ABSTRACT
Avian influenza viruses can cause severe disease in domestic and wild birds and are a pandemic threat. Phylodynamics is the study of how epidemiological, evolutionary, and immunological processes can interact to shape viral phylogenies. This review summarizes how phylodynamic methods have and could contribute to the study of avian influenza viruses. Specifically, we assess how phylodynamics can be used to examine viral spread within and between wild or domestic bird populations at various geographical scales, identify factors associated with virus dispersal, and determine the order and timing of virus lineage movement between geographic regions or poultry production systems. We discuss factors that can complicate the interpretation of phylodynamic results and identify how future methodological developments could contribute to improved control of the virus.
Background
Avian influenza viruses (AIVs) pose a considerable risk to human and animal health (Mostafa et al., Citation2018; Lycett et al., Citation2019; Rimi et al., Citation2019). These viruses belong to the species Alphainfluenzavirus influenzae (previously known as Influenza A virus (IAV)) (Lefkowitz et al., Citation2018; ICTV, Citation2022), and have single-stranded, negative-sense, and eight-segmented RNA genomes (Seiler et al., Citation2018; Rimi et al., Citation2019; Wille & Holmes, Citation2020). AIVs are subtyped into “HxNy” based on the antigenicity and genetic diversity of the two surface glycoproteins: haemagglutinin (H1 – H16 in birds) and neuraminidase (N1 – N9 in birds) (Yoon et al., Citation2014; Blaurock et al., Citation2020; Verhagen, Eriksson, et al., Citation2021). Wild aquatic birds, particularly Anseriformes (e.g. geese, ducks) and Charadriiformes (e.g. gulls, shorebirds), are the primary reservoirs of AIVs (Olsen et al., Citation2006). However, AIVs can spill over to cause sporadic infection or sustained transmission within domestic avian hosts (Mostafa et al., Citation2018; Lycett et al., Citation2019). We can categorize viruses as low pathogenic avian influenza viruses (LPAIVs) and highly pathogenic avian influenza viruses (HPAIVs) based on their pathogenicity in chickens and the presence of insertions in the HA cleavage site (More et al., Citation2017). LPAIVs cause asymptomatic infection or mild disease in domestic birds, thereby harming the poultry industry via decreased egg or meat production, higher vaccination expenses, and trade restrictions (Busani et al., Citation2007; Gonzales et al., Citation2021; Ripa et al., Citation2021). Only LPAIVs of H5 and H7 subtypes are known to evolve into HPAIVs, a process that involves the insertion of multiple basic amino acids in the HA cleavage site (Rott, Citation1992; Alexander, Citation2007). HPAIVs cause severe disease and fatalities in both domestic birds (Verhagen et al., Citation2014; Nuñez & Ross, Citation2019) and wild birds, respectively harming the economy and conservation efforts (Kleyheeg et al., Citation2017; Banyard et al., Citation2022; Lean et al., Citation2022). Several AIV strains can also infect humans and other mammalian species (e.g. swine, seals) and, thus, pose a potential pandemic threat (Ren et al., Citation2016; Nuñez & Ross, Citation2019; Blagodatski et al., Citation2021; Agüero et al., Citation2023; Puryear et al., Citation2023; Vreman et al., Citation2023).
Phylodynamics studies how epidemiological, immunological, and evolutionary processes shape viral genetic diversity. Approaches developed within this framework can help recover viral dispersal patterns and evolutionary processes, even when virus genomic data is sampled relatively sparsely from an infected population (Grenfell et al., Citation2004; Volz et al., Citation2013; Rife et al., Citation2017). Time-scaled phylogenies can be inferred using molecular clock models, which quantify the rate of genetic change over time and therefore enable phylogenetic branch lengths to be expressed as units of time rather than as nucleotide substitutions per site (Drummond et al., Citation2006; Pybus & Rambaut, Citation2009) (Box 1). RNA viruses, including AIVs, typically have exceptionally short generation times, high evolutionary rates, and large population sizes (Duffy, Citation2018; Wille & Holmes, Citation2020). Consequently, genetic substitutions in viral genomes often occur on similar time scales as transmission events between hosts. Hence, it is possible to reconstruct outbreak dynamics from time-scaled phylogenies, as they contain a “molecular footprint” of viral spread (Grenfell et al., Citation2004; Lemey et al., Citation2009; Pybus & Rambaut, Citation2009). When genome sampling location is available, we can use phylodynamic techniques to reconstruct the geographical distribution of viral lineages (“phylogeography”), thereby revealing valuable information about viral spread and factors associated with faster or more frequent viral lineage movement events (Lemey et al., Citation2010; Faria et al., Citation2011; Gill et al., Citation2016).
The most common tools for phylodynamic analyses employ a Bayesian Markov Chain Monte Carlo (MCMC) framework to efficiently explore highly complex models involving many different parameters (Drummond & Rambaut, Citation2007; Bouckaert et al., Citation2014). A Bayesian framework has several advantages compared to the maximum likelihood or parsimony-based approaches. Firstly, Bayesian approaches allow for the incorporation of multiple sources of data or prior knowledge (e.g. divergence times, substitution rates) (Alfaro & Holder, Citation2006; Baele et al., Citation2017; Chakraborty et al., Citation2021). Perhaps more importantly, such approaches generate posterior distributions of phylogenetic trees, thus allowing uncertainty in parameter estimates to be captured (this has been reviewed extensively elsewhere, (e.g. Faria et al., Citation2011; Volz et al., Citation2013; Gill et al., Citation2016; Rasmussen & Grünwald, Citation2020; Dellicour et al., Citation2021). Maximum likelihood (ML)-based methods are more limited in scope of possible analyses, but are often less computationally intensive than the more popular Bayesian approaches (Baele et al., Citation2018; Sagulenko et al., Citation2018; Ishikawa et al., Citation2019). This can be beneficial when dealing with large datasets, limited computed resources, or when faster but lower complexity models are appropriate to inform emergency responses. Such ML phylodynamic methods typically use a single ML tree, enabling faster time-to-answer compared to Bayesian phylodynamic inference.
Recent trending decreases in the cost and time required to generate and analyse virus genetic data have led to rapid innovation within the field of phylodynamics and a subsequent increase in popularity (Gill et al., Citation2016; Rife et al., Citation2017; Grubaugh et al., Citation2019; Cardona-Ospina et al., Citation2021). This review highlights how phylodynamic methods have and may continue to aid the study of AIV spatiotemporal dispersal. We first explore recent studies that use phylodynamics to infer AIV dispersal dynamics within or between wild birds and domestic poultry populations at various geographical scales and discuss factors that can complicate the generation of reliable conclusions. Specifically, we discuss the inference of factors associated with AIV transmission, the order and timing of transmission and lineage dispersal events during an outbreak, and how viral lineages can move between different regions and sectors of poultry production systems. We then consider future challenges and opportunities for using phylodynamic approaches within AIV research.
Wild bird populations
Tracing viral incursions
Phylodynamics has helped identify global movements of AIV lineages in wild bird populations (Baele et al., Citation2018; Zhang et al., Citation2023). Many studies have used time-calibrated phylogenies (Box 1) to estimate the sampling time and location of unobserved viral ancestors, and hence reconstruct the timings and origins of viral (particularly HPAIV) incursions into different wild bird populations (e.g. Lee et al., Citation2018; Hill et al., Citation2019; Zhang, Fan et al., Citation2020; Beerens et al., Citation2021; Liang, Krog, et al., Citation2021; Xie et al., Citation2022). For instance, one study showed that HPAIV H5N8 infections in wild birds present in the Netherlands in late 2020 were likely introductions from wild birds in Egypt and not elsewhere in Europe, as was anticipated based on proximity (Beerens et al., Citation2021). This finding demonstrates how phylogenetic approaches can provide information on AIV spread that might not be detectable using standard epidemiological analyses based on reported cases.
Complications in interpreting where virus lineages originate can arise in instances where gene segments from multiple genetically diverse AIVs reassort during co-infection (Araujo et al., Citation2018; Wille & Holmes, Citation2020; Verhagen, Fouchier et al., Citation2021). Gene segments acquired from each different parent virus typically require analysis using separate phylogenies to capture the different evolutionary histories of each parent lineage (Lu et al., Citation2014). Accordingly, reassortant sequences are sometimes represented using a phylogenetic network instead of a single tree (Frost et al., Citation2015; Stolz et al., Citation2022) or, more commonly, removed, with only one gene segment (usually haemagglutinin (HA) (Heaton et al., Citation2013)) selected for analyses. The latter approach limits our understanding of AIV diffusion (Lu et al., Citation2014; Parvin et al., Citation2014; Frost et al., Citation2015; Venkatesh et al., Citation2018). Although reassortant sequences could perhaps be better accommodated in AIV phylodynamic analyses using a similar approach to that detailed in Müller et al., (Citation2020), which was used to explicitly infer reassortment rates in different human influenza virus lineages, the complexity of this method likely prevents it being used widely (Müller et al., Citation2020).
Geographic structuring of genetic diversity
Phylodynamic methods have been used to investigate whether AIV lineages found in wild birds are structured according to avian flyways and host ecology (Hurt et al., Citation2014; Araujo et al., Citation2018; Mine et al., Citation2019; Sharshov et al., Citation2019; Verhagen et al., Citation2020; Zhang et al., Citation2023). Several studies indicate that avian hosts within geographically isolated regions sometimes harbour AIV segment lineages that are seemingly relatively distinct from other sampled lineages circulating globally (Hansbro et al., Citation2010; Hurt et al., Citation2014; Araujo et al., Citation2018; Wille et al., Citation2022). For instance, one study detected an H11N2 lineage in Adélie penguins (Pygoscelis adeliae) in Antarctica in 2013 that likely diverged approximately between the 1960s-1980s from the most closely related AIV sequences worldwide (Hurt et al., Citation2014). However, it is difficult to determine whether these findings represent true lineage geographic isolation or if lineages only appear geographically structured due to a scarcity of samples from nearby locations that may nevertheless be epidemiologically linked (e.g. southern Chile, Argentina). Other analyses reveal that AIV lineages can be shared between distant regions (Bahl et al., Citation2009; zu Dohna et al., Citation2009; Mine et al., Citation2019; Sharshov et al., Citation2019; Verhagen et al., Citation2020; Caliendo et al., Citation2022). For instance, recent discrete phylogeographic analyses (Box 1) based on the neuraminidase (NA) indicated likely intercontinental AIV dispersal between North America and Eurasia (Mine et al., Citation2019).
Viral phylogeography is the reconstruction of viral movement between geographic locations using virus genetic sequences. Phylogeographic approaches can be categorized based on whether the modelled locations are discrete (e.g. country, administrative district) or continuous (geographic coordinates) (Lemey et al., Citation2009; Faria et al., Citation2011).
Discrete trait analyses are sometimes used out of necessity, for instance, when precise geographic sampling coordinates are unavailable (De Maio et al., Citation2015; Hill et al., Citation2015; Lycett et al., Citation2019). However, these approaches are usefully applied when sequences cluster naturally by geographic location because viral movement is affected by geographical (e.g. oceans, mountains) and/or political (e.g. borders) barriers (Alkhamis et al., Citation2015; De Maio et al., Citation2015; Zhang, Chen et al., Citation2020). Several software packages (e.g. TreeTime (Sagulenko et al., Citation2018), PastML (Ishikawa et al., Citation2019)) have been developed that enable reconstruction of ancestral characters within a maximum-likelihood framework. The most commonly used software packages for phylodynamic inference, BEAST (Drummond & Rambaut, Citation2007) and BEAST2 (Bouckaert et al., Citation2014), rely on a Bayesian inference framework and offer several different approaches for phylogeography using discrete traits. We summarize different maximum likelihood and Bayesian approaches below.
Maximum likelihood ancestral character reconstruction: Maximum likelihood approaches can also be used to estimate the most likely states of discrete traits (e.g. which country or host species) at internal nodes of phylogenies (Cunningham et al., Citation1998; Schmidt & von Haeseler, Citation2009; Hadfield et al., Citation2018; Sagulenko et al., Citation2018; Ishikawa et al., Citation2019). These approaches focus on first estimating the most likely phylogeny given the data (i.e. the maximum likelihood tree), and then estimating the most likely history of discrete states at each node (Cunningham et al., Citation1998; Schmidt & von Haeseler, Citation2009; Sagulenko et al., Citation2018; Ishikawa et al., Citation2019). Branch length or evolutionary time is accounted for, such that geographic movements are more likely to occur on longer branches. This is in contrast to Bayesian approaches implemented in BEAST and BEAST2, which estimate the history of discrete traits for each tree in the posterior tree distribution and hence can more fully account for phylogenetic uncertainty but as a result can be significantly slower (Faria et al., Citation2011; Volz et al., Citation2013; Gill et al., Citation2016; Dellicour et al., Citation2021).
Discrete trait analysis: In one common approach (often known as “discrete trait analysis”, whilst being only one of several approaches for phylogeographic inference using discrete traits), phylogenetic branch locations are estimated using continuous-time Markov chains, i.e. modelled as moving instantaneously at specific rates between a fixed number of discrete locations (Lemey et al., Citation2009; Faria et al., Citation2011). (A). A frequently applied extension of this model known as Bayesian stochastic search variable selection (BSSVS) attempts to limit the number of possible recovered transitions between pairs of locations to those that adequately explain the phylogenetic diffusion process (Lemey et al., Citation2009). Biased sampling can affect the statistical inference of discrete trait analyses as the relative sampling intensities of different discrete locations or traits affect the estimates of viral movements (De Maio et al., Citation2015; Layan et al., Citation2023).
Figure 1. Conceptual representation of common phylogeographic methods. A: Time-calibrated phylogeny estimated with a phylogeographic model with discrete traits. The inferred locations of the ancestral internal nodes (squares) are estimated from the set of discrete locations predefined at the tips (i.e. locations of sampled sequences; circles). B: Discrete trait (e.g. location or host species) analysis. Here, arrows indicate statistically significant virus lineage transitions between bird types. Arrow thickness corresponds to the inferred viral flow rate. C: Time-calibrated phylogeny estimated with continuous phylogeographic inference. The inferred geographic coordinates of the internal nodes (i.e. unsampled virus ancestor) can differ from the geographical sampling coordinates of the sequences at the tips. Both internal nodes and tips are coloured by location. D: Continuous phylogeographic reconstruction using information contained in estimated phylogenies such as C, in which the dots represent the internal and external nodes of the time-scaled phylogeny, coloured according to time. The curvature direction of the lines between dots indicates the inferred direction of viral movement. Coloured polygons represent the statistical uncertainty of the inferred internal node locations, which is derived from a posterior tree distribution.
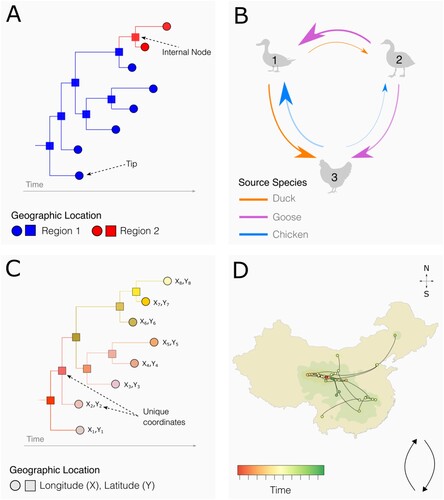
Structured coalescent approaches: Structured coalescent approaches, including those implemented in MASCOT (Müller et al., Citation2018) and BASTA (De Maio et al., Citation2015) within BEAST 2, explicitly model ancestry within and movement between discrete subpopulations, known as “demes” (A) (Vaughan et al., Citation2014; De Maio et al., Citation2015; Müller et al., Citation2018). These methods can be less susceptible to sampling bias than classical coalescent models in certain instances (De Maio et al., Citation2015; Müller et al., Citation2018; Layan et al., Citation2023). However, structured coalescent phylogeographic models are typically more computationally demanding than discrete trait phylogeographic models, and thus are mostly applied to small numbers of demes (De Maio et al., Citation2015; Müller et al., Citation2018; Layan et al., Citation2023). Additionally, although population size can vary between demes, structured coalescent models assume that virus population size in each deme remains constant, which may be less appropriate for investigating AIV lineage expansions in naïve host populations or AIVs with strong seasonality in transmission (De Maio et al., Citation2015; Layan et al., Citation2023). Both discrete trait analyses and structured coalescent approaches can be used to model virus movement between other discrete traits (Faria et al., Citation2011; De Maio et al., Citation2015), such as host species (B) (Ren et al., Citation2016; Hicks et al., Citation2020). Furthermore, generalized linear model (GLM) extensions of these methods (Lemey et al., Citation2012, Citation2014; Faria et al., Citation2013; Müller et al., Citation2019) can be used to evaluate covariates of virus movement, such as avian host density at each location or geographic distance between pairs of locations.
Multitype birth–death models: Like structured coalescent approaches, multitype birth–death (MTBD) models (e.g. as implemented in the BEAST 2 package bdmm (Kühnert et al., Citation2016)) are able to explicitly model transmission within structured populations, and represent an extension of the birth–death models described in Box 2. MTBD models involve the estimation of time-varying “birth” rates (transmission rate), “death” rates (rate of becoming non-infectious or death) for each of several discrete subpopulations, and “per-lineage migration rate” (changes in the subpopulation of an individual due to migration) (FitzJohn, Citation2012; Stadler & Bonhoeffer, Citation2013; Kühnert et al., Citation2016; Barido-Sottani et al., Citation2020). Unlike the structured coalescent approaches, which assume a constant population size within demes, the MTBD allows for population size to change through time (Stadler et al., Citation2015; Seidel et al., Citation2020).
Continuous phylogeography: In the continuous phylogeographic model, virus lineage movement is modelled between geographical coordinates (e.g. latitude and longitude) (C & D) (Lemey et al., Citation2009, Citation2010). Virus lineage movement is most commonly modelled using a relaxed random walk model (Lemey et al., Citation2010), which allows the rate of viral dispersal to vary across the phylogeny. To evaluate spatiotemporal covariates associated with virus dispersal route or velocity, we can conduct post-hoc analysis using the R package “seraphim” (Dellicour et al., Citation2016).
Sampling biases (i.e. disproportionate sampling of virus genomes compared to true infection prevalence) can strongly impact phylogeographic analyses, with under-sampled locations more likely to be inferred as sinks when using discrete trait approaches (De Maio et al., Citation2015; Kalkauskas et al., Citation2021; Layan et al., Citation2023). The international spread of AIV has been predominately inferred from virus genetic data collected in North America and northern Europe (Hill et al., Citation2022). Wild bird sampling remains relatively limited in several areas of Central and South America, Africa, the Middle East, and polar regions, which may result in these regions being overestimated as sink locations for virus lineages (Hurt et al., Citation2014; Araujo et al., Citation2018; Fusaro et al., Citation2019; Naguib et al., Citation2019; Kalonda et al., Citation2020; Hill et al., Citation2021; Lo et al., Citation2022). Accordingly, phylodynamic investigations of the global spread of AIV would benefit from increased AIV genomic surveillance in undersampled locations and host species.
Migratory flyways and mixing zones
Over 50 billion birds are estimated to migrate annually, with most flyways linking breeding grounds at high latitudes and over-wintering sites at low latitudes (Boere et al., Citation2006; Bahl et al., Citation2013). Several different phylodynamic studies using discrete trait analyses (Box 1) report that AIV lineage movement rates are greater within flyways than between flyways in Asia (Central Asian, East Asian) (Tian, Zhou, et al., Citation2015) and in North America (Central, Mississippi, Atlantic) (Scotch et al., Citation2014; Fries et al., Citation2015; Li et al., Citation2018). Continuous phylogeographic analyses (Box 1), in which virus lineage movement is modelled as a diffusion process between geographical coordinates (Lemey et al., Citation2009, Citation2010), demonstrated that H5N1 movement throughout Asia and Russia (1996–2011) correlates with the geographical extent of known flyways (Trovão et al., Citation2015). While a crude simplification to capture avian movement trends, “flyways” appear a crucial facilitator of long-distance AIV spread in wild birds.
Several studies indicate that AIV transmission between birds during congregation at breeding grounds or staging areas where multiple flyways overlap (“mixing zones”) may shape the subsequent global dissemination of AIV lineages (Ramey et al., Citation2010; Gerloff et al., Citation2013; Huang et al., Citation2014; Lee et al., Citation2015; Venkatesh et al., Citation2018; Mine et al., Citation2019; Gass et al., Citation2023). For example, Mine et al. (Citation2019) determined that AIVs sampled in mixing zones in Mongolia and Siberia from wild birds that use different flyways were often phylogenetically intermixed. The study used the Bayesian tip-association significance testing (BaTS) software, which implements post-hoc statistical tests on time-calibrated trees to determine if sequences significantly cluster by a trait, such as sampling location. This approach enables rapid assessment of whether viral genetic diversity at tree tips is geographically structured, but, unlike discrete or continuous phylogeographic approaches, cannot be used to estimate full histories of virus lineage movement. The above-mentioned phylogenetic clustering patterns reported in Mine et al. (Citation2019) are consistent with birds sharing AIVs in mixing zones before dissemination along different flyways, hence enabling long-distance diffusion of viral lineages between multiple continents or regions (Mine et al., Citation2019). Consequently, the authors hypothesized that cross-flyway viral diffusion may have contributed to the simultaneous outbreaks of H5N6 HPAIVs in East Asia and Europe in 2017–2018 (Mine et al., Citation2019). Similar findings have been observed in mixing zones in the Nile Delta and the Republic of Georgia (Gerloff et al., Citation2013; Venkatesh et al., Citation2018).
Several phylodynamic studies have shown high AIV genetic diversity in some mixing zones (Ramey et al., Citation2010; Gerloff et al., Citation2013; Venkatesh et al., Citation2018, Citation2020; Mine et al., Citation2019). High-density congregation of wild birds associated with different flyways at mixing zones may provide ideal conditions for AIV reassortment by increasing the probability of co-infections with diverse genotypes and subtypes (Venkatesh et al., Citation2018; Mine et al., Citation2019). However, further research is required to robustly determine if reassortant AIV genotypes exist at higher frequencies in mixing zones than in non-mixing zones. While phylodynamic approaches have been valuable in demonstrating the role of flyways and mixing zones in structuring AIV dispersal and reassortment patterns, several challenges remain. The scarcity of both wild bird migration data and wild bird sampling in some regions (particularly low- and middle-income countries) limits our ability to fully characterize how migratory flyways impact AIV dispersal patterns globally (Takekawa et al., Citation2010; Palm et al., Citation2015; Tian, Zhou, et al., Citation2015; Fusaro et al., Citation2019; Mine et al., Citation2019; Yong et al., Citation2021; Zhang et al., Citation2023). Secondly, using environmental and flyway data averaged over months or years limits our ability to understand the impacts of changes in weather or environmental conditions on wild bird-mediated AIV dispersal patterns (Kirby et al., Citation2008; Vandegrift et al., Citation2010; Iwamura et al., Citation2013; Bahl et al., Citation2016; Sullivan et al., Citation2018).
Roles of different host taxa
Several studies employing phylodynamic methods demonstrate how movement variation between wild birds from different taxonomic orders might impact AIV dispersal patterns (Ramey et al., Citation2010; Miller et al., Citation2011; Wille et al., Citation2011; Hall et al., Citation2013; Hill et al., Citation2022; Gass et al., Citation2023). In the boreal and temperate territories of the Northern Hemisphere, migratory Charadriiformes (e.g. shorebirds, gulls) are more abundant and undertake long-distance migrations across oceans more frequently than migratory Anseriformes (e.g. ducks, geese) (Ramey et al., Citation2010; Miller et al., Citation2011; Wille et al., Citation2011; Hall et al., Citation2013; Hill et al., Citation2022; Gass et al., Citation2023). Consequently, it has been suggested that Charadriiformes may facilitate intercontinental AIV transmission in these areas more than Anseriformes (Cappelle et al., Citation2012; Gaidet et al., Citation2012; Gaidet, Citation2016; Rimondi et al., Citation2018; Hoye et al., Citation2021; Wille et al., Citation2023). Phylodynamic support for this hypothesis exists in the seemingly more frequent detection of inter-hemispheric reassortment events between American and Eurasian lineages associated with Charadriiformes compared to Anseriformes (Bahl et al., Citation2009; Ramey et al., Citation2010; Wille et al., Citation2011; Van Borm et al., Citation2012; Hall et al., Citation2013; Lang et al., Citation2016). Conversely, in areas such as the tropical regions of West Africa, there is a high abundance of long-distance migratory Anseriformes but a low abundance of Charadriiformes migrants. There, Anseriformes are instead thought to be the primary drivers of virus movement between regions and continents (Cappelle et al., Citation2012; Gaidet, Citation2016).
Discrete trait analyses (Box 1) have been used to explore how wild bird species with contrasting migration behaviours may differently influence AIV spread (Hill et al., Citation2020, Citation2022). For instance, one study suggested that in Egypt and adjacent Black Sea-Mediterranean countries, local migrant species (common shelduck (Tadorna tadorna)) generally contributed to local AIV amplification, while longer distance migrants (northern shoveler (Spatula clypeata) and northern pintail (Anas acuta)) carried AIV lineages over longer distances (Hill et al., Citation2020). Careful analyses and interpretation of results regarding the contribution of different species to AIV dispersal is critical because virus genome sequences are often biased towards hunted species (e.g. game birds) or those that are easily detected when they die (e.g. larger inland birds such as mute swans (Cygnus olor)) (Runstadler et al., Citation2013; Lebarbenchon et al., Citation2015; Bahl et al., Citation2016; Beerens et al., Citation2021; McBride et al., Citation2021; Hill et al., Citation2022). The existence of within-species variation in movement ecology, both between juveniles and adults (van Dijk et al., Citation2014) and between resident and migratory populations from the same species (Lisovski et al., Citation2018), further complicates generalization of host species traits associated with long-distance AIV spread.
Between wild and domestic birds
Frequency and timing of movement
AIVs are regularly transmitted between wild and domestic birds (Fusaro et al., Citation2011; Lebarbenchon & Stallknecht, Citation2011; Bahl et al., Citation2016; Nuñez & Ross, Citation2019). The relative frequency of viral cross-species transmission events can be estimated using phylodynamic tools such as Markov jumps counting in combination with discrete trait phylogeography (Minin & Suchard, Citation2008a, Citationb; Faria et al., Citation2011) (Box 1). Markov jump counting approaches enable the counting of the expected number of transitions between modelled discrete traits, such as country or host species, along phylogenetic branches (Minin & Suchard, Citation2008a, Citationb; Faria et al., Citation2011). For example, Markov jumps counting analyses were used to infer that North America, east and southeast Asia are hotspots for cross-species transmission between wild and domestic birds (Ren et al., Citation2016). Likewise, one large study of a globally sampled H9 AIV dataset used both discrete trait analyses and Markov jump counting to identify spatial asymmetry in the geographical areas where wild-to-domestic and/or domestic-to-wild viral transmission most often occurred (Bahl et al., Citation2016). These methods must be used cautiously because Markov jumps analyses are very sensitive to disproportionate sampling from each group relative to true virus prevalence (Layan et al., Citation2023). This is particularly problematic for AIV, where genome sequences are almost always more frequently available from poultry relative to wild birds (Bahl et al., Citation2016; Ren et al., Citation2016; Yang, Xie et al., Citation2019).
The relative rates of transmission between domestic and wild bird populations can also be investigated using structured coalescent approaches and multitype birth–death models, which may be less sensitive biased sampling (Box 1) (De Maio et al., Citation2015; Grear et al., Citation2017; Yang, Müller, et al., Citation2019; Guinat et al., Citation2022). Such methods can allow the transition rates between populations or demes to vary depending on the direction or estimate a single rate regardless of the directionality (De Maio et al., Citation2015; Kühnert et al., Citation2016; Müller et al., Citation2018; Barido-Sottani et al., Citation2020). One study of a 2014 HPAIV outbreak in North American domestic poultry, caused by wild bird lineage spill-over, inferred that minimal viral movement had occurred between wild and domestic birds over the subsequent course of the domestic outbreak (Grear et al., Citation2017). Furthermore, estimates of basic reproductive number (R0; the expected number of susceptible individuals in a naïve population infected by one infected host – see Box 2) indicated that the poultry outbreak size was stable (R0 ≈ 1) (Grear et al., Citation2017). Taken together, these findings suggested that the poultry outbreak was largely self-sustaining (Grear et al., Citation2017).
Several studies indicate that AIV transmission between wild and domestic birds can vary seasonally (Alarcon et al., Citation2018; Ferenczi et al., Citation2021; Gonzales et al., Citation2021; Zhang et al., Citation2021; Liang, Nissen, et al., Citation2021). Recurrent temporal peaks in cross-species transmission events can be investigated using phylodynamic tools that infer population demographic history (Frost & Volz, Citation2010; Karcher et al., Citation2020) (Box 2). For example, one study observed that the effective population size of clade 2.3.4.4b H5N8 viruses in Chinese poultry, as estimated by a skyride coalescent model (Gill et al., Citation2013) (Box 2), increased during winter 2020/21, as previously observed for H5 lineages/clades during winter 2013/14 and 2016/17 (Zhang et al., Citation2021). Seasonal increases in viral effective population size and other phylogenetic evidence, such as estimated dates of incursion of new lineages, were interpreted as consistent with immigrating wild birds recurrently introducing new H5 viruses into domestic poultry (Zhang et al., Citation2021).
Past population dynamics can be estimated through phylodynamic inference by analysing how virus effective population size (Ne) changes over time. Briefly, the effective population size represents the size of an idealized viral outbreak (i.e. one without selection or population structure) that experiences the same level of genetic drift as the studied population (Magiorkinis et al., Citation2013). Ne estimates are affected by transmission rates and therefore almost always cannot be scaled directly to the number of infected individuals (Frost & Volz, Citation2010). Furthermore, uneven sampling strategies such as focused sampling during transmission peaks can bias the estimation of effective population size (Karcher et al., Citation2020; Parag et al., Citation2020; Cappello & Palacios, Citation2022). Nevertheless, estimates of Ne can provide important information about the viral outbreak dynamics, including capturing seasonality or the possible efficacy of interventions in reducing subsequent outbreak size (Frost & Volz, Citation2010; Rife et al., Citation2017; Drummond et al., Citation2005) (A).
Methods that are commonly employed to estimate population size trajectories over an epidemic include the Bayesian skyline (Drummond et al., Citation2005), the skyride (Minin et al., Citation2008), the birth–death skyline (Stadler et al., Citation2013), and the skygrid (Gill et al., Citation2013).
Several approaches estimate population size history alongside other tree parameters based on the principle that when the population size is small, sampled viruses are more likely to share a common ancestor in the very recent past, and therefore lineages coalesce (join) faster (Drummond et al., Citation2005). Therefore, a faster rate of branch coalescence suggests a comparatively smaller population at that time point (Drummond et al., Citation2005). The Bayesian skyline model requires pre-determination of the number of points at which effective population size can change, generating estimates that are summaries of multiple step-wise changes (Drummond et al., Citation2005). The skyride model does not require predefined points, and introduces a method of temporal smoothing based on the assumption that Ne is correlated across successive coalescent intervals (Minin et al., Citation2008). The skygrid model modifies and extends the skyride model by allowing the Ne trajectory to change at specific time-points pre-specified by the user (Gill et al., Citation2013). A further extension of the skygrid model implements a generalized linear model (GLM) to test how time-varying covariates, such as monthly temperature, are associated with temporal changes in Ne in a method known as “skygrid-GLM” (Gill et al., Citation2016).
The birth–death skyline () provides an alternative method to these coalescent-based approaches (e.g. Bayesian skyline, skyride, and skygrid) (Stadler et al., Citation2013). While in the Bayesian skyline approach only the effective population size changes over time, the birth–death skyline model infers temporal changes in transmission, death/recovery, and sampling rates at discrete intervals alongside variation in population size (Stadler et al., Citation2013). Birth–death models infer a forward-in-time process, starting with the common ancestor of all sampled infections, followed by bifurcating new lineages representing observed and unobserved transmission events, thus generating a tree (Stadler et al., Citation2013). In contrast, the coalescent is modelled backwards-in-time starting from the sampled sequences in the present time until the most recent common ancestor of the sample in the past, with the internal nodes representing the merging or coalescing of two lineages into their most recent common ancestor (i.e. coalescent event) (Drummond et al., Citation2005; Frost & Volz, Citation2010).
Figure 2. Exemplar outputs of phylodynamic methods used to infer past demographic parameters. A: Effective population size (Ne) over time: the solid line is the posterior median estimate for Ne, and the surrounding ribbon indicates the 95% Bayesian credibility interval. The bold vertical dashed line represents the median estimate of the time of the root. Thinner vertical lines indicate the lower (left) and highest (right) values of the 95% highest posterior density interval for the root age. B: Effective reproduction number (Re) over time: the solid line is the posterior median estimate for Re, and the surrounding ribbon indicates the 95% Bayesian highest posterior density interval. The horizontal dashed line indicates Re of 1.
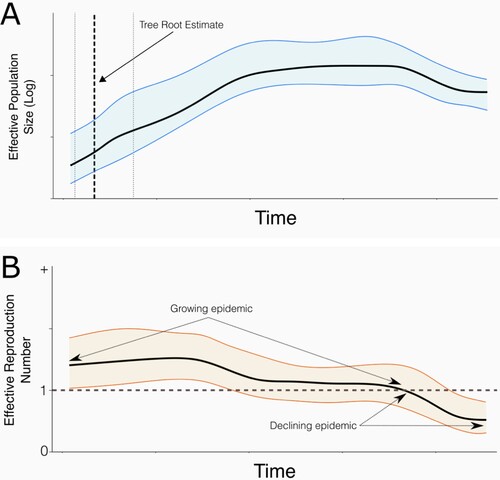
Compared to coalescent approaches, birth–death skyline models can more easily explicitly infer the number of expected secondary infections caused by an infected individual (B), either as a time-varying average (Re: effective reproduction number), or at the start of an outbreak when all individuals are considered as a completely susceptible population (R0: basic reproduction number) (Stadler et al., Citation2012, Citation2013; Volz et al., Citation2013). Changes in effective reproduction number can reflect depletion of the susceptible population as well as control interventions, with Re > 1 indicating a growing epidemic, Re < 1 a declining epidemic, and Re ≈ 1 that the outbreak is stable (Stadler et al., Citation2013; Domingo, Citation2020; Gostic et al., Citation2021).
Factors and settings associated with viral movement
To identify factors associated with higher rates of cross-species AIV transmission or lineage dispersal, phylodynamic analyses previously relied primarily on post-hoc literature searches for relevant events that occurred concurrently with reconstructed viral movements (Vijaykrishna et al., Citation2013). For example, one study suggested that higher rainfall might increase H10 subtype AIV spillover from wild aquatic birds to poultry in Australia because inferred spill-over events occurred more frequently during periods of increased rainfall in the region (Vijaykrishna et al., Citation2013). More recently, the incorporation of GLMs into discrete trait analyses (frequently referred to as “DTA-GLMs”) (Box 1) (Lemey et al., Citation2012, Citation2014; Gill et al., Citation2016) has enabled hypotheses to be formally tested during the phylogenetic estimation process (Beard et al., Citation2014). For instance, a DTA-GLM showed that H5N1 spill-over from wild birds to poultry in Egypt tended to occur in locations with a higher density of birds and humans, higher elevation, and several meteorological variables (Magee et al., Citation2015).
To implement effective infection control, it is necessary to understand the settings in which AIVs move between wild and domestic birds. AIV outbreaks frequently occur in Asian wetlands or rice fields where free-grazing ducks are reared at high density and in close contact with wild birds, suggesting that these areas may support transmission between wild and domestic birds (Hulse-Post et al., Citation2005; Martin et al., Citation2011; Cappelle et al., Citation2014; Prosser et al., Citation2016; Sullivan et al., Citation2018). Several phylodynamic analyses have supported this epidemiological finding, for example, indicating that the spread and maintenance of H5N8 lineages in the Republic of Korea were positively associated with regions of high wild waterfowl immigration and domestic duck density (Hill et al., Citation2015). Low precision of metadata on sampled locations of virus sequences often limits analyses to considering predictors at district-level scales, making it harder to account for local heterogeneity in ecological suitability for wild birds or farming intensity (Hill et al., Citation2015; Bahl et al., Citation2016; Hill et al., Citation2020). Furthermore, these methods have not been frequently applied in regions such as Africa and the Middle East where AIV genomic surveillance is limited (Naguib et al., Citation2019; Ayala et al., Citation2020; Kalonda et al., Citation2020).
Between avian and mammalian species
HPAIVs and LPAIVs can occasionally infect humans and therefore are a public health threat (Webster et al., Citation1992; Li et al., Citation2019; Lycett et al., Citation2019). Epidemiological studies suggest that most infected patients had recent exposure to live poultry or had visited live-bird markets, rather than exposure to wild birds or non-avian species (Zhou et al., Citation2013; Yu et al., Citation2014; Yang et al., Citation2017; Li et al., Citation2019; Oliver et al., Citation2022). Phylogenetic analyses of virus genomes from humans and birds have been frequently used to support that poultry were the likely origin (Liu et al., Citation2013; Joseph et al., Citation2017; Yang et al., Citation2017; Zhang et al., Citation2021). Sporadic AIV transmission from birds to mammals can, in theory, select for variants that have increased transmissibility in mammals (Balzli et al., Citation2016; Bourret, Citation2018; Nuñez & Ross, Citation2019; Zhao et al., Citation2019) and therefore potentially increase pandemic risk (Nelson et al., Citation2012; Bourret, Citation2018; Bravo-Vasquez et al., Citation2020). Phylogenetic analyses have identified multiple AIV lineages associated with cross-species transmission from birds to swine or other mammals (Bodewes et al., Citation2016; Ramey et al., Citation2017; Zhao et al., Citation2019; Chauhan & Gordon, Citation2021; Rijks et al., Citation2021). These transmission events sometimes result in sustained transmission, particularly within swine populations (Nelson et al., Citation2015; Bourret, Citation2018). For example, a phylodynamic study used time-calibrated phylogenies to determine that H10N7 viruses that caused significant mortality in harbour seals (Phoca vitulina) in 2014 in Europe were closely related to various avian-origin H10N7 viruses detected in wild birds in the Netherlands (Bodewes et al., Citation2015, Citation2016). More recently, H5Nx clade 2.3.4.4b viruses in red foxes and wild birds in Europe (the Netherlands) were found to be closely genetically related (Rijks et al., Citation2021). Our understanding of the frequency of spill-over to, and dissemination within, mammalian species is limited by the sparseness of virus genomic surveillance in these taxa (Runstadler et al., Citation2013; Bodewes et al., Citation2016; Ren et al., Citation2016).
Domestic poultry populations
HPAIV origins and diversification
HPAIVs pose a severe problem for the poultry industry, and understanding their origins could help predict and prevent future outbreaks (Nuñez & Ross, Citation2019; Beerens et al., Citation2021). Time-calibrated phylogenies of HA show that multiple H5 and H7 HPAIV genotypes have independently evolved from ancestral LPAIV viruses, potentially facilitated by high poultry density and contact rates within intensive farming systems (Monne et al., Citation2014; Dhingra et al., Citation2018; Seekings et al., Citation2018; Escalera-Zamudio et al., Citation2020). Phylogeographic investigations have tracked the geographic origins of multiple HPAIV genotypes, and in rare cases, even suggested a possible source farm (Monne et al., Citation2014; Dhingra et al., Citation2018; Seekings et al., Citation2018).
Geographic spread within poultry populations
Discrete trait analyses (Box 1) have frequently been employed to reconstruct AIV spatial spread within domestic poultry populations (Jin et al., Citation2014; Bahl et al., Citation2016; Zhang et al., Citation2020; Harvey et al., Citation2021). For example, southern China was shown to be an epicentre for the national spread of H5N6 HPAIV in poultry from 2013 to 2017 (Zhang et al., Citation2020) and was identified as a potential source of a national wave of H9N2 infections in domestic birds (Jin et al., Citation2014).
Some studies have employed structured coalescent models (Box 1) to reconstruct AIV spatial spread in domestic poultry (Yang et al., Citation2019; Hicks et al., Citation2020). Such analyses have shown that viral diffusion tended to occur within, rather than between, North American states during an H5N2 outbreak (Hicks et al., Citation2020). However, as previously described, sampling bias or the presence of unsampled locations (Box 1) can be problematic for discrete trait phylogeographic models, and results must be interpreted with caution given regional differences in AIV genomic surveillance capacity (De Maio et al., Citation2015; Layan et al., Citation2023). LPAIV surveillance is particularly challenging because infections rarely cause severe disease in poultry and can easily be missed (Hurt et al., Citation2014; Parvin et al., Citation2020). Although HPAIV is likely easier to detect due to its higher pathogenicity, in some countries farmers may avoid reporting HPAIV cases for fear that birds will be culled without financial compensation (Chattopadhyay et al., Citation2018; Parvin et al., Citation2020; Moyen et al., Citation2021; Ripa et al., Citation2021).
Phylogeographic analysis can be performed using continuous models (Box 1) in which the spread of viruses is modelled using geographical coordinates (Lemey et al., Citation2009, Citation2010). Continuous phylogeographic analyses can be preferable to discrete analyses when it is beneficial to understand virus spread in both sampled and intermediate unsampled locations (Box 1) (Lemey et al., Citation2009, Citation2010). Continuous phylogeographic analysis indicated that the HPAIV H5N1 movement in Java in 2003 was characterized by short-range dispersal events interspersed with occasional long-range movements (Lam et al., Citation2012). Similar approaches were also used to show that short-distance viral movement was more common than long-distance movements during Italy's 2016–17 HPAI H5N8 epidemic, with the first outbreak wave generally restricted to the north-eastern areas of the country (Harvey et al., Citation2021). Biased sampling between geographical locations can result in a failure to determine the true origin of the outbreak and in the underestimation of viral diffusion rates into an oversampled region from an undersampled area when using continuous phylogeographic methods (Hill et al., Citation2021; Kalkauskas et al., Citation2021). The incorporation of sequence-free samples from affected yet unsampled areas may somewhat alleviate the effect of sampling bias in continuous phylogeographic analyses (as proposed in Kalkauskas et al. (Citation2021)). However, this approach requires a prior understanding of the spatial distribution of outbreaks.
Drivers of dispersal
Understanding which species or breeds are most important for maintaining AIV within poultry systems can allow for the preferential targeting of surveillance and control efforts toward certain host types (Hill et al., Citation2015; Barman et al., Citation2017; Hicks et al., Citation2020; Youk et al., Citation2020; Harvey et al., Citation2021). A study using Markov jump and reward analysis showed that, in live bird markets in the Republic of Korea, the transmission rate of H9N2 from domestic ducks to chickens was higher than the rate in the opposite direction (Youk et al., Citation2020). Similarly, structured coalescent approaches (Box 1) demonstrated that the H5N2 viral transmission rates from layer chicken to turkey populations were higher than the reverse during a 2014–2015 outbreak in North America (Hicks et al., Citation2020).
Phylogeographic analyses helped identify factors associated with AIV spread in domestic birds (Yang, Müller, et al., Citation2019; Dellicour, Lemey, et al., Citation2020; Dellicour, Lequime, et al., Citation2020; Hicks et al., Citation2020), thus highlighting which components of the production system may be most affected in future outbreaks. DTA-GLMs (Box 1) are commonly used for this purpose and have identified several economic and agricultural factors significantly associated with AIV dispersal in domestic birds in China (such as poultry population density, freight transportation, the number of markets selling poultry or poultry products, and high human density) (Lu et al., Citation2017). When both precise location and detailed geographical environmental data are available, we can also investigate factors associated with viral dispersal in a continuous phylogeographic analysis using the R package “seraphim” (Dellicour et al., Citation2016) (Box 1). For example, this approach identified weak support for the association of several spatial factors (e.g. human, chicken and duck population densities, inaccessibility, savanna) with heterogeneity in lineage dispersal velocity of H5N1 in the Mekong region (Cambodia, Laos, Thailand, Vietnam) (Dellicour, Lemey, et al., Citation2020).
The impact of commercial poultry movement networks on AIV dissemination in domestic birds represents an important, but particularly challenging, area of research (Lu et al., Citation2017; Yang et al., Citation2020; Moyen et al., Citation2021). Poultry trading records are difficult to obtain on a large scale, especially in low- and middle-income countries where poultry production systems can be highly complex and dynamic, and where these data are not already routinely collected (Yang et al., Citation2020; Moyen et al., Citation2021). As such, most phylogeographic studies have used proxies for poultry trade networks (Lu et al., Citation2017; Yang et al., Citation2020). For example, one study used DTA-GLMs (Box 1) to show that a proxy network of poultry transportation in China, as determined by a gravity model built from metrics of domestic poultry production and egg production, was positively associated with the large-scale movement of three AIV subtypes (H5N1, H7N9, and H5N6) (Yang et al., Citation2020). Greater availability of accurate poultry trade data would likely allow for more rigorous assessment of the impact of poultry movements on AIV dispersal (Parvin et al., Citation2020; Yang et al., Citation2020; Moyen et al., Citation2021).
Across borders
Although AIV dispersal in domestic poultry typically occurs within countries, several phylodynamic studies have investigated lineage movements across borders (Melville & Shortridge, Citation2006; Yang, Müller et al., Citation2019; Yang, Xie et al., Citation2019). The occurrence of long-distance trade-facilitated AIV movements was highlighted by a 2004 report that linked the 1500 km spread of HPAIV H5N1 from Lanzhou (Gansu Province, Northwest China) to Lhasa (Tibetan Autonomous Region) to the transport of domestic birds (Melville & Shortridge, Citation2006). More recently, studies have begun to explore larger-scale factors that can drive interprovincial or international virus movement (Yang, Müller et al., Citation2019; Yang, Xie, et al., Citation2019). Through a GLM extension of a structured coalescent model (Box 1), one study identified annual levels of international live poultry trade between countries and national poultry production as predictors of cross-border H9N2 virus movement in domestic Asian birds (Yang, Chowdury et al., Citation2019). Illegal trade may also drive the international spread of AIV. However, phylodynamic investigations are limited in their ability to investigate the impact of illegal bird trade on the global dispersal of AIV, as this predictor cannot be easily quantified (Tian, Zhou, et al., Citation2015; Yang, Müller, et al., Citation2019; Yang, Xie, et al., Citation2019).
5.5. Evaluating control efforts
In addition to helping guide the design of novel measures aimed at tackling AIV spread, phylodynamic analyses have been used to evaluate the efficacy of previously implemented control measures (Lee et al., Citation2014; Tian, Cui, et al., Citation2015; Nickbakhsh et al., Citation2016; Kwon et al., Citation2020; Chakraborty et al., Citation2022). By incorporating time-varying predictors into a GLM extension of a structured coalescent phylogeographic model (Box 1), one study showed that duck culling in France likely reduced the spread of HPAIV H5N8 between French administrative divisions (Chakraborty et al., Citation2022). Several studies have also employed phylodynamic methods that infer past population demographic dynamics to explore the effectiveness of control measures (Lee et al., Citation2014; Tian, Cui, et al., Citation2015; Kwon et al., Citation2020). For example, Kwon et al. (Citation2020) argued that an observed fall in H5N1 virus effective population size in Bangladesh over 3 years was due to reduced virus prevalence in domestic birds following the concurrent introduction of wide-scale vaccination, although alternative explanations are possible (Kwon et al., Citation2020). Likewise, one study used estimates of effective reproduction numbers (Box 2) to infer that HPAI-targeted control measures (e.g. culling of infected flocks, pre-emptive culling of neighbouring flocks) introduced in Italy in 2000 successfully slowed the epidemic growth of a novel HPAI outbreak but not that of its LPAI progenitor lineage (Nickbakhsh et al., Citation2016).
Outlook
Whilst this review highlights where phylodynamic analyses have contributed significantly to our understanding of AIV over the last few decades, we identify several areas that can aid future progress of the field. First, we can benefit from the wide range of new approaches developed and extended during the COVID-19 pandemic. For example, methods that enable accessible, “real-time” and easily scalable incorporation of sequences into viral phylogenies (e.g. Nextstrain (Hadfield et al., Citation2018)) could be used more extensively to support surveillance, and methods that incorporate host travel history within phylogeographic analyses could be explored to accommodate bird migration histories (Lemey et al., Citation2020).
Despite many recent methodological advances, formally integrating different data types within phylodynamic analyses remains a key challenge (Frost et al., Citation2015; Baele et al., Citation2017). Approaches that can better integrate temporally varying environmental and demographic data alongside genetic sequences would be extremely valuable to study how bird movements drive AIV lineage spread. Ideally, such models would be developed to handle a broad array of new data types, including bird tracking data from mobile global positioning systems (e.g. GPS-3G-Bluetooth technologies developed to investigate bird spatial behaviour (Yu et al., Citation2022)), satellite imagery of high-risk locations for AIV transmission between wild and domestic species, and poultry trade data. In locations where it is difficult for authorities to access trading records comprehensively, purpose-built apps could facilitate collection of more complete poultry trade network data for use in such new models (Ravindran, Citation2021; Grubaugh et al., Citation2019; Lycett et al., Citation2019; Cardona-Ospina et al., Citation2021). The development of methods that effectively accommodate reassortment in phylodynamic analyses in a user-friendly fashion would help better understand AIV spread (Frost et al., Citation2015; Lycett et al., Citation2019; Verhagen, Fouchier, et al., Citation2021).
It is essential to improve AIV genome sequence and metadata availability (Kalkauskas et al., Citation2021; Layan et al., Citation2023). Missing metadata (such as date of collection, host type or species, precise location for wild birds, or production context for domestic poultry) reduces the value of virus genomes within phylodynamics. Likewise, as discussed throughout this review, sampling biases increase the risk of drawing incorrect conclusions from phylodynamic analyses. It is therefore important to grow capacity for AIV genomic monitoring in currently under-represented countries and sampling from understudied species.
Finally, tackling challenges related to feasibility and resource-intensity is essential for enhancing phylodynamic studies of AIVs. At present, and particularly when dealing with large genomic datasets, a significant level of technical expertise and robust computing infrastructure is often required to formulate appropriate phylodynamic models, execute them, and interpret their results (Duchene et al., Citation2018; Sagulenko et al., Citation2018; Attwood et al., Citation2022). As such, the continued development of faster tools (e.g. recently introduced ML methods (Sagulenko et al., Citation2018; Ishikawa et al., Citation2019)) and the advancement of computational packages that allow existing tools to make more effective use of available computer hardware (e.g. BEAGLE (Ayres et al., Citation2012)) are critical, especially in more resource-constrained environments and where results are intended to inform emergency responses (Suchard & Rambaut, Citation2009; Baele et al., Citation2019). The use of cloud computing in phylodynamic analyses, which was critical for handling the unprecedently large genomic datasets produced the COVID-19 pandemic, could be beneficial in helping researchers handle increased numbers of genome sequences. Greater investment in appropriate training and computational infrastructure in many lower- and middle-income countries would improve global accessibility of phylodynamic approaches (Rife et al., Citation2017; Hill et al., Citation2021; Attwood et al., Citation2022).
Conclusions
AIVs can severely harm domestic and wild birds, and their effective control in birds can help protect the health of humans and other mammalian species. Phylodynamic approaches can be insightful in reconstructing the spatiotemporal dispersal of AIVs, with models capable of analysing viral diffusion within and between different host populations and locations. However, limitations in phylodynamic models exist when key metadata are missing, virus genomic sampling is uneven, and for analysing reassortant viruses. Addressing these challenges will be important to further fulfil the potential of phylodynamic analyses to improve human and animal health.
Additional Information
Author contributions: L.C. wrote the manuscript. S.C.H., G.F., and J.R, edited the manuscript and supervised the work.
Disclosure Statement
No potential conflict of interest was reported by the authors.
Additional information
Funding
References
- Agüero, M., Monne, I., Sánchez, A., Zecchin, B., Fusaro, A., Ruano, M.J., Del Valle Arrojo, M., Fernández-Antonio, R., Souto, A.M., Tordable, P., Cañás, J., Bonfante, F., Giussani, E., Terregino, C. & Orejas, J.J. (2023). Highly pathogenic avian influenza A(H5N1) virus infection in farmed minks, Spain, October 2022. European Communicable Disease Bulletin, 28, 2300001. Sweden.
- Alarcon, P., Brouwer, A., Venkatesh, D., Duncan, D., Dovas, C.I., Georgiades, G., Monne, I., Fusaro, A., Dan, A., Śmietanka, K., Ragias, V., Breed, A.C., Chassalevris, T., Goujgoulova, G., Hjulsager, C.K., Ryan, E., Sánchez, A., Niqueux, E., Tammiranta, N., Zohari, S., Stroud, D.A., Savić, V., Lewis, N.S. & Brown, I.H. (2018). Comparison of 2016–17 and previous epizootics of highly pathogenic avian influenza H5 Guangdong lineage in Europe. Emerging Infectious Diseases, 24, 2270–2283.
- Alexander, D.J. (2007). An overview of the epidemiology of avian influenza. Vaccine, 25, 5637–5644.
- Alfaro, M.E. & Holder, M.T. (2006). The posterior and the prior in Bayesian phylogenetics. Annual Review of Ecology, Evolution, and Systematics, 37, 19–42.
- Alkhamis, M.A., Moore, B.R. & Perez, A.M. (2015). Phylodynamics of H5N1 highly pathogenic avian influenza in Europe, 2005–2010: potential for molecular surveillance of new outbreaks. Viruses, 7, 3310–3328.
- Araujo, J., Petry, M.V., Fabrizio, T., Walker, D., Ometto, T., Thomazelli, L.M., Scherer, A.L., Serafini, P.P., Neto, I.S., Krauss, S., Webster, R.G., Webby, R.J. & Durigon, E.L. (2018). Migratory birds in southern Brazil are a source of multiple avian influenza virus subtypes. Influenza and Other Respiratory Viruses, 12, 220–231.
- Attwood, S.W., Hill, S.C., Aanensen, D.M., Connor, T.R. & Pybus, O.G. (2022). Phylogenetic and phylodynamic approaches to understanding and combating the early SARS-CoV-2 pandemic. Nature Reviews Genetics, 23, 547–562.
- Ayala, A.J., Yabsley, M.J. & Hernandez, S.M. (2020). A review of pathogen transmission at the backyard chicken–wild bird interface. Frontiers in Veterinary Science, 7, 539925.
- Ayres, D.L., Darling, A., Zwickl, D.J., Beerli, P., Holder, M.T., Lewis, P.O., Huelsenbeck, J.P., Ronquist, F., Swofford, D.L., Cummings, M.P., Rambaut, A. & Suchard, M.A. (2012). BEAGLE: an application programming interface and high-performance computing library for statistical phylogenetics. Systematic Biology, 61, 170–173.
- Baele, G., Ayres, D.L., Rambaut, A., Suchard, M.A. & Lemey, P. (2019). High-performance computing in Bayesian phylogenetics and phylodynamics using BEAGLE BT - evolutionary genomics: statistical and computational methods. In M. Anisimova (Ed.), (pp. 691–722). New York: Springer.
- Baele, G., Dellicour, S., Suchard, M.A., Lemey, P. & Vrancken, B. (2018). Recent advances in computational phylodynamics. Current Opinion in Virology, 31, 24–32.
- Baele, G., Suchard, M.A., Rambaut, A. & Lemey, P. (2017). Emerging concepts of data integration in pathogen phylodynamics. Systematic Biology, 66, e47–e65. Oxford University Press.
- Bahl, J., Krauss, S., Kühnert, D., Fourment, M., Raven, G., Pryor, S.P., Niles, L.J., Danner, A., Walker, D., Mendenhall, I.H., Su, Y.C.F., Dugan, V.G., Halpin, R.A., Stockwell, T.B., Webby, R.J., Wentworth, D.E., Drummond, A.J., Smith, G.J.D. & Webster, R.G. (2013). Influenza A virus migration and persistence in North American wild birds. PLoS Pathogens, 9, e1003570.
- Bahl, J., Pham, T.T., Hill, N.J., Hussein, I.T.M., Ma, E.J., Easterday, B.C., Halpin, R.A., Stockwell, T.B., Wentworth, D.E., Kayali, G., Krauss, S., Schultz-Cherry, S., Webster, R.G., Webby, R.J., Swartz, M.D., Smith, G.J.D. & Runstadler, J.A. (2016). Ecosystem interactions underlie the spread of avian influenza a viruses with pandemic potential. PLoS Pathogens, 12, e1005620.
- Bahl, J., Vijaykrishna, D., Holmes, E.C., Smith, G.J.D. & Guan, Y. (2009). Gene flow and competitive exclusion of avian influenza A virus in natural reservoir hosts. Virology, 390, 289–297.
- Balzli, C., Lager, K., Vincent, A., Gauger, P., Brockmeier, S., Miller, L., Richt, J.A., Ma, W., Suarez, D. & Swayne, D.E. (2016). Susceptibility of swine to H5 and H7 low pathogenic avian influenza viruses. Influenza and Other Respiratory Viruses, 10, 346–352.
- Banyard, A.C., Lean, F.Z.X., Robinson, C., Howie, F., Tyler, G., Nisbet, C., Seekings, J., Meyer, S., Whittard, E., Ashpitel, H.F., Bas, M., Byrne, A.M.P., Lewis, T., James, J., Stephan, L., Lewis, N.S., Brown, I.H., Hansen, R.D.E. & Reid, S.M. (2022). Detection of highly pathogenic avian influenza virus H5N1 clade 2.3.4.4b in great skuas: a species of conservation concern in Great Britain. Viruses, 14, 212.
- Barido-Sottani, J., Vaughan, T. & Stadler, T. (2020). A multitype birth–death model for Bayesian inference of lineage-specific birth and death rates. Systematic Biology, 69, 973–986.
- Barman, S., Marinova-Petkova, A., Hasan, M.K., Akhtar, S., El-Shesheny, R., Turner, J.C., Franks, J., Walker, D., Seiler, J., Friedman, K., Kercher, L., Jeevan, T., Darnell, D., Kayali, G., Jones-Engel, L., McKenzie, P., Krauss, S., Webby, R.J., Webster, R.G. & Feeroz, M.M. (2017). Role of domestic ducks in the emergence of a new genotype of highly pathogenic H5N1 avian influenza A viruses in Bangladesh. Emerging Microbes & Infections, 6, e72.
- Beard, R., Magee, D., Suchard, M.A., Lemey, P. & Scotch, M. (2014). Generalized linear models for identifying predictors of the evolutionary diffusion of viruses. AMIA Joint Summits on Translational Science Proceedings. AMIA Joint Summits on Translational Science, 2014, 23–28. American Medical Informatics Association.
- Beerens, N., Heutink, R., Harders, F., Roose, M., Pritz-Verschuren, S.B.E., Germeraad, E.A. & Engelsma, M. (2021). Incursion of novel highly pathogenic avian influenza A(H5N8) virus, the Netherlands, October 2020. Emerging Infectious Diseases, 27, 1750–1753.
- Blagodatski, A., Trutneva, K., Glazova, O., Mityaeva, O., Shevkova, L., Kegeles, E., Onyanov, N., Fede, K., Maznina, A., Khavina, E., Yeo, S.-J., Park, H. & Volchkov, P. (2021). Avian influenza in wild birds and poultry: dissemination pathways, monitoring methods, and virus ecology. Pathogens, 10, 630.
- Blaurock, C., Scheibner, D., Landmann, M., Vallbracht, M., Ulrich, R., Böttcher-Friebertshäuser, E., Mettenleiter, T.C. & Abdelwhab, E.M. (2020). Non-basic amino acids in the hemagglutinin proteolytic cleavage site of a European H9N2 avian influenza virus modulate virulence in turkeys. Scientific Reports, 10, 21226.
- Bodewes, R., Bestebroer, T.M., van der Vries, E., Verhagen, J.H., Herfst, S., Koopmans, M.P., Fouchier, R.A.M., Pfankuche, V.M., Wohlsein, P., Siebert, U., Baumgärtner, W. & Osterhaus, A.D.M.E. (2015). Avian influenza A(H10N7) virus-associated mass deaths among harbor seals. Emerging Infectious Diseases, 21, 720–722.
- Bodewes, R., Zohari, S., Krog, J.S., Hall, M.D., Harder, T.C., Bestebroer, T.M., van de Bildt, M.W.G., Spronken, M.I., Larsen, L.E., Siebert, U., Wohlsein, P., Puff, C., Seehusen, F., Baumgärtner, W., Härkönen, T., Smits, S.L., Herfst, S., Osterhaus, A.D.M.E., Fouchier, R.A.M., Koopmans, M.P. & Kuiken, T. (2016). Spatiotemporal analysis of the genetic diversity of seal influenza A(H10N7) virus, Northwestern Europe. Journal of Virology, 90, 4269–4277.
- Boere, G.C., Galbraith, C.A. & Stroud, D.A. (2006). Waterbirds around the world: a global overview of the conservation, management and research of the world’s waterbird flyways. https://searchworks.stanford.edu/view/6969576
- Bouckaert, R., Heled, J., Kühnert, D., Vaughan, T., Wu, C.-H., Xie, D., Suchard, M.A., Rambaut, A. & Drummond, A.J. (2014). BEAST 2: a software platform for Bayesian evolutionary analysis. PLoS Computational Biology, 10, e1003537.
- Bourret, V. (2018). Avian influenza viruses in pigs: an overview. Veterinary Journal, 239, 7–14.
- Bravo-Vasquez, N., Yao, J., Jimenez-Bluhm, P., Meliopoulos, V., Freiden, P., Sharp, B., Estrada, L., Davis, A., Cherry, S., Livingston, B., Danner, A., Schultz-Cherry, S. & Hamilton-West, C. (2020). Equine-like H3 avian influenza viruses in wild birds, Chile. Emerging Infectious Diseases, 26, 2887–2898. Centers for Disease Control and Prevention.
- Busani, L., Dalla Pozza, M., Bonfanti, L., Toson, M., Ferrè, N. & Marangon, S. (2007). Intervention strategies for low-pathogenic avian influenza control in Italy. Avian Diseases, 51, 470–473.
- Caliendo, V., Lewis, N.S., Pohlmann, A., Baillie, S.R., Banyard, A.C., Beer, M., Brown, I.H., Fouchier, R.A.M., Hansen, R.D.E., Lameris, T.K., Lang, A.S., Laurendeau, S., Lung, O., Robertson, G., van der Jeugd, H., Alkie, T.N., Thorup, K., van Toor, M.L., Waldenström, J., Yason, C., Kuiken, T. & Berhane, Y. (2022). Transatlantic spread of highly pathogenic avian influenza H5N1 by wild birds from Europe to North America in 2021. Scientific Reports, 12, 11729.
- Cappelle, J., Servan de Almeida, R., Fofana, B., Dakouo, M., Balança, G., Gil, P., Albina, E. & Gaidet, N. (2012). Circulation of avian influenza viruses in wild birds in Inner Niger Delta, Mali. Influenza and Other Respiratory Viruses, 6, 240–244.
- Cappelle, J., Zhao, D., Gilbert, M., Nelson, M.I., Newman, S.H., Takekawa, J.Y., Gaidet, N., Prosser, D.J., Liu, Y., Li, P., Shu, Y. & Xiao, X. (2014). Risks of avian influenza transmission in areas of intensive free-ranging duck production with wild waterfowl. EcoHealth, 11, 109–119.
- Cappello, L. & Palacios, J.A. (2022). Adaptive preferential sampling in phylodynamics with an application to SARS-CoV-2. Journal of Computational and Graphical Statistics, 31, 541–552.
- Cardona-Ospina, J.A., Rojas-Gallardo, D.M., Garzón-Castaño, S.C., Jiménez-Posada, E.V. & Rodríguez-Morales, A.J. (2021). Phylodynamic analysis in the understanding of the current COVID-19 pandemic and its utility in vaccine and antiviral design and assessment. Human Vaccines & Immunotherapeutics, 17, 2437–2444.
- Chakraborty, D., Guinat, C., Müller, N.F., Briand, F.-X., Andraud, M., Scoizec, A., Lebouquin, S., Niqueux, E., Schmitz, A., Grasland, B., Guerin, J.-L., Paul, M.C. & Vergne, T. (2021). Phylodynamic assessment of control measures for highly pathogenic avian influenza epidemics in France. bioRxiv, 2021.06.23.449570.
- Chakraborty, D., Guinat, C., Müller, N.F., Briand, F.-X., Andraud, M., Scoizec, A., Lebouquin, S., Niqueux, E., Schmitz, A., Grasland, B., Guerin, J.-L., Paul, M.C. & Vergne, T. (2022). Phylodynamic analysis of the highly pathogenic avian influenza H5N8 epidemic in France, 2016–17. Transboundary and Emerging Diseases, 69, e1574–e1583.
- Chattopadhyay, K., Fournié, G., Abul Kalam, M., Biswas, P.K., Hoque, A., Debnath, N.C., Rahman, M., Pfeiffer, D.U., Harper, D. & Heymann, D.L. (2018). A qualitative stakeholder analysis of avian influenza policy in Bangladesh. EcoHealth, 15, 63–71.
- Chauhan, R.P. & Gordon, M.L. (2021). Deciphering transmission dynamics and spillover of avian influenza viruses from avian species to swine populations globally. Virus Genes, 57, 541–555.
- Cunningham, C.W., Omland, K.E. & Oakley, T.H. (1998). Reconstructing ancestral character states: a critical reappraisal. Trends in Ecology & Evolution, 13, 361–366.
- De Maio, N., Wu, C.-H., O’Reilly, K.M. & Wilson, D. (2015). New routes to phylogeography: a Bayesian structured coalescent approximation. PLoS Genetics, 11, e1005421.
- Dellicour, S., Gill, M.S., Faria, N.R., Rambaut, A., Pybus, O.G., Suchard, M.A. & Lemey, P. (2021). Relax, keep walking - a practical guide to continuous phylogeographic inference with BEAST. Molecular Biology and Evolution, 38, 3486–3493.
- Dellicour, S., Lemey, P., Artois, J., Lam, T.T., Fusaro, A., Monne, I., Cattoli, G., Kuznetsov, D., Xenarios, I., Dauphin, G., Kalpravidh, W., Von Dobschuetz, S., Claes, F., Newman, S.H., Suchard, M.A., Baele, G. & Gilbert, M. (2020). Incorporating heterogeneous sampling probabilities in continuous phylogeographic inference — application to H5N1 spread in the Mekong region. Bioinformatics, 36, 2098–2104.
- Dellicour, S., Lequime, S., Vrancken, B., Gill, M.S., Bastide, P., Gangavarapu, K., Matteson, N.L., Tan, Y., du Plessis, L., Fisher, A.A., Nelson, M.I., Gilbert, M., Suchard, M.A., Andersen, K.G., Grubaugh, N.D., Pybus, O.G. & Lemey, P. (2020). Epidemiological hypothesis testing using a phylogeographic and phylodynamic framework. Nature Communications, 11, 5620.
- Dellicour, S., Rose, R., Faria, N.R., Lemey, P. & Pybus, O.G. (2016). SERAPHIM: studying environmental rasters and phylogenetically informed movements. Bioinformatics, 32, 3204–3206.
- Dhingra, M.S., Artois, J., Dellicour, S., Lemey, P., Dauphin, G., Von Dobschuetz, S., Van Boeckel, T.P., Castellan, D.M., Morzaria, S. & Gilbert, M. (2018). Geographical and historical patterns in the emergences of novel highly pathogenic avian influenza (HPAI) H5 and H7 viruses in poultry. Frontiers in Veterinary Science, 5, 84.
- Domingo, E. (2020). Chapter 7 - Long-term virus evolution in nature. In E.B.T.-V. as P. (Second E. Domingo (Ed.), (pp. 225–261).
- Drummond, A.J., Rambaut, A., Shapiro, B. & Pybus, O.G. (2005). Bayesian coalescent inference of past population dynamics from molecular sequences. Molecular Biology and Evolution, 22, 1185–1192.
- Drummond, A.J., Ho, S.Y.W., Phillips, M.J. & Rambaut, A. (2006). Relaxed phylogenetics and dating with confidence. PLoS Biology, 4, e88.
- Drummond, A.J. & Rambaut, A. (2007). BEAST: Bayesian evolutionary analysis by sampling trees. BMC Evolutionary Biology, 7, 214.
- Duchene, S., Duchene, D.A., Geoghegan, J.L., Dyson, Z.A., Hawkey, J. & Holt, K.E. (2018). Inferring demographic parameters in bacterial genomic data using Bayesian and hybrid phylogenetic methods. BMC Evolutionary Biology, 18, 95.
- Duffy, S. (2018). Why are RNA virus mutation rates so damn high? PLoS Biology, 16, e3000003.
- Escalera-Zamudio, M., Golden, M., Gutiérrez, B., Thézé, J., Keown, J.R., Carrique, L., Bowden, T.A. & Pybus, O.G. (2020). Parallel evolution in the emergence of highly pathogenic avian influenza A viruses. Nature Communications, 11, 5511.
- Faria, N.R., Suchard, M.A., Rambaut, A. & Lemey, P. (2011). Toward a quantitative understanding of viral phylogeography. Current Opinion in Virology, 1, 423–429.
- Faria, N.R., Suchard, M.A., Rambaut, A., Streicker, D.G. & Lemey, P. (2013). Simultaneously reconstructing viral cross-species transmission history and identifying the underlying constraints. Philosophical Transactions of the Royal Society of London. Series B, Biological Sciences, 368, 20120196.
- Ferenczi, M., Beckmann, C. & Klaassen, M. (2021). Rainfall driven and wild-bird mediated avian influenza virus outbreaks in Australian poultry. BMC Veterinary Research, 17, 306.
- FitzJohn, R.G. (2012). Diversitree: comparative phylogenetic analyses of diversification in R. Methods in Ecology and Evolution, 3, 1084–1092.
- Fries, A.C., Nolting, J.M., Bowman, A.S., Lin, X., Halpin, R.A., Wester, E., Fedorova, N., Stockwell, T.B., Das, S.R., Dugan, V.G., Wentworth, D.E., Gibbs, H.L. & Slemons, R.D. (2015). Spread and persistence of influenza A viruses in waterfowl hosts in the North American Mississippi migratory flyway. Journal of Virology, 89, 5371–5381.
- Frost, S.D.W., Pybus, O.G., Gog, J.R., Viboud, C., Bonhoeffer, S. & Bedford, T. (2015). Eight challenges in phylodynamic inference. Epidemics, 10, 88–92.
- Frost, S.D.W. & Volz, E.M. (2010). Viral phylodynamics and the search for an “effective number of infections”. Philosophical Transactions of the Royal Society of London. Series B, Biological Sciences, 365, 1879–1890.
- Fusaro, A., Monne, I., Salviato, A., Valastro, V., Schivo, A., Amarin, N.M., Gonzalez, C., Ismail, M.M., Al-Ankari, A.-R., Al-Blowi, M.H., Khan, O.A., Maken Ali, A.S., Hedayati, A., Garcia Garcia, J., Ziay, G.M., Shoushtari, A., Al Qahtani, K.N., Capua, I., Holmes, E.C. & Cattoli, G. (2011). Phylogeography and evolutionary history of reassortant H9N2 viruses with potential human health implications. Journal of Virology, 85, 8413–8421.
- Fusaro, A., Zecchin, B., Vrancken, B., Abolnik, C., Ademun, R., Alassane, A., Arafa, A., Awuni, J.A., Couacy-Hymann, E., Coulibaly, M.B., Gaidet, N., Go-Maro, E., Joannis, T., Jumbo, S.D., Minoungou, G., Meseko, C., Souley, M.M., Ndumu, D.B., Shittu, I., Twabela, A., Wade, A., Wiersma, L., Akpeli, Y.P., Zamperin, G., Milani, A., Lemey, P. & Monne, I. (2019). Disentangling the role of Africa in the global spread of H5 highly pathogenic avian influenza. Nature Communications, 10, 5310.
- Gaidet, N. (2016). Ecology of avian influenza virus in wild birds in tropical Africa. Avian Diseases, 60, 296–301.
- Gaidet, N., Ould El Mamy, A.B., Cappelle, J., Caron, A., Cumming, G.S., Grosbois, V., Gil, P., Hammoumi, S., de Almeida, R.S., Fereidouni, S.R., Cattoli, G., Abolnik, C., Mundava, J., Fofana, B., Ndlovu, M., Diawara, Y., Hurtado, R., Newman, S.H., Dodman, T. & Balança, G. (2012). Investigating avian influenza infection hotspots in old-world shorebirds. PLoS One, 7, e46049.
- Gass Jr, J.D., Dusek, R.J., Hall, J.S., Hallgrimsson, G.T., Halldórsson, H.P., Vignisson, S.R., Ragnarsdottir, S.B., Jónsson, J.E., Krauss, S., Wong, S.-S., Wan, X.-F., Akter, S., Sreevatsan, S., Trovão, N.S., Nutter, F.B., Runstadler, J.A. & Hill, N.J. (2023). Global dissemination of influenza A virus is driven by wild bird migration through Arctic and subarctic zones. Molecular Ecology, 32, 198–213.
- Gerloff, N.A., Jones, J., Simpson, N., Balish, A., Elbadry, M.A., Baghat, V., Rusev, I., de Mattos, C.C., de Mattos, C.A., Zonkle, L.E.A., Kis, Z., Davis, C.T., Yingst, S., Cornelius, C., Soliman, A., Mohareb, E., Klimov, A. & Donis, R.O. (2013). A high diversity of eurasian lineage low pathogenicity avian influenza A viruses circulate among wild birds sampled in Egypt. PLoS One, 8, e68522–e68522.
- Gill, M.S., Lemey, P., Bennett, S.N., Biek, R. & Suchard, M.A. (2016). Understanding past population dynamics: Bayesian coalescent-based modeling with covariates. Systematic Biology, 65, 1041–1056.
- Gill, M.S., Lemey, P., Faria, N.R., Rambaut, A., Shapiro, B. & Suchard, M.A. (2013). Improving Bayesian population dynamics inference: a coalescent-based model for multiple loci. Molecular Biology and Evolution, 30, 713–724.
- Gonzales, J.L., Pritz-Verschuren, S., Bouwstra, R., Wiegel, J., Elbers, A.R.W. & Beerens, N. (2021). Seasonal risk of low pathogenic avian influenza virus introductions into free-range layer farms in the Netherlands. Transboundary and Emerging Diseases, 68, 127–136.
- Gostic, K.M., McGough, L., Baskerville, E.B., Abbott, S., Joshi, K., Tedijanto, C., Kahn, R., Niehus, R., Hay, J.A., De Salazar, P.M., Hellewell, J., Meakin, S., Munday, J.D., Bosse, N.I., Sherrat, K., Thompson, R.N., White, L.F., Huisman, J.S., Scire, J., Bonhoeffer, S., Stadler, T., Wallinga, J., Funk, S., Lipsitch, M. & Cobey, S. (2021). Practical considerations for measuring the effective reproductive number. PLoS Computational Biology, 16, e1008409.
- Grear, D.A., Hall, J.S., Dusek, R.J. & Ip, H.S. (2017). Inferring epidemiologic dynamics from viral evolution: 2014–2015 Eurasian/North American highly pathogenic avian influenza viruses exceed transmission threshold, R(0) = 1, in wild birds and poultry in North America. Evolutionary Applications, 11, 547–557.
- Grenfell, B.T., Pybus, O.G., Gog, J.R., Wood, J.L.N., Daly, J.M., Mumford, J.A. & Holmes, E.C. (2004). Unifying the epidemiological and evolutionary dynamics of pathogens. Science (New York, N.Y.), 303, 327–332. United States.
- Grubaugh, N.D., Ladner, J.T., Lemey, P., Pybus, O.G., Rambaut, A., Holmes, E.C. & Andersen, K.G. (2019). Tracking virus outbreaks in the twenty-first century. Nature Microbiology, 4, 10–19.
- Guinat, C., Valenzuela Agüí, C., Vaughan, T.G., Scire, J., Pohlmann, A., Staubach, C., King, J., Świętoń, E., Dán, Á, Černíková, L., Ducatez, M.F. & Stadler, T. (2022). Disentangling the role of poultry farms and wild birds in the spread of highly pathogenic avian influenza virus in Europe. Virus Evolution, 8, veac073.
- Hadfield, J., Megill, C., Bell, S.M., Huddleston, J., Potter, B., Callender, C., Sagulenko, P., Bedford, T. & Neher, R.A. (2018). Nextstrain: real-time tracking of pathogen evolution. Bioinformatics, 34, 4121–4123.
- Hall, J.S., Teslaa, J.L., Nashold, S.W., Halpin, R.A., Stockwell, T., Wentworth, D.E., Dugan, V. & Ip, H.S. (2013). Evolution of a reassortant North American gull influenza virus lineage: drift, shift and stability. Virology Journal, 10, 179.
- Hansbro, P.M., Warner, S., Tracey, J.P., Arzey, K.E., Selleck, P., O’Riley, K., Beckett, E.L., Bunn, C., Kirkland, P.D., Vijaykrishna, D., Olsen, B. & Hurt, A.C. (2010). Surveillance and analysis of avian influenza viruses, Australia. Emerging Infectious Diseases, 16, 1896–1904.
- Harvey, W.T., Mulatti, P., Fusaro, A., Scolamacchia, F., Zecchin, B., Monne, I. & Marangon, S. (2021). Spatiotemporal reconstruction and transmission dynamics during the 2016–17 H5N8 highly pathogenic avian influenza epidemic in Italy. Transboundary and Emerging Diseases, 68, 37–50.
- Heaton, N.S., Sachs, D., Chen, C.-J., Hai, R. & Palese, P. (2013). Genome-wide mutagenesis of influenza virus reveals unique plasticity of the hemagglutinin and NS1 proteins. Proceedings of the National Academy of Sciences of the United States of America, 110, 20248–20253.
- Hicks, J.T., Lee, D.-H., Duvvuri, V.R., Kim Torchetti, M., Swayne, D.E. & Bahl, J. (2020). Agricultural and geographic factors shaped the North American 2015 highly pathogenic avian influenza H5N2 outbreak. PLoS Pathogens, 16, e1007857–e1007857.
- Hill, N.J., Bishop, M.A., Trovão, N.S., Ineson, K.M., Schaefer, A.L., Puryear, W.B., Zhou, K., Foss, A.D., Clark, D.E., MacKenzie, K.G., Gass, Jr. J.D., Borkenhagen, L.K., Hall, J.S. & Runstadler, J.A. (2022). Ecological divergence of wild birds drives avian influenza spillover and global spread. PLoS Pathogens, 18, e1010062.
- Hill, N.J., Smith, L.M., Muzaffar, S.B., Nagel, J.L., Prosser, D.J., Sullivan, J.D., Spragens, K.A., DeMattos, C.A., DeMattos, C.C., El Sayed, L., Erciyas-Yavuz, K., Davis, C.T., Jones, J., Kis, Z., Donis, R.O., Newman, S.H. & Takekawa, J.Y. (2020). Crossroads of highly pathogenic H5N1: overlap between wild and domestic birds in the Black Sea-Mediterranean impacts global transmission. Virus Evolution, 7, veaa093–veaa093.
- Hill, S.C., Hansen, R., Watson, S., Coward, V., Russell, C., Cooper, J., Essen, S., Everest, H., Parag, K.V., Fiddaman, S., Reid, S., Lewis, N., Brookes, S.M., Smith, A.L., Sheldon, B., Perrins, C.M., Brown, I.H. & Pybus, O.G. (2019). Comparative micro-epidemiology of pathogenic avian influenza virus outbreaks in a wild bird population. Philosophical Transactions of the Royal Society of London. Series B, Biological Sciences, 374, 20180259.
- Hill, S.C., Lee, Y.-J., Song, B.-M., Kang, H.-M., Lee, E.-K., Hanna, A., Gilbert, M., Brown, I.H. & Pybus, O.G. (2015). Wild waterfowl migration and domestic duck density shape the epidemiology of highly pathogenic H5N8 influenza in the Republic of Korea. Infection, Genetics and Evolution, 34, 267–277.
- Hill, V., Ruis, C., Bajaj, S., Pybus, O.G. & Kraemer, M.U.G. (2021). Progress and challenges in virus genomic epidemiology. Trends in Parasitology, 37, 1038–1049.
- Hoye, B.J., Donato, C.M., Lisovski, S., Deng, Y.-M., Warner, S., Hurt, A.C., Klaassen, M. & Vijaykrishna, D. (2021). Reassortment and persistence of influenza A viruses from diverse geographic origins within Australian wild birds: evidence from a small, isolated population of ruddy turnstones. Journal of Virology, 95, e02193-20.
- Huang, Y., Wille, M., Benkaroun, J., Munro, H., Bond, A.L., Fifield, D.A., Robertson, G.J., Ojkic, D., Whitney, H. & Lang, A.S. (2014). Perpetuation and reassortment of gull influenza A viruses in Atlantic North America. Virology, 456–457, 353–363.
- Hulse-Post, D.J., Sturm-Ramirez, K.M., Humberd, J., Seiler, P., Govorkova, E.A., Krauss, S., Scholtissek, C., Puthavathana, P., Buranathai, C., Nguyen, T.D., Long, H.T., Naipospos, T.S.P., Chen, H., Ellis, T.M., Guan, Y., Peiris, J.S.M. & Webster, R.G. (2005). Role of domestic ducks in the propagation and biological evolution of highly pathogenic H5N1 influenza viruses in Asia. Proceedings of the National Academy of Sciences of the United States of America, 102, 10682–10687.
- Hurt, A.C., Vijaykrishna, D., Butler, J., Baas, C., Maurer-Stroh, S., Silva-de-la-Fuente, M.C., Medina-Vogel, G., Olsen, B., Kelso, A., Barr, I.G. & González-Acuña, D. (2014). Detection of evolutionarily distinct avian influenza A viruses in Antarctica. mBio, 5, e01098. American Society of Microbiology.
- ICTV. (2022). International Committee on Taxonomy of Viruses (ICTV). Retrieved July 18, 2022, from https://ictv.global/taxonomy/.
- Ishikawa, S.A., Zhukova, A., Iwasaki, W. & Gascuel, O. (2019). A fast likelihood method to reconstruct and visualize ancestral scenarios. Molecular Biology and Evolution, 36, 2069–2085.
- Iwamura, T., Possingham, H.P., Chadès, I., Minton, C., Murray, N.J., Rogers, D.I., Treml, E.A. & Fuller, R.A. (2013). Migratory connectivity magnifies the consequences of habitat loss from sea-level rise for shorebird populations. Proceedings. Biological Sciences, 280, 20130325.
- Jin, Y., Yu, D., Ren, H., Yin, Z., Huang, Z., Hu, M., Li, B., Zhou, W., Yue, J. & Liang, L. (2014). Phylogeography of avian influenza A H9N2 in China. BMC Genomics, 15, 1110.
- Joseph, U., Su, Y.C.F., Vijaykrishna, D. & Smith, G.J.D. (2017). The ecology and adaptive evolution of influenza A interspecies transmission. Influenza and Other Respiratory Viruses, 11, 74–84. John Wiley and Sons Inc.
- Kalkauskas, A., Perron, U., Sun, Y., Goldman, N., Baele, G., Guindon, S. & De Maio, N. (2021). Sampling bias and model choice in continuous phylogeography: getting lost on a random walk. PLoS Computational Biology, 17, e1008561.
- Kalonda, A., Saasa, N., Nkhoma, P., Kajihara, M., Sawa, H., Takada, A. & Simulundu, E. (2020). Avian influenza viruses detected in birds in Sub-saharan Africa: a systematic review. Viruses, 12, 993.
- Karcher, M.D., Carvalho, L.M., Suchard, M.A., Dudas, G. & Minin, V.N. (2020). Estimating effective population size changes from preferentially sampled genetic sequences. PLoS Computational Biology, 16, e1007774.
- Kirby, J.S., Stattersfield, A.J., Butchart, S.H.M., Evans, M.I., Grimmett, R.F.A., Jones, V.R., O’Sullivan, J., Tucker, G.M. & Newton, I. (2008). Key conservation issues for migratory land- and waterbird species on the world’s major flyways. Bird Conservation International, 18, S49–S73.
- Kleyheeg, E., Slaterus, R., Bodewes, R., Rijks, J.M., Spierenburg, M.A.H., Beerens, N., Kelder, L., Poen, M.J., Stegeman, J.A., Fouchier, R.A.M., Kuiken, T. & van der Jeugd, H.P. (2017). Deaths among wild birds during highly pathogenic avian influenza A(H5N8) virus outbreak, the Netherlands. Emerging Infectious Diseases, 23, 2050–2054.
- Kühnert, D., Stadler, T., Vaughan, T.G. & Drummond, A.J. (2016). Phylodynamics with migration: a computational framework to quantify population structure from genomic data. Molecular Biology and Evolution, 33, 2102–2116.
- Kwon, J.-H., Lee, D.-H., Criado, M.F., Killmaster, L., Ali, M.Z., Giasuddin, M., Samad, M.A., Karim, M.R., Hasan, M., Brum, E., Nasrin, T. & Swayne, D.E. (2020). Genetic evolution and transmission dynamics of clade 2.3.2.1a highly pathogenic avian influenza A/H5N1 viruses in Bangladesh. Virus Evolution, 6, veaa046.
- Lam, T.T.-Y., Hon, C.-C., Lemey, P., Pybus, O.G., Shi, M., Tun, H.M., Li, J., Jiang, J., Holmes, E.C. & Leung, F.C.-C. (2012). Phylodynamics of H5N1 avian influenza virus in Indonesia. Molecular Ecology, 21, 3062–3077.
- Lang, A.S., Lebarbenchon, C., Ramey, A.M., Robertson, G.J., Waldenström, J. & Wille, M. (2016). Assessing the role of seabirds in the ecology of influenza A viruses. Avian Diseases, 60, 378–386.
- Layan, M., Müller, N.F., Dellicour, S., De Maio, N., Bourhy, H., Cauchemez, S. & Baele, G. (2023). Impact and mitigation of sampling bias to determine viral spread: evaluating discrete phylogeography through CTMC modeling and structured coalescent model approximations. Virus Evolution, 9, vead010.
- Lean, F.Z.X., Vitores, A.G., Reid, S.M., Banyard, A.C., Brown, I.H., Núñez, A. & Hansen, R.D.E. (2022). Gross pathology of high pathogenicity avian influenza virus H5N1 2021–2022 epizootic in naturally infected birds in the United Kingdom. One Health, 14, 100392.
- Lebarbenchon, C., Jaeger, A., Feare, C., Bastien, M., Dietrich, M., Larose, C., Lagadec, E., Rocamora, G., Shah, N., Pascalis, H., Boulinier, T., Le Corre, M., Stallknecht, D.E. & Dellagi, K. (2015). Influenza A virus on oceanic islands: host and viral diversity in seabirds in the Western Indian Ocean. PLoS Pathogens, 11, e1004925–e1004925.
- Lebarbenchon, C. & Stallknecht, D.E. (2011). Host shifts and molecular evolution of H7 avian influenza virus hemagglutinin. Virology Journal, 8, 328.
- Lee, C.-C.D., Zhu, H., Huang, P.-Y., Peng, L., Chang, Y.-C., Yip, C.-H., Li, Y.-T., Cheung, C.-L., Compans, R., Yang, C., Smith, D.K., Lam, T.T.-Y., King, C.-C. & Guan, Y. (2014). Emergence and evolution of avian H5N2 influenza viruses in chickens in Taiwan. Journal of Virology, 88, 5677–5686.
- Lee, D.-H., Torchetti, M.K., Winker, K., Ip, H.S., Song, C.-S. & Swayne, D.E. (2015). Intercontinental spread of Asian-origin H5N8 to North America through Beringia by migratory birds. Journal of Virology, 89, 6521–6524.
- Lee, Y.-N., Cheon, S.-H., Lee, E.-K., Heo, G.-B., Bae, Y.-C., Joh, S.-J., Lee, M.-H. & Lee, Y.-J. (2018). Pathogenesis and genetic characteristics of novel reassortant low-pathogenic avian influenza H7 viruses isolated from migratory birds in the Republic of Korea in the winter of 2016–2017. Emerging Microbes & Infections, 7, 182.
- Lefkowitz, E.J., Dempsey, D.M., Hendrickson, R.C., Orton, R.J., Siddell, S.G. & Smith, D.B. (2018). Virus taxonomy: the database of the International Committee on Taxonomy of Viruses (ICTV). Nucleic Acids Research, 46, D708–D717.
- Lemey, P., Hong, S.L., Hill, V., Baele, G., Poletto, C., Colizza, V., O’Toole, Á, McCrone, J.T., Andersen, K.G., Worobey, M., Nelson, M.I., Rambaut, A. & Suchard, M.A. (2020). Accommodating individual travel history and unsampled diversity in Bayesian phylogeographic inference of SARS-CoV-2. Nature Communications, 11, 5110.
- Lemey, P., Rambaut, A., Bedford, T., Faria, N., Bielejec, F., Baele, G., Russell, C.A., Smith, D.J., Pybus, O.G., Brockmann, D. & Suchard, M.A. (2014). Unifying viral genetics and human transportation data to predict the global transmission dynamics of human influenza H3N2. PLoS Pathogens, 10, e1003932.
- Lemey, P., Rambaut, A., Bedford, T., Faria, N.R., Bielejec, F., Baele, G., Russell, C.A., Smith, D.J., Pybus, O.G., Brockmann, D. & Suchard, M.A. (2012). The seasonal flight of influenza: a unified framework for spatiotemporal hypothesis testing. arXiv: Populations and Evolution. 1210.5877.
- Lemey, P., Rambaut, A., Drummond, A.J. & Suchard, M.A. (2009). Bayesian phylogeography finds its roots. PLoS Computational Biology, 5, e1000520.
- Lemey, P., Rambaut, A., Welch, J.J. & Suchard, M.A. (2010). Phylogeography takes a relaxed random walk in continuous space and time. Molecular Biology and Evolution, 27, 1877–1885.
- Li, L., Bowman, A.S., DeLiberto, T.J., Killian, M.L., Krauss, S., Nolting, J.M., Torchetti, M.K., Ramey, A.M., Reeves, A.B., Stallknecht, D.E., Webby, R.J. & Wan, X.-F. (2018). Genetic evidence supports sporadic and independent introductions of subtype H5 low-pathogenic avian influenza A viruses from wild birds to domestic poultry in North America. Journal of Virology, 92, e00913–18.
- Li, Y.-T., Linster, M., Mendenhall, I.H., Su, Y.C.F. & Smith, G.J.D. (2019). Avian influenza viruses in humans: lessons from past outbreaks. British Medical Bulletin, 132, 81–95.
- Liang, Y., Krog, J.S., Ryt-Hansen, P., Pedersen, A.G., Kvisgaard, L.K., Holm, E., Nielsen, P.D., Hammer, A.S., Madsen, J.J., Thorup, K., Larsen, L.E. & Hjulsager, C.K. (2021). Molecular characterization of highly pathogenic avian influenza viruses H5N6 detected in Denmark in 2018–2019. Viruses, 13, 1052.
- Liang, Y., Nissen, J.N., Krog, J.S., Breum, SØ, Trebbien, R., Larsen, L.E. & Hjulsager, C.K. (2021). Novel clade 2.3.4.4b highly pathogenic avian influenza A H5N8 and H5N5 viruses in Denmark, 2020. Viruses, 13, 886.
- Lisovski, S., van Dijk, J.G.B., Klinkenberg, D., Nolet, B.A., Fouchier, R.A.M. & Klaassen, M. (2018). The roles of migratory and resident birds in local avian influenza infection dynamics. Journal of Applied Ecology, 55, 2963–2975.
- Liu, D., Shi, W., Shi, Y., Wang, D., Xiao, H., Li, W., Bi, Y., Wu, Y., Li, X., Yan, J., Liu, W., Zhao, G., Yang, W., Wang, Y., Ma, J., Shu, Y., Lei, F. & Gao, G.F. (2013). Origin and diversity of novel avian influenza A H7N9 viruses causing human infection: phylogenetic, structural, and coalescent analyses. Lancet, 381, 1926–1932.
- Lo, F.T., Zecchin, B., Diallo, A.A., Racky, O., Tassoni, L., Diop, A., Diouf, M., Diouf, M., Samb, Y.N., Pastori, A., Gobbo, F., Ellero, F., Diop, M., Lo, M.M., Diouf, M.N., Fall, M., Ndiaye, A.A., Gaye, A.M., Badiane, M., Lo, M., Youm, B.N., Ndao, I., Niaga, M., Terregino, C., Diop, B., Ndiaye, Y., Angot, A., Seck, I., Niang, M., Soumare, B., Fusaro, A. & Monne, I. (2022). Intercontinental spread of Eurasian highly pathogenic avian influenza A(H5N1) to Senegal. Emerging Infectious Diseases, 28, 234–237.
- Lu, L., Leigh Brown, A.J. & Lycett, S.J. (2017). Quantifying predictors for the spatial diffusion of avian influenza virus in China. BMC Evolutionary Biology, 17, 16.
- Lu, L., Lycett, S.J. & Leigh Brown, A.J. (2014). Reassortment patterns of avian influenza virus internal segments among different subtypes. BMC Evolutionary Biology, 14, 16.
- Lycett, S.J., Duchatel, F. & Digard, P. (2019). A brief history of bird flu. Philosophical Transactions of the Royal Society of London. Series B, Biological Sciences, 374, 20180257.
- Magee, D., Beard, R., Suchard, M.A., Lemey, P. & Scotch, M. (2015). Combining phylogeography and spatial epidemiology to uncover predictors of H5N1 influenza A virus diffusion. Archives of Virology, 160, 215–224.
- Magiorkinis, G., Sypsa, V., Magiorkinis, E., Paraskevis, D., Katsoulidou, A., Belshaw, R., Fraser, C., Pybus, O.G. & Hatzakis, A. (2013). Integrating phylodynamics and epidemiology to estimate transmission diversity in viral epidemics. PLoS Computational Biology, 9, e1002876.
- Martin, V., Pfeiffer, D.U., Zhou, X., Xiao, X., Prosser, D.J., Guo, F. & Gilbert, M. (2011). Spatial distribution and risk factors of highly pathogenic avian influenza (HPAI) H5N1 in China. PLoS Pathogens, 7, e1001308.
- McBride, D.S., Lauterbach, S.E., Li, Y.-T., Smith, G.J.D., Killian, M.L., Nolting, J.M., Su, Y.C.F. & Bowman, A.S. (2021). Genomic evidence for sequestration of influenza A virus lineages in sea duck host species. Viruses, 13, 172.
- Melville, D.S. & Shortridge, K.F. (2006). Spread of H5N1 avian influenza virus: an ecological conundrum. Letters in Applied Microbiology, 42, 435–437.
- Miller, M., Takekawa, J., Fleskes, J., Orthmeyer, D., Casazza, M. & Perry, W. (2011). Spring migration of Northern Pintails from California’s Central Valley wintering area tracked with satellite telemetry: routes, timing, and destinations. Canadian Journal of Zoology, 83, 1314–1332.
- Mine, J., Uchida, Y., Sharshov, K., Sobolev, I., Shestopalov, A. & Saito, T. (2019). Phylogeographic evidence for the inter- and intracontinental dissemination of avian influenza viruses via migration flyways. PLoS One, 14, e0218506–e0218506.
- Minin, V.N., Bloomquist, E.W. & Suchard, M.A. (2008). Smooth skyride through a rough skyline: Bayesian coalescent-based inference of population dynamics. Molecular Biology and Evolution, 25, 1459–1471.
- Minin, V.N. & Suchard, M.A. (2008a). Counting labeled transitions in continuous-time Markov models of evolution. Journal of Mathematical Biology, 56, 391–412.
- Minin, V.N. & Suchard, M.A. (2008b). Fast, accurate and simulation-free stochastic mapping. Philosophical Transactions of the Royal Society of London. Series B, Biological Sciences, 363, 3985–3995.
- Monne, I., Fusaro, A., Nelson, M.I., Bonfanti, L., Mulatti, P., Hughes, J., Murcia, P.R., Schivo, A., Valastro, V., Moreno, A., Holmes, E.C. & Cattoli, G. (2014). Emergence of a highly pathogenic avian influenza virus from a low-pathogenic progenitor. Journal of Virology, 88, 4375–4388.
- More, S., Bicout, D., Bøtner, A., Butterworth, A., Calistri, P., Depner, K., Edwards, S., Garin-Bastuji, B., Good, M., Gortázar Schmidt, C., Michel, V., Miranda, M.A., Nielsen, S.S., Raj, M., Sihvonen, L., Spoolder, H., Thulke, H.-H., Velarde, A., Willeberg, P., Winckler, C., Breed, A., Brouwer, A., Guillemain, M., Harder, T., Monne, I., Roberts, H., Baldinelli, F., Barrucci, F., Fabris, C., Martino, L., Mosbach-Schulz, O., Verdonck, F., Morgado, J. & Stegeman, J.A. (2017). Avian influenza. EFSA Journal, 15, e04991.
- Mostafa, A., Abdelwhab, E.M., Mettenleiter, T.C. & Pleschka, S. (2018). Zoonotic potential of influenza A viruses: a comprehensive overview. Viruses, 10, 497.
- Moyen, N., Hoque, M.A., Mahmud, R., Hasan, M., Sarkar, S., Biswas, P.K., Mehedi, H., Henning, J., Mangtani, P., Flora, M.S., Rahman, M., Debnath, N.C., Giasuddin, M., Barnett, T., Pfeiffer, D.U. & Fournié, G. (2021). Avian influenza transmission risk along live poultry trading networks in Bangladesh. Scientific Reports, 11, 19962.
- Müller, N.F., Dudas, G. & Stadler, T. (2019). Inferring time-dependent migration and coalescence patterns from genetic sequence and predictor data in structured populations. Virus Evolution, 5, vez030.
- Müller, N.F., Rasmussen, D. & Stadler, T. (2018). MASCOT: parameter and state inference under the marginal structured coalescent approximation. Bioinformatics, 34, 3843–3848.
- Müller, N.F., Stolz, U., Dudas, G., Stadler, T. & Vaughan, T.G. (2020). Bayesian inference of reassortment networks reveals fitness benefits of reassortment in human influenza viruses. Proceedings of the National Academy of Sciences of the United States of America, 117, 17104–17111.
- Naguib, M.M., Verhagen, J.H., Samy, A., Eriksson, P., Fife, M., Lundkvist, Å, Ellström, P. & Järhult, J.D. (2019). Avian influenza viruses at the wild-domestic bird interface in Egypt. Infection Ecology & Epidemiology, 9, 1575687.
- Nelson, M.I., Gramer, M.R., Vincent, A.L. & Holmes, E.C. (2012). Global transmission of influenza viruses from humans to swine. The Journal of General Virology, 93, 2195–2203.
- Nelson, M.I., Viboud, C., Vincent, A.L., Culhane, M.R., Detmer, S.E., Wentworth, D.E., Rambaut, A., Suchard, M.A., Holmes, E.C. & Lemey, P. (2015). Global migration of influenza A viruses in swine. Nature Communications, 6, 6696.
- Nickbakhsh, S., Hall, M.D., Dorigatti, I., Lycett, S.J., Mulatti, P., Monne, I., Fusaro, A., Woolhouse, M.E.J., Rambaut, A. & Kao, R.R. (2016). Modelling the impact of co-circulating low pathogenic avian influenza viruses on epidemics of highly pathogenic avian influenza in poultry. Epidemics, 17, 27–34.
- Nuñez, I.A. & Ross, T.M. (2019). A review of H5Nx avian influenza viruses. Therapeutic Advances in Vaccines and Immunotherapy, 7, 2515135518821625.
- Oliver, I., Roberts, J., Brown, C., Byrne, A., Mellon, D., Hansen, R., Banyard, A., James, J., Donati, M., Porter, R., Ellis, J., Cogdale, J., Lackenby, A., Chand, M., Dabrera, G., Brown, I.H. & Zambon, M. (2022). A case of avian influenza A(H5N1) in England, January 2022. Eurosurveillance, 27, 2200061.
- Olsen, B., Munster, V.J., Wallensten, A., Waldenström, J., Osterhaus, A.D.M.E. & Fouchier, R.A.M. (2006). Global patterns of influenza A virus in wild birds. Science, 312, 384–388.
- Palm, E.C., Newman, S.H., Prosser, D.J., Xiao, X., Ze, L., Batbayar, N., Balachandran, S. & Takekawa, J.Y. (2015). Mapping migratory flyways in Asia using dynamic Brownian bridge movement models. Movement Ecology, 3, 3.
- Parag, K.V., du Plessis, L. & Pybus, O.G. (2020). Jointly inferring the dynamics of population size and sampling intensity from molecular sequences. Molecular Biology and Evolution, 37, 2414–2429.
- Parvin, R., Heenemann, K., Halami, M.Y., Chowdhury, E.H., Islam, M.R. & Vahlenkamp, T.W. (2014). Full-genome analysis of avian influenza virus H9N2 from Bangladesh reveals internal gene reassortments with two distinct highly pathogenic avian influenza viruses. Archives of Virology, 159, 1651–1661.
- Parvin, R., Nooruzzaman, M., Kabiraj, C.K., Begum, J.A., Chowdhury, E.H., Islam, M.R. & Harder, T. (2020). Controlling avian influenza virus in Bangladesh: challenges and recommendations. Viruses, 12, 751.
- Prosser, D.J., Palm, E.C., Takekawa, J.Y., Zhao, D., Xiao, X., Li, P., Liu, Y. & Newman, S.H. (2016). Movement analysis of free-grazing domestic ducks in Poyang Lake, China: a disease connection. International Journal of Geographical Information Science: IJGIS, 30, 869–880.
- Puryear, W., Sawatzki, K., Hill, N., Foss, A., Stone, J., Doughty, L., Walk, D., Gilbert, K., Murray, M., Cox, E., Patel, P., Mertz, Z., Ellis, S., Taylor, J., Fauquier, D., Smith, A., DiGiovanni, R., van de Guchte, A., Gonzalez-Reiche, A.S., Khalil, Z., van Bakel, H., Torchetti, M., Lantz, K., Lenoch, J. & Runstadler, J. (2023). Highly pathogenic avian influenza A(H5N1) virus outbreak in new England seals, United States. Emerging Infectious Disease Journal, 29, 786.
- Pybus, O.G. & Rambaut, A. (2009). Evolutionary analysis of the dynamics of viral infectious disease. Nature Reviews. Genetics, 10, 540–550.
- Ramey, A.M., Hill, N.J., Cline, T., Plancarte, M., De La Cruz, S., Casazza, M.L., Ackerman, J.T., Fleskes, J.P., Vickers, T.W., Reeves, A.B., Gulland, F., Fontaine, C., Prosser, D.J., Runstadler, J.A. & Boyce, W.M. (2017). Surveillance for highly pathogenic influenza A viruses in California during 2014–2015 provides insights into viral evolutionary pathways and the spatiotemporal extent of viruses in the Pacific Americas Flyway. Emerging Microbes & Infections, 6, e80–e80.
- Ramey, A.M., Pearce, J.M., Ely, C.R., Guy, L.M.S., Irons, D.B., Derksen, D.V. & Ip, H.S. (2010). Transmission and reassortment of avian influenza viruses at the Asian-North American interface. Virology, 406, 352–359.
- Rasmussen, D.A. & Grünwald, N.J. (2020). Phylogeographic approaches to characterize the emergence of plant pathogens. Phytopathology, 111, 68–77.
- Ravindran, S. (2021). Smartphone science: apps test and track infectious diseases. Nature, 593, 302–303.
- Ren, H., Jin, Y., Hu, M., Zhou, J., Song, T., Huang, Z., Li, B., Li, K., Zhou, W., Dai, H., Shi, W., Yue, J. & Liang, L. (2016). Ecological dynamics of influenza A viruses: cross-species transmission and global migration. Scientific Reports, 6, 36839.
- Rife, B.D., Mavian, C., Chen, X., Ciccozzi, M., Salemi, M., Min, J. & Prosperi, M.C.F. (2017). Phylodynamic applications in 21st century global infectious disease research. Global Health Research and Policy, 2, 13.
- Rijks, J.M., Hesselink, H., Lollinga, P., Wesselman, R., Prins, P., Weesendorp, E., Engelsma, M., Heutink, R., Harders, F., Kik, M., Rozendaal, H., van den Kerkhof, H. & Beerens, N. (2021). Highly pathogenic avian influenza A(H5N1) virus in wild red foxes, the Netherlands, 2021. Emerging Infectious Diseases, 27, 2960–2962.
- Rimi, N.A., Hassan, M.Z., Chowdhury, S., Rahman, M., Sultana, R., Biswas, P.K., Debnath, N.C., Islam, S.S. & Ross, A.G. (2019). A decade of avian influenza in Bangladesh: where Are We Now? Tropical Medicine and Infectious Disease, 4, 119.
- Rimondi, A., Gonzalez-Reiche, A.S., Olivera, V.S., Decarre, J., Castresana, G.J., Romano, M., Nelson, M.I., van Bakel, H., Pereda, A.J., Ferreri, L., Geiger, G. & Perez, D.R. (2018). Evidence of a fixed internal gene constellation in influenza A viruses isolated from wild birds in Argentina (2006–2016). Emerging Microbes & Infections, 7, 194.
- Ripa, R.N., Sealy, J.E., Raghwani, J., Das, T., Barua, H., Masuduzzaman, M., Saifuddin, A., Huq, M.R., Uddin, M.I., Iqbal, M., Brown, I., Lewis, N.S., Pfeiffer, D., Fournie, G. & Biswas, P.K. (2021). Molecular epidemiology and pathogenicity of H5N1 and H9N2 avian influenza viruses in clinically affected chickens on farms in Bangladesh. Emerging Microbes & Infections, 10, 1–408.
- Rott, R. (1992). The pathogenic determinant of influenza virus. Veterinary Microbiology, 33, 303–310.
- Runstadler, J., Hill, N., Hussein, I.T.M., Puryear, W. & Keogh, M. (2013). Connecting the study of wild influenza with the potential for pandemic disease. Infection, Genetics and Evolution: Journal of Molecular Epidemiology and Evolutionary Genetics in Infectious Diseases, 17, 162–187.
- Sagulenko, P., Puller, V. & Neher, R.A. (2018). Treetime: maximum-likelihood phylodynamic analysis. Virus Evolution, 4, vex042.
- Schmidt, H.A. & von Haeseler, A. (2009). Phylogenetic inference using maximum likelihood methods. In A.-M. Vandamme, M. Salemi & P. Lemey (Eds.), The Phylogenetic Handbook: A Practical Approach to Phylogenetic Analysis and Hypothesis Testing (2nd ed., pp. 181–209). Cambridge: Cambridge University Press.
- Scotch, M., Lam, T.T.-Y., Pabilonia, K.L., Anderson, T., Baroch, J., Kohler, D. & DeLiberto, T.J. (2014). Diffusion of influenza viruses among migratory birds with a focus on the southwest United States. Infection, Genetics and Evolution, 26, 185–193.
- Seekings, A.H., Slomka, M.J., Russell, C., Howard, W.A., Choudhury, B., Nuñéz, A., Löndt, B.Z., Cox, W., Ceeraz, V., Thorén, P., Irvine, R.M., Manvell, R.J., Banks, J. & Brown, I.H. (2018). Direct evidence of H7N7 avian influenza virus mutation from low to high virulence on a single poultry premises during an outbreak in free range chickens in the UK, 2008. Infection, Genetics and Evolution: Journal of Molecular Epidemiology and Evolutionary Genetics in Infectious Diseases, 64, 13–31.
- Seidel, S., Stadler, T. & Vaughan, T.G. (2020). Estimating disease spread using structured coalescent and birth-death models: a quantitative comparison. bioRxiv, 2020.11.30.403741.
- Seiler, P., Kercher, L., Feeroz, M.M., Shanmuganatham, K., Jones-Engel, L., Turner, J., Walker, D., Alam, S.M.R., Hasan, M.K., Akhtar, S., McKenzie, P., Franks, J., Krauss, S., Webby, R.J. & Webster, R.G. (2018). H9N2 influenza viruses from Bangladesh: transmission in chicken and New World quail. Influenza and Other Respiratory Viruses, 12, 814–817.
- Sharshov, K., Mine, J., Sobolev, I., Kurskaya, O., Dubovitskiy, N., Kabilov, M., Alikina, T., Nakayama, M., Tsunekuni, R., Derko, A., Prokopyeva, E., Alekseev, A., Shchelkanov, M., Druzyaka, A., Gadzhiev, A., Uchida, Y., Shestopalov, A. & Saito, T. (2019). Characterization and phylodynamics of reassortant H12Nx viruses in Northern Eurasia. Microorganisms 7, 643.
- Stadler, T. & Bonhoeffer, S. (2013). Uncovering epidemiological dynamics in heterogeneous host populations using phylogenetic methods. Philosophical Transactions of the Royal Society of London. Series B, Biological Sciences, 368, 20120198.
- Stadler, T., Kouyos, R., von Wyl, V., Yerly, S., Böni, J., Bürgisser, P., Klimkait, T., Joos, B., Rieder, P., Xie, D., Günthard, H.F., Drummond, A.J. & Bonhoeffer, S. (2012). Estimating the basic reproductive number from viral sequence data. Molecular Biology and Evolution, 29, 347–357. United States.
- Stadler, T., Kühnert, D., Bonhoeffer, S. & Drummond, A.J. (2013). Birth–death skyline plot reveals temporal changes of epidemic spread in HIV and hepatitis C virus (HCV). Proceedings of the National Academy of Sciences, 110, 228–233.
- Stadler, T., Vaughan, T.G., Gavryushkin, A., Guindon, S., Kühnert, D., Leventhal, G.E. & Drummond, A.J. (2015). How well can the exponential-growth coalescent approximate constant-rate birth-death population dynamics? Proceedings. Biological Sciences, 282, 20150420.
- Stolz, U., Stadler, T., Müller, N.F. & Vaughan, T.G. (2022). Joint inference of migration and reassortment patterns for viruses with segmented genomes. Molecular Biology and Evolution, 39, msab342.
- Suchard, M.A. & Rambaut, A. (2009). Many-core algorithms for statistical phylogenetics. Bioinformatics, 25, 1370–1376.
- Sullivan, J.D., Takekawa, J.Y., Spragens, K.A., Newman, S.H., Xiao, X., Leader, P.J., Smith, B. & Prosser, D.J. (2018). Waterfowl spring migratory behavior and avian influenza transmission risk in the changing landscape of the East Asian-australasian flyway. Frontiers in Ecology and Evolution, 6.
- Takekawa, J.Y., Newman, S.H., Xiao, X., Prosser, D.J., Spragens, K.A., Palm, E.C., Yan, B., Li, T., Lei, F., Zhao, D., Douglas, D.C., Muzaffar, S.B. & Ji, W. (2010). Migration of waterfowl in the East Asian flyway and spatial relationship to HPAI H5N1 outbreaks. Avian Diseases, 54, 466–476.
- Tian, H., Cui, Y., Dong, L., Zhou, S., Li, X., Huang, S., Yang, R. & Xu, B. (2015). Spatial, temporal and genetic dynamics of highly pathogenic avian influenza A (H5N1) virus in China. BMC Infectious Diseases, 15, 54.
- Tian, H., Zhou, S., Dong, L., Van Boeckel, T.P., Cui, Y., Newman, S.H., Takekawa, J.Y., Prosser, D.J., Xiao, X., Wu, Y., Cazelles, B., Huang, S., Yang, R., Grenfell, B.T. & Xu, B. (2015). Avian influenza H5N1 viral and bird migration networks in Asia. Proceedings of the National Academy of Sciences of the United States of America, 112, 172–177.
- Trovão, N.S., Suchard, M.A., Baele, G., Gilbert, M. & Lemey, P. (2015). Bayesian inference reveals host-specific contributions to the epidemic expansion of influenza A H5N1. Molecular Biology and Evolution, 32, 3264–3275.
- Van Borm, S., Rosseel, T., Vangeluwe, D., Vandenbussche, F., van den Berg, T. & Lambrecht, B. (2012). Phylogeographic analysis of avian influenza viruses isolated from Charadriiformes in Belgium confirms intercontinental reassortment in gulls. Archives of Virology, 157, 1509–1522.
- van Dijk, J.G.B., Hoye, B.J., Verhagen, J.H., Nolet, B.A., Fouchier, R.A.M. & Klaassen, M. (2014). Juveniles and migrants as drivers for seasonal epizootics of avian influenza virus. The Journal of Animal Ecology, 83, 266–275.
- Vandegrift, K.J., Sokolow, S.H., Daszak, P. & Kilpatrick, A.M. (2010). Ecology of avian influenza viruses in a changing world. Annals of the New York Academy of Sciences, 1195, 113–128.
- Vaughan, T.G., Kühnert, D., Popinga, A., Welch, D. & Drummond, A.J. (2014). Efficient Bayesian inference under the structured coalescent. Bioinformatics, 30, 2272–2279.
- Venkatesh, D., Brouwer, A., Goujgoulova, G., Ellis, R., Seekings, J., Brown, I.H. & Lewis, N.S. (2020). Regional transmission and reassortment of 2.3.4.4b highly pathogenic avian influenza (HPAI) viruses in Bulgarian poultry 2017/18. Viruses, 12, 605.
- Venkatesh, D., Poen, M.J., Bestebroer, T.M., Scheuer, R.D., Vuong, O., Chkhaidze, M., Machablishvili, A., Mamuchadze, J., Ninua, L., Fedorova, N.B., Halpin, R.A., Lin, X., Ransier, A., Stockwell, T.B., Wentworth, D.E., Kriti, D., Dutta, J., van Bakel, H., Puranik, A., Slomka, M.J., Essen, S., Brown, I.H., Fouchier, R.A.M. & Lewis, N.S. (2018). Avian influenza viruses in wild birds: virus evolution in a multihost ecosystem. Journal of Virology, 92, e00433-18.
- Verhagen, J.H., Eriksson, P., Leijten, L., Blixt, O., Olsen, B., Waldenström, J., Ellström, P. & Kuiken, T. (2021). Host range of influenza A virus H1 to H16 in eurasian ducks based on tissue and receptor binding studies. Journal of Virology, 95, e01873-20.
- Verhagen, J.H., Fouchier, R.A.M. & Lewis, N. (2021). Highly pathogenic avian influenza viruses at the wild-domestic bird interface in Europe: future directions for research and surveillance. Viruses, 13, 212.
- Verhagen, J.H., Poen, M., Stallknecht, D.E., van der Vliet, S., Lexmond, P., Sreevatsan, S., Poulson, R.L., Fouchier, R.A.M. & Lebarbenchon, C. (2020). Phylogeography and antigenic diversity of low-pathogenic avian influenza H13 and H16 viruses. Journal of Virology, 94, e00537–20.
- Verhagen, J.H., van Dijk, J.G.B., Vuong, O., Bestebroer, T., Lexmond, P., Klaassen, M. & Fouchier, R.A.M. (2014). Migratory birds reinforce local circulation of avian influenza viruses. PLoS One, 9, e112366–e112366.
- Vijaykrishna, D., Deng, Y.-M., Su, Y.C.F., Fourment, M., Iannello, P., Arzey, G.G., Hansbro, P.M., Arzey, K.E., Kirkland, P.D., Warner, S., O’Riley, K., Barr, I.G., Smith, G.J.D. & Hurt, A.C. (2013). The recent establishment of North American H10 lineage influenza viruses in Australian wild waterfowl and the evolution of Australian avian influenza viruses. Journal of Virology, 87, 10182–10189.
- Volz, E.M., Koelle, K. & Bedford, T. (2013). Viral phylodynamics. PLoS Computational Biology, 9, e1002947–e1002947.
- Vreman, S., Kik, M., Germeraad, E., Heutink, R., Harders, F., Spierenburg, M., Engelsma, M., Rijks, J., van den, B.J. & Beerens, N. (2023). Zoonotic mutation of highly pathogenic avian influenza H5N1 virus identified in the brain of multiple wild carnivore species. Pathogens, 12. 168.
- Webster, R.G., Bean, W.J., Gorman, O.T., Chambers, T.M. & Kawaoka, Y. (1992). Evolution and ecology of influenza A viruses. Microbiological Reviews, 56, 152–179.
- Wille, M., Grillo, V., Ban de Gouvea Pedroso, S., Burgess, G.W., Crawley, A., Dickason, C., Hansbro, P.M., Hoque, M.A., Horwood, P.F., Kirkland, P.D., Kung, N.Y.-H., Lynch, S.E., Martin, S., McArthur, M., O’Riley, K., Read, A.J., Warner, S., Hoye, B.J., Lisovski, S., Leen, T., Hurt, A.C., Butler, J., Broz, I., Davies, K.R., Mileto, P., Neave, M.J., Stevens, V., Breed, A.C., Lam, T.T.Y., Holmes, E.C., Klaassen, M. & Wong, F.Y.K. (2022). Australia as a global sink for the genetic diversity of avian influenza A virus. PLoS Pathogens, 18, e1010150.
- Wille, M. & Holmes, E.C. (2020). The ecology and evolution of influenza viruses. Cold Spring Harbor Perspectives in Medicine, 10, a038489.
- Wille, M., Lisovski, S., Roshier, D., Ferenczi, M., Hoye, B.J., Leen, T., Warner, S., Fouchier, R.A.M., Hurt, A.C., Holmes, E.C. & Klaassen, M. (2023). Strong host phylogenetic and ecological effects on host competency for avian influenza in Australian wild birds. Proceedings of the Royal Society B: Biological Sciences, 290, 20222237.
- Wille, M., Robertson, G.J., Whitney, H., Bishop, M.A., Runstadler, J.A. & Lang, A.S. (2011). Extensive geographic mosaicism in avian influenza viruses from gulls in the northern hemisphere. PLoS One, 6, e20664.
- Xie, R., Edwards, K.M., Wille, M., Wei, X., Wong, S.-S., Zanin, M., El-Shesheny, R., Ducatez, M., Poon, L.L.M., Kayali, G., Webby, R.J. & Dhanasekaran, V. (2022). The episodic resurgence of highly pathogenic avian influenza H5 virus. bioRxiv, 2022.12.18.520670.
- Yang, G., Chowdury, S., Hodges, E., Rahman, M.Z., Jang, Y., Hossain, M.E., Jones, J., Stark, T.J., Di, H., Cook, P.W., Ghosh, S., Azziz-Baumgartner, E., Barnes, J.R., Wentworth, D.E., Kennedy, E. & Davis, C.T. (2019). Detection of highly pathogenic avian influenza A(H5N6) viruses in waterfowl in Bangladesh. Virology, 534, 36–44.
- Yang, J., Müller, N.F., Bouckaert, R., Xu, B. & Drummond, A.J. (2019). Bayesian phylodynamics of avian influenza A virus H9N2 in Asia with time-dependent predictors of migration. PLoS Computational Biology, 15, e1007189.
- Yang, J., Xie, D., Nie, Z., Xu, B. & Drummond, A.J. (2019). Inferring host roles in Bayesian phylodynamics of global avian influenza A virus H9N2. Virology, 538, 86–96.
- Yang, L., Zhu, W., Li, X., Chen, M., Wu, J., Yu, P., Qi, S., Huang, Y., Shi, W., Dong, J., Zhao, X., Huang, W., Li, Z., Zeng, X., Bo, H., Chen, T., Chen, W., Liu, J., Zhang, Y., Liang, Z., Shi, W., Shu, Y. & Wang, D. (2017). Genesis and spread of newly emerged highly pathogenic H7N9 avian viruses in mainland China. Journal of Virology, 91, e01277–17.
- Yang, Q., Zhao, X., Lemey, P., Suchard, M.A., Bi, Y., Shi, W., Liu, D., Qi, W., Zhang, G., Stenseth, N.C., Pybus, O.G. & Tian, H. (2020). Assessing the role of live poultry trade in community-structured transmission of avian influenza in China. Proceedings of the National Academy of Sciences, 117, 5949–5954.
- Yong, D.L., Heim, W., Chowdhury, S.U., Choi, C.-Y., Ktitorov, P., Kulikova, O., Kondratyev, A.V., Round, P.D., Allen, D.N.S., Trainor, C., Gibson, L. & Szabo, J.K. (2021). The state of migratory landbirds in the East Asian flyway: distributions, threats, and conservation needs. Frontiers in Ecology and Evolution, 9.
- Yoon, S.-W., Webby, R. & Webster, R. (2014). Evolution and ecology of influenza A viruses. Current Topics in Microbiology and Immunology, 385, 359–75.
- Youk, S.-S., Lee, D.-H., Jeong, J.-H., Pantin-Jackwood, M.J., Song, C.-S. & Swayne, D.E. (2020). Live bird markets as evolutionary epicentres of H9N2 low pathogenicity avian influenza viruses in Korea. Emerging Microbes & Infections, 9, 616–627.
- Yu, H., Wu, J.T., Cowling, B.J., Liao, Q., Fang, V.J., Zhou, S., Wu, P., Zhou, H., Lau, E.H.Y., Guo, D., Ni, M.Y., Peng, Z., Feng, L., Jiang, H., Luo, H., Li, Q., Feng, Z., Wang, Y., Yang, W. & Leung, G.M. (2014). Effect of closure of live poultry markets on poultry-to-person transmission of avian influenza A H7N9 virus: an ecological study. Lancet, 383, 541–548.
- Yu, H., Deng, J., Leen, T., Li, G. & Klaassen, M. (2022). Continuous on-board behaviour classification using accelerometry: A case study with a new GPS-3G-bluetooth system in Pacific black ducks. Methods in Ecology and Evolution, 13, 1429–1435.
- Zhang, G., Li, B., Raghwani, J., Vrancken, B., Jia, R., Hill, S.C., Fournié, G., Cheng, Y., Yang, Q., Wang, Y., Wang, Z., Dong, L., Pybus, O.G. & Tian, H. (2023). Bidirectional movement of emerging H5N8 avian influenza viruses between Europe and Asia via migratory birds since early 2020. Molecular Biology and Evolution, 40, msad019.
- Zhang, J., Chen, Y., Shan, N., Wang, X., Lin, S., Ma, K., Li, B., Li, H., Liao, M. & Qi, W. (2020). Genetic diversity, phylogeography, and evolutionary dynamics of highly pathogenic avian influenza A (H5N6) viruses. Virus Evolution, 6, veaa079–veaa079.
- Zhang, J., Li, X., Wang, X., Ye, H., Li, B., Chen, Y., Chen, J., Zhang, T., Qiu, Z., Li, H., Jia, W., Liao, M. & Qi, W. (2021). Genomic evolution, transmission dynamics, and pathogenicity of avian influenza A (H5N8) viruses emerging in China, 2020. Virus Evolution, 7, veab046–veab046.
- Zhang, T., Fan, K., Zhang, X., Xu, Y., Xu, J., Xu, B. & Li, R. (2020). Diversity of avian influenza A(H5N6) viruses in wild birds in southern China. The Journal of General Virology, 101, 902–909.
- Zhao, P., Sun, L., Xiong, J., Wang, C., Chen, L., Yang, P., Yu, H., Yan, Q., Cheng, Y., Jiang, L., Chen, Y., Zhao, G., Jiang, Q. & Xiong, C. (2019). Semiaquatic mammals might be intermediate hosts to spread avian influenza viruses from avian to human. Scientific Reports, 9, 11641.
- Zhou, X., Li, H., Ni, X., Liu, M., Hu, M., Wu, J., Xia, W., Yang, S., Liu, Y., Xiong, Z. & Chen, H. (2013). Laboratory diagnosis and epidemiology of avian influenza A (H7N9) virus infection in humans in Nanchang city, China. Japanese Journal of Infectious Diseases, 66, 558–560.
- zu Dohna, H., Li, J., Cardona, C.J., Miller, J. & Carpenter, T.E. (2009). Invasions by Eurasian avian influenza virus H6 genes and replacement of the virus’ North American clade. Emerging Infectious Diseases, 15, 1040–1045.