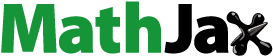
ABSTRACT
This intensive longitudinal study examined Finnish technical university teachers’ (N = 22) self-reported emotions and electrodermal activity during teaching-related events (teaching, tutoring, planning, assessing). Emotions were examined in the context of control-value theory (Pekrun, R. 2006. “The Control-Value Theory of Achievement Emotions: Assumptions, Corollaries and Implications for Educational Research and Practice.” Educational Psychology Review 18 (4): 315–41. https://doi.org/10.1007/s10648-006-9029-9). An experience sampling method with event-contingent sampling was applied via a smartphone application for a measurement period of two weeks. Participants were also equipped with a smart ring biosensor that recorded their electrodermal activity (EDA, an indicator of physiological arousal). Results indicated that teaching-related events led to predominantly positive prospective and retrospective emotions. Interactive events, such as teaching and tutoring, were especially effective in generating positive outcomes and activating emotions. Results showed that that teaching-related events had higher EDA levels than typical work hours. In addition, higher within-teacher EDA levels during teaching-related events slightly increased the likelihood of reporting positive emotional valence after teaching. Further, results indicated that teacher-student interaction (teaching and tutoring events) and higher EDA during, and emotional activity before, teaching-related events increased the likelihood of activating emotions after teaching. The results can be generalized with reservations due to the small number of participants.
Introduction
The study of emotions has become a significant area of interest in both academic and professional contexts. A deeper understanding of emotions has brought us closer to the many ways in which they are intertwined with individual performance and well-being. Within the field of education, there is a growing focus on the emotional processes of teachers and students. Emotions have been analyzed in various ways in which they may influence both teachers’ and their students’ cognition, motivation and behavior (Sutton and Wheatley Citation2003). Positive emotions and satisfaction, as well as stress and complaints, also represent the most common factors that have been shown to contribute to a blurred conceptualization of well-being (Hascher and Waber Citation2021). Studies addressing teacher well-being have highlighted the relationship between teacher well-being and various factors and desired outcomes, such as teacher sleep quality, teacher retention, teacher-student relationships and student outcomes (Dreer Citation2023). Nevertheless, ongoing research on emotions continues to challenge researchers at both a theoretical and a methodological level. Further research is also required to gain a more comprehensive understanding of emotional processes in different educational contexts. For instance, while previous research on teacher and student emotions has been conducted primarily in a school context, a more profound understanding is needed of the underlying emotional processes of higher education teaching and learning (Mendzheritskaya and Hansen Citation2019).
This multi-method study investigated teachers’ self-reported emotions and electrodermal activity (EDA) in the context of higher education. Teacher emotions were approached by applying Pekrun’s (Citation2006; Pekrun, Marsh, and Elliot Citation2023) Three-Dimensional Taxonomy of Achievement Emotions, in which emotions are viewed as multicomponent changes in an organism’s psychophysical system. The EDA data reflected the participants’ physiological arousal related to their psychological and emotional states (Boucsein Citation2012). The study examined associations between higher education teachers’ (N = 22) self-reported emotions and EDA during four different teaching-related working events, i.e. teaching, tutoring, planning and assessing, carried out in a Finnish research university in the field of engineering education. An experience sampling method (ESM, see Hektner, Schmidt, and Csikszentmihalyi Citation2007) was applied via LearningTracker smartphone application (Nokelainen and Hartikainen Citation2020) that allowed the participants to self-report their perceived emotions in authentic working situations. Physiological measurements of EDA were also conducted in authentic working situations, via ambulatory Moodmetric (Vigofere Ltd.) smart ring biosensor (Suoja et al. Citation2017).
The objective of the study was to gain a deeper understanding of the emotions that higher education teachers experience in everyday work situations. The study aimed to identify teaching-related situations in which teachers experience positive or negative (activating and deactivating, see Pekrun Citation2006) emotions, and to gain insight into how teachers respond physiologically to such situations by including the data with short-term measures of teachers’ EDA. This information will be of practical use in higher education institutions for the improvement of teachers’ work conditions and well-being. Furthermore, identifying perceived emotions and peaks of arousal in teachers’ work may provide further justification for implementing long-term measurements. Whether related to positive or negative emotions, prolonged activation of the stress-responsive systems, chronic or repeated exposure to stress hormones has been associated with a range of negative psychological and physiological outcomes (Dickerson and Zoccola Citation2009). Furthermore, the study had a methodological objective, given that research on emotions is faced with the need to complement the often-used self-reporting methods with physiological or behavioral measures taken in real time during authentic activities (Pekrun Citation2019). New methodological solutions were being explored to measure a person’s perceived emotions as closely as possible to the actual work situation, and to measure physiological arousal in authentic situations and environments.
Theoretical framework
Achievement emotions
Research on emotions usually recognizes that emotions consist of various components (Moors Citation2009). In this study, we approach teacher emotions by applying Pekrun’s (Citation2006; Pekrun, Marsh, and Elliot Citation2023) Three-Dimensional Taxonomy of Achievement Emotions, in which emotions are viewed as multicomponent changes in an organism’s psychophysical system, which may include affective, cognitive, physiological, motivational and expressive-behavioral components. Achievement emotions are defined as emotions that occur in response to events and actions that are judged according to competence-based standards of quality (Pekrun Citation2006; Pekrun, Marsh, and Elliot Citation2023). While achievement emotions have traditionally been viewed as emotional responses to success and failure, the definition extends this view to include emotions related to ongoing activities that are evaluated based on competence (i.e. judged to be performed competently, see Pekrun, Marsh, and Elliot Citation2023). Pekrun’s (Citation2006) control-value theory posits that achievement emotions are proximally determined by an individual’s cognitive appraisal of control and value. Control appraisals relate to the perceived controllability of achievement activities and their outcomes. These appraisals are often indicated by expectations and competence perceptions, such as self-efficacy (i.e. task-specific self-confidence) and self-concepts of ability. Value appraisals pertain to the subjective value or importance of these activities and outcomes that can be intrinsic (e.g. an innate interest in math) or extrinsic (e.g. valuing an activity because it is likely to bring some external reward).
Control-value theory (Pekrun Citation2006) is included with three dimensions: Valence classifies the emotions to positive (enjoyment, relaxation, hope, anticipatory relief, relief, anticipatory joy, joy, pride, contentment, gratitude) and negative (anger, boredom, frustration, anxiety, hopelessness, shame, disappointment, sadness). Activity classifies emotions based on their degree of activation (arousal) to activating (enjoyment, hope, joy, pride, anger, anxiety, shame) or deactivating (relaxation, anticipatory relief, contentment, relief, boredom, frustration, hopelessness, joy, sadness, disappointment, gratitude). Object focus determines whether the focus of the emotion is on the activity (enjoyment, relaxation, anger, boredom, frustration) or on the outcome. Furthermore, the outcome emotion is classified as either prospective (hope, relief, anxiety, hopelessness, joy) or retrospective (joy, pride, contentment, relief, shame, anger, sadness, disappointment, gratitude). It is thus possible to distinguish between concurrent emotions, which occur in parallel to an ongoing action or event, prospective emotions related to future actions and events, and retrospective emotions related to past actions and events (Pekrun, Marsh, and Elliot Citation2023).
The present study focused on teachers’ self-reported prospective and retrospective outcome-related emotions that teachers were able to self-report in authentic work situations before and after teaching sessions. Emotions were subsequently classified according to the dimensions of valence (positive and negative) and activation (activating and deactivating). As emotions such as relaxation or boredom imply both physiological and cognitive deactivation (Pekrun et al. Citation2002), the data in this study were supplemented with an investigation of physiological activation (emotional arousal), employing EDA measurements. It is assumed that activating positive emotions strengthens intrinsic and extrinsic motivation, whereas deactivating negative emotions are believed to be detrimental (Pekrun et al. Citation2002). However, the effects of deactivating positive emotions and activating negative emotions are assumed to be more complex; for instance, while anxiety can impair interest and intrinsic motivation, it can simultaneously induce extrinsic motivation to invest effort in avoiding failures (Pekrun et al. Citation2002).
Electrodermal activity
Research has been increasingly interested in the physiological component of emotions. Physiological changes that express emotions constitute energy in motion that can be measured using various physiological sensors. The sensory input of an internal and/or external stimulus (including an emotional trigger) is delivered to and processed by the brain: the brain sends ‘order messages’ via sympathetic or parasympathetic nerve fibers of the autonomic nervous system to specific body parts to respond adequately to the stimulus (Balters and Steinert Citation2017). Despite a growing body of research investigating the relationship between the autonomic nervous system and emotions, the findings remain inconclusive. The issue of whether there is coherence in emotion (within the autonomic nervous system, as well as between the autonomic nervous system and other emotional components) remains a topic of debate amongst researchers, and whether the autonomic nervous system activation differs for specific emotions (Levenson Citation2014). Variation in the results considering these complex associations might be, for example, due to choices of measures and unclear terminology of emotions (Kreibig Citation2010).
One of the most commonly used and tested indicators of the autonomic nervous system activation is electrodermal activity (EDA) (Boucsein Citation2012; Dawson, Schell, and Filion Citation2007). In the field of educational studies, the use of EDA has been continuously increasing (Ba and Hu Citation2023). Brain studies support the use of EDA as a sensitive and convenient measurement of physiological changes related to emotions (Critchley Citation2002). One of the most significant advantages of EDA in emotional research is that it differs from numerous other measures, such as heart rate, in that it measures arousal via eccrine sweating, thereby reflecting solely the activation of the sympathetic nervous system and not the parasympathetic nervous system (Boucsein Citation2012; Dawson, Schell, and Filion Citation2007). Consequently, EDA can be seen as a strong indicator of physiological arousal and emotions (Dawson, Schell, and Filion Citation2007).
EDA has been found to be positively connected with both positive (e.g. Muldner, Burleson, and VanLehn Citation2010) and negative emotions (e.g. Harley, Jarrell, and Lajoie Citation2019; Villanueva et al. Citation2018), as well as with both positively and negatively experienced stress (Boucsein Citation2012). It has also been connected to physical and cognitive demands (e.g. Cha et al. Citation2022). In Kreibig’s (Citation2010) review on autonomic nervous system activation and emotions, low EDA was associated only with emotions that do not urge for action, namely non-crying and acute sadness, contentment and relief. Emotions that reflect an action tendency (such as anger, anxiety, disgust, fear, anticipatory or crying sadness, amusement, happiness, joy, pride, surprise) were connected to increases in EDA (Kreibig Citation2010). The aforementioned emotions associated with increases and decreases in EDA align with those classified as activating emotions in the Control-value theory (see Pekrun Citation2006). This provides empirical support for the theoretical assumption that the dimension of activity reflects the physiological component of emotions (Pekrun, Marsh, and Elliot Citation2023). The connection between experienced emotional activity and EDA has also been supported in empirical studies (e.g. Gomez, Stahel, and Danuser Citation2004). However, in their review of the use of EDA in learning contexts, Horvers et al. (Citation2021) found that while other studies had found associations between self-reports of emotional valence and EDA, some had not, especially when using retrospective self-report methods.
Teacher emotions
A major relationship in which emotions play a key part is the relationship between teachers and students (Quinlan Citation2016). Teachers’ emotions and emotional or affective bonds formed with their students can inform their teaching practice such as decisions about teaching strategies, curriculum selection and lesson planning (Hagenauer and Volet Citation2014). Earlier research has also indicated that social interactions are also a key factor in teacher well-being (Hascher and Waber Citation2021). In the study by Kiltz et al. (Citation2020), the general well-being of university teachers (N = 10 teachers and N = 6 students) was reflected in their lesson planning and teaching practices, their feelings of being energetic, present and interested in their lessons, and their experiences of relationships with their students, whereas unwellness resulted in lower quality teaching, less connection with students and negative emotional experiences in the classroom.
Empirical research in the university context has also demonstrated that teachers’ emotions are related to student interactions, as well as to their experiences of teaching as a profession and their teaching practices. In Hagenauer and Volet’s (Citation2014) study, university teachers’ emotions were linked to their beliefs about the intrinsic value of teaching as a profession and to the degree to which their expectations regarding students’ engagement within the student–teacher relationship were met; teachers enjoyed teaching best in classes where teacher–student relationships were perceived as productive, whereas uncontrolled situations could cause feelings such as anxiety, fear, nervousness or insecurity. Postareff and Lindblom-Ylänne (Citation2011) reported that the most commonly positive emotions reported by university teachers (N = 97) were enjoyment of teaching and enthusiasm for teaching, while the most reported negative emotions were reluctance towards a teaching form or method. In Trigwell’s (Citation2011) study, university teachers (N = 175) who reported higher levels of emotions such as pride and motivation, and lower levels of frustration, described their teaching in terms of a focus more on what the student is doing and experiencing, whereas when teachers experienced higher levels of anxiety or nervousness or embarrassment, they were more likely to report using more teacher-centered methods.
Studies using physiological measures of teachers’ emotions have particularly highlighted the links between physiological arousal and teachers’ self-efficacy or control over classroom situations and students. Hagenauer, Hascher, and Volet (Citation2015) found that secondary school teachers’ and students’ interpersonal relationships positively predicted teachers’ (N = 132) joy and negatively predicted their anxiety, while anger was predicted by lack of discipline in class. Junker, Donker, and Mainhard (Citation2021) studied 40 secondary school teachers in authentic teaching sessions and found that teachers’ high heart rates were associated with low student engagement and teacher-centered activities. In Junker and Holodynski’s (Citation2022) study the most common issues related to teachers’ (N = 8, various school levels) high EDA levels in authentic teaching sessions were uncertainty, time management, and students’ disruptive behavior. Jõgi et al. (Citation2023) have examined the relationships between classroom teachers’ (N = 45) daily physiological stress and their self-efficacy beliefs and found that physiological stress (salivary cortisol levels) in the classroom depended on teachers’ belief in their professional skills, such as finding appropriate teaching strategies and handling discipline problems. Although high arousal has mainly been associated with negative perceived emotions in these studies, Donker et al.’s (Citation2020) study showed that secondary school teachers’ (N = 80) classroom agency (e.g. to provide leadership and structure in teaching) was associated with high heart rates and related to positive emotions after teaching.
On the other hand, a teacher’s emotions may also be influenced by other factors related to the social environment, such as cooperation with colleagues or performance situations as part of the teaching process. For instance, De Zordo, Hagenauer, and Hascher (Citation2019) found that teacher students’ beliefs in their teaching and teamwork skills were connected to their emotions. Students’ co-operation with other students has also been associated with increases in EDA (e.g. Moya-Albiol et al. Citation2013; Sariñana-González, Romero-Martínez, and Moya-Albiol Citation2017). Hoogerheide et al.’s (Citation2018) study showed significantly higher EDA when 54 university students in a laboratory environment were instructed to teach to an imaginary peer student via web camera than when they were studying learning material or solving a problem. Elfering and Grebner (Citation2011) studied ten graduate students during oral presentations and discovered that EDA rose among all presenters and remained high after the presentations among presenters with lower self-confidence.
Research questions and hypotheses
Although there is a growing body of research on teachers’ emotions, there is still a need for further research on teachers’ emotions in higher education contexts and emotions in teaching-related situations beyond the teaching situation. Furthermore, little is still known about teachers’ perceived emotions in relation to physiological responses. In this study, we have focused on examining both teaching and tutoring situations that involve interaction, as well as planning and assessment, which represent the broader job description of the higher education teacher. Specific features of this study compared to previous studies are: (1) teachers were asked to report only teaching-related events using a smartphone application during the measurement period, (2) they self-rated their emotional state at the beginning and end of each event, (3) they wore a smart ring to record their EDA during the events. This design also allows us to examine the stability of emotional states during the events, i.e. if a teaching-related event began with a positive emotion, did it also end with a positive emotion? Most previous studies have focused on comparing differences between individuals, whereas in this study we can also analyze teacher-specific emotional states and EDA during teaching events, as all time-variant variables in the analyses were centered within and between teachers (as clusters).
Based on the earlier research presented in the previous chapter, we have formulated the following research questions and hypotheses:
RQ 1: How are prospective and retrospective academic outcome emotions related to teaching, tutoring, planning and assessing student work?
Hypothesis 1 (RQ 1): (H1a) We expect that the teachers are most emotionally positively affected during teaching and tutoring as social interactions are important antecedents for emotions in teaching. (H1b) In addition, we hypothesize that teaching and tutoring of students (as interactive situations) raises most activating emotions as interactive situations have shown to be the main source of positive activating emotions.
RQ 2: How is EDA related to teaching, tutoring, planning and assessing student work?
Hypothesis 2 (RQ 2): (H2a) We expect to see higher EDA during all teaching-related events compared to a working day baseline level: The earlier results indicate that the increased level of attention and cognitive demand produces changes in EDA. (H2b) Further, the highest EDA are expected to appear during interactive situations (such as teaching and tutoring) as they have been previously connected to higher EDA than other types of tasks (studying learning material or solving a problem), and to student-related factors that increase EDA. In addition, performing settings have increased EDA levels. (H2c) Finally, we expect to see higher EDA when teachers work with another person compared to working alone, as cooperative settings have shown to increase automatic nervous system activation.
RQ 3: How is EDA during teaching-related events related to a) emotional valence and b) emotional activity after the events?
Hypothesis 3 (RQ 3): (H3a) Regarding emotional valence during teaching-related events, we expect to see higher physiological arousal levels with both positive and negative outcome emotions. In practice, this means that we expect the regression estimates of EDA to be close to zero, since a high EDA can be associated with both negative and positive valence. (H3b) Regarding emotional activity, we expect to see higher physiological arousal levels with (positive and negative) activating emotions and lower physiological arousal levels with (positive and negative) deactivating emotions.
Methods
Participants
The participants were 22 (14 women, 63.6%) teachers out of approximately 200 total teaching staff members in a Finnish technical university. Participants’ average age was 40.4 years (SD = 7.5), and their total work experience average was 17.2 years (SD = 7.5). They represented several technical HE disciplines, including Architecture, Automation technology, Chemistry, Civil Engineering, Electrical engineering, Mathematics, Physics and Photonics.
Procedure
Data was collected during measurement periods that lasted from 6 to 18 days. The voluntary participants were met in one-on-one sessions and introduced to the ethical principles of the study, including the voluntary nature of participation, the right to withdraw from the study at any time, and the assurance that no individual participants could be recognized from the results. Participants were introduced to the research process, the type of data that would be collected, and made aware of the confidentiality and handling of the data. They gave written informed consent before participating. The study was conducted according to the guidelines of the Finnish National Research Ethics Committee, and the ethics approval was given by the Tampere University Research Ethics Board (decision number tty16/09/23).
The preparation of the data for analysis and the development of the analysis programs (Nokelainen and Aldahdouh Citation2020) followed a rigorous process. After completing data collection in late 2018, the compilation of the panel data from various sources and comprehensive validity checks on the analysis programs were conducted throughout 2019. This meticulous process ensured that the panel data were ready for analysis by 2020. Such a detailed and systematic approach to data preparation and program development was crucial for ensuring the accuracy and reliability of the analysis.
The participants were advised to wear the smart ring as much as possible during the first 12 hours so it could calibrate to each participant’s personal EDA range. They were also instructed to report all teaching-related events via a smartphone application on a daily basis. Participants reported 219 events during the study: Teaching (111 events from 19 teachers, M = 5.9, SD = 4.1), tutoring (19 events from 11 teachers, M = 1.7, SD = 1.0), planning (73 events from 14 teachers, M = 5.1, SD = 5.2) and assessing (16 events from 10 teachers, M = 1.5, SD = 1.1). The average number of teaching-related events per participant was 10.0 (SD = 8.1). The reported events duration was from 30 to 489 minutes with an average value of 86.1 minutes (SD = 57.6).
Measurements
A smart ring was used to measure EDA from the palmar side of the hand (Suoja et al. Citation2017). Studies by Torniainen et al. (Citation2015) and Pakarinen, Pietilä, and Nieminen (Citation2019) have demonstrated high similarity between the raw signals obtained from the smart ring (sampling rate 3 Hz) and non-ambulatory laboratory-grade equipment (sampling rates of SA9309M and BIOPAC MP160 were 256 Hz and 2000Hz, respectively). The smart ring records five tonic (related to SCL, skin conductance level: slow climbing general level changes in autonomic physiological arousal) and 17 phasic (SCRn, skin conductance response frequency: faster changes in autonomic physiological arousal) EDA features (Pakarinen, Pietilä, and Nieminen Citation2019). Based on these features, it produces a dynamic double normalized MM index value (1-100) in a one-minute time interval, which is suitable for longitudinal measurements (Salonius et al. Citation2024). As the MM index is normalized, its value range in this study was comparable across participants. Before the actual measurement, the participants were instructed to wear the ring for approximately 24 hours, the calibration period of the ring. More information about EDA data processing is available in the online Supplementary Material.
The experience sampling method (ESM) (via a smartphone application) was used to self-report daily emotions during teaching-related events. When the application was started, participants responded to the following multiple-choice questions: I’m working in a (classroom, public space on campus, public space outside campus or private apartment); I’m working (by myself, with another person, in a group of 3–30 persons, in a group of more than 30 persons). In addition, they indicated the type of the event by either answering an open question. Following these questions, they were asked to select one prospective outcome-related emotion (Pekrun Citation2006) related to the forthcoming event: When thinking about my working in this event, I feel (‘hope’, ‘joy’, ‘relief’, ‘anxiety’ or ‘hopelessness’). After completing the task, the participants answered a question related to the retrospective outcome-related emotion (Pekrun Citation2006): When thinking about my working after this event, I feel (‘joy’, ‘pride’, ‘contentment’, ‘relief’, ‘gratitude’, ‘shame’, ‘anger’, ‘sadness’ or ‘disappointment’). More information about ESM data collection is available in the online Supplementary Material.
Statistical analyses
EDA from 22 measurement instruments (smart ring) was merged and prepared for analysis (SCL values from 1 to 100 μS and SCR frequencies from 0 to 25) with the Java-based MergeSR program (Nokelainen and Aldahdouh Citation2020). The merged smart ring data were connected to smartphone application data with the Java-based AnalyseSRLT program (Nokelainen and Aldahdouh Citation2020). The final data contained average physiological arousal values for 219 teaching-related events (‘EDA’ variable, values from 1 - 100), an index of teaching-related events for each participant across the measurement period (‘Time’ variable, values from 1), a numerical code for each teaching-related event (‘teachEvent’ variable: 1 = ‘Teaching’, 2 = ‘Tutoring’, 3 = ‘Planning’, 4 = ‘Assessing’), emotional valence before and after each teaching event (‘ValenceBefore’ and ‘ValenceAfter’ variables: 0 = ‘Negative’, 1 = ‘Positive’) and emotional activation before and after each teaching event (‘ActivityBefore’ and ‘ActivityAfter’ variables: 0 = ‘Deactivating’, 1 = ‘Activating’). The ‘teachEvent’ variable was further recoded into the ‘Interaction’ variable to indicate whether students were present in the teaching-related event or not (indication of student presence in the teaching-related event: 0 = ‘No’ (‘Planning’ and ‘Assessing’), 1 = ‘Yes’ (‘Teaching’ and ‘Tutoring’)). Two-level Kendall’s tau-b (τb) correlations are available in the online Supplementary Material.
The resulting panel data were analyzed in the R statistical computing environment (R Core Team Citation2022). Analyses related to RQ 1 were conducted with basic R functions. RQ 2 was analyzed with Bayesian multinomial and Gaussian multilevel regression analyses (brms::brm program, Bürkner Citation2017). RQ 3 was analyzed with Bayesian logistic multilevel regression analysis with the brm program.
The response variables in the Bayesian multilevel regression analysis were average electrodermal activity during teaching-related events (RQ 2), emotional valence (RQ 3a) and emotional activity (RQ 3b) after teaching-related events. The predictors in these analyses included self-rated emotional valence and activity before teaching-related events and physiologically measured average EDA during the teaching-related events. In addition, interaction with students was included as a predictor. Predictors were centered within clusters (cwc) and between clusters (cbc) to distinguish and analyze effects at both the within-teacher and between-teacher levels, facilitating the separation of individual teacher effects from broader, between-teacher differences. Participants grand mean centered (cgm) age (years), gender (0 = ‘Woman’, 1 = ‘Man’) and total work experience (years) were also included as predictors.
The need for multilevel modeling (MLM) was tested for each response variable with the misty::multilevel.icc program (Yanagida Citation2022). The results showed that MLM was needed as EDA during teaching-related events had a large (48.6%) variance (due to clusters, i.e. teachers) and emotional activity after teaching-related events had a large (59.8%) variance. Despite the small variance explained by the grouping structure (2.3%) for emotional valence after teaching-related events, we applied an MLM approach to account for response clustering by teacher and to reduce error in the analysis results.
Default priors were used in the regression analyses, as they are weakly informative and offer sufficient regularization to guide model fitting while allowing the data to significantly influence the results, especially given the lack of previous strong prior knowledge. A Bernoulli distribution using a logit link function was used to appropriately handle the binary response variables (‘ValenceAfter’, ‘ActivityAfter’), a Gaussian family with identity link was used for the continuous response variable (‘EDA’), and a Categorical family with logit link was used for the categorical response variable (‘teachEvent’). The default thinning of 1 and a total of 12000 iterations in four chains for the posterior sampling were used for each model. Following MLM practice, each modeling process was started with an unconditional (‘baseline’, ‘null’ or ‘empty’) model, followed by a model where the time component was considered. Next, possible effects of the control variables were examined. Finally, the actual predictor variables were added to the following models one by one. If the new model contained credible effects, it was compared to the previous model using the Pareto Smoothed Importance-Sampling Leave-One-Out cross-validation (PSIS-LOO, Vehtari, Gelman, and Gabry Citation2017) using the difference in expected log predictive density (elpd_diff value and its S.E.) calculated with the loo::loo_compare program (Vehtari et al. Citation2023).
Results
RQ 1: How are prospective and retrospective academic outcome emotions related to teaching, tutoring, planning and assessing student work?
Table 1. Perceived prospective and retrospective academic emotions during teaching-related events (N = 219).
Prospective activating emotions were clearly more common (n = 180, 82.2%) than deactivating emotions (n = 39, 17.8%). This was also the case after the teaching events. Prospective and retrospective activating emotions were quite dominant especially related to the teacher-student interaction events (teaching, tutoring), suggesting support for H1b.
RQ 2: How is EDA related to teaching, tutoring, planning and assessing student work?
To further investigate hypotheses related to RQ 2, Bayesian hierarchical linear models were fitted using a Gaussian family with an identity link function (). Average EDA during teaching-related events was the response variable, and models were developed incrementally, adding predictors to assess different teaching contexts, time, and social interactions during work. The unconditional model (Model 0) included only a random intercept for each teacher, which revealed significant variability in EDA with an average of 68.44 ( [61.81, 75.24]). Time was included as a predictor in Model 1 to consider detrending.
Table 2. Bayesian multilevel models predicting variation in teaching-related events with EDA.
Including the nature of teaching-related events in Model 2 showed that EDA did not significantly differ between these events, with an average value of 66.55 ([59.21, 73.77]). The coefficients for tutoring, planning, and assessing relative to teaching (the reference category) were not credible, providing no support for H2b regarding higher EDA during interactive teaching activities.
Model 3 included overall daily EDA during working hours as a predictor. Results showed that it had a credible positive relationship with event-related EDA (B = 0.82, [0.54, 1.09]), indicating that teachers with generally higher levels of arousal throughout the day also tend to experience higher arousal during teaching activities. This suggests that these teachers’ higher baseline arousal levels extend into specific teaching-related events, rather than teaching-related events themselves causing elevated arousal. Therefore, the relationship reflects a predisposition for higher arousal among certain teachers, rather than an overall elevation during teaching-related events compared to the daily baseline. Consequently, while H2a is supported in the sense that teachers exhibit higher arousal during these events relative to their overall baseline, it is important to note that this is driven by individuals with inherently higher arousal levels.
Model 4 introduced the number of persons present in each event as a predictor to examine the influence of social context on EDA. The results did not support H2c, indicating that the presence of others did not have a consistent effect on EDA during teaching-related events. Interestingly, in this model, EDA was higher during teaching and tutoring (interaction with students) than planning and assessing. However, as the trend was credible only for comparison between teaching and planning (B = −9.87, [−19.46, −0.01]), we cannot suggest even partial support for H2b.
RQ 3a: How is EDA related to emotional valence after teaching-related events?
Table 3. Bayesian logistic multilevel models predicting variation in emotional valence after daily teaching-related events.
In the first, unconditional model (Model 0, ), the intercept estimate was = 2.68 (
[2.01, 3.69]), indicating that the likelihood of experiencing a positive emotion was 14.6 times higher (Odds Ratio,
=
), or 93.6% more probable (
(Positive Emotion) =
/(1+
)
0.936), than the likelihood of experiencing a negative emotion after a teaching-related event. The between-teacher variance in intercepts was
= 0.49 (
[0.22, 1.33]), showing moderate variability in baseline emotional valence among teachers.
Model 1 included time as a predictor for detrending purposes. The coefficient for the time variable was non-credible, indicating that the passage of time did not influence the likelihood of reporting positive emotional valence.
Next, controlling variables such as age, gender, total work experience, and interaction with students were tested but found to have no credible relationship with the response variable. Therefore, they were excluded from further models.
Model 2 added EDA as a predictor, considering both within-teacher and between-teacher variations. The coefficient for within-teacher EDA was credible ( = 0.03,
[0.00, 0.07]), suggesting that higher within-teacher EDA levels during events slightly increased the likelihood of reporting positive emotional valence afterward. The positive
coefficient partially supported H3a (expectation of zero coefficient with equal amount of negative and positive outcome emotions), as the response variables distribution was biased towards positive outcome emotions (
= 201, 91.8%, see ).
Model 3 added emotional valence before the teaching-related event as a predictor. The within-teacher coefficient was credible ( = 1.64,
[0.30, 2.99]), suggesting that positive emotional valence before teaching significantly increased the likelihood of reporting positive emotions afterward. No between-teacher level associations or interactions between predictors were observed.
Model 4 included emotional activity before the teaching-related event as a predictor, but no credible relationship with the response variable was found.
RQ 3b: How is EDA related to emotional activity after teaching-related events?
Table 4. Bayesian logistic multilevel models predicting variation in emotional activity after daily teaching-related events.
The unconditional Model 0 established a baseline understanding of the variance in emotional activity. The intercept estimate was = 1.72 (
[1.12, 2.47]), indicating a general tendency for teachers to report activating emotions after teaching events. The between-teacher variance in intercepts was
= 0.87 (
[0.06, 3.31]), suggesting moderate variability in baseline emotional activity among teachers.
The coefficient of Time (Model 1) was non-credible, indicating that the passage of time did not significantly influence the likelihood of reporting activating emotional activity. However, time was included in the model for detrending.
Next, possible effects of controlling variables were examined. Only the dichotomous teacher-student interaction variable (0 = ‘No interaction’, 1 = ‘Interaction’) had a credible effect as both within-teacher ( = 0.94,
[0.15, 1.78]) and between-teacher (
= 3.04,
[0.74, 5.60]) interactions with students were positively related to emotional activity after teaching-related events. This finding supports the earlier H1b, suggesting that interactive teaching situations (i.e. teaching and tutoring) raise emotional activity. Other controlling variables were found to have no credible effect and were thus excluded from subsequent models.
Adding EDA as a predictor (Model 2), while maintaining the teacher-student interaction as a control, suggested a trend where higher within-teacher EDA levels might increase the likelihood of reporting activating emotional activity after teaching-related events. However, this finding was only close to credible ( = 0.02,
[−0.01, 0.05]), partially supporting H3b.
Introducing emotional activity before teaching-related events as a predictor (Model 3) showed a credible effect ( = 2.70,
[1.60, 3.89]), highlighting that reporting activating emotions before the event increased the likelihood of reporting activating emotions afterward. Interactions between EDA and emotional activity were explored, but no credible interaction effects were identified. Within the teacher level, EDA’s close to credible positive relationship with activating emotional activity after teaching-related events remained in this model.
Adding emotional valence before teaching-related events as a predictor (Model 4) revealed non-credible effects. However, relationship between the within-teacher level EDA and emotional activity after teaching-related events became credible, = 0.03,
[0.00, 0.06].
Discussion
The objective of the study was to advance the understanding of higher education teachers’ emotions and their associations with physiological arousal (EDA) in teaching-related working situations. The study also presented and tested new methods for reporting and physiologically measuring emotions in authentic situations and environments.
The first RQ investigated how HE teachers’ prospective and retrospective outcome emotions differed during everyday teaching-related events involving teaching, tutoring, planning and assessment. Results showed that teaching-related events predominantly led to positive prospective and retrospective emotions. Positive outcomes were more frequent after the events, especially in interactive situations like teaching and tutoring, partially supporting H1a. Activating emotions were more common than deactivating ones, particularly in teacher-student interactions, reinforcing H1b. Interactive teaching situations notably increased emotional activity, emphasizing the role of engagement in fostering positive emotional experiences.
The second RQ investigated how arousal was related to different teaching-related events. Results demonstrated that teaching-related events had higher EDA levels compared to typical working hours, aligning with H2a. This suggests that teaching activities are associated with increased physiological arousal. However, it is important to note that this elevation was primarily driven by teachers who exhibited higher baseline arousal levels throughout the day. There were no significant differences in EDA among different types of teaching-related events (teaching, tutoring, planning, assessing), thus not supporting H2b. The expected higher EDA in interactive events did not materialize. Additionally, the presence of others during these events did not consistently affect EDA levels, which contradicts H2c. These findings imply that while teaching generally elevates EDA, the specific context and social presence have less influence than anticipated at the group level. It is possible that individual differences in stress responses among teachers could obscure group-level effects, suggesting the need for further research into personal variability in stress experiences.
The third RQ investigated the relation between arousal levels and prospective and retrospective outcome valence and activation. Results revealed that within-teacher EDA levels were a credible predictor of emotional valence after teaching, with higher EDA slightly increasing the likelihood of positive emotions. As the response distribution was heavily biased towards positive outcome emotions, this finding supports Hypothesis H3a. Additionally, positive emotional valence before teaching was a strong predictor of positive emotions afterward, emphasizing the significance of pre-existing emotional states. Further, results showed that student interactions during teaching events (i.e. ‘Teaching’ and ‘Tutoring’) and higher pre-teaching emotional activity were significant predictors of activating emotional activity afterward. Also, higher within-teacher EDA levels were (weakly) associated with activating emotional activity outcomes. These findings supported H3b.
As expected, the findings indicated that interaction plays an important role in teachers’ emotions. Interactive work events with students (teaching and tutoring) were mostly related to positive emotions, also supporting previous research that has connected interpersonal relationships to teachers’ joy (Hagenauer, Hascher, and Volet Citation2015). However, while tutoring sessions with students were mostly related to positive emotions, teaching situations were related to both positive and negative emotions. This may be since more people are involved in teaching situations, which makes interactions more complex. A teaching situation includes several factors that may affect the interaction, such as disciplinary issues related to a bigger number of students present (Hagenauer and Volet Citation2014; Hagenauer, Hascher, and Volet Citation2015), choices regarding teaching methods (Kiltz et al. Citation2020; Trigwell Citation2011) or a teacher’s self-efficacy regarding teaching (De Zordo, Hagenauer, and Hascher Citation2019).
The most negative emotions and highest EDA were related to teaching-related planning events. The finding shows the multi-faceted nature of teacher profession in relation to the exploration of emotions. Furthermore, negative emotions were experienced more before than after teaching-related events. These findings are interesting for further research in the field of teacher well-being. Instead of focusing solely on actual teaching situations, there is also a need for a deeper understanding of teachers’ working processes prior to teaching. The period before teaching may cause emotional stimulation that is not yet well recognized, such as how prolonged it is and how it is recovered from. Recovery from higher levels of arousal should be paid more attention to in future studies on teachers’ emotions. In this study, however, the results indicate that some recovery occurs from emotional activity while teachers’ prospective emotions were more activating and retrospective emotions more deactivating.
Teachers’ EDA levels were related to their retrospective emotional activity, supporting the control-value theory of achievement emotions (Pekrun, Marsh, and Elliot Citation2023) and empirical results (e.g. Gomez, Stahel, and Danuser Citation2004) that suggest that emotional activity corresponds to physiological arousal. Furthermore, negative emotions were more related to higher EDA than positive emotions. This connection has also been found in some previous studies (e.g. Harley, Jarrell, and Lajoie Citation2019; Villanueva et al. Citation2018). Teachers’ increased autonomic nervous system activation has been associated with many stressors in particular in actual teaching-related events, such as low student engagement (Junker, Donker, and Mainhard Citation2021), uncertainty, time management and students’ disruptive behavior (Junker and Holodynski Citation2022), teachers’ classroom agency (Donker et al. Citation2020) and teachers’ self-efficacy (Jõgi et al. Citation2023). In the current study, however, high EDA was found to be associated with tutoring and co-operative planning with students or other teachers. Nevertheless, it is consistent with previous research on co-operation raising EDA (Moya-Albiol et al. Citation2013; Sariñana-González, Romero-Martínez, and Moya-Albiol Citation2017). Overall, the data showed that physiological responses were triggered by both positive and negative emotions. When studying emotions in the future, physiological measures will need to be complemented by multi-method settings to gain a better understanding of teachers’ emotions. Qualitative data, for example, would allow a more in-depth understanding of why and how negative and positive emotions are associated with certain situations.
The findings partly supported previous research and demonstrated the emotional nature of teachers’ work. The diversity of teachers’ emotions and how they relate to different work situations, however, requires much more research. In the current data, teaching-related situations were characterised by positive rather than negative teacher emotions which could also be explained by the teachers’ work experience. Therefore, further research should include teachers from different career stages and different fields with diverse teaching traditions.
Limitations
This study has some limitations that need to be acknowledged. First, the sample size was small, so the results must be interpreted with reservations. Future research on teachers’ EDA in authentic teaching situations with larger sample sizes is needed to make further conclusions. Second, because the study was conducted in authentic work situations, the researchers had no control over which events the teachers chose or remembered to report. This may have opened the door to bias through self-selection of reported events. Even though they were carefully instructed to report all teaching-related events, it is possible that some events, such as the ones that included some unexpected factors that stole participants’ attention from the study, may have been missed. In addition, it is possible that the reported events may have included activities from more than one category (planning, teaching, tutoring, assessing). However, we expect the categories to be representative of the activity that played the greatest role during the event. Third, the study applied the ESM via a smart device application, in which teachers could choose only one emotion to best describe their state. Choosing only one emotion is not straightforward. Nevertheless, the teachers’ choice can still be seen as adequate to reflect the levels of valence and activation, which were of interest in this research instead of discrete emotions. In future studies, this could be developed further to include more nuances of the emotions, such as the intensity of emotional states or the action tendency the emotion relates to.
Implications
Multi-method research designs in the study of teacher emotions and the data collected in authentic work situations are important for a more nuanced understandings of the realities of teachers’ work. The inferred cost of work-related stress calls for prevention strategies that aim to detect early warning signs at the workplace (Setz et al. Citation2010). The findings of this research suggest that including physiological measurements in emotion research may provide new approaches particularly regarding hidden experiences or unconscious emotional processes. For instance, Quinlan (Citation2016) reminds us of the several studies of teachers’ emotions that highlight the kinds of emotional rules that make the expression of negative emotions particularly difficult for teachers (e.g. Liljestrom, Roulston, and Demarrais Citation2007; Zhang and Zhu Citation2008) and the fact that these emotional rules need to be identified and challenged when they no longer serve our teaching, our students and ourselves. The negative emotions may also be hidden behind self-reports collected for research purposes, or remain unconscious to teachers themselves, e.g. if high levels of arousal are caused by positive emotions and enthusiasm.
Recordings of physiological arousal can complement experienced emotions and, therefore, provide greater understanding of the connections between them. However, the measurement of physiological arousal can also provide possibilities to assess the physiological stress of teachers, even if no complementary information on emotional valence is available. EDA activation does not directly provide information on whether the user’s experience is positive or negative. In practice, both positively and negatively experienced stress induce similar effects in EDA (Boucsein Citation2012). Nevertheless, stress can have serious effects on one’s health and well-being (Yaribeygi et al. Citation2017), which is why continuous high EDA levels should be treated as warning signals, regardless of their source. This study focused on short-term measurements, but the results may provide some guidance for longer-term measurements in further research. For instance, the findings justify the inclusion of new teacher-related work situations in research that may have previously been under-represented in the scope of teacher work. What happens before the teaching session can be as important for teachers’ psychological and physiological well-being as what happens during the teaching session.
Due to the many complicated components and mechanisms of emotions, there is a need for continuous development of new methodological approaches combining both physiological and self-report data. Considering the sympathetic nature of the EDA and the possibility of obtaining EDA measures via mobile devices, such as wristbands, in real life during prolonged periods of time, more studies on EDA and job stress can be expected to appear in the near future. The recent review study by Horvers et al. (Citation2021), focusing on the use of EDA in learning contexts, has shown that electrodes on the fingers of the non-dominant hand are most promising for measuring EDA. This study has introduced a process of measuring EDA via smart ring devices on the finger, which proved to be a promising method for studying emotions. In addition to being considered a reliable device for research purposes, the data collected by the device allows participants to monitor their own arousal, which both enhances their interest in participating in research and increases their understanding of their own arousal. Furthermore, in addition to the individual perspective on emotions, new technology provides new opportunities for future research to address both intrapersonal and interpersonal processes. For instance, wearable devices enable simultaneous measurements and self-reports of both students’ and teachers’ physiological changes providing a wider perspective on academic emotions.
Supplementary Material
Download MS Word (466.4 KB)Acknowledgements
The authors would like to thank Dr. Sonja Niiranen and Ms. Eija Lehtonen for their valuable help with data collection.
Disclosure statement
No potential conflict of interest was reported by the author(s).
References
- Ba, S., and X. Hu. 2023. “Measuring Emotions in Education Using Wearable Devices: A Systematic Review.” Computers & Education 200: 1–20. https://doi.org/10.1016/j.compedu.2023.104797.
- Balters, S., and M. Steinert. 2017. “Capturing Emotion Reactivity Through Physiology Measurement as a Foundation for Affective Engineering in Engineering Design Science and Engineering Practices.” Journal of Intelligent Manufacturing 28 (7): 1585–607. https://doi.org/10.1007/s10845-015-1145-2.
- Boucsein, W. 2012. Electrodermal Activity. 2nd ed. New York: Springer. https://doi.org/10.1007/978-1-4614-1126-0
- Bürkner, P.-C. 2017. “brms: An R Package for Bayesian Multilevel Models Using Stan.” Journal of Statistical Software 80 (1): 1–28. https://doi.org/10.18637/jss.v080.i01
- Cha, J.S., F. Ausri, L. Mudge, and Y. Denny. 2022. “Effectiveness of Smart Wrist Wearables for Distinguishing Physical and Cognitive Demands.” IISE Transactions on Healthcare Systems Engineering 13 (2): 150–60. https://doi.org/10.1080/24725579.2022.2142867.
- Critchley, H.D. 2002. “Review: Electrodermal Responses: What Happens in the Brain.” The Neuroscientist 8 (2): 132–42. https://doi.org/10.1177/107385840200800209.
- Dawson, M.E., A.M. Schell, and D.L. Filion. 2007. “The Electrodermal System.” In Handbook of Psychophysiology, edited by J. T. Cacioppo, L. G. Tassinary, and G. Berntson, 157–81. Cambridge, UK: Cambridge University Press. https://doi.org/10.1017/CBO9780511546396.007
- De Zordo, L., G. Hagenauer, and T. Hascher. 2019. “Student Teachers’ Emotions in Anticipation of Their First Team Practicum.” Studies in Higher Education 44 (10): 1758–67. https://doi.org/10.1080/03075079.2019.1665321.
- Dickerson, S., and P. Zoccola. 2009. “Towards a Biology of Social Support.” In Oxford Handbook of Positive Psychology, edited by S. Lopez and C. R. Snyder, 2nd ed., 518–26. New York: Oxford University Press. https://doi.org/10.1093/oxfordhb/9780195187243.013.0049
- Donker, M.H., T. van Gog, T. Goetz, A.-L. Roos, and T. Mainhard. 2020. “Associations Between Teachers’ Interpersonal Behavior, Physiological Arousal, and Lesson-Focused Emotions.” Contemporary Educational Psychology 63 (2020): 101906. https://doi.org/10.1016/j.cedpsych.2020.101906.
- Dreer, B. 2023. “On the Outcomes of Teacher Wellbeing: A Systematic Review of Research.” Frontiers in Psychology 14. https://doi.org/10.3389/fpsyg.2023.1205179.
- Elfering, A., and S. Grebner. 2011. “Ambulatory Assessment of Skin Conductivity During First Thesis Presentation: Lower Self-Confidence Predicts Prolonged Stress Response.” Applied Psychophysiology and Biofeedback 36 (2): 93–9. https://doi.org/10.1007/s10484-011-9152-3.
- Gomez, P., W.A. Stahel, and B. Danuser. 2004. “Respiratory Responses During Affective Picture Viewing.” Biological Psychology 67 (3): 359–73. https://doi.org/10.1016/j.biopsycho.2004.03.013.
- Hagenauer, G., T. Hascher, and S.E. Volet. 2015. “Teacher Emotions in the Classroom: Associations with Students’ Engagement, Classroom Discipline and the Interpersonal Teacher-Student Relationship.” European Journal of Psychology of Education 30 (4): 385–403. https://doi.org/10.1007/s10212-015-0250-0.
- Hagenauer, G., and S. Volet. 2014. “‘I Don’t Think I Could, You Know, Just Teach Without Any Emotion’: Exploring the Nature and Origin of University Teachers’ Emotion.” Research Papers in Education 29 (2): 240–62. https://doi.org/10.1080/02671522.2012.754929.
- Harley, J.M., A. Jarrell, and S.P. Lajoie. 2019. “Emotion Regulation Tendencies, Achievement Emotions, and Physiological Arousal in a Medical Diagnostic Reasoning Simulation.” Instructional Science 47 (2): 151–80. https://doi.org/10.1007/s11251-018-09480-z.
- Hascher, T., and J. Waber. 2021. “Teacher Well-Being: A Systematic Review of the Research Literature from the Year 2000–2019.” Educational Research Review 34:100411. https://doi.org/10.1016/j.edurev.2021.100411.
- Hektner, J.M., J.A. Schmidt, and M. Csikszentmihalyi. 2007. Experience Sampling Method: Measuring the Quality of Everyday Life. Thousand Oaks: Sage. https://doi.org/10.4135/9781412984201
- Hoogerheide, V., A. Renkl, L. Fiorella, F. Paas, and T. van Gog. 2018. “Enhancing Example-Based Learning: Teaching on Video Increases Arousal and Improves Problem-Solving Performance.” Journal of Educational Psychology 111 (1): 45–56. https://doi.org/10.1037/edu0000272.
- Horvers, A., N. Tombeng, T. Bosse, A.W. Lazonder, and I. Molenaar. 2021. “Detecting Emotions Through Electrodermal Activity in Learning Contexts: A Systematic Review.” Sensors 21 (23): 7869. https://doi.org/10.3390/s21237869.
- Jõgi, A., A. Aulén, E. Pakarinen, and M. Lerkkanen. 2023. “Teachers’ Daily Physiological Stress and Positive Affect in Relation to Their General Occupational Well-Being.” British Journal of Educational Psychology 93 (1): 368–85. https://doi.org/10.1111/bjep.12561.
- Junker, R., M.H. Donker, and T. Mainhard. 2021. “Potential Classroom Stressors of Teachers: An Audiovisual and Physiological Approach.” Learning and Instruction 75:101495. https://doi.org/10.1016/j.learninstruc.2021.101495.
- Junker, R., and M. Holodynski. 2022. “Teaching Versus Watching? Using Video Feedback to Counter Teacher Stress.” Teacher Development 26 (4): 472–91. https://doi.org/10.1080/13664530.2022.2089221.
- Kiltz, L., R. Rinas, M. Daumiller, M. Fokkens-Bruinsma, and E. Jansen. 2020. “‘When They Struggle, I Cannot Sleep Well Either’: Perceptions and Interactions Surrounding University Student and Teacher Well-Being.” Frontiers in Psychology 11. https://doi.org/10.3389/fpsyg.2020.578378.
- Kreibig, S.D. 2010. “Autonomic Nervous System Activity in Emotion: A Review.” Biological Psychology 84 (3): 394–421. https://doi.org/10.1016/j.biopsycho.2010.03.010.
- Levenson, R.W. 2014. “The Autonomic Nervous System and Emotion.” Emotion Review 6 (2): 100–12. https://doi.org/10.1177/1754073913512003.
- Liljestrom, A., K. Roulston, and K. Demarrais. 2007. “‘There’s No Place for Feeling Like This in the Workplace’: Women Teachers’ Anger in School Settings.” In Emotion in Education, edited by P. A. Schutz and R. Pekrun, 275–91. Burlington, MA: Academic Press. https://doi.org/10.1016/B978-012372545-5/50017-4
- Mendzheritskaya, J., and M. Hansen. 2019. “The Role of Emotions in Higher Education Teaching and Learning Processes.” Studies in Higher Education 44 (10): 1709–11. https://doi.org/10.1080/03075079.2019.1665306.
- Moors, A. 2009. “Theories of Emotion Causation: A Review.” Cognition & Emotion 23 (4): 625–62. https://doi.org/10.1080/02699930802645739.
- Moya-Albiol, L., S. De Andrés-García, M.V. Sanchis-Calatayud, P. Sariñana-González, N. Ruiz-Robledillo, A. Romero-Martínez, and E. González-Bono. 2013. “Psychophysiological Responses to Cooperation: The Role of Outcome and Gender.” International Journal of Psychology 48 (4): 542–50. https://doi.org/10.1080/00207594.2012.666552.
- Muldner, K., W. Burleson, and K. VanLehn. 2010. “‘Yes!’: Using Tutor and Sensor Data to Predict Moments of Delight During Instructional Activities.” In User Modeling, Adaptation, and Personalization, edited by P. De Bra, A. Kobsa, and D. Chin,, vol. 6075, 159–70. Berlin Heidelberg: Springer. https://doi.org/10.1007/978-3-642-13470-8_16
- Nokelainen, P., and A. Aldahdouh. 2020. MergeSR and AnalyseSRLT Software for Longitudinal Psychophysiological and Experience Sampling Data Analysis. Tampere: Tampere University.
- Nokelainen, P., and S. Hartikainen. 2020. LearningTracker Software for Experience Sampling Data Collection. Tampere: Tampere University.
- Pakarinen, T., J. Pietilä, and H. Nieminen. 2019. “Prediction of Self-Perceived Stress and Arousal Based on Electrodermal Activity.” In 41st Annual International Conference of the IEEE Engineering in Medicine and Biology Society (EMBC), 2191–2195. https://doi.org/10.1109/EMBC.2019.8857621
- Pekrun, R. 2006. “The Control-Value Theory of Achievement Emotions: Assumptions, Corollaries and Implications for Educational Research and Practice.” Educational Psychology Review 18 (4): 315–41. https://doi.org/10.1007/s10648-006-9029-9.
- Pekrun, R. 2019. “Inquiry on Emotions in Higher Education: Progress and Open Problems.” Studies in Higher Education 44 (10): 1806–11. https://doi.org/10.1080/03075079.2019.1665335.
- Pekrun, R., T. Goetz, W. Titz, and R.P. Perry. 2002. “Academic Emotions in Students’ Self-Regulated Learning and Achievement: A Program of Qualitative and Quantitative Research.” Educational Psychologist 37 (2): 91–105. https://doi.org/10.1207/S15326985EP3702_4.
- Pekrun, R., H.W. Marsh, A.J. Elliot, et al. 2023. “A Three-Dimensional Taxonomy of Achievement Emotions.” Journal of Personality and Social Psychology 124 (1): 145–78. https://doi.org/10.1037/pspp0000448.
- Postareff, L., and S. Lindblom-Ylänne. 2011. “Emotions and Confidence Within Teaching in Higher Education.” Studies in Higher Education 36 (7): 799–813. https://doi.org/10.1080/03075079.2010.483279.
- Quinlan, K.M. 2016. “How Emotion Matters in Four Key Relationships in Teaching and Learning in Higher Education.” College Teaching 64 (3): 101–11. https://doi.org/10.1080/87567555.2015.1088818.
- R Core Team. 2022. “R: A Language and Environment for Statistical Computing”. R Foundation for Statistical Computing, Vienna, Austria. https://www.R-project.org.
- Salonius, H., P. Kytölä, S. Pakarinen, A. Mäkikangas, and M. Saija. 2024. Associations of continuous electrodermal activity with job burnout and work engagement.” SSRN. https://doi.org/10.2139/ssrn.4698943
- Sariñana-González, P., Á. Romero-Martínez, and L. Moya-Albiol. 2017. “Cooperation Induces an Increase in Emotional Response, as Measured by Electrodermal Activity and Mood.” Current Psychology 36 (2): 366–75. https://doi.org/10.1007/s12144-016-9426-2.
- Setz, C., B. Arnrich, J. Schumm, R. La Marca, G. Tröster, and U. Ehlert. 2010. “Discriminating Stress from Cognitive Load Using a Wearable EDA Device.” IEEE Transactions on Information Technology in Biomedicine 14 (2): 410–7. https://doi.org/10.1109/TITB.2009.2036164.
- Suoja, K., J. Liukkonen, J. Jussila, H. Salonius, N. Venho, V. Sillanpää, V. Vuori, and N. Helander. 2017. “Application for Pre-Processing and Visualization of Electrodermal Activity Wearable Data.” In IFMBE Proceedings, 93–6. Cham: Springer. https://doi.org/10.1007/978-981-10-5122-7_24
- Sutton, R.E., and K.F. Wheatley. 2003. “Teachers’ Emotions and Teaching: A Review of the Literature and Directions for Future Research.” Educational Psychology Review 15 (4): 327–58. https://doi.org/10.1023/A:1026131715856.
- Torniainen, J., B. Cowley, A. Henelius, K. Lukander, and S. Pakarinen. 2015. “Feasibility of an Electrodermal Activity Ring Prototype as a Research Tool.” Proceedings of the Engineering in Medicine and Biology Society (EMBC), 37th Annual International Conference of the IEEE, 6433–6436. https://doi.org/10.1109/EMBC.2015.7319865.
- Trigwell, K. 2011. “Relations Between Teachers’ Emotions in Teaching and Their Approaches to Teaching in Higher Education.” Instructional Science 40 (3): 607–21. https://doi.org/10.1007/s11251-011-9192-3.
- Vehtari, A., J. Gabry, M. Magnusson, Y. Yao, P.-C. Bürkner, T. Paananen, and A. Gelman. 2023. “loo: Efficient leave-one-out cross-validation and WAIC for Bayesian models”. R package version 2.6.0. https://mc-stan.org/loo.
- Vehtari, A., A. Gelman, and J. Gabry. 2017. “Practical Bayesian Model Evaluation Using Leave-One-Out Cross-Validation and WAIC.” Statistics and Computing 27 (5): 1413–32. https://doi.org/10.1007/s11222-016-9696-4.
- Villanueva, I., B.D. Campbell, A.C. Raikes, S.H. Jones, and L.G. Putney. 2018. “A Multimodal Exploration of Engineering Students’ Emotions and Electrodermal Activity in Design Activities.” Journal of Engineering Education 107 (3): 414–41. https://doi.org/10.1002/jee.20225.
- Yanagida, T. 2022. “misty: Miscellaneous Functions”. R package version 0.4.6. https://CRAN.R-project.org/package=misty.
- Yaribeygi, H., Y. Panahi, H. Sahraei, T.P. Johnston, and A. Sahebkar. 2017. “The Impact of Stress on Body Function: A Review.” EXCLI Journal 16:1057–72. https://doi.org/10.17179/EXCLI2017-480.
- Zhang, Q., and W. Zhu. 2008. “Exploring Emotion in Teaching: Emotional Labor, Burnout and Satisfaction in Chinese Higher Education.” Communication Education 57 (1): 105–22. https://doi.org/10.1080/03634520701586310.