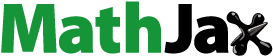
ABSTRACT
This paper contributes to the understanding of university spinoff (USO) development by analysing structural properties of their shareholder networks over time and across different regions. Theoretically, we propose a new stage-based typology of USO development across regions. Empirically, the study utilises a sample of 1033 academic spinoffs founded by 87 universities across 12 unitary regions in the UK considering the diversity of spatial contexts in the USO development. We undertake a social network analysis of relations USOs form with their parent universities and shareholders by adopting ‘betweenness centrality’ and ‘structural holes’ as two key measures. By employing this novel network-based view of firm development across regions, this study builds on the development model of USOs by identifying three key phases of USO development: (1) organisation phase, (2) exploitation phase, and (3) maturity and reorganisation phase. Second, we observe differences in USOs in terms of shareholder network development across diverse regional contexts. We propose a novel typology of entrepreneurial regions to better understand the diverse spatiality of USOs: peripheral lock-in, entrepreneurial periphery, rigid core, and entrepreneurial core. We call for further research to capture the long-term development and variable growth paths of USOs.
1. Introduction
University spin-off (USO) is a unique type of firm (Prokop Citation2021a) characterised by high levels of innovation (Bagchi-Sen, Baines, and Smith Citation2020), attraction of venture capital funding (Mueller, Westhead, and Wright Citation2012), and factors related to their survival (Prokop, Huggins, and Bristow Citation2019). While much of the research attention devoted to USOs tended to focus on their formation (e.g. Ramaciotti and Rizzo Citation2014), in recent years, there has been increasing interest in the performance of these ventures (Bolzani, Rasmussen, and Fini Citation2021), and the influence of spatial (Bagchi-Sen, Baines, and Smith Citation2020) and institutional contexts (Marzocchi, Kitagawa, and Sánchez-Barrioluengo Citation2019) on their activity and performance.
Networks with a range of actors can be clearly recognised as critical to a USO’s success (Vohora, Wright, and Lockett Citation2004). In particular, a USO’s network capability is significant for its growth, performance and sustainability (Walter, Auer, and Ritter Citation2006), suggesting that networks are equally important at every stage of the USO’s development. Although much of the USO literature stresses the role of networks (e.g. Bourelos, Magnusson, and McKelvey Citation2012), the majority of studies on the networks of USOs have centred around qualitative small-sample approaches (e.g. Vohora, Wright, and Lockett Citation2004) where networks are merely recognised as part of firm development. Consequently, the evolution of USOs has been left under-theorised.
This paper asks the following research questions: (1) how do USO shareholder networks evolve and what do they reveal about firm development? (2) Are the USO shareholder networks different across regions? In order to answer these research questions, we use a sample of 1033 USOs from 87 universities across 12 unitary regions in the UK. The paper undertakes social network analysis of relations USOs form with their parent universities and other shareholders to describe network positions of USOs at different firm development stages. We then examine whether USO shareholder networks show the same characteristics across different spatial contexts. Based on the analyses, a typology of regions is constructed to better understand the nature of the USO networks.
The following parts of the paper review the USO development and network literatures in section 2, outline the methodology in section 3, report and discuss findings in section 4, while section 5 concludes the article with policy recommendations.
2. USO development and networks
2.1. Networks and USOs
Networks are critical to understanding USOs in a university-centred environment, as indicated by much of the academic entrepreneurship literature (e.g. Hayter Citation2016a) highlighting the importance of networks developed as part of university support for USOs (Patzelt and Shepherd Citation2009). For example, networks are provided by Technology Transfer Office (TTO) professionals (Lockett, Wright, and Franklin Citation2003), especially those linked to venture capitalists, entrepreneurs (Franklin, Wright, and Lockett Citation2001), university alumni (Berggren Citation2011), senior management talent (Shane Citation2004), or other university TTOs (Lockett, Wright, and Franklin Citation2003). In particular, the UK universities that were more successful in developing USOs had greater ‘network capital’ (Huggins Citation2010) and existed in better connected university ecosystems (Prokop Citation2022), due to a more open-minded approach to technology transfer (Franklin, Wright, and Lockett Citation2001). This resonates with Roper and Hewitt-Dundas's (Citation2013) research that identified universities holding more central positions in networks. It is important to note that different types of universities pursue diverse entrepreneurial pathways and USO formation strategies reflect their own resource and capability limitations, but also their geographical focus (Sanchez-Barrioluengo, Kitagawa, and Uyarra Citation2019).
These networks accessible to USOs could be strongly related to their university’s reputation effect endowing USOs with credibility (Mueller, Westhead, and Wright Citation2012). Thus, the university’s internal characteristics, related to the volume of potential entrepreneurial opportunities, are interlinked with the university’s ability to equip a USO with more intangible characteristics crucial to its future success. Furthermore, Grandi and Grimaldi (Citation2003) point out that the first networks that USOs build are in fact inherited from their parent organisations, indicating how critical university resources are for these companies.
Networking allows firms to overcome the liability of newness (Chan, Oerlemans, and Pretorius Citation2010), build credibility (Bower Citation2003), access resources (Kitagawa and Robertson Citation2012), secure necessary funding and further opportunities for growth (Chan, Oerlemans, and Pretorius Citation2010) and survival (Prokop, Huggins, and Bristow Citation2019). In other words, USOs develop networks primarily to access resources otherwise not available to them (Grandi and Grimaldi Citation2003). This is, however, far from an exhaustive list of what networks bring to USOs. Vohora, Wright, and Lockett (Citation2004) stress that networks with industry and external entrepreneurs play a key role in opportunity recognition, at the very early stage of USO formation. This is further supported by Landry, Amara, and Rherrad (Citation2006), who found that the likelihood of USO formation is significantly influenced by the academic’s social capital.
Since proximity of firms induces networks, and networks lead to opportunities (Huggins Citation2008; Fini et al. Citation2011), it is clear that the spatial character of networks is critical. This importance of local social capital has played a fundamental role in the development of the high-tech cluster, exemplified by the cases in the UK such as Cambridgeshire (Garnsey and Heffernan Citation2005), and Oxfordshire’s biotechnology cluster with distinct entrepreneurial culture and local networks (Lawton Smith, Romeo, and Bagchi-Sen Citation2008). Similarly, studies in Sweden show that the successful development of USOs is linked to the continuing generations of networks developed by the USOs (Berggren and Lindholm Dahlstrand Citation2009), thus ensuring a continuous stream of knowledge-based entrepreneurship contributing to the local/regional economic development. These examples clearly indicate that evolutionary aspects play a central role in academic entrepreneurship considerations (Lawton Smith, Romeo, and Bagchi-Sen Citation2008), as networks are built over time (Siegel, Veugelers, and Wright Citation2007). Benneworth and Charles (Citation2005) go even further, describing the university as a central regional innovation agent, which could utilise USOs to diffuse knowledge in underperforming regions, transforming its innovative capability. Thus, USOs could play the role of regional knowledge brokers.
The crucial difference between the networks at different stages of development is found in its orientation, where they transition from academically- to more commercially-oriented networks (Pérez Pérez and Mártinez Sánchez Citation2003). This evolutionary aspect of inter-firm networks signifies that small firms switch from reliance on more personal-level social capital towards network capital, depicting inter-organisational connections of more calculative and deterministic character (Huggins Citation2010). It is critical to recognise that such connectedness transformation is not a uniform process across all USOs. In fact, USOs need to adapt their networks by building and restructuring their network position, aligning it with changing needs and expectations over time (Rasmussen, Mosey, and Wright Citation2015), especially as their strong network position is related to performance (Scholten et al. Citation2015). Furthermore, USO productivity is related to academic entrepreneurs having stronger links with external entrepreneurs or industry (Bourelos, Magnusson, and McKelvey Citation2012). Prokop, Huggins, and Bristow (Citation2019) in their study of UK USOs report that USOs’ survival is dependent on their shareholder network structure, with those holding less constrained positions characterised by lower failure rate. Networks clearly play a critical role in the development of USOs and require further exploration to build on the existing development models.
2.2. USO development
The USO growth model has been inspired by the original growth model of high-technology firms (Galbraith Citation1982) in general. However, the academic entrepreneurship literature so far has predominantly focused on the early stages of USO firm development, typically before the initial public offering (IPO) (Vohora, Wright, and Lockett Citation2004; Rasmussen Citation2011; Parmentola and Ferretti Citation2018). Consequently, the importance of long-term contribution of USOs to the economic development (Vincett Citation2010) has been under-represented in the literature. To better understand the USO performance, an examination of the USO development over time is critical, as each stage of development poses a different set of challenges for these ventures. For instance, ‘goal conflicts’ between venture capital and USOs constitute one of the challenges for early stage of USO development (Sandrom et al. Citation2016) as venture capitalists are less likely to invest in the starting phase of the USO (Wright et al. Citation2006).
Within the early stage, more specifically, four distinct phases of USO development have been identified (Ndonzuau, Pirnay, and Surlemont Citation2002; Shane Citation2004; Vohora, Wright, and Lockett Citation2004; Rasmussen Citation2011; Parmentola and Ferretti Citation2018): (1) ‘research’, providing a clear link of the to-be-developed intellectual property (IP) to the university it originated from; (2) ‘opportunity recognition’, in which theoretical IP is assessed against possible applied or commercial purposes; (3) ‘proof of concept’, in which the theoretical IP has been developed to a level where it can be proved to be a workable/practical IP; and (4) ‘start-up’, in which various core resources are accumulated/assembled to create a separate legal entity to develop the IP into a finished product or service and generate revenue.
Even the ‘late-stage’ models (e.g. Parmentola and Ferretti Citation2018) end immediately after the firm starts selling the product, breaks even or attracts major investment. One could argue that this is a point at which the firm, however inflated by venture capital investments, is still in its infancy as it has not achieved the required scale of operations (Bigdeli, Li, and Shi Citation2015) and therefore is still subject to a high degree of risk, where the company's status could change. Similarly, the Ndonzuau et al.’s (Citation2002) final stage of creating economic value is quite broadly defined that it could depict any moment in USO’s post formation existence. This is especially paradoxical when taking into account the studies that focus on a USO’s IPO (Bonardo, Paleari, and Vismara Citation2011), or those that point out that a USOs’ significant contribution to economic development starts after firms become publicly listed, as this is when they actually start to grow (Lawton Smith, Romeo, and Bagchi-Sen Citation2008). It is worth stressing, nevertheless, that many USOs do not even sell their products/services even after reaching the IPO stage, suggesting considerable heterogeneity (Siegel, Veugelers, and Wright Citation2007), and an increased difficulty in capturing their development in a single stage-based model.
Furthermore, while the models focus on the early stages until the USO firm is formed, they only account for a very short and intensive period of time in the life of a USO, leaving a vacuum between company formation and achieving Vohora et al.’s (Citation2004) ‘sustainability’, or ‘creation of economic value’, as per Ndonzuau, Pirnay, and Surlemont (Citation2002). There is a clear need to account for the longer term, especially as the dynamic growth period of USOs could take even 30 years (Vincett Citation2010) before levelling off. This means that for USOs created in the past 10–20 years (and more) the existing theoretical explanations of their development may reach their limits or even become redundant.
2.3. Conceptualising local and regional contexts
USOs are surrounded by specific contextual and institutional conditions facilitating the entrepreneurial climate of the region, underlying variable performance of these ventures across different spatial contexts (Prokop Citation2021b). Elements that pertain to institutions cannot be easily imitated (Braunerhjelm Citation2007). Local and regional contexts play a crucial role in USO support (Fini et al. Citation2011), as universities are immobile entities (Berbegal-Mirabent, Lafuente, and Solé Citation2013) and thus need to utilise the available regional resources and capabilities to their best advantage. Universities’ relationships with local economic growth are dynamic and change over time due to both internal and external conditions (Lawton Smith et al. Citation2014). Consequently, the performance of USOs across regions varies significantly.
Ramaciotti and Rizzo (Citation2014) found that USO formation is greater in Italian regions that have less innovative activity, suggesting a knowledge spillover role for these firms; yet USOs of higher quality (Fini et al. Citation2017) and characterised by greater performance (Iacobucci and Micozzi Citation2015) are found in more developed regions, reflecting the complexity involved in spatial aspects of academic entrepreneurship. Rasmussen (Citation2008) notes that actively engaged policymakers stimulate skills development among the TTO staff as well as encourage universities to experiment with new approaches and initiatives (515). Nevertheless, it is important not to lose sight of support available to avoid duplicating initiatives at a cost to the taxpayer: in places where regional policies stimulate entrepreneurship through a certain set of initiatives, e.g. provision of finance or tax incentives, universities focused on USOs should ideally invest their time in developing support initiatives not available at a regional level (Fini et al. Citation2011). As a result, it is important to understand what type of regional environments USOs occupy and how these relate to their development.
The role played by local industry structures and access to investments, funding and context-dependent entrepreneurial support mechanisms and other resources is further conceptualised in the literature on ‘entrepreneurial ecosystems’ that stresses the relevance of local entrepreneurial assets and networks for start-ups creation and growth (Stam Citation2015). Brown and Mason (Citation2017) suggest two types of such contextual characteristics: ‘embryonic’ and ‘scale-up’ ecosystems. The first describing ecosystems with low start-up activity, dependent on established businesses, locally-oriented, and building non-local connections only when necessary to access resources. In contrast, the ‘scale-up’ ecosystems are characterised by a dynamic venture formation activity focused on growth, where successful firms lead to entrepreneurial recycling, and firms integrated into wide-ranging networks of local, regional and international nature. As such, firms finding themselves in such radically different ecosystems may experience diverse growth patterns.
The difference in the characteristics of USOs at different stages of development affect their networks situated in different regional contexts. Based on the review of literature above, the paper aims to better understand how shareholder networks of USOs evolve and what they reveal about the firms and the entrepreneurial characteristics of their regional environments, specifically in terms of network resources available across regions. The remaining parts of this paper examine these networks empirically.
3. Research design and methods
3.1. Research context
The sample we employ comes from multiple sources to ensure cross-validation of data. First, we used www.spinoutsuk.co.uk to identify firms listed as USOs formed between 2000 and 2013. As the website lists USOs and other firms linked to universities (i.e. staff and graduate start-ups) we visited websites of all UK universities to validate the names and affiliations of USOs and collect further firms, especially those founded before the year 2000. Our next step involved a FAME (Financial Analysis Made Easy) database search to further confirm the relationship between universities and the firms and collect additional information on USOs, including firm demographics, and their shareholders. Resultantly, the study employs a sample framework of 1331 USOs established by 87 universities across 12 unitary regions in the UK. The data was captured in 2014 and includes USOs of varying ages, between 0 and 55 years with an average age of 9. Within the sample, 1033 firms were still live in May 2014, and these are the focus of our study.
The regional context of USOs in the UK presents a complex picture, as depicted in . There are twelve NUTS1 regions in the UK with varying characteristics. The regions characterised by higher levels of economic output with higher concentrations of venture capital firms – London and South East – perform quite well, though not extremely so in terms of USO formation and survival of USOs. In fact, we observe that the region with the highest number of USOs is Scotland, highest number of USOs averaged by the number of universities is Northern Ireland, whilst the greatest survival rate of USOs is in East Midlands. The participation of the USOs in the regional populations of firms is largest in Scotland, whilst well below the national average for regions such as London, East of England or even South East. However, little is known about the evolution of the USO networks in relation to the entrepreneurial characteristics of each of these regions. Specifically, the distinct character of USO companies is not captured particularly well within Brown and Mason’s (Citation2017) taxonomy, with Scotland and London sharing parts of both embryonic and scale-up ecosystems.
Table 1. Regional USO characteristics.
3.2. Methods
This study examines USOs from UK universities by adopting a network-based view, in particular observing the evolution of the USO networks, with their development in relation to diverse regional entrepreneurial characteristics. It undertakes social network analysis of relations USOs form with their parent universities and other shareholders, in total comprising of 12,960 actors. Our network boundary delineation is based on a realist approach (Laumann, Marsden, and Prensky Citation1992) – in this case based on membership of those who have ownership of spinoff companies. Such networks are composed of ties where actors acted on connections and formalised them, frequently by exchanging equity in company for support. Given their nature, they also represent ties of trust. Shareholder networks are not exhaustive of all connections spinoff companies have, however, they represent a significant portion of connections that brought benefits to USOs. These also comprise of the key actors that play important roles in spinoff companies (Prokop Citation2021a). Such delineation and focus on specific network construct is important in the analysis of social networks to uncover meaning (Mitchell Citation1969). It is important to highlight the use of shareholder data is common in the study of interorganisational networks (e.g. Li et al. Citation2016; Prokop, Huggins, and Bristow Citation2019).
Whilst several different variables could be used to describe network positions of USOs, we employ two key measures: ‘betweenness centrality’ (Freeman Citation1977) and ‘structural holes’ (Burt Citation1992) as the key measures used in many influencing studies (Huggins, Prokop, and Thompson Citation2020; Prokop, Huggins, and Bristow Citation2019).
Betweenness centrality (BC) is a measure of the extent to which an actor plays a role of a hub in a network, i.e. the
’s centrality is based on how many other actors in the network have to communicate with each other (or are linked) through
(Wasserman and Faust Citation1994). In other words, betweenness centrality measures the probability that the interaction between actor
and actor
will travel through actor
accounting for all possible connections within the network for actors
and
. Therefore, the betweenness centrality BC of actor
is defined here as:
where
is the number of shortest paths (i.e. geodesics) between actors
and
, whilst
is the probability that these paths include actor
(Freeman Citation1977). The sum of those probabilities for all network actors for actor
, provides an index of
’s betweenness centrality. It takes a minimum value of 0 when
is not part of any geodesics, whilst it reaches a maximum value when
is part of all geodesics of length greater than 1 (Freeman Citation1977). In order to standardise the index so that the measure takes values between 0 and 1 it is divided by the maximum value
being the number of pairs of actors excluding
(Freeman Citation1977; Wasserman and Faust Citation1994). Betweenness centrality indicates USO’s importance in a network (i.e. holding central or powerful position) (Borgatti, Everett, and Johnson Citation2018), given that it plays a mediating role between actors in a network, in essence being an information hub/broker. USOs with high levels of betweenness centrality are actors that mediate in greater information/resource transfer between network actors, making such USOs more powerful by the position held, information/resources transmitted, and its ability to disrupt such transmission (Borgatti, Everett, and Johnson Citation2018). Holding such a role may indicate credibility in a network where information/resource flow relies on an actor with higher betweenness centrality.
Structural holes (SH) measure the position of an actor dependent on the proportion of nonredundant ties in its network (Burt Citation1992), with
having a stronger position when
’s network includes more actors that are not connected to each other. The
’s relations become redundant from the moment actors
and
form a tie. The measurement of structural holes is expressed as a constraint
of
on
, with
being more constrained in a network characterised by a higher number of redundant ties (Burt Citation1992). As such
has limited (if any) opportunities to benefit from structural holes. We first denote aggregate constraint
of actor
as a sum of all constraint of
on
, where constraint
is specified as follows:
with:
where
stands for the proportion of relation values
from actors
to
, and
to
, divided by the sum of all relation values
of actor
(Burt Citation1992). The value of structural holes SH for actor
is simply:
Structural holes signal USO’s skilful exploitation of opportunities that enhance its success (Prokop, Huggins, and Bristow Citation2019). Since a structural hole depicts lack of a connection between two immediate actors of a USO, it awards the USO with opportunity to play one against the other (Burt Citation1992) and explore non-redundant information/resource (Borgatti, Everett, and Johnson Citation2018) – since disconnected actors have no way to share information/resources. In other words, structural holes measure the extent to which an actor is constrained within its most immediate network to utilise existing gaps in other actors’ relationships (i.e. opportunities). Actors with high level of structural holes have more such gaps which translate to opportunities that can be exploited.
Each score is then converted into an index with an average value of 100 and standard deviation of 1, with the values of betweenness centrality and structural holes first standardised and then increased by 100 to ease interpretation. Within the data range, each 0.5 value difference constitutes an actual difference of approximately 5% for BC and approximately 9% for SH variables. In order to understand the evolution of the USO, and analyse the firm performance beyond formation, this study takes a longer time framework, in essence building on and extending the stage-based frameworks discussed above.
4. Findings and discussion
This section presents the results of the social network analysis of the USOs aiming to better understand the interplay between the firm itself, and the USO shareholder network characteristics across different regional contexts. We first focus on the firm (USO) level networks by depicting how USOs at different development stages structure networks with their universities and shareholders, and then consider the regional level of USO networks presenting a typology of entrepreneurial regions.
4.1. USO networks – firm level
shows the evolution of the USO networks for firms aged 0–30.Footnote1 The network structure is captured with betweenness centrality and structural holes measures which are averaged for each individual firm age-year. There is a non-linearity observed in the development of USO networks, in particular with a clear starting point where USOs present weak centrality positions being strongly constrained in their networks. Over the first few years the situation clearly changes where USOs between ages 5 and 15 achieve peaks in developing their networks across both measures. Older USOs show signs of a greater level of fluctuation.
Figure 1. Stages of USO development. Note: Data limited to active USOs aged 0–30 years due to inconsistent annual data availability for older spinoffs. The resultant N = 1024 spinoffs, with the exclusion of only 9 firms.
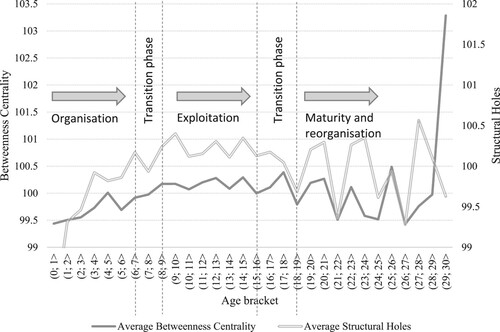
In order to understand what the USO network evolution data says about USO development we employ a simple measure in the mean average for both BC and SH variables. Reaching the mean value for BC and SH development signifies a start of a new development stage where USOs have configured their networks to optimal or above optimal levels, enabling them to benefit from accumulated social capital (Coleman Citation1988; Burt Citation1992; Zaheer and Soda Citation2009). What this means for USOs is that they are now playing important roles (at least at the average level) in the network – where the reliance of network actors on information and resources travelling through USOs has increased (Borgatti, Everett, and Johnson Citation2018). Equally so, once the USO network characteristics for BC and SH dip below the mean value, it signifies suboptimal network configurations and a different phase in USO development. This can be interpreted as loss of importance of USOs in the network below the average value for the network. The mean valueFootnote2 for BC is 100.05 and for SH it is 99.93.
As such we identify two clear transition stages, first, when USOs reach the mean value for SH (start of the transition) and for BC (end of the transition). We call this transition stage optimum, and find that it corresponds with Vohora et al.’s (Citation2004) threshold of sustainability, where a firm transforms its network resources and capabilities into more profitable, reorganising its network structure. This is where we expect the academically-oriented networks of USOs morphing into commercially-oriented ones (Pérez Pérez and Mártinez Sánchez Citation2003). Second, when the USOs’ networks are in decline and cross the mean value for BC (start of the transition) and SH (end of the transition). We identify this transition stage as lock-in. Whilst previous research did not identify this juncture, there is a distinct exhaustion in USO network development where the aggregate constraint increases and betweenness centrality declines. This could lead to failure of the USO (Prokop, Huggins, and Bristow Citation2019), as the returns from existing contacts diminish, resulting in increased redundancy of connections and demotion from the previously powerful central position.
These transition stages separate three distinct phases in USO development: (1) organisation phase, (2) exploitation phase, and (3) maturity and reorganisation phase. The organisation phase covers the early years of USO development post-formation. The network-based analysis shows that USOs in this phase are characterised by centralising the network position and increased entrepreneurial behaviour (Burt Citation1992). This phase reflects Vohora et al.’s (Citation2004) ‘re-orientation’ phase, where firms accumulate resources and translate them into new sets of entrepreneurial capabilities. Here, these are network resources that enable USOs to develop their technologies by mobilising their contacts (Parmentola and Ferretti Citation2018), connecting with investors and growing.
When the USO transforms its network position towards achieving the optimum, it transitions towards the exploitation phase, where USOs stabilise their network structure. Exploitation phase goes further than Vohora et al.’s (Citation2004) ‘sustainable returns’ stage. In the exploitation phase the USO is characterised by above optimal network characteristics, where the board composition changes in line with required skillsets (Bjornali and Gulbrandsen Citation2010) and new networks that need accessing. The length of this phase is determined by the firm’s observation of the lock-in effect, where previously new and heterophilous relations become homogenised over time by shared experiences, routines and groupthink. This could also be reflected through a tendency for homophilous management team formation in new ventures (Parker Citation2009) and further additions to the USO’s board from founder’s networksFootnote3 (Bjornali and Gulbrandsen Citation2010), essentially having a cumulative effect of increasing network constraint. The phase ends when the USO starts losing its centrality and its aggregate constraint increases, essentially leading the USO towards a network lock-in characterised by the firm’s increased unresponsiveness to market dynamics, limited innovation levels, and an intensified reliance on a smaller network of stronger ties (Capaldo Citation2007). The end of the lock-in transition signifies a start of the next phase: maturity and reorganisation. This is observed from both measures crossing their mean values multiple times, indicating a level of dynamism in network positions. Spinoffs at this stage of development form new ties and disband old ones in pursuit of new knowledge and to ensure long term survival (Huggins Citation2010) by avoiding network lock-in and regaining the optimum. To achieve this, USOs need to become more selective in network development (Walker, Kogut, and Shan Citation1997) in order to increase their levels of opportunities (Burt Citation1992) that are related to higher probability of success (Prokop, Huggins, and Bristow Citation2019). These phases represent a non-linear network development. We depict the USO development model based on the network data in .
4.2. USO networks – regional level
We aggregate the network characteristics of the USOs to depict average structural positions of USOs based across twelve unitary regions in the UK (). There is a clear divergence between regional entrepreneurial conditions as reflected in network attributes. The Greater South East regions, inclusive of London, South East and East of England, are home to USOs with the strongest network positions across both betweenness centrality and structural holes. Whilst USO numbers and their survival in these regions are not exceptional (), USOs based in these most economically developed regions clearly benefit from accumulated stocks of structural network capital (Huggins and Prokop Citation2017). This suggests that USOs based in these regions gain access more quickly to more important actors than USOs based elsewhere. This could be due to a presence of well-performing universities and companies in these regions or regional innovative performance, with the causality of the mechanism remaining unknown (Huggins and Prokop Citation2017). Whilst the data does not allow us to comment on prior informal contacts actors in these regions have, the greater presence of some of the key actors in these regions (e.g. venture capitalists, entrepreneurial talent), past connections, could offer some of the potential explanations for the observed results. Conversely, the most peripheral economic regions of North East and Wales present further diversity in terms of their network positions, with Wales characterised by higher level of survival of its USOs also showing stronger network characteristics (Prokop, Huggins, and Bristow Citation2019).
depicts these values in a graphical form, where we centre the axes at 100 for both BC and SH. We identify a new variety of entrepreneurial regions. Instead of a typical dichotomy of core versus periphery, four types of regions emerge: (a) peripheral lock-in, (b) entrepreneurial periphery, (c) rigid core, and (d) entrepreneurial core.
Table 2. Regionalised characteristics of the USO networks.
The peripheral lock-in regions (e.g. North East) are characterised by constrained network positions of their USOs struggling to access a more diverse pool of actors, which may be either available in scarcity or not available at all. USOs in such regions struggle to build their centrality, as the availability of ecosystem actors is markedly lower (Xu and Dobson Citation2019). For example, it is possible to observe limited pockets of external entrepreneurial talent to form strong management teams or attract venture capital investment, highly concentrated in London, Cambridge and Oxford (Prokop Citation2021b). This may be further complemented by the low participation of the USOs in such regions in their respective clusters (Bagchi-Sen, Baines, and Smith Citation2020).
In comparison, the entrepreneurial periphery depicts a type of region (e.g. Wales) where USOs are not well-connected overall, but enjoy access to some key actors channelling entrepreneurial opportunities (Burt Citation1992). The limited connectedness may stem from locational conditions (Huggins and Prokop Citation2017) and less developed ecosystems, making access to a large pool of actors difficult. At the same time, USOs in entrepreneurial periphery could be better able to catalyse limited local network resources, and where possible connect with the important ecosystem actors from outside their regions (Prokop Citation2021b). This may be observed through a more strategic orientation towards securing investors and entrepreneurial talent (Huggins Citation2010), regardless of where it is located.
USOs that reside in a rigid core type region (e.g. Scotland) benefit from high levels of connectivity, but have limited entrepreneurial opportunities potentially stemming from network closure effects. Such regions may contain quite well developed and connected local ecosystems reflecting network dynamics of scale-up ecosystems (Brown and Mason Citation2017), that have a good pool of actors in vicinity. However, the higher level of aggregate constraint indicates a potential homophily and disconnect of such ecosystems (i.e. spatial dynamics of embryonic ecosystems – Brown and Mason Citation2017), essentially limiting availability of diverse strategic actors and opportunities that come with them (Burt Citation1992). We may observe that USOs in rigid core regions have good access to entrepreneurial talent, investment and markets, but due to high centrality and disconnect they may be characterised by groupthink and limited ability to identify a successful development path (Capaldo Citation2007).
The entrepreneurial core typifies regions (e.g. South East) where USOs hold central positions in the network with access to a greater level of entrepreneurial opportunities. This preferential network structure may be a consequence of network capital accumulated by the local ecosystem actors (Huggins and Prokop Citation2017), in particular its self-reinforcing character (Minniti Citation2005). It is important to note that the entrepreneurial core is aligned with the regions that are also the most economically developed in the UK, clearly offering an environment rich not only in the numbers of actors but also their higher level of diversity, enabling USOs positioned there to quickly grow their betweenness centrality and benefit from higher proportion of structural holes. This means that such regions have easier access to a wide-ranging entrepreneurial talent, investors and other key ecosystem actors (Hayter et al. Citation2018).
5. Conclusion
This study employs a novel network-based view of the USO development across diverse regions and regional entrepreneurial environments, resulting in two key contributions. First, we build on Vohora et al.’s (Citation2004) development model by identifying two additional phases: exploitation, and maturity and reorganisation, capturing the late-stage development and building on previous USO development models (e.g. Parmentola and Ferretti Citation2018). The exploitation phase is when USOs capitalise on their developed networks, further reinforcing them through exploring new opportunities (Burt Citation1992) and strengthening their centrality position. This is followed by the maturity and reorganisation phase when USOs actively respond to a threat of network lock-in, homophily and limited development opportunities. This stage is characterised by greater dynamism in network development that is characterised by more strategic and calculative behaviour (Huggins Citation2010).
Second, following Hayter (Citation2016b) indicating the importance of the regional characteristics to the performance of USO networks, we observe differences in USO network development across regional contexts, suggesting a novel typology of entrepreneurial regions. Specifically, we identify four such types: (a) peripheral lock-in, (b) entrepreneurial periphery, (c) rigid core, and (d) entrepreneurial core. Peripheral lock-in regions are characterised by their USOs having weaker network positions observed in lower values for betweenness centrality and structural holes of individual USOs compared to those based in other regions. Such regions offer lower stocks of network capital to their firms (Huggins and Prokop Citation2017). In contrast, entrepreneurial periphery regions show greater level of opportunities being accessible to firms, even though the availability of actors is limited. This may be a result of USOs from these regions developing connections to actors in other regions (Prokop Citation2021b). USOs based in rigid core regions enjoy stronger connectivity with local/regional actors, however suffer from peripherality and limited opportunities for growth. USOs in such regions struggle to access particular types of actors e.g. entrepreneurial talent (Prokop Citation2021b), important to developing the companies. Finally, entrepreneurial core regions offer their firms the best network capital, enabling the USOs based in these regions to grow more central positions and explore a greater level of access to more diverse opportunities, perpetuating success (Minniti Citation2005).
5.1. Implications
A variety of national and regional policy measures has been put in place to stimulate the creation of university spin-off firms over the last decades. Universities have changed their policies, incentive mechanisms to encourage academic entrepreneurship. In this context, the findings presented here signify a number of policy and management implications.
5.1.1 Managerial implications
The USO development follows a non-linear path, and instead of stability (Vohora, Wright, and Lockett Citation2004) the later stages are characterised by further instability, as visible from our analysis of aggregated data, where the company needs to reorganise its networks to ensure survival. This finding extends the theoretical works on stages of growth of spinoff companies (e.g. Ndonzuau, Pirnay, and Surlemont Citation2002; Gübeli and Doloreux Citation2005). In particular, our research highlights the continuous challenges even for the established firms.
For university administrators interested in the success of their spinoff companies, our findings suggest a longer-term engagement in supporting the firms, potentially through the technology transfer officers to monitor spinoff companies and be available to engage with them by mobilising their connections to university ecosystem actors (Hayter et al. Citation2018) and also encouraging USOs to proactively build new unique connections. For investors with equity stakes in spinoffs it is important to recognise that even at later stages they may have to return to a more active role either proactively – recognising the upcoming network lock-in and steering the firm’s strategic development, or responsively by tapping into their vast networks to help the spinoffs reorganise their management teams.
5.1.2 Policy implications
This long-term view of spinoff development opens new avenues for policymakers, university administrators, and investors, to better understand the needs of companies for steering and support. In particular, the policymakers need to recognise that business support should not only just focus on the initial development phases, but may also be required at later stages, especially when things may appear stable, potentially indicating a network lock-in.
The taxonomy of regions presented here offers a more complex perspective on regional entrepreneurship, and specifically indicates the struggle of poorer connectivity among more peripheral regions (peripheral lock-in and entrepreneurial periphery), compared to a stronger network capital accumulation available in the entrepreneurial core (Huggins and Prokop Citation2017). The policymakers may consider placing a greater emphasis on building inter-regional networks to at least partially alleviate the issues faced by peripheral regions that lack the necessary connections in their localities.
Finally, the regional network results also present a perplexing nature of academic entrepreneurship, where peripheral regions with weaker network capital observe superior survival outcomes, whilst entrepreneurial core regions achieve mediocre survival results at best. A similar picture was observed in Mueller, Westhead, and Wright (Citation2012) with regards to venture capital finance. This could be explained by different risk profiles of these regions, with more selectivity taking place in the periphery. It is important for policymakers to observe this ‘efficiency’ in employing network resources, when deciding which regions should receive support and the extent of it.
5.2. Limitations and recommendations
This research is not without limitations, and a more comprehensive picture of networks could be presented if a larger network was captured rather than just shareholder-based one. However, it is extremely difficult to identify the full picture of network with a variety of actors (Hayter Citation2016a) and confirm their belonging, which would provide insights on the availability of potential for actions, in addition to network capital acted on (Huggins Citation2010). Scholars in the field of academic entrepreneurship may build on the findings presented in this paper by capturing such networks of spinoff companies to examine their evolution. Further to that, future research could focus on the questions of which connections lead to particular shareholders taking equity in spinoff companies and what the connections between individual shareholders are. Whilst this paper employed two social network measures well established in innovation and economic geography literatures (Huggins, Prokop, and Thompson Citation2020), a more intricate picture could be captured by observing other network characteristics, which may require additional data – for example specifying the value of each relation. We also recognise that even though the paper captures a large span of USO lifecycle, this is still a relatively short period of development captured, as indicated by Vincett (Citation2010). Future studies would do well to expand on this, especially highlighting the complex interplay between institutional and regional contexts, as the USOs become more established firms.
Acknowledgements
The financial support from the Economic and Social Research Council is greatly appreciated. The manuscript has also benefited from very helpful comments of the special issue editors and colleagues, and two anonymous reviewers, for which we are extremely grateful.
Disclosure statement
No potential conflict of interest was reported by the authors.
Additional information
Funding
Notes
1 The choice of this age group is dictated by the more inconsistent data availability for older USOs across regions.
2 Whilst the full sample of firms has an average of 100 for each of the measures, because we select a slightly reduced sample (aged 0–30) and average these measures for each individual USO age, the mean value for such a changed sample does not equal 100.00.
3 Whilst at first the new additions increase the USO’s structural position, further additions from the same networks of the founders introduce redundant ties.
References
- Bagchi-Sen, S., N. Baines, and Lawton Smith. 2020. Characteristics and Outputs of University Spin-offs in the United Kingdom. International Regional Science Review. doi:10.1177/0160017620925129
- Benneworth, P., and D. Charles. 2005. “University Spin-off Policies and Economic Development in Less Successful Regions: Learning from two Decades of Policy Practice.” European Planning Studies 13 (4): 537–57.
- Berbegal-Mirabent, J., E. Lafuente, and F. Solé. 2013. “The Pursuit of Knowledge Transfer Activities: An Efficiency Analysis of Spanish Universities.” Journal of Business Research 66 (10): 2051–9.
- Berggren, E. 2011. “The Entrepreneurial University’s Influence on Commercialization of Academic Research –the Illustrative Case of Chalmers University of Technology.” International Journal of Entrepreneurship and Small Business 12 (4): 429–44.
- Berggren, E., and Å Lindholm Dahlstrand. 2009. “Creating an Entrepreneurial Region: Two Waves of Academic Spin-Offs from Halmstad University.” European Planning Studies 17 (8): 1171–89.
- Bigdeli, A. Z., F. Li, and X. Shi. 2015. “Sustainability and Scalability of University Spinouts: A Business Model Perspective.” R&D Management 46 (3): 504–18.
- Bjornali, E. S., and M. Gulbrandsen. 2010. “Exploring Board Formation and Evolution of Board Composition in Academic Spin-Offs.” Journal of Technology Transfer 35: 92–112.
- Bolzani, D., E. Rasmussen, and R. Fini. 2021. “Spin-offs’ Linkages to Their Parent Universities Over Time: The Performance Implications of Equity, Geographical Proximity, and Technological Ties.” Strategic Entrepreneurship Journal 15 (4): 590–618.
- Bonardo, D., S. Paleari, and S. Vismara. 2011. “Valuing University-Based Firms: The Effects of Academic Affiliation on IPO Performance.” Entrepreneurship Theory and Practice 35 (4): 755–76.
- Borgatti, S. P., M. G. Everett, and J. C. Johnson. 2018. Analyzing Social Networks, (2nd ed). London: Sage Publications Ltd.
- Bourelos, E., M. Magnusson, and M. McKelvey. 2012. “Investigating the Complexity Facing Academic Entrepreneurs in Science and Engineering: The Complementarities of Research Performance, Networks and Support Structures in Commercialisation.” Cambridge Journal of Economics 36 (3): 751–80.
- Bower, J. D. 2003. “Business Model Fashion and the Academic Spinout Firm.” R&D Management 33 (2): 97–106.
- Braunerhjelm, P. 2007. “Academic Entrepreneurship: Social Norms, University Culture and Policies.” Science and Public Policy 34 (9): 619–31.
- Brown, R., and C. Mason. 2017. “Looking Inside the Spiky Bits: A Critical Review and Conceptualisation of Entrepreneurial Ecosystems.” Small Business Economics 49: 11–30.
- Burt, R. S. 1992. Structural Holes. The Social Structure of Competition. London: Harvard University Press.
- Capaldo, A. 2007. “Network Structure and Innovation: The Leveraging of a Dual Network as a Distinctive Relational Capability.” Strategic Management Journal 28: 585–608.
- Chan, K.-Y. A., L. A. G. Oerlemans, and M. W. Pretorius. 2010. “Knowledge Exchange Behaviours of Science Park Firms: The Innovation Hub Case.” Technology Analysis & Strategic Management 22 (2): 207–28.
- Coleman, J. S. 1988. “Social Capital in the Creation of Human Capital.” The American Journal of Sociology 94: S95–S120.
- Fini, R., K. Fu, M. T. Mathisen, E. Rasmussen, and M. Wright. 2017. “Institutional Determinants of University Spin-Off Quantity and Quality: A Longitudinal, Multilevel, Cross-Country Study.” Small Business Economics 48 (2): 361–91.
- Fini, R., R. Grimaldi, S. Santoni, and M. Sobrero. 2011. “Complements or Substitutes? The Role of Universities and Local Context in Supporting the Creation of Academic Spin-Offs.” Research Policy 40 (8): 1113–27.
- Franklin, S. J., M. Wright, and A. Lockett. 2001. “Academic and Surrogate Entrepreneurs in University Spin-out Companies.” Journal of Technology Transfer 26: 127–41.
- Freeman, L. C. 1977. “A Set of Measures of Centrality Based on Betweenness.” Sociometry 40 (1): 35–41.
- Galbraith, J. 1982. “The Stages of Growth.” Journal of Business Strategy 3: 70–9.
- Garnsey, E., and P. Heffernan. 2005. “High-Technology Clustering Through Spin-out and Attraction: The Cambridge Case.” Regional Studies 39 (8): 1127–44.
- Grandi, A., and R. Grimaldi. 2003. “Exploring the Networking Characteristics of New Venture Founding Teams.” Small Business Economics 21 (4): 329–41.
- Gübeli, M. H., and D. Doloreux. 2005. “An Empirical Study of University Spin off Development.” European Journal of Innovation Management 8 (3): 269–82.
- Hayter, C. S. 2016a. “Constraining Entrepreneurial Development: A Knowledge-Based View of Social Networks among Academic Entrepreneurs.” Research Policy 45: 475–90.
- Hayter, C. S. 2016b. “A Trajectory of Early-Stage Spinoff Success: The Role of Knowledge Intermediaries Within an Entrepreneurial University Ecosystem.” Small Business Economies 47: 633–56.
- Hayter, C. S., A. J. Nelson, S. Zayed, and A. C. O’Connor. 2018. “Conceptualizing Academic Entrepreneurship Ecosystems: A Review, Analysis and Extension of the Literature.” The Journal of Technology Transfer 43: 1039–82.
- Huggins, R. 2008. “Universities and Knowledge-Based Venturing: Finance, Management and Networks in London.” Entrepreneurship and Regional Development 20: 185–206.
- Huggins, R. 2010. “Forms of Network Resource: Knowledge Access and the Role of Inter-Firm Networks.” International Journal of Management Reviews 12 (3): 335–52.
- Huggins, R., and D. Prokop. 2017. “Network Structure and Regional Innovation: A Study of University–Industry Ties.” Urban Studies 54 (4): 931–52.
- Huggins, R., D. Prokop, and P. Thompson. 2020. “Universities and Open Innovation: The Determinants of Network Centrality.” The Journal of Technology Transfer 45: 718–57.
- Iacobucci, D., and A. Micozzi. 2015. “How to Evaluate the Impact of Academic Spin-Offs on Local Development: An Empirical Analysis of the Italian Case.” The Journal of Technology Transfer 40: 434–52.
- Kitagawa, F., and S. Robertson. 2012. “High-Tech Entrepreneurial Firms in a University-Based Business Incubator: Spaces of Knowledge, Resource Heterogeneity and Capital Formation.” The International Journal of Entrepreneurship and Innovation 13 (4): 249–59.
- Landry, R., N. Amara, and I. Rherrad. 2006. “Why are Some University Researchers More Likely to Create Spin-Offs Than Others? Evidence from Canadian Universities.” Research Policy 35 (10): 1599–615.
- Laumann, E. O., P. V. Marsden, and D. Prensky. 1992. “The Boundary Specification Problem in Network Analysis.” In Research Methods in Social Network Analysis, edited by L. C. Freeman, D. R. White, and A. K. Romney, 61–88. New Brunswick, NJ: Transaction Publishers.
- Lawton Smith, H., D. Chapman, P. Wood, T. Barnes, and S. Romeo. 2014. “Entrepreneurial Academics and Regional Innovation Systems: The Case of Spin-Offs from London's Universities.” Environment and Planning C: Government and Policy 32: 341–59.
- Lawton Smith, H., S. Romeo, and S. Bagchi-Sen. 2008. “Oxfordshire Biomedical University Spin-Offs: An Evolving System.” Cambridge Journal of Regions, Economy and Society 1 (2): 303–19.
- Li, J., D. Ren, X. Feng, and Y. Zhang. 2016. “Network of Listed Companies Based on Common Shareholders and the Prediction of Market Volatility.” Physica A: Statistical Mechanics and its Applications 462: 508–21.
- Lockett, A., M. Wright, and S. Franklin. 2003. “Technology Transfer and Universities’ Spin-Out Strategies.” Small Business Economics 20: 185–200.
- Marzocchi, C., F. Kitagawa, and M. Sánchez-Barrioluengo. 2019. “Evolving Missions and University Entrepreneurship: Academic Spin-Offs and Graduate Start-ups in the Entrepreneurial Society.” The Journal of Technology Transfer 44: 167–88.
- Minniti, M. 2005. “Entrepreneurship and Network Externalities.” Journal of Economic Behavior & Organization 57: 1–27.
- Mitchell, J. C. 1969. “The Concept and use of Social Networks.” In Social Networks in Urban Situations, edited by J. C. Mitchell, 1–50. Manchester: Manchester University Press.
- Mueller, C., P. Westhead, and M. Wright. 2012. “Formal Venture Capital Acquisition: Can Entrepreneurs Compensate for the Spatial Proximity Benefits of South East England and ‘Star’ Golden-Triangle Universities?” Environment and Planning A: Economy and Space 44 (2): 281–96.
- Ndonzuau, F. N., F. Pirnay, and B. Surlemont. 2002. “A Stage Model of Academic Spin-off Creation.” Technovation 22 (5): 281–9.
- Parker, S. C. 2009. “Can Cognitive Biases Explain Venture Team Homophily?” Strategic Entrepreneurship Journal 3: 67–83.
- Parmentola, A., and M. Ferretti. 2018. “Stages and Trigger Factors in the Development of Academic Spin-Offs An Explorative Study in Southern Italy.” European Journal of Innovation Management 21 (3): 478–500.
- Patzelt, H., and D. Shepherd. 2009. “Strategic Entrepreneurship at Universities: Academic Entrepreneurs’ Assessment of Policy Programs.” Entrepreneurship Theory and Practice 33 (1): 319–40.
- Pérez Pérez, M., and A. M. Mártinez Sánchez. 2003. “The Development of University Spin-Offs: Early Dynamics of Technology Transfer and Networking.” Technovation 23 (10): 823–31.
- Prokop, D. 2021a. “The Academic Spinoff Theory of the Firm.” The International Journal of Entrepreneurship and Innovation. doi:10.1177/14657503211066013.
- Prokop, D. 2021b. “University Entrepreneurial Ecosystems and Spinoff Companies: Configurations, Developments and Outcomes.” Technovation 107: 102286.
- Prokop, D. 2022. “The Composition of University Entrepreneurial Ecosystems and Academic Entrepreneurship: A UK Study.” International Journal of Innovation and Technology Management. doi:10.1142/S0219877022500201.
- Prokop, D., R. Huggins, and G. Bristow. 2019. “The Survival of Academic Spinoff Companies: An Empirical Study of key Determinants.” International Small Business Journal: Researching Entrepreneurship 37 (5): 502–35.
- Ramaciotti, L., and U. Rizzo. 2014. “The Determinants of Academic Spin-off Creation by Italian Universities.” R&D Management 45 (5): 501–14.
- Rasmussen, E. 2008. “Government Instruments to Support the Commercialization of University Research: Lessons from Canada.” Technovation 28 (8): 506–17.
- Rasmussen, E. 2011. “Understanding Academic Entrepreneurship: Exploring the Emergence of University Spin-off Ventures Using Process Theories.” International Small Business Journal 29 (5): 448–71.
- Rasmussen, E., S. Mosey, and M. Wright. 2015. “The Transformation of Network Ties to Develop Entrepreneurial Competencies for University Spin-Offs.” Entrepreneurship & Regional Development 27 (7-8): 430–57.
- Roper, S., and N. Hewitt-Dundas. 2013. “Catalysing Open Innovation Through Publicly-Funded R&D: A Comparison of University and Company-Based Research Centres.” International Small Business Journal 31 (3): 275–95.
- Sanchez-Barrioluengo, M., F. Kitagawa, and E. Uyarra. 2019. “Understanding the Evolution of the Entrepreneurial University. The Case of English Higher Education Institutions.” Higher Education Quarterly. 73 (4): 469–95.
- Sandrom, C., K. Wennberg, M. Walllin, and Y. Zherlygina. 2016. “Public Policy for Academic Entrepreneurship Initiatives: A Review and Critical Discussion.” Journal of Technology Transfer 43: 1232–1256.
- Scholten, V., O. Omta, R. Kemp, and T. Elfring. 2015. “Bridging Ties and the Role of Research and Start-up Experience on the Early Growth of Dutch Academic Spin-Offs.” Technovation 45-46: 40–51.
- Shane, S. 2004. “Encouraging University Entrepreneurship? The Effect of the Bayh-Dole Act on University Patenting in the United States.” Journal of Business Venturing 19 (1): 127–51.
- Siegel, D. S., R. Veugelers, and M. Wright. 2007. “Technology Transfer Offices and Commercialization of University Intellectual Property: Performance and Policy Implications.” Oxford Review of Economic Policy 23 (4): 640–60.
- Stam, E. 2015. “Entrepreneurial Ecosystems and Regional Policy: A Sympathetic Critique.” European Planning Studies 23: 1759–69.
- Vincett, P. S. 2010. “The Economic Impacts of Academic Spin-off Companies, and Their Implications for Public Policy.” Research Policy 39: 736–47.
- Vohora, A., M. Wright, and A. Lockett. 2004. “Critical Junctures in the Development of University High-Tech Spinout Companies.” Research Policy 33 (1): 147–75.
- Walker, G., B. Kogut, and W. Shan. 1997. “Social Capital, Structural Holes and the Formation of an Industry Network.” Organization Science 8 (2): 109–25.
- Walter, A., M. Auer, and T. Ritter. 2006. “The Impact of Network Capabilities and Entrepreneurial Orientation on University Spin-off Performance.” Journal of Business Venturing 21 (4): 541–67.
- Wasserman, S., and K. Faust. 1994. Social Network Analysis. Methods and Applications. Cambridge: Cambridge University Press.
- Wright, M., A. Lockett, B. Clarysse, and M. Binks. 2006. “University Spin-out Companies and Venture Capital.” Research Policy 35 (4): 481–501.
- Xu, Z., and S. Dobson. 2019. “Challenges of Building Entrepreneurial Ecosystems in Peripheral Places.” Journal of Entrepreneurship and Public Policy 8 (3): 408–30.
- Zaheer, A., and G. Soda. 2009. “Network Evolution: The Origins of Structural Holes.” Administrative Science Quarterly 54: 1–31.