of a new multi-disciplinary and elective course for the manufacturing engineering-related master programmes at X University. After a brief description of the course, the results from each step of the proposed approach are presented in the following paragraphs.
4.1. Motivation for the design and a brief description of the course
Digitalisation has the potential to transform numerous industry sectors by introducing new technologies such as embedded sensors, cyber-physical systems, and data analysis, collectively referred to as ‘Industry 4.0’ (Gulati and Soni Citation2015; Wee et al. Citation2015). Industry workers require technical skills and awareness to drive competitiveness and business value due to rapid technological developments (Hadgraft and Kolmos Citation2020). One specific area of recent developments includes the use of data science techniques for data-driven decision-making to improve products or production systems (Lorenz et al. Citation2015). Therefore, this demands understanding the manufacturing domain (e.g. production processes), information technology (IT) systems, and being skilled in applying data science techniques (Lorenz et al. Citation2015). Manufacturing-related engineering programmes should be updated to teach data science techniques such as Artificial intelligence (AI) and Machine learning (ML) and provide a better understanding of their applications in different industries, in response to this demand (Coşkun, Kayıkcı, and Gençay Citation2019). Li et al. (Citation2021) recently presented such requirements by addressing the widening skills gap in the process of digital transformation of the manufacturing industry. They recommend updating traditional manufacturing education to focus more on data and analytics. Curricula in relevant engineering programmes need to be updated with data science skills and knowledge, as per Li et al. (Citation2021). Rooted in this real-world demand, we designed a new course to teach students data science techniques, including AI and ML, to improve production systems and product development by demonstrating real-world case studies throughout the course.
4.2. Results from Step 1 (identification of the ILOs of the course)
shows the focus points that are considered essential in identifying the ILOs for this course, which are the right balance between theory and practice, the proper application of data science techniques by exemplifying with industrial case studies selected from the product realisation life cycle and following a structured data science methodology. The points illustrated in served as the basis to answer the question, ‘What should students know or be able to do as a result of the course’.
Additionally, the identified ILOs are analysed in terms of their clarity and levels in Bloom’s taxonomy by the course design team. summarises the levels of Bloom’s taxonomy associated with each of the identified ILOs of the course.
Table 1. Analysis of the identified ILOs in terms of clarity and levels in Bloom’s taxonomy.
4.3. Results from Step 2.1 (identification of the ILOs’ criteria and their weights)
After the identification of the ILOs’ criteria by the course design team, they are evaluated by a focus group consisting of three randomly selected student representatives of the course to calculate the criteria’ weights to be utilised in the application of the TOPSIS method. In accordance with the university’s policy, student representatives are chosen through a random selection process from among the participants enrolled in a course. These selected student representatives are entrusted with the responsibility of representing both themselves and their fellow students during course evaluation meetings held throughout and after the course. Their role as student representatives holds significant importance as they aid the course examiner or teacher in gaining a deeper understanding of students’ expectations and their overall course experiences.
Furthermore, to understand the general portfolio of the students taking the course, they are asked to fulfill a self-introductory survey at the beginning of the course. It is seen from the survey’s results given in that most of the students are studying in the Master Programs of Production Engineering (MPPEN) and Product Development (MPPDE). Further, some of them are studying in other master’s programmes, and only two of them are exchange students.
The initial evaluation process involved a small focus group of three students who were randomly selected to represent the student cohort of the course. From a methodological perspective, the literature on MCDM techniques such as TOPSIS does not prescribe a specific number of experts or decision-makers needed but rather suggests selecting them based on their expertise and knowledge of the subject matter. While acknowledging the potential benefits of co-creation and student participation in learning, teaching, and assessment activities, a typology by Bovill (Citation2019) shows that co-creation can involve various numbers of students from previous years, the current cohort, or future students, ranging from the individual student to small groups or even an entire class. Naturally, the process and experience of co-creation would differ significantly depending on whether one staff member works with a single student or a larger group of students and can focus on different aspects of the course. To summarise, we recognise that the selection of the students who participated in co-creating the course design in our study was appropriate from both methodological (i.e. these students had diverse backgrounds and experiences that aligned well with the overall course profile) and co-creation perspectives, as explained above.
The following table shows the weights of the ILOs’ criteria based on the aggregated evaluation matrixes obtained from the focus group using GMM and the normalisation technique.
According to , the most critical ILOs’ criteria are practical-based knowledge and key transferable skills. This result is not surprising since the course combines the theoretical understanding of the concepts by demonstrating practical applications and use cases of real-world industrial problems selected from the product realisation life cycle.
Table 2. The weights of the ILOs’ criteria.
4.4. Results from Step 2.2 (application of TOPSIS method for prioritisation of ILOs)
The core steps of the TOPSIS method are performed to rank the identified ILOs under each ILOs criteria by using their weights as given in . shows the ranking results of the TOPSIS method.
According to , based on the evaluation of the students’ representatives of the course, the three most critical ILOs are: ‘ILO9. Critically analyse and argue key ethical principles and potential impacts of AI on people and society and evaluate social and human requirements of systems and scenarios’, ‘ILO7. Interpret and discuss state-of-the-art knowledge from scientific papers related to data science in mechanical engineering’, and ‘ILO8. Implement commonly used AI/ML algorithms, analyse their performance, and discuss their application using industrial applications from product realisation life cycle’. These ILOs should be prioritised to provide ‘‘key transferable skills’’ in combination with ‘‘practical-based knowledge’’, which are essential features of the educational process enhancing students’ employability.
4.5. Results from Step 3 (designing of TLAs)
In this course, project-based learning, which is an experiential learning method (Crawley et al. Citation2014), is designed to provide students with opportunities to explore new concepts, take on new problems, formulate the problems by experiencing in different ways, and reflect on these experiences to improve their performance in an iterative cycle based on a structured project methodology. This methodology is called the Cross-Industry Standard for Process Mining (CRISP-DM), a systematic approach for planning, executing and deploying data science projects (Wirth and Hipp Citation2000, april). The course is divided into four modules, and each module covers the following topics:
Module 1 – Introduction to Data Science
Fundamentals of data science (AI/ML)
An overview of data-driven modelling
Introducing toolboxes for data scientists
Module 2 – Data Mining & Visualisation
Introduction to the data mining process and structured work procedures
Plotting for exploratory data analysis
An overview of data quality dimensions
Methods for data pre-processing
Module 3 – AI and ML
A general introduction to AI and ML
Examples of ML algorithms to understand in what situations they can be used
Examples of deep learning
Analysis of different industrial applications from product realisation life cycle using AI/ML
The ethics of AI (is covered by reading scientific papers and discussion in literature seminar presentation)
Module 4 – How to drive AI in your business – Project work
Practicing with group work project for understanding AI/ML systems through the appropriate formulation of the selected industrial cases from product realisation life cycle
Different TLAs based on active and experiential learning methods are used during the course as summarised below:
Lectures: To support the basis for the theoretical understanding of data science concepts.
Guest lectures: To support the basis for understanding different industrial data science applications.
Workshops: To reinforce the learning via more engagement with the students related to data utilisation and analytics of the selected industrial cases from the product realisation life cycle.
Software seminars: To support learning the necessary data scientist toolboxes, which are expected to be used in examination project work.
Self-paced hands-on exercises supported by Q&A sessions afterward: To train in an interactive tutorial to prepare the students for software seminars and examination project work. This is a student-led learning activity.
Literature seminar: To present and discuss scientific papers related to applications of data science in the product realisation process
Examination project work: To practice skills learned throughout the course based on CRISP-DM methodology.
A summary of the TLAs for each course module is given in .
Table 3. A summary of the designed TLAs for each course module.
4.6. Results from Step 4 (designing of ATs)
Different ATs are designed based on open problem-solving, meaning that the expected outcomes of tasks are not specified on a detailed level. Instead, the deliverables are more defined as objectives that allow for several possible solutions. It becomes essential for the students to argue for and against different alternatives. On the other hand, students must also be approved on some ATs individually, such as the reports of self-paced hands-on exercises, mandatory online knowledge test, a programming assignment, and group-based ATs such as literature seminar presentation and examination project work to pass the course. Grading is based on the examination project work, including a technical report and recorded oral presentation directly related to one of the top prioritised ILOs of the course (i.e. ILO8).
According to the prioritised ILOs based on the focus group’s views, there are many possibilities to use such information to improve further the course, such as re-designing some of the TLAs, ATs, or specific learning content. For instance, ILO7 and ILO9 are aligned with the literature seminar presentation that aims to provide insights into the development of data science applications in product realisation and discuss how future product developers and production engineers can implement and learn from state-of-the-art applications. This literature seminar presentation was only conducted once during the course. However, it could also be designed as a literature seminar series that runs throughout the course. This may contribute to reinforced learning with respect to the prioritised ILOs (e.g. literature seminar part 1 that focuses on the ethics of AI and literature seminar part 2 that focuses on the state-of-the-art applications of data science).
5. Discussion
5.1. Highlights and contributions of the proposed approach
This article aims to provide a systematic approach that combines CAT with an MCDM method (TOPSIS) to facilitate student involvement in the course design. This approach rests on a motivational perspective on teaching and learning (Ginsberg and Wlodkowski Citation2009) that includes the creation of challenging and thoughtful learning experiences that incorporate students’ views. Positive perceptions of students about the learning environment influence not only academic achievement but also qualitative learning outcomes (Lizzio, Wilson, and Simons Citation2002; Trigwell and Prosser Citation1991). Students are the most valuable resources for creating meaning in their learning. Thus, they need to be provided with opportunities to be involved in course design, i.e. act as co-creators. This would make students more motivated, engaged, and responsible for a deeper understanding of their own learning (Bovill, Cook-Sather, and Felten Citation2011). Existing approaches have primarily utilised an open discussion process for gathering qualitative data on students’ views. The use of such approaches involves gathering and analysing data through methods such as focus groups, interviews, observations, and co-creation workshops to understand the subjective experiences and perspectives of students and other stakeholders involved in the process (Bovill Citation2019; Bovill Citation2020a). While such approaches are still useful and provide valuable insights, they often lack systematic procedures that make it more difficult to integrate the findings into the course design. Because they typically focus on an in-depth exploration of specific cases or contexts, their findings may not be easily generalisable, which makes it difficult to apply them in broader decision-making contexts (Ochieng Citation2009). Additionally, reproducing and interpreting the data obtained through qualitative approaches can be challenging due to subjective interpretation and resource-intensive data gathering and analysis (Creswell Citation2012). MCDM techniques offer a solution to these issues, enabling decision-makers to make quick, accurate, and reliable decisions while integrating either qualitative and quantitative data or a mix of them at the same time (Zavadskas, Turskis, and Kildienė Citation2014). MCDM can also provide some other benefits, including structured decision-making to ensure all factors are considered and weighted appropriately, transparency and objectivity to communicate the rationale behind decisions, consistency, and repeatability across different contexts, increased efficiency, and improved stakeholder involvement in the decision-making process (Triantaphyllou Citation2000). Therefore, this study contributes to the literature by proposing a structured approach for involving students’ views in the course design by integrating MCDM and CAT to enhance the integration of subjective judgments when prioritising students’ requirements regarding ILOs.
The idea of students becoming involved in co-creating course design, curricula, and pedagogic approaches has become increasingly popular in higher education over recent years (Wood and Cajkler Citation2016). On the other hand, this literature pays relatively little attention to the issue of power relationships between academic staff and students (Könings, Bovill, and Woolner Citation2017). Positioning students as partners with valuable perspectives is key to supporting collegial partnerships between academic staff and students to clarify and improve teaching and learning practices (Bovill, Cook-Sather, and Felten Citation2011). However, it is important to note that increased student involvement in pedagogical planning does not mean replacing teachers’ expertise and their key role in facilitating learning (Bovill, Cook-Sather, and Felten Citation2011). We are aware of this in our approach, so the proposed approach takes into account treating students and teachers in this way (i.e. students are assumed to have knowledge of what they want to learn, and teachers hold the nature of expertise during the course design process). The practical purpose here is to co-designing the course and, perhaps more importantly, to develop a true way of collaborative approach that welcomes students’ views.
The present study implemented the proposed approach to create a novel multi-disciplinary and industry-focused course, as described in Section 4. This course merges two different domains (data science and manufacturing), and it applies project-based learning with real-world industrial case studies from the product realisation life cycle to improve products and production systems. With industrial digitalisation, it has been becoming increasingly important for industry workers to possess the technical skills and competence to utilise data and perform data analytics. This emerging trend requires well-educated engineers who can contribute to the digitalisation of the manufacturing industry by filling the data science and analytics skills gap (Li et al. Citation2021). Therefore, there is a dire need to design novel pedagogical initiatives to develop competence and skills, particularly within manufacturing-related engineering programmes for industrial data-driven applications. In this context, this course can also be considered a good example of pedagogical initiatives that contribute to transforming traditional manufacturing-related engineering education curricula towards an emphasis on data and analytics. This study highlights how such a course can be designed by incorporating students’ views and active involvement. This supports the integration of multiple domains, a blend of theory and practice, and achieves constructive alignment of the course elements. While this study offers valuable contributions, it should be noted that the absence of longitudinal evaluation data can be acknowledged as a limitation of our current research. This limitation highlights the importance of future studies taking into account longitudinal dimensions when evaluating the effectiveness of the co-creation approach. Hence, it is important for forthcoming research to address this limitation and explore the possibility of conducting longitudinal assessments to gain a more profound understanding of how the co-creation approach may have sustained impacts on students’ outcomes and experiences.
5.2. Experiences, insights, and potential impact on students’ learning
By engaging with students in the proposed approach, it can be easier to gain an explicit understanding of student learning experiences, student prior learning, and which elements in their learning they believe are important for them to concentrate on at that point in time (e.g. what they prioritise in the ILOs, and this is most likely associated with the learning challenges based on their prior experiences). Therefore, such insights can then be used to guide subsequent planning of the other design elements, and the co-creation of ILOs can be a continuous part of the improved design of the course. On the positive side, students’ involvement in the co-creation of ILOs tends to improve the understanding of students’ needs, and we believe that it results in a more suitable course design. Yet, the potential for participation may be limited by only prioritising the ILOs in the design process in this study. However, it is important to note that the proposed approach can be easily expanded by involving the students’ views in designing the other elements of the course.
We conducted interviews with focus group to gain insight into the students’ perspectives and experiences during the co-creation of the course and to evaluate the effectiveness of the proposed approach. This interview was divided into two sections. The initial section comprises three questions aimed at obtaining a comprehension of the students’ views on the significance of co-creating a course design and gauging whether the process was practically advantageous to them. Additionally, the subsequent section also contains four questions focused on comprehending the students’ overall experience with the process. summarises focus group interview results As summarised in , it should be noted that applying the proposed approach provided supporting evidence of a positive impact on the student’s motivation and engagement with the course objectives. Furthermore, the evidence from the literature also indicates that co-creating learning and teaching can contribute to enhancing graduate attributes and employability, and to deepening student learning and engagement (Bovill Citation2020b). Our approach is intended to stimulate the creation of learning atmospheres in which students and teachers feel respected and connected and understand that students are effective in learning something they value. also summarises the focus group interview results on students’ experiences of co-creating course design.
Table 4. Summary of focus group interview results on students’ perceptions of co-creating course design.
Table 5. Summary of focus group interview results on co-creation process experience.
6. Conclusions
This study proposes a systematic approach to course design that involves students as co-creators to improve their learning experience by engaging them in course design. Student inputs were used to align design specifications for a new course by prioritising ILOs and aligning TLAs/ATs based on their views. The results showed that by involving students in the course design process, their views can be captured and integrated into the design, resulting in a course that is better aligned with their needs and interests. Furthermore, the participating students in this study provided feedback indicating that the proposed approach positively impacted their motivation, engagement with course learning objectives, collaboration with teachers, and overall achievement of the ILOs. Thus, this study can serve as a source of inspiration for educators to co-create academic courses in higher education with their students, especially for industry-oriented and multi-disciplinary engineering courses.
Acknowledgements
The authors would like to thank the students who took part in the study, as well as to the Production Area of Advance at Chalmers University of Technology for their support.
Disclosure statement
No potential conflict of interest was reported by the author(s).
Correction Statement
This article has been corrected with minor changes. These changes do not impact the academic content of the article.
Additional information
Notes on contributors
Ebru Turanoglu Bekar
Ebru Turanoglu Bekar is currently a Senior Lecturer at the Department of Industrial and Materials Science at the Chalmers University of Technology. Her research focuses on building a structured way to analyse industrial big data and develop algorithms based on artificial intelligence/machine learning techniques in Smart Maintenance. Ebru also actively participates in the teaching of Production Engineering, in particular course development and management, and lecturing.
Anders Skoogh
Anders Skoogh is currently a Professor of production maintenance with the Department of Industrial and Materials Science, Chalmers University of Technology. He is also a Research Group Leader for Production Service and Maintenance Systems. He is also the Director of Chalmers’ Master’s programme in Production Engineering and a Board Member of Sustainability Circle (https://www.sustainabilitycircle.se) with responsibilities for research collaboration.
Jon Bokrantz
Jon Bokrantz is currently a Researcher at the Department of Industrial and Materials Science at the Chalmers University of Technology. His research focuses on industrial maintenance management, and especially the interplay of technology, people, and organisation. His work contributes to the advancement and diffusion of modernised maintenance practices in digitalised manufacturing to maximise operational performance, in particular Smart Maintenance. Jon also conducts research on industrial applications of data science (e.g. machine learning).
References
- Allan, J. 1996. “Learning Outcomes in Higher Education.” Studies in Higher Education 21 (1): 93–108. https://doi.org/10.1080/03075079612331381487.
- Anderson, T., and J. Shattuck. 2012. “Design-Based Research.” Educational Researcher 41 (1): 16–25. https://doi.org/10.3102/0013189X11428813.
- Biggs, J. 1996. “Enhancing Teaching Through Constructive Alignment.” Higher Education 32 (3): 347–364. https://doi.org/10.1007/BF00138871.
- Biggs, J., and C. Tang. 2011. Teaching for Quality Learning at University. McGraw-hill education (UK).
- Blair, E., and K. Valdez Noel. 2014. “Improving Higher Education Practice Through Student Evaluation Systems: Is the Student Voice Being Heard?” Assessment & Evaluation in Higher Education 39 (7): 879–894. https://doi.org/10.1080/02602938.2013.875984.
- Bloom, B. S. 1956. “Taxonomy of Educational Objectives. Vol. 1: Cognitive Domain.” New York: McKay 20 (24): 1.
- Blumberg, P. 2009. “Maximizing Learning Through Course Alignment and Experience with Different Types of Knowledge.” Innovative Higher Education 34 (2): 93–103. https://doi.org/10.1007/s10755-009-9095-2.
- Bovill, C. 2019. “A co-Creation of Learning and Teaching Typology: What Kind of co-Creation are you Planning or Doing?” International Journal for Students as Partners 3 (2): 91–98. https://doi.org/10.15173/ijsap.v3i2.3953.
- Bovill, C. 2020a. Co-creating Learning and Teaching: Towards Relational Pedagogy in Higher Education. St Albans: Critical Publishing.
- Bovill, C. 2020b. “Co-creation in Learning and Teaching: The Case for a Whole-Class Approach in Higher Education.” Higher Education 79 (6): 1023–1037. https://doi.org/10.1007/s10734-019-00453-w.
- Bovill, C., and C. J. Bulley. 2011. “A Model of Active Student Participation in Curriculum Design: Exploring Desirability and Possibility.” In Improving Student Learning (18) Global Theories and Local Practices: Institutional, Disciplinary and Cultural Variations, edited by C. Rust, 176–188. Oxford: The Oxford Centre for Staff and Educational Development.
- Bovill, C., A. Cook-Sather, and P. Felten. 2011. “Students as co-Creators of Teaching Approaches, Course Design, and Curricula: Implications for Academic Developers.” International Journal for Academic Development 16 (2): 133–145. https://doi.org/10.1080/1360144X.2011.568690.
- Bovill, C., A. Cook-Sather, P. Felten, L. Millard, and N. Moore-Cherry. 2016. “Addressing Potential Challenges in co-Creating Learning and Teaching: Overcoming Resistance, Navigating Institutional Norms and Ensuring Inclusivity in Student–Staff Partnerships.” Higher Education 71 (2): 195–208. https://doi.org/10.1007/s10734-015-9896-4.
- Brooman, S., S. Darwent, and A. Pimor. 2015. “The Student Voice in Higher Education Curriculum Design: Is There Value in Listening?” Innovations in Education and Teaching International 52 (6): 663–674. https://doi.org/10.1080/14703297.2014.910128.
- Christie, M., and E. De Graaff. 2017. “The Philosophical and Pedagogical Underpinnings of Active Learning in Engineering Education.” European Journal of Engineering Education 42 (1): 5–16. https://doi.org/10.1080/03043797.2016.1254160.
- Cober, R., E. Tan, J. Slotta, H. J. So, and K. D. Könings. 2015. “Teachers as Participatory Designers: Two Case Studies with Technology-Enhanced Learning Environments.” Instructional Science 43 (2): 203–228. https://doi.org/10.1007/s11251-014-9339-0.
- Cook-Sather, A. 2011. “Layered Learning: Student Consultants Deepening Classroom and Life Lessons.” Educational Action Research 19 (1): 41–57. https://doi.org/10.1080/09650792.2011.547680.
- Cook-Sather, A. 2014. “Multiplying Perspectives and Improving Practice: What Can Happen When Undergraduate Students Collaborate with College Faculty to Explore Teaching and Learning.” Instructional Science 42 (1): 31–46. https://doi.org/10.1007/s11251-013-9292-3.
- Coşkun, S., Y. Kayıkcı, and E. Gençay. 2019. “Adapting Engineering Education to Industry 4.0 Vision.” Technologies 7 (1): 10. https://doi.org/10.3390/technologies7010010.
- Crawley, E. F., J. Malmqvist, S. Östlund, D. R. Brodeur, and K. Edström. 2014. “The CDIO Approach.” In Rethinking Engineering Education, edited by Sören Östlund and Kristina Edström, 11–45. Cham: Springer.
- Creswell, J. W. 2012. Educational Research: Planning, Conducting, and Evaluating Quantitative and Qualitative Research. Boston: Pearson Education, Inc.
- Davis, B., and D. Sumara. 2002. “Constructivist Discourses and the Field of Education: Problems and Possibilites.” Educational Theory 52 (4): 409. https://doi.org/10.1111/j.1741-5446.2002.00409.x.
- Dymova, L., P. Sevastjanov, and A. Tikhonenko. 2013. “A Direct Interval Extension of TOPSIS Method.” Expert Systems with Applications 40 (12): 4841–4847. https://doi.org/10.1016/j.eswa.2013.02.022.
- Edström, K., J. Törnevik, M. Engström, and Å Wiklund. 2003. “Student Involvement in Principled Change: Understanding the Student Experience.” In Proceedings of the 11th International Symposium Improving Student Learning, OCSLD, Oxford, England.
- Erpolat Taşabat, S. 2019. “A Novel Multicriteria Decision-Making Method Based on Distance, Similarity, and Correlation: DSC TOPSIS.” Mathematical Problems in Engineering 2019: 1–20.
- Ginsberg, M. B., and R. J. Wlodkowski. 2009. Diversity and Motivation: Culturally Responsive Teaching in College. San Francisco: John Wiley & Sons.
- Gulati, R., and T. Soni. 2015. “Digitization: A Strategic key to Business.” Journal of Advances in Business Management 1 (2): 60–67. https://doi.org/10.14260/jadbm/2015/8.
- Hadgraft, R. G., and A. Kolmos. 2020. “Emerging Learning Environments in Engineering Education.” Australasian Journal of Engineering Education 25 (1): 3–16. https://doi.org/10.1080/22054952.2020.1713522.
- Hwang, C. L., and K. Yoon. 1981. “Methods for Multiple Attribute Decision Making.” In Multiple Attribute Decision Making, edited by M. Beckmann and H. P. Künzi, 58–191. Berlin, Heidelberg: Springer.
- Kamvysi, K., K. Gotzamani, A. Andronikidis, and A. C. Georgiou. 2014. “Capturing and Prioritizing Students’ Requirements for Course Design by Embedding Fuzzy-AHP and Linear Programming in QFD.” European Journal of Operational Research 237 (3): 1083–1094. https://doi.org/10.1016/j.ejor.2014.02.042.
- Könings, K. D., C. Bovill, and P. Woolner. 2017. “Towards an Interdisciplinary Model of Practice for Participatory Building Design in Education.” European Journal of Education 52 (3): 306–317. https://doi.org/10.1111/ejed.12230.
- Könings, K. D., and S. McKenney. 2017. “Participatory Design of (Built) Learning Environments.” European Journal of Education 52 (3): 247–252. https://doi.org/10.1111/ejed.12232.
- Könings, K. D., T. Seidel, and J. J. van Merriënboer. 2014. “Participatory Design of Learning Environments: Integrating Perspectives of Students, Teachers, and Designers.” Instructional Science 42 (1): 1–9. https://doi.org/10.1007/s11251-013-9305-2.
- Krathwohl, D. R. 2002. “A Revision of Bloom's Taxonomy: An Overview.” Theory Into Practice 41 (4): 212–218. https://doi.org/10.1207/s15430421tip4104_2.
- Kuh, G. D., J. Kinzie, J. H. Schuh, and E. J. Whitt. 2011. Student Success in College: Creating Conditions That Matter. San Francisco: John Wiley & Sons.
- Li, G., C. Yuan, S. Kamarthi, M. Moghaddam, and X. Jin. 2021. “Data Science Skills and Domain Knowledge Requirements in the Manufacturing Industry: A gap Analysis.” Journal of Manufacturing Systems 60: 692–706. https://doi.org/10.1016/j.jmsy.2021.07.007.
- Liu, S. F., Y. L. Lee, Y. Z. Lin, and C. F. Tseng. 2013. “Applying Quality Function Deployment in Industrial Design Curriculum Planning.” International Journal of Technology and Design Education 23 (4): 1147–1160. https://doi.org/10.1007/s10798-012-9228-2.
- Lizzio, A., K. Wilson, and R. Simons. 2002. “University Students’ Perceptions of the Learning Environment and Academic Outcomes: Implications for Theory and Practice.” Studies in Higher Education 27 (1): 27–52. https://doi.org/10.1080/03075070120099359.
- Lorenz, M., M. Rüßmann, R. Strack, K. L. Lueth, and M. Bolle. 2015. Man and Machine in Industry 4.0: How Will Technology Transform the Industrial Workforce Through 2025. The Boston Consulting Group, 2.
- Malmqvist, J., M. Knutson Wedel, and M. Enelund. 2011. “Constructive Alignment (CA) for Degree Projects-Intended Learning Outcomes, Teaching and Assessment.” In Proceedings of 7th International CDIO Conference, Copenhagen, Denmark.
- Martens, S. E., S. N. Meeuwissen, D. H. Dolmans, C. Bovill, and K. D. Könings. 2019. “Student Participation in the Design of Learning and Teaching: Disentangling the Terminology and Approaches.” Medical Teacher 41: 1203–1205. https://doi.org/10.1080/0142159X.2019.1615610.
- McKenney, S., and T. C. Reeves. 2018. Conducting Educational Design Research. London: Routledge.
- Meyers, N. M., and D. D. Nulty. 2009. “How to use (Five) Curriculum Design Principles to Align Authentic Learning Environments, Assessment, Students’ Approaches to Thinking and Learning Outcomes.” Assessment & Evaluation in Higher Education 34 (5): 565–577. https://doi.org/10.1080/02602930802226502.
- Ochieng, P. A. 2009. “An Analysis of the Strengths and Limitation of Qualitative and Quantitative Research Paradigms.” Problems of Education in the 21st Century 13: 13.
- Olson, D. L. 2004. “Comparison of Weights in TOPSIS Models.” Mathematical and Computer Modelling 40 (7-8): 721–727. https://doi.org/10.1016/j.mcm.2004.10.003.
- Ryan, A., and D. Tilbury. 2013. Flexible Pedagogies: New Pedagogical Ideas. London.: Higher Education Academy.
- Seale, J. 2009. “Doing Student Voice Work in Higher Education: An Exploration of the Value of Participatory Methods.” British Educational Research Journal 36 (6): 995–1015. https://doi.org/10.1080/01411920903342038.
- Shih, H. S., H. J. Shyur, and E. S. Lee. 2007. “An Extension of TOPSIS for Group Decision Making.” Mathematical and Computer Modelling 45 (7–8): 801–813. https://doi.org/10.1016/j.mcm.2006.03.023.
- Skoogh, A., B. Johansson, and E. J. Williams. 2012, December. “Constructive Alignment in Simulation Education.” In Proceedings of the 2012 Winter Simulation Conference (WSC), 1–11. IEEE.
- Thanassoulis, E., P. K. Dey, K. Petridis, I. Goniadis, and A. C. Georgiou. 2017. “Evaluating Higher Education Teaching Performance Using Combined Analytic Hierarchy Process and Data Envelopment Analysis.” Journal of the Operational Research Society 68 (4): 431–445. https://doi.org/10.1057/s41274-016-0165-4.
- Triantaphyllou, E. 2000. “Multi-criteria Decision Making Methods.” In Multi-Criteria Decision Making Methods: A Comparative Study, edited by Panos M. Pardalos and Donald Hearn, 5–21. Boston, MA: Springer.
- Trigwell, K., and M. Prosser. 1991. “Improving the Quality of Student Learning: The Influence of Learning Context and Student Approaches to Learning on Learning Outcomes.” Higher Education 22 (3): 251–266. https://doi.org/10.1007/BF00132290.
- Tsinidou, M., V. Gerogiannis, and P. Fitsilis. 2010. “Evaluation of the Factors That Determine Quality in Higher Education: An Empirical Study.” Quality Assurance in Education 18 (3): 227–244.
- Tzeng, G. H., and J. J. Huang. 2011. Multiple Attribute Decision Making: Methods and Applications. Boca Raton: CRC press.
- Vanfretti, L., and M. Farrokhabadi. 2015. “Consensus-based Course Design and Implementation of Constructive Alignment Theory in a Power System Analysis Course.” European Journal of Engineering Education 40 (2): 206–221. https://doi.org/10.1080/03043797.2014.944101.
- Velasquez, M., and P. T. Hester. 2013. “An Analysis of Multi-Criteria Decision Making Methods.” International Journal of Operations Research 10 (2): 56–66.
- Wang, X., Y. Su, S. Cheung, E. Wong, and T. Kwong. 2013. “An Exploration of Biggs’ Constructive Alignment in Course Design and its Impact on Students’ Learning Approaches.” Assessment & Evaluation in Higher Education 38 (4): 477–491. https://doi.org/10.1080/02602938.2012.658018.
- Wee, D., R. Kelly, J. Cattel, and M. Breunig. 2015. “Industry 4.0-how to Navigate Digitization of the Manufacturing Sector.” McKinsey & Company 58: 7–11.
- Wirth, R., and J. Hipp. 2000, April. “CRISP-DM: Towards a Standard Process Model for Data Mining.” In Proceedings of the 4th International Conference on the Practical Applications of Knowledge Discovery and Data Mining, edited by Rakesh Agrawal and Paul Stolorz, 29–39. London, UK: Springer-Verlag.
- Wolf-Wendel, L., K. Ward, and J. Kinzie. 2009. “A Tangled Web of Terms: The Overlap and Unique Contribution of Involvement, Engagement, and Integration to Understanding College Student Success.” Journal of College Student Development 50 (4): 407–428. https://doi.org/10.1353/csd.0.0077.
- Wood, P., and W. Cajkler. 2016. “A Participatory Approach to Lesson Study in Higher Education.” International Journal for Lesson and Learning Studies 5 (1): 4–18. https://doi.org/10.1108/IJLLS-08-2015-0027.
- Yüksel, F. Ş., A. N. Kayadelen, and F. Antmen. 2023. “A Systematic Literature Review on Multi-Criteria Decision Making in Higher Education.” International Journal of Assessment Tools in Education 10 (1): 12–28. https://doi.org/10.21449/ijate.1104005.
- Zavadskas, E. K., Z. Turskis, and S. Kildienė. 2014. “State of art Surveys of Overviews on MCDM/MADM Methods.” Technological and Economic Development of Economy 20 (1): 165–179. https://doi.org/10.3846/20294913.2014.892037.
- Zopounidis, C., and M. Doumpos. 2002. “Multicriteria Classification and Sorting Methods: A Literature Review.” European Journal of Operational Research 138 (2): 229–246. https://doi.org/10.1016/S0377-2217(01)00243-0.
Appendices
Appendix A – The designed matrix for the evaluation of the ILOs criteria
Appendix B – The designed matrix for the evaluation of the identified ILOs of the course