ABSTRACT
Students’ learning environments often change during school career, due to school transitions and the introduction of educational innovations, causing discontinuity in teaching and learning. Success of students entering a new learning environment depends in part on their prior expectations of education, as these influence later perceptions. Heterogeneity between students, however, needs to be acknowledged. In seeking to determine expectation profiles and their potential linkage to divergent perceptions of education, we longitudinally surveyed secondary school students regarding their expectations before, and their perceptions one and two years after entering an innovative learning environment. We identified three expectation profiles that differed in terms of students’ motivation, learning conceptions, and affective strategies: optimistic, moderately optimistic, and pessimistic. For each profile, perceptions developed differently over time, with the moderately optimistic and pessimistic students being at risk in different ways. Results imply that expectations deserve a more prominent place when designing innovative learning environments.
Introduction
Do you know what?’ Pippi said. ‘It was awfully fun jolly to come to school to find out what it was like, but I don’t think I care about going to school any more … . It makes me dizzy in the head. I hope that you, teacher, won’t be sorry. (Lindgren Citation1999).
This quote about Pippi Longstocking’s disappointing first experiences at school nicely illustrates what can happen if students’ experiences of education do not live up to their expectations. During their school career learners are confronted with several changes in their learning environment for instance, when they transition to a new school (Rodrigues et al., Citation2018) or take part in educational innovations implemented in their current school. Before entering a new learning environment, students form expectations of the reuired effort and the content of the programme (Eegdeman, van Klaveren, and Meeter (Citation2020). Student expectations have been described as their ideas about what the new learning environment will be like and how they should behave in it (Maloshonok and Terentev Citation2017). Discontinuity in learning and teaching challenges student learning processes (Bormann and Thies Citation2019; Jackson et al. Citation2000; Keane et al. Citation2022) and has negative consequences for the majority of students (Brahm, Jenert, and Wagner Citation2017). Among other consequences, students can become disengaged, stressed, or drop out (Byrne and Flood Citation2005; Cooke, Sims, and Peyrefitte Citation1995; Eccles and Wigfield Citation2002; Rossi, Krouse, and Klein Citation2021; Wigfield and Eccles Citation2000).
To support students while entering a new learning environment, the impact of student expectations and the level to which these are met, needs to be acknowledged. Efforts could be directed towards better student preparation for the new learning environment. Also, teachers could account for student expectations (Overman et al. Citation2019). However, teachers need support to better understand their students and help them adjust to the new learning environment, while diversity in student expectations is not yet understood in the literature (Cole, Kennedy, and Ben-Avie Citation2009). Thinking about valuable interventions is hard when we do not know how students differ in their expectations and how this impacts students’ perceptions of learning during a period of entering a new learning environment. Therefore, the aim of the present study is to explore the existence of subgroups of students sharing similar expectations of a new learning environment. Subsequently we aim to find out how students of these subgroups longitudinally develop regarding their perceptions of the learning environment after entering the new learning environment.
Expectations of a new learning environment
Student expectations of how an upcoming learning environment will look like can have positive and detrimental effects on learning in all stages of the school career. When expectations are not met, learners experience difficulties in adjusting to a new learning environment (Jackson et al. Citation2000; Pancer et al. Citation2000) and have lower levels of satisfaction, engagement, and affective commitment that may drive dropout rates (Byrne and Flood Citation2005; Cooke, Sims, and Peyrefitte Citation1995). Unmet expectations can negatively influence achievement (McGhie Citation2017). This has been shown in the context of transitions between different schools, but the introduction of innovative instructional methods within a curriculum, too, changes students’ learning environment. Both situations can be seen as “turning points or as a period between two periods of stability” (Anderson et al. Citation2011, p. 30 in Coertjens et al. Citation2017). Students, thus, encounter even more transitions during their school career than only when changing schools. There are indications that student expectations likely influence how well they will adjust to a new way of teaching and learning as well (Appleton-Knapp and Krentler Citation2006), but few researchers have studied the impact of student expectations and perceptions in such intra-school transitions.
Expectations in longitudinal perspective
Students’ expectations of a new learning environment matter, because they influence perceptions of that new environment and ultimately behaviours. Expectations influence and bias later perception processes by influencing information-gathering processes and causing selective perception (Olson, Roese, and Zanna Citation1996). They affect the interpretation of incoming information, in turn, biasing subsequent behaviour, as people are likely to behave in a manner that is consistent with their expectations and thereby often create what they expect (Olson, Roese, and Zanna Citation1996). According to the expectancy-value theory, students who expect their new learning environment to be in line with their ideas about what is desirable in education will feel relatively confident and good-tempered, and be more motivated as a result. The expectancy-value theory states that the interplay between expectations and personal values impact different aspects of student behaviour, such as effort, persistence, and performance (Eccles and Wigfield Citation2002; Wigfield and Eccles Citation2000). Those students expecting a mismatch may feel doubtful and bad-tempered, especially if they feel they have limited control over their learning (Carver and Scheier Citation2001). These students may become less motivated and engaged, although it is not yet known if the impact of such mismatch is similar for all students.
According to the non-linear dynamic model (Carver and Scheier Citation2001), the extent to which students are engaged, motivated, and invest efforts depends on their “recent history” regarding their expecations (and the match with personal values). This means that when expectations and engagement are high while students encounter a new learning environment that tempers this optimism, engagement slowly decreases until at a certain point an abrupt drop in motivation and engagement occurs. When, by contrast, expectations and engagement are low and students face situations that encourage optimism, engagement and motivation gradually increases up to a point where a sudden rise can be expected. To be able to understand student expectations and their impact on perceptions better, it is vital that we acknowledge this diversity and try to develop a nuanced understanding of the development of student expectations.
Individual differences in student expectations
Although, researchers have acknowledged that there is much variation in students’ expectations, many studies have investigated this variance in student expectations by searching for linear relationships (Appleton-Knapp and Krentler Citation2006; Byrne and Flood Citation2005; Waters, Lester, and Cross Citation2014), which could result in an oversimplified picture. Students are not a homogeneous whole and heterogeneity in student properties should be acknowledged (Feinstein and Peck Citation2008; Seidel Citation2006; Vanthournout et al. Citation2013) when analysing the data.
In an attempt to address heterogenity, the expectancy-disconfirmation paradigm makes a conceptual, a priori distinction between 1) students whose perceptions are in agreement with expectations (zero disconfirmation); 2) students whose perceptions exceed expectations (positive disconfirmation); and 3) students whose perceptions do not live up to their expectations (negative disconfirmation) (Appleton-Knapp and Krentler Citation2006). Although offering a way to categorise students in subgroups, this paradigm is not data-driven. Grounding clusters or profiles in empirical research data has been suggested to provide a more detailed understanding of student characteristics and learning behaviours (Vanthournout et al. Citation2013). The aim is, then, is to identify “complex but meaningful patterns of commonality among people, not in terms of the average person or effects, but in terms of coherent, distinct, and relatively homogenous subgroups of people” (Feinstein and Peck Citation2008, 1).
In the current study we will identify expectation profiles or subgroups of secondary education students who have similar expectations of a soon-to-be-introduced innovative learning environment, based on profiles identified in the data. Based on this data-driven grouping of students, we aimed to investigate how students’ perceptions of that environment evolved for the different subgroups after they entered the new learning environment. To this end, we conducted a longitudinal study spanning three school years. Student expectations and perceptions were concerned with the general characteristics of powerful learning environments that promote the acquisition of high-quality knowledge, problem-solving skills, self-directed learning skills, and transfer of knowledge and skills (f.e., De Corte et al. Citation2003). Drawing on earlier research (Appleton-Knapp and Krentler Citation2006; Holbrook et al. Citation2014), we hypothesised that we would identify at least a positive/optimistic and a negative/pessimistic profile.
For ultimately being able to account for differences between students having different expectations, we first also need to be able to recognise those students. To better understand the diversity between subgroups of students, we described them in terms of the learning-related student characteristics that were typical of each profile and that are known to be related to both environmental factors as well as learning outcomes (Vermunt and Donche Citation2017). Vermunt and Vermetten (Citation2004) identified five such characteristics, specifically 1) cognitive processing strategies, or the cognitive activities that students undertake to process the content to be learned; 2) regulation strategies, or students’ approaches to regulating and steering their own learning; 3) learning orientations, or students’ personal goals or motives for learning and going to school; 4) students’ conceptions of learning; and 5) affective processing strategies, or students’ emotional and affective states that influence learning processes. Moreover, research has demonstrated that students’ learning approaches (Coertjens et al., Citation2017; Donche, Coertjens, and Van Petegem Citation2010; Vanthournout et al. Citation2013), as well as their motivation (Brahm, Jenert, and Wagner Citation2017; Kyndt et al. Citation2015; Otis, Grouzet, and Pelletier Citation2005) and learning conceptions (Rodriguez and Cano Citation2007) develop over time. Building on these insights, we hypothesise these learning-related student characteristics to impact how students expect and experience the transition to a new learning environment. synthesises the conceptual framework proposed in this study.
Figure 1. Visualisation of the variables involved in the study.
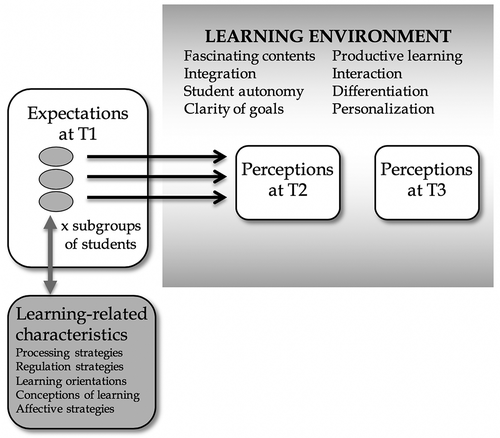
To recap, this paper seeks to identify students’ expectation profiles before, and related perception patterns after entering a curriculum innovation and to compare longitudinal expectation-perception patterns across those subgroups. The following research questions will be addressed:
1a. Which expectation profiles can be identified in the context of students entering a new learning environment, based on student expectations with respect to eight characteristics of the learning environment, being fascinating contents, productive learning, integration, student autonomy, interaction, differentiation, clarity of goals, and personalisation?
1b. How do these expectation profiles differ regarding learning-related student characteristics (including cognitive processing strategies, regulation strategies, learning orientations, conceptions of learning, and affective processing strategies) as measured before entering the learning environment as well as in the years thereafter?
2. How does the longitudinal development of student expectations and their later perceptions of each of the characteristics of learning environment differ across the distinct expectation profiles?
Method
Study context
The study was set in the context of secondary education in the Netherlands, where a curriculum innovation for all subject matters was being introduced nationwide with the aim to promote the acquisition of knowledge and skills that prepare students better for tertiary education. Students started in this curriculum innovation when entering in the 10th grade. This enabled us to study student expectations in 9th graders, before entering the new learning environment and follow-up their perceptions in the 2 years afterwards. In this learning environment, students are encouraged to self-direct their learning and to collaborate with peers, while the teacher acts like a coach, encouraging interaction between students and teacher. The environment accommodates individual differences, uses updated and broadened learning contents, and emphasises coherence between knowledge and skills and the application of knowledge in subject-matter domains.
Participants
Participants were students from five Dutch secondary schools that either prepared students for higher professional education in a 5-year programme (senior general education) or for university in a 6-year programme (pre-university education). The schools participated with all their students in 9th grade, which is the equivalent of 3rd-year secondary school in the Netherlands. We surveyed them at three points in time (T): before they were about to participate in a curriculum innovation (T1), 1 year after the introduction of this new learning environment (T2), and another year later, when students had 2 years of experience (T3). At T1, the sample consisted of 842 students (see for demographic information for the sample). At T2, the sample had decreased in size to 727 10th grade students, likely because of incidental absence of students and because some of them had not been promoted to the 10th grade. At T3, one school refrained from further participation, leaving a sample of 433 11th grade students from four schools.
Table 1. Background information of participants at T1.
Measures
Assessment of students’ expectations and perceptions
To measure students’ expectations and perceptions of the learning environment, we administered two parallel questionnaires, specifically: the Inventory of Expected Study Environment-Extended (IESEE) and the Inventory of Perceived Study Environment - Extended (IPSEE) (Könings et al. Citation2008). Both questionnaires consist of 67 items and cover eight scales that reflect characteristics of a contemporary education: 1) fascinating contents, with items about the extent to which the learning contents are interesting, challenging, and personally relevant; 2) productive learning, referring to little emphasis on the sole reproduction of learning contents; 3) integration, not only the integration of new and prior knowledge, but also of different knowledge domains and of knowledge and skills; 4) student autonomy, denoting the extent of agency in terms of deciding on the learning content, learning method, and time planning; 5) interaction, such as collaboration with peers and interaction with the teacher; 6) differentiation, inquiring about opportunities to choose and make different tasks, solve problems in different ways, and use different learning materials; 7) clarity of goals, covering items about the clarity of instructional goals and task demands; and 8) personalisation, addressing the availability of teacher support.
presents a sample item for each scale and the internal consistencies of the IESEE and IPSEE. For each item students had to indicate its expectation (“I expect this to happen” – IESEE) or presence (“this is happening” – IPSEE). Statements were rated on a 6-point rating scale, ranging from totally disagree (l) to totally agree (6). Cronbach’s alpha coefficients of the scales varied between .66 and .85.
Table 2. Internal consistencies of the IESEE at T1, and the IPSEE at T2 and T3.
Assessment of learning-related student characteristics
To measure the learning-related student characteristics, we administered the Inventory of Learning Styles for Secondary Education (ILS-SE; Vermunt, Bouhuijs, and Picarelli Citation2003). The questionnaire comprises 100 items in five clusters that refer to five learning-related student characteristics and are each subdivided into several scales. Items were rated on a 5-point scale, indicating the degree to which the statement reflected students’ own learning (from 1 = never/totally disagree to 5 = always/totally agree). presents the reliability coefficients of all scales at each point of measurement.
Table 3. Internal consistencies of the ILS-SE scales at T1, T2, and T3.
Procedure
We invited all students who were present at school on the day of data collection to complete the paper-and-pencil questionnaires during regular school hours, which yielded a very high response rate. Before students completed the questionnaires, we orally informed them about the content and procedure of the study. Ethical approval was not sought for this study as an ethical review board did not yet exist at the university at the time of data collection. Students completed the IESEE and ILS-SE at T1, and the IPSEE and ILS-SE at T2 and T3. The IESEE and IPSEE took 30–40 min and the ILSE-SE 20–30 min to complete.
Data analysis
We performed Latent Class Analysis (LCA), using WINMIRA software (Von Davier Citation1999) on the data collected at T1 in order to identify homogeneous expectation profiles. Consequently, we performed analyses of variance to explore differences in learning-related characteristics across profiles and deepen our understanding of the profiles. We used Cohen’s d to indicate effect sizes, with a Cohen’s d of 0.2 to 0.3 denoting small effects, around 0.5 suggesting medium effects, and larger than 0.8 implying large effects (Cohen Citation1988).
Furthermore, we used repeated measures analyses to investigate whether the development of expectations and perceptions over time differed across profiles. Since we anticipated that the short-term effects (T1 – T2) of expectations would be larger than the long-term effects (T2 – T3), we ran two separate analyses: one comparing the T1 and T2 data and another one comparing the data pertaining to T2 and T3. The strength of the overall effects would likely have been underestimated if effects had been analysed at once.
Findings
Identification of student subgroups using latent class analysis
For defining student expectation profiles based on their expectation regarding eight charactertistics of the learning environment (research question 1a), we started with computing LCA solutions. To decide on the models’ goodness of fit of these different LCA solutions, we used the BIC and CAIC indices (Rost Citation2004) that compare the function of likelihood (Log L) of the data with the sample size (N) and the number of parameters to be estimated (k). Empirical distributions were generated using the bootstrapping method. The estimated parameters served as a basis for the re-simulated data sets. All 3- to 10-class solutions showed fits larger than zero and, thus, it can be assumed that the observed data and the model match (Von Davier Citation1999). In the next step, we compared the solutions’ BIC and CAIC indices. The solution with three classes showed the lowest BIC and CAIC indices and, therefore, fits the data best (Log L = −2962.08, k = 26, BIC = 6095.14, CAIC = 6121.14, Cressie-Read paemp. = .11, Pearson paemp. = .25). Additionally, the probability of expected class membership was satisfactory for this the three-class solution (.74 for class 1; .88 for class 2; .91 for class 3).
Interpretation of latent classes
For answering research question 1a, we identified three classes emerging from Latent Class Analysis: subgroup 1 (22.7% of the students; N = 163), subgroup 2 (49.0% of the students; N = 352), and subgroup 3 (28.3% of the students; N = 203), respectively. displays the mean expectation scores of each subgroup on the eight scales of the IESEE. The black line represents the expectations of students within subgroup 1. As can be inferred, these expectations were positive on all scales, and were even highly positive when it came to the integration of learning contents, student autonomy, interaction with peers, and differentiation in learning. Subgroup 2, which is presented by the grey line, is also characterised by high expectations, although expectations were low in the case of productive learning and differentiation. Finally, students in subgroup 3, presented by the dotted line, had low expectations of all scales. The only component of which they had moderately positive expectations was integration. Descriptive statistics for each subgroup can be found in .
Figure 2. Statistically expected student scores for the three subgroups, reflecting students’ expectations of each of the eight components of their future learning environment.
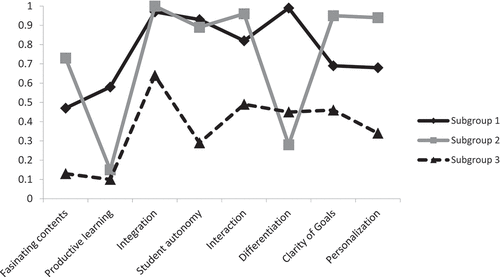
Table 4. Mean expectation scores (obtained at T1) and perception scores (obtained at T2 and T3) and related standard deviations, indicated for each subgroup separately.
Relating latent classes to learning-related student characteristics
For answering research question 1b on describing the differences between the subgroups regarding learning-related student charactertistics, we explored differences in these student characteristics across subgroups. What stood out was that students in subgroup 1 – when compared to peers in subgroup 2 – made less use of stepwise processing strategies, were less focused on the intake of knowledge, and tended to use less external regulation strategies (see left part of ). Compared to subgroup 3, they reported less lack of regulation strategies, and had a less ambivalent learning orientation, fewer motivation and concentration problems, and less fear of failure. Moreover, they were more oriented towards the construction of knowledge and used better strategies to keep a good state of mind than subgroup 3 (see right part of ). Subgroup 2 also reported less lack of regulation strategies, and had a less ambivalent learning orientation, fewer motivation and concentration problems, and less fear of failure than subgroup 3. Additionally, they were more personally interested in learning, had a higher vocation-oriented motivation for learning, they considered learning more as construction and use of knowledge, and had better strategies to keep a good state of mind than subgroup 3 (see bottom of ).
Figure 3. Substantiation of the three student subgroups at T1: Significant differences between learning-related student characteristics.
Note. For all analyses df = 2.
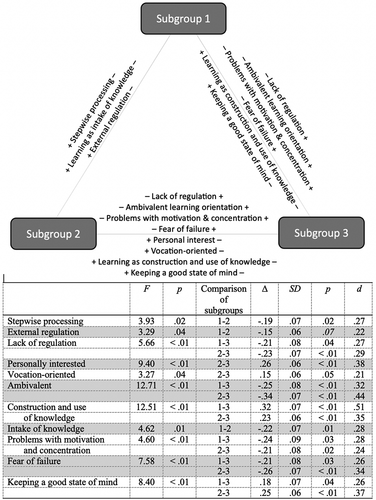
As the subgroups differed substantially in terms of associated learning-related student characteristics at T1, we additionally explored whether these differences continued to exist or had altered at T2 and T3. Repeated measures analyses on the ILS-SE scales yielded significant longitudinal effects or trends on four scales (p < .10). First, we found that motivation and concentration problems tended to increase more sharply between T1 and T2 in subgroup 1 than in subgroup 2 (FT1-T2 = 3.20, p = .04, Δ = .17, SD = .08, p = .08, d = .23) or subgroup 3 (Δ = .20, SD = .09, p = .06, d = .26). In the next year, however, these problems tended to decrease in subgroup 1, while they continued to rise steadily in subgroup 2 (FT2-T3 = 2.29, p = .10, Δ = .19, SD = .09, p = .10, d = .21) until at T3 the respective scores of both subgroups converged (see ). Furthermore, it showed that between T2 and T3 students in subgroup 2 increasingly used stepwise information processing strategies, while in subgroup 1 its use decreased (FT2-T3 = 3.07, p = .05, Δ = .20, SD = .08, p = .05, d = .32). And, lack of regulation tended to increase in subgroup 2, while it decreased in subgroup 3 (FT2-T3 = 2.33, p = .10, Δ = .21, SD = .10, p = .10, d = .27). Finally, while students in subgroup 2 tended to increasingly adopted an ambivalent motivational orientation towards learning, the opposite was true for their peers in subgroup 3 (FT2-T3 = 2.82, p = .06, Δ = .20, SD = .09, p = .08, d = .27).
Without having the pretention to ascribe absolute labels to students in different subgroups, there appeared to be a quite stable pattern in student characteristics. Students in the optimistic subgroup tend to have more constructivistic conceptions of learning, in combination with fewer motivational and regulation problems. They have a more meaning-directed learning style (Vermunt Citation1996; Vermunt and Verloop Citation1999) than the other subgroups. Therefore, this subgroup will be called the optimistic, meaning-directed subgroup in the rest of this paper. The most noticeable difference with the mixed optimistic profile is that these students are more reproduction-oriented and externally regulated. A more precise characterisation of this subgroup is the mixed optimistic, reproduction-directed subgroup. The students in the pessimistic subgroup differ from the other subgroups by displaying more problematic learning-related characteristics: More lack of regulation, motivational problems, and less constructivistic conceptions of learning. These characteristics fit into the undirected learning style. This subgroup will be called the pessimistic, undirected subgroup.
Longitudinal development of expectations and perceptions
To answer research question 2 on the longitudinal development of student expectations and their later perceptions across the distinct expectation profiles, we explored and compared expectation-perception patterns across subgroups as they evolved from T1 to T3 (see ). We found significant subgroup effects between T1 and T2 on all scales, and only one effect between T2 and T3 (see ). In the following, we will describe the post-hoc effects (i.e. comparisons between subgroups) with a medium or large effect size in more detail.
Table 5. Overview of the significant results of the ANOVAs, comparing the three subgroups in terms of the longitudinal differences between expectations at T1 and perceptions at T2 and between perceptions at T2 and T3.
We found five medium or large effects between expectations at T1 and perceptions at T2. First, the degree of negative disconfirmation on the fascinating contents scale was higher for optimistic, meaning-directed subgroup (d = .46) and 2 (d = .54) than for the pessimistic, undirected subgroup. Also on the productive learning scale, the optimistic, meaning-directed subgroup experienced greater negative disconfirmation than the pessimistic, undirected subgroup (d = .54). With regard to student autonomy, both the optimistic, meaning-directed and the mixed optimistic, reproduction-directed subgroups experienced more negative disconfirmation than the pessimistic, undirected subgroup (d = 1.08 and d = .53, respectively), and the optimistic, meaning-directed subgroup experienced a greater mismatch than mixed optimistic, reproduction-directed subgroup (d = .49). Fourth, the extent to which expectations of differentiation opportunities were not met was higher for the optimistic, meaning-directed subgroup than for (d = .45) and the mixed optimistic, reproduction-directed subgroup (d = .76) and 3 (d = .45). Finally, the mixed optimistic, reproduction-directed subgroup saw their expectations of clear goals more negatively disconfirmed than their peers in the optimistic, meaning-directed subgroup (d = .30).
The one effect we found between T2 and T3 concerned the student autonomy scale, where perceptions improved more strongly for the optimistic, meaning-directed subgroup than for the mixed optimistic, reproduction-directed subgroup (d = .36). As can be inferred from , longitudinal expectation-perception patterns (T1-T3) differed between subgroups, with the optimistic, meaning-directed subgroup showing a “recovery” after T2 to eventually reach similar scores as the mixed optimistic, reproduction-directed subgroup at T3.
In general, we observed some regularity in the direction of the effects over the scales. The scores of the optimistic, meaning-directed subgroup decreased more between T1 and T2 than the scores of the pessimistic, undirected subgroup (on five scales). Scores of students in the optimistic, meaning-directed subgroup decreased more than of those of students in the mixed optimistic, reproduction-directed subgroup on three scales (two medium/large sized effects and one small effect), while only one small effect pointed in the other direction, χ2(1) = .13, p < .01. Finally, scores of the mixed optimistic, reproduction-directed subgroup decreased more than of the pessimistic, undirected subgroup on six scales (three medium/large effects and three small effects) and decreased less on one scale (small effect), χ2(1) = .31, p < .01.
Discussion
In the present paper, we have sought to capture heterogeneity in students’ expectations and subsequent perceptions of a new learning environment and to explain expectation-perception patterns in terms of the profile-specific learning-related student characteristics. We identified three student subgroups, specifically: The optimistic, meaning-directed subgroup being the students with high expectations; the mixed optimistic, reproduction-directed subgroup consisting of students with (very) high expectations in combination with some low expectations; and the pessimistic, undirected subgroup being students with lowest expectations. Especially the distinction between the first two subgroups is noteworthy. The fact that the mixed optimistic, reproduction-directed subgroup had lower expectations of productive learning and differentiation can be explained by their learning-related characteristics. Since they considered learning more as intake of knowledge, used stepwise processing strategies, and were more externally regulated in their learning, a high emphasis on productive learning and differentiation would not have fitted in with their usual way of learning. Also longitudinally, students in the mixed optimistic, reproduction-directed subgroup differed from the others. While their use of stepwise information processing strategies increased, their lack of regulation got more severe and their motivation for learning became ever more ambivalent. Prior research has demonstrated that both motivation (Brahm, Jenert, and Wagner Citation2017) and deep learning (Coertjens et al. Citation2017) decrease after the transition from secondary to higher education. Our findings suggest that similar detrimental effects can occur when starting with innovative instructional methods within the curriculum.
Another important finding is that students in the optimistic, meaning-directed subgroup saw the largest increase in motivation and concentration difficulties after entering the new learning environment, but that they seem to have recovered from this setback after the first year. In the other subgroups, however, these problems were more persistent. The experience of students in the optimistic, meaning-directed subgroup might resemble what Cole (Citation2017) has coined “first-year stagnation”, describing a period of limited growth in motivation and deep learning approaches in the first year after a transition, followed by some sort of recovery in the year thereafter. The pessimistic, undirected students in our study already lacked appropriate regulation strategies and motivation from the start and we found no signs of recovery with respect to these characteristics in the years afterwards. This finding ties in with a study by Fryer (Citation2017) who found that students who entered university with low levels of deep processing strategies remained at low levels after the transition.
In terms of perceptions of the new learning environment, we saw that the optimistic, meaning-directed subgroup experienced the largest mismatches between expectations and perceptions, although they mostly still perceived the learning environment more positively than peers in the pessimistic-undirected subgroup. The combination with the found favourable learning-related characteristics in this subgroup (such as constructivist conceptions of learning, better regulation strategies, less fear of failure, and better skills to keep a good state of mind) may have helped them to deal with the new situation better. More positive perceptions on student autonomy and a decline in motivation difficulties after entering the new learning environment also indicate that students in the optimistic, meaning-directed subgroup had appropriate strategies to cope with the new environment and to adjust accordingly. By contrast, students in the mixed optimistic-reproduction-directed subgroup, who initially experienced less of a mismatch between expectations and perceptions, had more difficulties in adjusting over time and their perceptions did not get more positive over the years. The differences between the optimistic, meaning-directed subgroup and the mixed optimistic-reproduction-directed subgroup add nuance to Keane and colleagues’ findings that students with positive expectations perceive little mismatch after the transition (Keane et al. Citation2022) or Maloshonok and Terentev’s finding (Maloshonok and Terentev Citation2017) who concluded that mismatches between expectations and perceived reality cannot be predicted by personal background characteristics and motivation at the beginning. Our latent class analyses findings enable to investigate complex within-student interplay of expectations and differentiate between different subgroups of students with initial positive expectations (Kosel, Wolter, and Seidel Citation2021). The combination of the expectations held by students the optimistic and mixed-optimistic subgroup and their different learning-related student characteristics likely were related to the more succesfull longitudinal development in the optimistic, meaning-directed subgroup and the difficulties perceived by the mixed optimistic-reproduction-directed subgroup. Finally, low-expectation students in the pessimistic, undirected subgroup experienced the least mismatches, but their perceptions remained the most negative of all. As this subgroup had the most problematic learning-related characteristics, the fact that they experienced the best match between expectations and perceptions does not convince that they successfully made the transition, although this would have been hypothesised by the expectancy-disconfirmation paradigm (Appleton-Knapp and Krentler Citation2006; Holbrook et al. Citation2014).
The present study has theoretical implications in the light of existing knowledge regarding the expectancy-disconformation paradigm as well as the expectancy-value theory and the non-linear dynamical model. First, our findings add to the existing literature in that it moves beyond conceptual categorisations, such as those of the expectancy-disconfirmation paradigm that categorises students into students with zero disconfirmation, positive disconfirmation, and negative disconfirmation (Appleton-Knapp and Krentler Citation2006; Holbrook et al. Citation2014). By grounding our expectation profiles in empirical student data, we were able to identify two subgroups of students who might have experienced negative disconfirmation (subgroup 1 and 2), but who differed in terms of their learning-related characteristics and, consequently, indicated varying degrees of success in adjusting to the new environment: while the optimistic, meaning-directed subgroup experienced a first-year stagnation that they seemed to overcome in the second year, their peers in the mixed optimistic-reproduction-directed subgroup did not automatically seem to recover and appeared thus at risk to suffer from entering a new learning environment. The pessimistic, undirected subgroup appeared to have the most realistic expectations, and as such would fit the “zero disconfirmation” category (Appleton-Knapp and Krentler Citation2006; Holbrook et al. Citation2014). Although in theory this group would seem rather unproblematic or even ideal, the present study makes clear that these students might also be at risk when entering a new learning environment, because of their persistent negative view of the learning environment and the associated motivation and regulation problems.
Secondly, our findings add empirical evidence to expectancy-value theory and the non-linear dynamical model by Carver and Scheier (Citation2001) and give some support that it can be used to explain students’ expectation-perception patterns. As described in the expectancy-value theory we found that positive expecations related to high motivation, while negative expectations related to poor motivation and little investment of effort. For explaining our longitudinal findings and the differences between the subgroups, the non-linear dynamical model provides insights. This model postulates that students’ level of motivation and invested effort depends on their initial level of optimism and engagement. In our study, pessimistic students had low saw their expectations largely confirmed and their learning-related characteristics remained generally unfavourable throughout. In optimistic students, on the other hand, initial levels of optimism were high and although they might have experienced larger mismatches than others, they continued to have positive perceptions of the new environment and, based on a survey of their learning-related characteristics, did not present serious adjustment problems. The two aforementioned groups could therefore be positioned on the opposite ends of the non-linear dynamic model presented in . We would expect the mixed optimistic-reproduction-directed subgroup to be located more towards the centre of the model, since they entered the learning environment with tempered optimism, which, as the model predicts, could result in sudden drops in motivation and investment of effort if expectations are not met, which is precisely what we found.
Figure 6. Relative positions of the three subgroups in Carver and Scheier’s non-linear dynamic model (adapted from Carver and Scheier Citation2001).
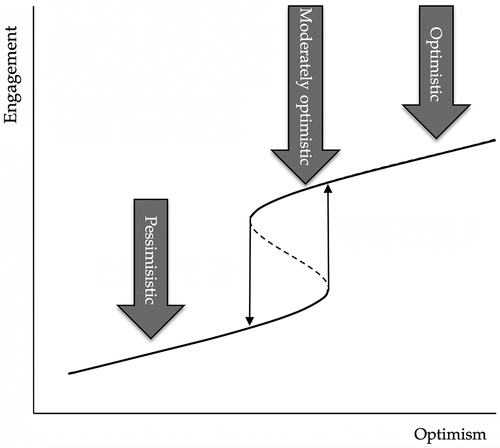
The present research also has several practical implications. First, the detection of different student subgroups can help teachers to identify groups of students who might be at risk to struggle when entering a new learning environment and, consequently, to adjust their teaching behaviour before and during the transition to the different student groups and their associated needs, as Cole, Kennedy, and Ben-Avie (Citation2009) already pointed out. More specifically, educational designers could profit from differentiating not only with regard to individual characteristics and class levels into account, but also from information regarding these student profiles (Seidel Citation2006). Students in the mixed optimistic-reproduction-directed subgroup, for instance, could be empowered if they were given a more proactive role and were encouraged to take more control over their learning. As a result, they could become active stakeholders in the teaching/learning process (Könings, Brand-Gruwel, and van Merriënboer Citation2010; Könings et al. Citation2021). Hence, they would benefit from participation in instructional design or redesign, as this will promote their sense of ownership, responsibility, and engagement, as well as metacognition and reflection (Könings, Brand-Gruwel, and van Merriënboer Citation2010; Könings et al. Citation2021). To boost pessimistic, undirected students, efforts could be channelled into improving students’ expectations before they enter the new learning environment (Rovers et al. Citation2018), as this will enhance student motivation and preparedness to monitor and adjust their learning according to the requirements of that new environment. Pessimistic students, too, would benefit from participatory design in terms of enhanced reflection and metacognition (Könings, Brand-Gruwel, and van Merriënboer Citation2011; Könings et al. Citation2021). Finally, transitions can be improved for students by aligning the two learning environments before and after the transition (Torenbeek, Jansen, and Hofman Citation2010).
Three limitations to this study should be addressed. First, it does not provide deeper insights that contribute to explaining the different ways students coped with the changed learning environment, nor does it offer evidence-based suggestions on how to best deal with the subgroups of students. We, therefore, welcome further research into the details of the adaptation process of students within the different subgroups, as well as the effects of student participation in the educational design process, of efforts to improve student expectations and to align learning environments on successful adjustment during an large change in the learning environment. Second, our findings might not be generalisable to all educational settings since the specific instructional characteristics of the educational context may have affected the content of student expectations. We therefore invite future researchers to compare different learning environments (constructivist vs. reproductive, for example) and investigate how students with different expectation profiles adapt to these environments. The relative importance of students’ level of optimism and a proactive learning approach on the effective adjustment to a new learning environment still has to be determined. To study the process of adjustment throughout such process, longitudinal research spanning several years is to be preferred to cross-sectional studies. Limitations like, dropout of participants and uncontrollable other influencing factors are difficult to prevent.
Conclusion
The present study provides positive evidence regarding the relevance of student expectations in the context of entering a new learning environment and innovative teaching methods. Not only in transitions between schools, but also when educational innovations are implemented do expectations play an important role for student learning. Our identification of different expectation profiles and associated learning-related student characteristics enhances understanding of the heterogeneity within a student cohort and of divergent perception patterns after entering the new environment. We strongly suggest that theory and practice no longer consider students as a homogeneous whole when it comes to their entry characteristics and ways of adjusting to a new environment. This study indicates that students in two out of three subgroups experience difficulties in different ways, underscoring the need for interventions that offer these students additional support.
Disclosure statement
No potential conflict of interest was reported by the author(s).
Additional information
Notes on contributors
Karen D. Könings
Karen D. Könings is an Associate Professor at the Department of Educational Design and Research at Maastricht University (The Netherlands) and an Honorary Professor at University of East Anglia (UK). Her research interest is in improving learning and instruction by better accounting for the different perspectives teachers and students have on education.
Tina Seidel
Tina Seidel is a Full Professor of Educational Psychology at the School of Education, Technical University of Munich (Germany). Her research interest is in teaching and teacher education research.
References
- Anderson, M., J. Goodman, and N. Schlossberg 2011. Counseling Adults in Transition: Linking Schlossberg’s Theory with Practice in a Diverse World. 4th. M. Anderson, J. Goodman, & N. Schlossberg New York, NY: Springer Publishing Company.
- Appleton-Knapp, S. L., and K. A. Krentler. 2006. “Measuring Student Expectations and Their Effects on Satisfaction: The Importance of Managing Student Expectations.” Journal of Marketing Education 28: 254–264. doi:10.1177/0273475306293359.
- Bormann, I., and B. Thies. 2019. “Trust and Trusting Practices During Transition to Higher Education: Introducing a Framework of Habitual Trust.” Educational Research 61 (2): 161–180. doi:10.1080/00131881.2019.1596036.
- Brahm, T., T. Jenert, and D. Wagner. 2017. “The Crucial First Year: A Longitudinal Study of Students’ Motivational Development at a Swiss Business School.” Higher Education 73: 459–478. doi:10.1007/s10734-016-0095-8.
- Byrne, M., and B. Flood. 2005. “A Study of Accounting Students’ Motives, Expectations and Preparedness for Higher Education.” Journal of Further and Higher Education 29 (2): 111–124. doi:10.1080/03098770500103176.
- Carver, C. S., and M. F. Scheier. 2001. “Optimism, Pessimism, and Self-Regulation.” In Optimism and Pessimism: Implications for Theory, Research, and Practice, edited by E. C. Chang, 31–51. Washington, DC: American Psychological Association.
- Coertjens, L., T. Brahm, C. Trautwein, and S. Lindblom-Ylänne. 2017. “Students’ Transition into Higher Education from an International Perspective.” Higher Education 73: 357–369. doi:10.1007/s10734-016-0092-y.
- Coertjens, L., V. Donche, S. De Maeyer, T. van Daal, and P. Van Petegem. 2017. “The Growth Trend in Learning Strategies During the Transition from Secondary to Higher Education in Flanders.” Higher Education 73 (3): 499–518. doi:10.1007/s10734-016-0093-x.
- Cohen, J. 1988. Statistical Power Analysis for the Behavioral Sciences. 2nd. Hillsdale: Erlbaum.
- Cole, J. S. 2017. “Concluding Comments About Student Transition to Higher Education.” Higher Education 73 (3): 539–551. doi:10.1007/s10734-016-0091-z.
- Cole, J. S., M. Kennedy, and M. Ben-Avie. 2009. “The Role of Precollege Data in Assessing and Understanding Student Engagement in College James.” New Directions for Institutional Research 14 (7): 1–11.
- Cooke, D. K., R. L. Sims, and J. Peyrefitte. 1995. “The Relationship Between Graduate Student Attitudes and Attrition.” The Journal of Psychology 129 (6): 677–688. doi:10.1080/00223980.1995.9914938.
- De Corte, E., L. Verschaffel, N. Entwistle, and J. J. G. van Merriënboer, edited by. 2003. Powerful Learning Environments: Unravelling Basic Components and Dimensions. Oxford, UK: Elsevier Science.
- Donche, V., L. Coertjens, and P. Van Petegem. 2010. “Learning Pattern Development Throughout Higher Education: A Longitudinal Study.” Learning and Individual Differences 20: 256–259. doi:10.1016/j.lindif.2010.02.002.
- Eccles, J. S., and A. Wigfield. 2002. “Motivational Beliefs, Values, and Goals.” Annual Review of Psychology 53: 109–132. doi:10.1146/annurev.psych.53.100901.135153.
- Eegdeman, I., C. van Klaveren, and M. Meeter. 2020. “Content Expectations and Dropout in Dutch Vocational Education.” Empirical Research in Vocational Education and Training 12 (1): 1–23. doi:10.1186/s40461-020-00096-7.
- Feinstein, L., and S. C. Peck. 2008. “Unexpected Pathways Through Education: Why Do Some Students Not Succeed in School and What Helps Others Beat the Odds?” The Journal of Social Issues 64: 1–20. doi:10.1111/j.1540-4560.2008.00545.x.
- Fryer, L. K. 2017. “(Latent) Transitions to Learning at University: A Latent Profile Transition Analysis of First-Year Japanese Students.” Higher Education 73 (3): 519–537. doi:10.1007/s10734-016-0094-9.
- Holbrook, A., K. Shaw, J. Scevak, S. Bourke, R. Cantwell, and J. Budd. 2014. “PhD Candidate Expectations: Exploring Mismatch with Experience.” International Journal of Doctoral Studies 9: 329–346. doi:10.28945/2078.
- Jackson, L. M., S. Pancer, M. W. Pratt, and B. E. Hunsberger. 2000. “Great Expectations: The Relation Between Expectancies and Adjustment During the Transition to University.” Journal of Applied Social Psychology 30 (10): 2100–2125. doi:10.1111/j.1559-1816.2000.tb02427.x.
- Keane, T., T. Linden, P. Hernandez-Martinez, A. Molnar, and A. Blicblau. 2022. “Digital Technologies: Students’ Expectations and Experiences During Their Transition from High School to University.” Education and Information Technologies 1–21. doi:10.1007/s10639-022-11184-4.
- Könings, K. D., S. Brand-Gruwel, and J. J. G. van Merriënboer. 2010. “An Approach to Participatory Instructional Design in Secondary Education: An Exploratory Study.” Educational Research 52 (1): 45–59. doi:10.1080/00131881003588204.
- Könings, K. D., S. Brand-Gruwel, and J. J. G. van Merriënboer. 2011. “Participatory Instructional Redesign by Students and Teachers in Secondary Education: Effects on Perceptions of Instruction.” Instructional Science 39 (5): 737–762. doi:10.1007/s11251-010-9152-3.
- Könings, K. D., S. Brand-Gruwel, J. J. G. van Merriënboer, and N. J. Broers. 2008. “Does a New Learning Environment Come Up to Students’ Expectations? A Longitudinal Study.” Journal of Educational Psychology 100 (3): 535–548. doi:10.1037/0022-0663.100.3.535.
- Könings, K. D., S. Mordang, F. Smeenk, L. Stassen, and S. Ramani. 2021. “Learner Involvement in the Co-Creation of Teaching and Learning.” Medical Teacher 43 (8): 924–936. doi:10.1080/0142159X.2020.1838464.
- Kosel, C., I. Wolter, and T. Seidel. 2021. “Profiling Secondary School Students in Mathematics and German Language Arts Using Learning-Relevant Cognitive and Motivational-Affective Characteristics.” Learning and Instruction 73: 101434: 1–15.
- Kyndt, E., L. Coertjens, T. Van Daal, V. Donche, D. Gijbels, and P. Van Petegem. 2015. “The Development of Students’ Motivation in the Transition from Secondary to Higher Education: A Longitudinal Study.” Learning and Individual Differences 39: 114–123. doi:10.1016/j.lindif.2015.03.001.
- Lindgren, A. 1999. Pippi Goes to School. London, England: The Viking Press, Inc.
- Maloshonok, N., and E. Terentev. 2017. “The Mismatch Between Student Educational Expectations and Realities: Prevalence, Causes, and Consequences.” European Journal of Higher Education 7 (4): 356–372. doi:10.1080/21568235.2017.1348238.
- McGhie, V. 2017. “Entering University Studies: Identifying Enabling Factors for a Successful Transition from School to University.” Higher Education 73 (3): 407–422. doi:10.1007/s10734-016-0100-2.
- Olson, J. M., N. J. Roese, and M. P. Zanna. 1996. “Expectancies.” In Social Psychology: Handbook of Basic Principles, edited by E. T. Higgins and A. W. Kruglanski, 211–238. New York: Guilford Press.
- Otis, N., F. M. E. Grouzet, and L. G. Pelletier. 2005. “Latent Motivational Change in an Academic Setting: A 3-Year Longitudinal Study.” Journal of Educational Psychology 97 (2): 170. doi:10.1037/0022-0663.97.2.170.
- Overman, M., J. D. Vermunt, P. C. Meijer, and M. Brekelmans. 2019. “Teacher–student Negotiations During Context‐based Chemistry Reform: A Case Study.” Journal of Research in Science Teaching 56 (6): 797–820. doi:10.1002/tea.21528.
- Pancer, S. M., B. Hunsberger, M. W. Pratt, and S. Alisat. 2000. “Cognitive Complexity of Expectations and Adjustment to University in the First Year.” Journal of Adolescent Research 15: 38–57. doi:10.1177/0743558400151003.
- Rodrigues, R. G., M. Meeuwisse, T. Notten, and S. E. Severiens. 2018. “Preparing to Transition to Secondary Education: Perceptions of Dutch Pupils with Migrant Backgrounds.” Educational Research 60: 2, 222–240. doi:10.1080/00131881.2018.1463144.
- Rodriguez, L., and F. Cano. 2007. “The Learning Approaches and Epistemological Beliefs of University Students: A Cross‐sectional and Longitudinal Study.” Studies in Higher Education 32 (5): 647–667. doi:10.1080/03075070701573807.
- Rossi, R. A., A. M. Krouse, and J. Klein. 2021. “Undergraduate Student Stress, Classroom Engagement, and Self-Directed Learning Postcurricular Revision.” The Journal of Nursing Education 60 (10): 566–569. doi:10.3928/01484834-20210729-03.
- Rost, J. 2004. Testtheorie Und Testkonstruktion [Test Theory and Test Construction]. Bern, Switzerland: Hans Huber.
- Rovers, S. F. E., G. Clarebout, H. H. C. M. Savelberg, and J. J. G. van Merriënboer. 2018. “Improving Student Expectations of Learning in a Problem-Based Environment.” Computers in Human Behavior 87: 416–423. doi:10.1016/j.chb.2018.02.016.
- Seidel, T. 2006. “The Role of Student Characteristics in Studying Micro Teaching–learning Environments.” Learning Environments Research 9 (3): 253–271. doi:10.1007/s10984-006-9012-x.
- Torenbeek, M., E. Jansen, and A. Hofman. 2010. “The Effect of the Fit Between Secondary and University Education on First-Year Student Achievement.” Studies in Higher Education 35 (6): 659–675. doi:10.1080/03075070903222625.
- Vanthournout, G., L. Coertjens, D. Gijbels, V. Donche, and P. Van Petegem. 2013. “Assessing Students’ Development in Learning Approaches According to Initial Learning Profiles: A Person-Oriented Perspective.” Studies in Educational Evaluation 39: 33–40. https://doi.org/10.1016/j.stueduc.2012.08.002
- Vermunt, J. D. 1996. “Metacognitive, Cognitive and Affective Aspects of Learning Styles and Strategies: A Phenomenographic Analysis.” Higher Education 31: 25–50.
- Vermunt, J. D., P. A. J. Bouhuijs, and A. Picarelli. 2003. Vragenlijst Leerstijlen Voor Het Voortgezet Onderwijs (VLS-VO)[Inventory of Learning Styles for Secondary Education (ILS-SE)]. The Netherlands: Maastricht University.
- Vermunt, J. D., and V. Donche. 2017. “A Learning Patterns Perspective on Student Learning: State of the Art and Moving Forward.” Educational Psychology Review 29: 269–299.
- Vermunt, J. D., and N. Verloop. 1999. “Congruence and Friction Between Learning and Teaching.” Learning and Instruction 9: 257–280.
- Vermunt, J. D., and Y. J. Vermetten. 2004. “Patterns in Student Learning: Relationships Between Learning Strategies, Conceptions of Learning, and Learning Orientations.” Educational Psychology Review 16: 359–384.
- Von Davier, M. 1999. Winmira—a Program System for Analyses with the Rasch-Model, with the Latent Class Analysis and with the Mixed-Rasch Model. Kiel: IPN.
- Waters, S. K., L. Lester, and D. Cross. 2014. “Transition to Secondary School: Expectation versus Experience.” Australian Journal of Education 58: 153–166.
- Wigfield, A., and J. S. Eccles. 2000. “Expectancy–value Theory of Achievement Motivation.” Contemporary Educational Psychology 25: 68–81.