ABSTRACT
Ridesharing has been attracting increasing attention from both academia and industry. One of the challenges posed by the study of ridesharing is to identify the most valuable information for improving the ridesharing decisions taken by participants. Another challenge is to use harvesting techniques to extract specific types of travel-related information. Many methods have been developed by the community in order to solve these issues. However, due to a lack of information sharing between different transit authorities and the difficulty of identifying subjective perceptions of the experience of ridesharing, understanding and evaluating how social media data might support or obstruct goals for mobility, safety and environmental sustainability in ridesharing is a difficult task. In this survey, we first analyze the literature on ridesharing with a focus on the properties and model of service, and introduce a framework to describe the major components required for a ridesharing service. Then, we illustrate the potential value of information extracted from social media and present the rationale for harvesting travel-related data. Finally, we detail some possible directions and different approaches for using social media data, and highlight their assets and drawbacks.
1. Introduction
Ridesharing services aim to optimize the use of private cars, offering the prospect of roads with fewer cars, each of them carrying more people. With a one-time transaction, it allows passengers to be assigned to a nearby driver and be shuttled to their destination (Borcuch Citation2016). Although scholars and transport planners have paid more attention to taxi sharing (Ma, Zheng, and Wolfson Citation2013; d’Orey and Ferreira Citation2015; He and Shen Citation2015), ridesharing services are distinguished from taxis by the fact that drivers are generally non-professionals providing rides on a part-time basis using their personal vehicles. Due to the increasing empirical evidence indicating ridesharing’s usage and impact (Shaheen, Cohen, and Zohdy Citation2016), much strategic investment, such as that by Zipcar and Lyft in the USA, and DiDi in China, has been made to effectively participate in solving the on-demand ridesharing delivery tasks.
Ridesharing raises a number of public interest questions. Rayle et al. (Citation2016) collected completed surveys on the use of public transit and ridesharing in San Francisco. They showed that speed (e.g. short waiting time) and convenience (e.g. easy to call a car) were the main attractions for choosing ridesharing. Thus, the development of a ridesharing service has to take into account the user’s requirements, which also include: origins and destinations, trip purpose, acceptable ride time, as well as rider satisfaction in matching drivers, and routing of drivers to pick-up and deliver riders. This raises the possibility of using harvesting techniques to extract specific types of travel-related information. However, the potential of information harvesting to automatically (or semi-automatically) abstract data for ridesharing services is still unexplored. Three very real issues need to be resolved:
The governance of ridesharing systems may be undertaken by different authorities. As a result, the data that is collected is kept private by transit authorities and car manufacturers, which operate and manage their own sections of the overall system. The lack of sharing information in transportation presents significant difficulties for data harvesting. Additionally, the main barrier to automating information harvesting is the lack of a methodological framework for representing objects such as bus lines, and semantic annotation. Obtaining the data could be very cost effective and would provide text processing to associate objects with pre-specified transport-related topics, e.g. reporting transport-related incidents.
Most data posted by a transport authority (or supplier) will only have relevance to a particular geographic location. Although the road network has been incorporated to locate where transport activities or specific transport services have been accessed, it is still necessary to annotate such data with useful semantic information for further sentiment analysis on ridesharing, e.g. expressing an opinion regarding traveling with strangers. However, merely using the geo-location data cannot identify subjective perceptions of the experience of ridesharing.
With the ever-growing complexities of urban transit, many transit authorities do not have the means to monitor zones with high levels of activity (Collins, Hasan, and Ukkusuri Citation2013). Existing text mining techniques can assist in understanding regular overcrowding (e.g. during peak time) and thus make a detour to avoid the hotspots (Lin et al. Citation2017). Also, understanding the reasons that crowds form, especially in unexpected circumstances, is useful for adjusting the pickup times and minimizing the ride time of passengers. However, explaining unusual congestion due to special events is still problematic. It is therefore necessary to harvest data on the process of crowd formation, including the causes, duration and possible hotspots (Pereira et al. Citation2015).
To address these issues, we need information sources and methodologies for harvesting and evaluating an individual’s interests. The role that social media plays in transport decision making ranges from understanding choices and preferences (e.g. on routes and modes) to evaluating scheme participation (Grant-Muller et al. Citation2015). By incorporating social networks to form a social ridesharing community, user’s social links, interests, and concerns for the level of service quality, as well as travel activity patterns that influence trip choices, can be utilized in a wide spectrum of applications. provides an overview of a ridesharing community based on automatically matching potential participants who have strong or weak social links with each other. Unlike a centralized car-sharing or taxi-sharing system, drivers and passengers can exchange messages directly and coordinate their travel plans, such as the destination, drop-off location, and departure time, based on their current location and social network connections. Moreover, the user-generated content on social media allows passengers to voice their concerns in real time about current conditions in terms of safety, speed, and convenience etc. For example, Sina pages have ‘Like’ and ‘Share’ functions to indicate approval for posted messages and to distribute them to friends for seeking a ride-share partner. The feedback also contributes to gauging opinions on a range of topics.
In considering the role of social media in the ridesharing context, it is useful to first review the definitions and scope of ridesharing services and the opportunity that social media presents for the transport sector and participants. This forms the backdrop to the remainder of this survey, where the methods of collecting required data and current uses of social media in ridesharing are detailed using examples from recent research and real-life practice. We first analyze the literature on ridesharing, and introduce a framework to define the building blocks required for a ridesharing service in Section 2. We then uncover the kinds of valuable information that can be extracted from social media for improving the ridesharing decisions taken by participants. In considering the challenge of analyzing such information, Section 3 also presents the rationale for harvesting the data from some popular sources. Next, we detail some possible directions for using the valuable information to support the aims of mobility, safety and environmental sustainability in ridesharing. Section 4 also briefly describes different approaches followed by the community for the development of social ridesharing, highlighting their advantages and drawbacks. Section 5 discusses the current state and future directions in this area and Section 6 concludes the survey.
2. Shared rides service options
Several ridesharing services have been designed to enable drivers to efficiently share their cars with other travelers (Campana, Delmastro, and Bruno Citation2016). These services encompass a variety of types ranging from slugging, regular and causal carpooling, vanpooling/microtransit, ridesplitting, as well as dial-a-ride (Li et al. Citation2017; Ma and Wolfson Citation2013). Some differentiate shared services between: prearrangement by a service provider (match the supply and demand for riders in advance, e.g. vanpooling, dial-a-ride where the vehicles generally include vans and buses), and semi-organized modes (routing drivers to pick up and deliver passengers, e.g. causal carpooling, slugging, ridesplitting, where the drivers are private independent entities) (NASEM Citation2016). To review the literature on ridesharing, we used keywords for the different service type categories, and searched for them in the ‘Topic’ of publications indexed by the Web of Science database. A semi-automated filtering process was then conducted. Finally, a dataset of 388 papers published during 2000–2017 was collected for this study. More than 96% of the records consisted of journal papers. This result shows that studies published by journals remains the predominant method of dissemination in this field. shows the number of publications in the top-10 sources which are mainly from transport-related journals (a total of 151 records). We used the sum of citations as an estimate of the quality of the publications. As shown, Transportation Research Part B is ranked first in a number of citations followed by the European Journal of Operational Research, which is an operations research and management science journal.
Table 1. Source titles of publications including number of publications and citations.
In , although ridesharing related research still occupies only a small portion of the transport domain, the field of study is attracting more attention and is still growing. By analyzing keywords contained in all collected papers, we obtained a general view of research topics in this domain. 787 distinctive keywords were captured after the process of keyword extraction, filtering and grouping similar words. For example, ‘problem’, ‘algorithm’, and ‘transportation’, that make a small contribution to this field were dropped.
Figure 2. Number of papers published and citations in this field per year: (a) Total publications; and (b) Sum of times cited.
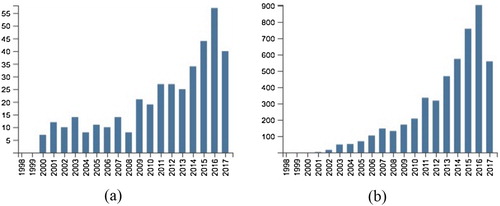
The occurrence-frequency of the most cited keywords can be visualized using a world cloud. From , we observed that the studies on (dynamic) dial-a-ride (90), (dynamic) ridesharing (51), carpooling (47), vehicle routing (23), and (static/dynamic) pickup and delivery (19), attracted the most attention. Dial-a-ride and carpooling are common ways to generate ridesharing plans. The difference between the two is whether the commitments are required in advance. Accordingly, the main issues in carpooling and dial-a-ride are assignment of riders with pickup requirements to drivers and the identification of each vehicle’s route respectively.
The analysis shows that matching and routing have been widely studied in the literature, creating the impression that ridesharing services are usually classified as either matching or routing. The driver-rider matching services predict individual willingness to share a ride according to travel requirements such as trip purpose, mode of transport and land use variables. Conversely, the trip routing services assign riders to drivers and construct feasible routes for drivers to minimize the travel costs. Examples include public vehicle systems (Zhu et al. Citation2016) and dedicated high occupancy vehicles that enable more convenient and flexible trips on request.
However, more needs to be and can be done. Additional services could include assigning a role to each of the participants or the pricing of ridesharing fares and so on. In this section, we analyze ridesharing services in terms of their properties and model, and finally introduce a framework, with which can better determine, classify, and evaluate how social media data might support or obstruct the goals of mobility, safety and environmental sustainability in ridesharing.
2.1. Features of ridesharing services
Before providing a framework for ridesharing services, we need to define how users plan their shared rides in advance by looking at currently available examples. We outline the needs that the service has to satisfy, the main features of the service itself, and the perceived value of it, both from the passenger and the driver’s point of view. A scenario is depicted in where there are three different locations that are connected and easily reachable by car. Passengers and drivers must know in advance all the details of the trip, and both parties agree on factors such as the time and the location of departure and arrival.
In this scenario, TINA is one driver located at place A, while PETER and BOB (who is the car-owner) are located at place B. These people have several interests that lead them to end at the same final destination C. BOB and TINA put some conditions on sharing their ride with others, in addition to the cost that he/she will share with the others. PETER logs into the service, selects the journey time and other details, and then selects, among different proposals, the one that best fits his/her individual needs, such as car type, user profile etc. PETER determines the meeting point and uses TINA’s car as the appropriate means of transportation, as well as making payment for the rides. The confidence in this service is ensured by a rating between PETER and TINA.
According to the above scenario, drivers and passengers can take a variety of steps to address the functionalities of ridesharing services. These include creating a personal motive to carpool, communicating this motive with others, negotiating and executing an agreed plan, as well as proving appropriate feedback (Galland et al. Citation2014). In particular, the statement of the service was based overall on the dynamic and real-time matching between passenger and driver (Gargiulo et al. Citation2015).
In terms of functionality, we suggest that the ridesharing service should be ‘accessible at any time’, ‘enable making the best decision’, ‘allow individual rationality’, ‘quickly respond to demands’ and have ‘ex-post incentive compatibility’. On the one hand, ‘accessible at any time’ allows every passenger to dynamically raise his/her ride request at various times and locations in a process that may be difficult to predict with certainty. On the other hand, ‘enable making the best decision’ refers to on-demand ride matching where riders with similar origins and destinations are matched to the same driver and vehicle in real time, and the ride and costs are split among the passengers. Several constraints on the feasibility of matches must be observed. Besides the timing of rides and the availability of spare seats, driver preferences (e.g. a maximum deviation from the direct route (Agatz et al. Citation2012)) needs to be accounted for in the ride-matching. A good recommendation system helps decrease the driver’s pressure-based risk factors associated with rushing to accept matches and pick up passengers (NAIC Citation2015). Another important aspect of practical ridesharing services is ‘individual rationality’. This is where the shared costs of passengers who accepted their fare quotes are saved for a future time, and the driver can be reimbursed for costs (e.g. gas, tolls, and parking) (Chan and Shaheen Citation2012). Considering the personal use of vehicles by drivers and passengers, rationality is modeled to maximize the satisfaction of people sharing a trip according to people’s interests, social links, and preference for connecting with others (Berlingerio et al. Citation2017). We also have to add a property, ‘quickly respond to demands’, which is replying to passengers immediately after submission of their ride request to minimize uncertainty about whether they can be serviced or how high their fares might be (Furuhata et al. Citation2015). This property contributes to reduce the likelihood of passengers dropping out. Finally, ‘ex-post incentive compatibility’ defines the trustworthiness of services through voting. It ensures that passengers consider the fares to be fair and provides incentives for passengers to submit their ride requests truthfully. Also, the driver’s trust should be guaranteed by the incentives provided. For example, drivers would like to know more about the matching and trust the service when it comes to sharing a ride with people they have never met (Gargiulo et al. Citation2015).
2.2. Ridesharing service model
Shared use of a vehicle has become a ubiquitous part of the urban transportation network, encompassing a variety of modes providing on-demand ride and delivery services. Together these services, coupled with traffic data sharing (e.g. congestion, parking, and public transportation), continue to facilitate last-minute planning and delivery selections (Shaheen, Cohen, and Zohdy Citation2016). Users can then combine information from multiple services and make smarter, more informed travel decisions to meet their own mobility needs. Conversely, the diverse needs of users may require various services, such as to provide insurance, gasoline, parking, and maintenance, and the participants pay a fee each time they use a vehicle. Traditional transportation methods are unable to accurately capture the ridesharing model, the time-space distribution of real-time ridesharing on the broader transportation network, and its evolution over time (Alexander and González Citation2015; Correia and Viegas Citation2011). We suggest that better metrics, modeling and methodologies for ridesharing services are needed to help travelers and public agencies participate in decisions by understanding the role of these services, and ultimately foster the participation of individuals in shared ride services in their transportation planning and decision-making processes.
Considering that vehicles, pedestrians and roadside units (RSU) can exchange messages directly (Zhao et al. Citation2014), the distributed placement of ridesharing services should be more representative of the transportation environment. In particular, these services are maintained and deployed at many locations within neighborhoods, public transit stations, employment centers, colleges/universities, and in-car systems. Each service participant plays a role, and can be helpful in optimizing the management or archiving of automated requests and the vast amount of traffic data. We have defined the service model as shown in and depict shared rides across an ecosystem of transportation services, generally (see ).
The model consists of five components: (i) rider agent (i.e. service data consumers); (ii) service publishers (e.g. drivers, RSU, and public transit stations); (iii) planner; (iv) distributor; and (V) conductor. The causes of change in the transportation behavior of users are assessed to forecast their requests on services as the inputs to a planner. A set of tasks and several complex steps within each task are then generated according to the ride requests raised at any time. Coupled with environmental awareness, a flexible delivery of request or service data is conducted by a distributor in the broader transportation network. Considering that the demand-responsive connections between drivers and passengers may not work well due to sudden traffic incidents, a try-best forwarding by transferring data to the relay transportation will be done. The distributor thus enables response to passenger demands in real-time. A conductor makes a decision on ride planning and cost sharing for individual rationality. The candidate ridesharing services offered in a distributed way can be then located by collecting demographic data and understanding casual user behavior. Furthermore, the conductor allows the passengers to vote on the services in terms of being satisfactory and trustworthy, giving drivers and passengers confidence in traveling by the ridesharing mode. The resulting online-enabled application is finally created by the conductor which puts the candidates, whose journey sections make up their activity on an event-driven basis, together (Butzin, Golatowski, and Timmermann Citation2016). This way we can monitor for successful execution, but also can add new ridesharing services on demand.
Shared ride services can be better understood by being incorporated into a planning pipeline, which includes transportation land-use, trip cost estimation, etc. Every pre-trip request of service types can invoke a journey plan on the basis of informed choices (e.g. of the desirability of travel, routes, transport modes and departure times). We illustrate the process that generates origin-destination (OD) pairs and times (see below). At the time of listing drivers and passengers, it enables the user to find his/her potential partners between either drivers or passengers (Furuhata et al. Citation2013), or passengers sharing a trip together (Deakin, Frick, and Shively Citation2010) and incorporate any special transportation needs of individuals. The user will next select an optimal on-trip service to adapt trips to actual events and incidents; selecting right up to the moment when the user reaches his/her destination. This way, a personalized travel plan can continuously select from a set of candidate services. Among various plans, the user will optimize the trade-off between benefits (gain short-term access to a vehicle without the costs and responsibilities of ownership), and costs, and finally confirm the selection. indicates that the demand types of ridesharing participants are of critical importance in service selection. For example, commuters with a regular work schedule and long-term relationships, are more likely to choose services that are economical, work-friendly, punctual, and safe. Therefore, recognizing the influence of individual or group behavior is a key component of service choices and transportation planning.
2.3. A framework for ridesharing services
Giannopoulos (Citation2004) defined several categories of information used in transport analysis. In , we highlight the role of this type of information in encouraging ridesharing by introducing a framework to show the cooperation of the building blocks in conducting a ridesharing service.
Road Networks: The contiguous road segments identified by interchanges, ramps and shortcuts (Pelzer et al. Citation2015), the unexpected incidents, as well as the predictable POI (points of interest) a user visits frequently, combine to create a heterogeneous real-world road network. This block supports the features of ridesharing services such as ‘quickly respond to demands’ and ‘making the best decision’.
Trip Constraints: This corresponds to the process of making an individual decision on travel that should satisfy various requirements. It may be considered as a prearrangement or an estimation of desired OD-pairs, travel time, the areas within a specified radius from pick-up locations, unexpected changes of schedule, and personal safety when traveling with strangers. As a key part of this framework, it can recognize ‘Travel Needs’, and further determine what type of patterns can work well for ridesharing, as well as implement features such as ‘allow individual rationality’.
Group preference: It is better for individuals to find a regular partner and group with similar preferences when sharing a ride (Elbery et al. Citation2013). Group preference promotes trust in ridesharing, where each member of the group should be familiar with at least one other group member. Therefore, it helps in conducting a service that allows for ‘ex-post incentive compatibility’.
Driving mode: Drivers in a ridesharing community are often motivated to pick up unfamiliar passengers to take advantage of high occupancy vehicle (HOV) lanes, lower tolls, and similar benefits (Shaheen, Cohen, and Zohdy Citation2016). In many cases, drivers accept the ride-share arrangements, not only in terms of minimum deviation from his/her own trip duration, but also of a maximum number of simultaneous riders (Agatz et al. Citation2012) and so on. This block influences ‘Ridesharing Patterns’ and ‘Travel Needs’, as it may alter the departure and arrival time of the driver considering the route as well as the ridesharing motivations.
Travel Needs: Identifying the requirements of participants and then incorporating them into trip planning processes can help understand an individual or group’s traveling lifestyle. These needs arise dynamically over time at various locations in a process that may be difficult to predict. This block has an impact on ‘Ridesharing Patterns’. For example, drivers can adopt a partial journey pattern (Furuhata et al. Citation2013) where riders with similar origins and destinations will be picked up along the driver’s personal trip routes when they are traveling to and from work. This block, in combination with ‘Ridesharing Pattern’ can act as a guide for decision-making in ride-matching.
Ridesharing Patterns: A rideshare route can be macroscopically divided into a sequence of partitions by pick-up locations requested dynamically over time. Different participants may have diverse needs, which affect a driver’s selection of the next stop when serving the passenger(s). Alternatively, the drivers may choose their patterns according to their driving modes, i.e. reducing the marginal travel cost required by detours necessary to accommodate passenger(s).
3. Generating ridesharing service related information from social media
Outside of existing transport data sources, online social media can serve as a prediction tool to enhance a rider’s ability for decision-making about travel scheduling. Therefore, in this section, we identify the needs for social data concerning the interests and relationships of participants in various aspects of ridesharing services, according to the building blocks introduced in Section 2. We also give a brief introduction to emerging platforms to show how social media can serve to find relevant information for organizing a ridesharing scheme at the end of this section.
3.1. Remarks on requirements for social media
Gaining access to vast amounts of performance metrics of ridesharing that measures: ridership, timeliness, efficiency, safety, and service coverage is a challenging issue for transit authorities (Collins, Hasan, and Ukkusuri Citation2013). Social data analysis can assist agencies in gauging these metrics based on rider sentiments by monitoring social media outlets (e.g. Twitter, Facebook). Meanwhile, passenger dissatisfaction with services due to the misallocation of scarce resources and driver attitudes would also be improved through the use of online social media.
The characteristics of social media are of particular value for informing short-term and dynamic decisions in ridesharing. We first assess how primary data needs in ridesharing are currently addressed using social media. In , the various social media are examined to develop common definitions of service objectives summarized from the framework adapted from Grant-Muller et al. (Citation2015).
Table 2. The role of social media information in ridesharing services.
Although existing sensing techniques such as wireless sensor networks (WSNs) facilitate vehicle assignment and route planning, they usually only solve critical traffic tasks in particular geographic areas (typically downtown) due to insufficient node coverage, high installation/maintenance cost, and lack of scalability (Guo et al. Citation2014). The sources of transport related social media may help the participants to acquire and share local user-contributed data, e.g. location, personal and surrounding context, traffic conditions, and further unknown ride offers and requests. For example, users can not only post text messages, but also use ‘Like’ and ‘Share’ functions on the social media platform. With participatory sensing, such information can be obtained to seek a potential ride-sharing partner by reusing both online and offline data. As shown in , the biggest concern is to have the potential to aggregate traveler information that can serve as a basis for identifying major needs and the perceived satisfaction for ridesharing services.
3.2. Data collection for characterizing the ridesharing problem
The traditional skills of transport engineering typically focus on naturally occurring data (Ritchie et al. Citation2013) which is an enactment of travel behavior in its own traffic setting. The main methods involved in working with naturally occurring data in the transportation platform are observation and documentary analysis (Hall and Krueger Citation2016; Lv et al. Citation2015). Rayle et al. (Citation2016) adopted a documentary analysis to conduct an intercept survey of ridesharing customers based on public documents (SFMTA Citation2015) in San Francisco. Another data collection method is widely used in more applied transportation domains. This focus means that more attention is given to the study of generated social data. Engaging with such data allows the participant’s reflections on transportation-related issues to generate insight into their motivations, decision-making process, and most crucially, the meaning that they attach to them. The collection methods for generated transport data at various levels of aggregation have already been recognized (Grant-Muller et al. Citation2014). This indicated that text mining needs to be carefully adapted and applied to elicit transport-related content contributed by users of social media.
Although there are social media platforms dedicated to reporting transport-related information (Grant-Muller et al. Citation2015), only a few studies have been performed on engaging with social media information for ridesharing in practice. As some techniques to generate transport related information are not specific to the ridesharing domain, an overview of some data sources, including different sources used in various fields is provided in this section. Some solutions which form the basis of the rationale to harvest the data from these sources are then presented in Section 3.2.2.
3.2.1. Data sources for ridesharing related information
Documentary sources have been playing a significant role in helping to easily access people’s ridesharing plans (Correia and Viegas Citation2011). For example, Liu, Zhang, and Yang (Citation2017) extracted household travel survey data to identify departure times based on all household member’s preferences for a shared ride of family members. Such data from traditional tools such as web-based transport surveys and questionnaires is particularly useful where the history of travel events or daily schedules has relevance. Yet, the cost of data collection tends to increase over time.
Microsoft’s GeoLife, ‘Building Social Networks Using Human Location History’, is a database dedicated to reporting individual’s travel sequences using sparse GPS data from sections of a multi-modal journey. Parts of this dataset were labeled by meaningful locations, e.g. home, work, which made them useful for performing ridesharing group recommendations (Lee and Liang Citation2011) or road network matching (Zheng et al. Citation2012) from check-in displacements retrieved. Sharing check-in histories information to provide general road networks also appears in more general social media platforms, such as Twitter, Facebook and Sina. WAZE (www.waze.com) allows user-submitted travel times and route details for estimating road conditions that facilitate finding rides that can be shared. These are not specific to the ridesharing domain, but transport-related information that is arguably the most crucial for matching users and paths can be found in most forms of social media. For example, Twitter data was applied to extract origin-destination trips as used in some ridesharing group query models. Lee, Gao, and Goulias (Citation2016) conducted trip planning by harvesting geo-locations from tweets in the Greater Los Angeles metropolitan area. For the origin-destination trips, treated to recognize ridesharing behavior, cell phone data, often containing and storing the call description record (CDR) over time and from different BTS towers (Çolak et al. Citation2015), can also provide a different point of view on the duration of OD-trips compared with social media. The subjects gave consent to be geotagged and have their data aggregated to identify their home and work locations as well as their commuting routes.
Considering that the data harvested from social media can complement traditional data collection (Maghrebi et al. Citation2015), various data sources were aggregated to capture similar mobility patterns using data fusion on social ties between users (Lee, Gao, and Goulias Citation2016; Shin Citation2017). This can help to further assess the potential for numerous transportation, land use, environmental, and social benefits that can be achieved by ridesharing (Alexander and González Citation2015; Correia and Viegas Citation2010; Cici et al. Citation2013).
3.2.2. Rationale for information harvesting in ridesharing
It is important to identify both the most valuable information for improving the ridesharing decisions made by participants and the most efficient extraction method of extracting it from a social media platform. These building blocks, shown in , specify the goals of harvesting ridesharing service related information. A ‘ridesharing service’ involves the pairing of drivers and riders, as well as the routing of drivers to pick up and deliver riders. It also includes a range of auxiliary services such as route planning, information dissemination, and payment and rate bids. The ‘data needed’, as listed in , can thus serve as the goal of the harvesting process.
The data posted on social media is unstructured and usually lacks contextual information (Minkov, Wang, and Cohen Citation2005). Thus, research is increasingly turning towards the processing of unstructured content (Bollen, Mao, and Pepe Citation2011; Schulz, Ristoski, and Paulheim Citation2013). Most of the solutions for transportation started decades ago with analyzing transport service related information, including accident reports and opinions on the quality-of-service. Moreover, they all have employed text mining to automatically (or semi-automatically) harvest valuable information. For a complete review and comparisons of such solutions, the interested reader can refer to (Grant-Muller et al. Citation2015).
In the ridesharing domain, geolocation (or spatial) based contents provided in social media can also be investigated to facilitate services, rather than only using geotagged data. In Maghrebi et al.’s (Citation2015) work, a set of potentially relevant messages must first be extracted from Twitter’s streaming API with a geo-location filter. The textual contents were then annotated by seven transport-related activities, and can be used to assist in further decoding the location activity pattern of social media users. The automatic association of a message with activities in four time-slots typically used text-mining technologies. Messages judged as relevant to activity patterns may be useful for such tasks, for example indicating the location of home, school and work, and recommending O–D, locations at which the passenger is likely to be soon (Lee and Liang Citation2011). Similarly, Ni, He, and Gao (Citation2017) filtered social media messages with the goal of eliciting tour events. Unlike existing semantic annotation methods, they created a hashtag matrix for every four-hour interval where the frequency of the hashtag denotes the occurrence of topics labeled by Twitter users. Thus, the relevance of annotated messages to the topics can be more thoroughly evaluated, and assist in identifying the most frequently occurring hashtags for characterizing transportation-related events. Although they focused on forecasting passenger flow, especially under event occurrences, the high-quality harvesting methodologies can facilitate route planning by keeping away from identified hot spots.
4. Using social media in ridesharing
Understanding people’s attitudes, beliefs, and travel behavior in real-world contexts is a much more technically challenging task. This section provides a short overview of the evidence on how social media can potentially help to bridge gaps in existing ridesharing systems, specifically:
How social media is being used in terms of, for example, generating dynamic shared-ride plans, improving group queries, optimizing ride matches, and for up to date information notices or other purposes involved in ridesharing.
Whether there is evidence of social media data to reveal the level of satisfaction with ridesharing services.
4.1. Daily pickup and delivery planning
Knowing users’ intensions for requesting trips when and where they are, helps recommend an appropriate pickup location and optimize each driver’s route from there to the rider’s destination (Santos et al. Citation2011). It can be considered as an Origin-Destination (OD) estimation for different purposes (home, work, other) and times of day. Usually, the use of location-sensitive data from CDR are explored as an ultimate solution in generating OD-trips (Çolak et al. Citation2015). However, low spatiotemporal coverage of a network due to distributed cellular zones limits the resolution of data collected. Instead, focusing on the content of social media rather than only using geotagged data enables the discovery of the users’ activities, e.g. entertainment, shopping and work, and forecasting when to start a new trip. Jin et al. (Citation2014) improved the gravity model to estimate an origin-destination (OD) matrix in Austin. It showed that a doubly-constrained model works better in the creation of production and attraction rates.
Unexpected transport overcrowding (Pereira et al. Citation2015) and traffic congestion should be understood, reported (Miller and Gupta Citation2012; Pereira, Rodrigues, and Ben-Akiva Citation2013), and passed into route planning (Pereira, Bazzan, and Ben-Akiva Citation2014). Kuflik et al. (Citation2017) confirmed that automatic harvesting of relevant, valuable transport events from Twitter is of great value. They created a relevant transport taxonomy and identified such events, including ‘traffic’, ‘fire’, ‘bridge’, ‘stuck’, and ‘failure’, using classifiers. Pereira et al. (Citation2015) treated the non-habitual overcrowding hotspots as a set of topic-based explanatories from social networks. They identified the impact of these hotspots by adding up the excess amount of arrivals in 30 min. This made it possible to retrieve the data showing when and where the hotspots were due to announced social events. Schulz, Ristoski, and Paulheim (Citation2013) focused on small scale incidents, e.g. car crashes, from Twitter. They classified the events into different semantic incident types by taking advantage of temporal and spatial constraints. Using very few social media posts, they claimed to be able to detect 15 types of incident from the government dataset. Similarly, Pereira et al. (Citation2015) explained mechanisms for the formation of unusual overcrowding hotspots and predicted the manner of their evolution. They collected special events from social networks and websites to quantify the impact of the simultaneous events associated with three areas, and to help transport managers understand the demand for public transport. To actively help passengers minimize their ride time on HOV lanes and toll roads, Wang, Dessouky, and Ordonez (Citation2016) suggested that traffic congestion be treated as the change in passenger travel time and toll cost due to vehicle load. In these cases, the pickup time can be adjusted heuristically by coordinating the benefits of people in a vehicle.
4.2. Co-passenger determination
Due to the reduced flexibility compared with using a private car, a person becomes more dependent on his/her partners when ridesharing. Instead of assessing the compatibility of potential partners on the basis of geographic proximity alone, we believe that psychological factors including relationships with car mates, constraints to independence, status as a passenger or driver, should be considered to influence the decision to share a ride or not (Oppenheim Citation1979). This view has been confirmed by a growing tranche of literature concerned with the attitudes and perceptions of individuals on the safety aspects of ridesharing. Mutabazi and Ackbaralib (Citation2010) reported the attitudes of students, parents and guardians on ridesharing for school trips by a questionnaire survey. It ranked concerns over traffic safety and personal security as the main reason for unwillingness to take part in car sharing.
Sentiment analysis has been applied over social media data to assess passenger satisfaction. On-going analysis is being conducted on levels of satisfaction with public transport (Collins, Hasan, and Ukkusuri Citation2013; Pender et al. Citation2013; Grant-Muller et al. Citation2015). Due to personal safety or social considerations (Agatz et al. Citation2012), we need to translate such social data into features that are relevant for a format of ridesharing that passengers and drivers would accept. Therefore, building trust between unacquainted ridesharing pairs and determining whether there are other rides in which people would prefer to travel together are essential attributes to building trust in ridesharing (Cici et al. Citation2014). The former technique could provide pairs with the opportunity to rate each other, and a diverse range of solutions was cited in Furuhata et al.’s work (Citation2013). This study aims to appraise the sentiment toward the latter.
de Almeida Correia, de Abreu e Silva, and Viegas (Citation2013) investigated the idea that people may feel more comfortable when riding with his/her friends or a regular partner, going from an acquaintanceship-based group (a household or a company). It also has been shown in Cici et al.’s work (Citation2014) that the decrease in the number of cars in a city is significantly higher when users ride with friends and two-hop friends (friends of friends). Due to the route overlap under human mobility patterns, they matched spatially and temporally similar trips and generated rideshare plans. Engaging with social media, as we have said, may carry an important advantage in determining the level of acquaintance between two riders and improving group queries. Elbery et al. (Citation2013) searched an individual’s direct and two-hop friends to identify potential co-passengers living in close geographic proximity using their check-in data. Also, Berlingerio et al. (Citation2017) claimed that it is possible to seek traveling buddies who have similar preferences on traveling instead of being from the same household or friends. They modeled people’s interest in sharing a trip from Twitter and a trajectory stream, to facilitate scalable and flexible companion discovery (Tang et al. Citation2013) by maximizing the quality of ridesharing. In particular, the GRAAL project performed an online survey to refine the model from crowd-sourced data, which expresses people’s approval of the ridesharing experience.
4.3. Agent-based simulation
For evaluation of ridesharing advisory services, the development of simulators started decades ago with the modeling of rerouting and activity rescheduling along with ride matching. The agent-based paradigm is a valuable approach because it allows for modeling interactions between individuals to reach an agreement on the route (Bellemans et al. Citation2012). For example, most of the agent-based simulation tools, such as TRANSIMS (Smith et al. Citation1995), MATSIM (Waraich et al. Citation2009), the work of (Bellemans et al. Citation2012), ABC (Kamar and Horvitz Citation2009) and ANDIAMO (Sottini, Abdel-Naby, and Giorgini Citation2006) have been developed to analyze travel demand and ridesharing plans at both the microscopic and macroscopic levels. Agent-based modeling tools allow the whole effect of each agent’s action on all the others to be considered.
We would like to determine whether social media can provide the information necessary for simulation. To answer this, we have to understand the characteristics of ridesharing. As Galland et al. (Citation2014) have found, the procedure of ridesharing with detailed agent-to-agent or agent-to-environment coordination, includes motive creation, plan negotiation, and execution. To simulate preferences for finding a carpool partner, the agent’s interests and social links should be incorporated as initial data. Hussain et al. (Citation2015) proposed an agent-based social simulation (ABSS) which explored social contexts, to group agents and facilitate interactions according to their agenda. ABSS simulated a carpool by sending a text message to a randomly chosen agent in the social group. In contrast, Galland et al. (Citation2014) created a private social network (PrivNet) to denote each agent’s profile including its role and the relationships with others. PrivNet identified the preferred candidates for ride matching in terms of: start location, OD, interests and requirements, from the agent profile.
Although a few teams that have developed agent groups used social contexts, these tools have not been designed for the execution of the carpooling process, e.g. rerouting and pick-up planning, with social media. Also, the feedback of information from the social interaction should be simulated for further activity rescheduling.
5. Discussion
The study of ridesharing started in the 1940′s for analyzing the travel patterns of commuters in order to decrease urban congestion. It has been revived recently with the sudden interest in harvesting transport-related information from social networks, and the lack of metrics and models for measuring environmental and economic impacts. After years of new developments, we propose in this section to establish the current status and identify the new challenges ahead of us.
The objective of the research community was first to understand the effect of ridesharing on a person’s mobility routines, both in a positive and a negative way. However, the results of this survey indicate that the impact of studies of on-demand ride services is somewhat limited. A few research programs have conducted national and regional travel surveys to record their impact in some specific cities, e.g. Lisbon (Correia and Viegas Citation2011) and San Francisco (Rayle et al. Citation2016). The challenge for evaluating the effect of ridesharing using this type of survey is the lack of data which can be used to explore the changes before and after a person begins using the service. Our proposal is to introduce a set of criteria to enable a comparison with traditional transport services. These criteria have been defined based on feasible measurements of the magnitude of the changes of factors such as travel cost, travel-time savings, and reduction of commuting stress, at the city and regional level. A question that is still open is how to evaluate the participant-level change in user experiences. For example, ‘do not need to park’ and ‘did not want to drive after drinking’ should be treated as benefits users gained from existing services. Due to better textual content and weighted friendship information, we believe that applying a survey on social media can enable a better understanding this type of potential impact by taking people’s interests into account.
One could argue that some services might only need information on trip origins, destinations, and departure time to query the potential partners, while some trust-conscious ridesharing could need to obtain psychological data in order to assess the compatibility of potential riders. Selecting a data source thus depends on the requirements of the specific applications. We believe that the choice of source is affected by several considerations. First, the source should be public and provide both location and textual content for the same users. Additionally, it should provide the same type or amount of data using a user-friendly API. Further, the incompleteness of data, representing the fraction of hour-long intervals when the user-related data is unknown to us, is not common in the data source for high-dimensional matching.
Another problem that should be addressed relates to the emerging services, such as the pricing and rating of drivers and passengers. Ridesharing participants are motivated by sharing travel costs with non-familiar persons so a strong trust basis is needed. A remaining challenge is to allocate costs to participants when taking an unplanned detour that would lead to additional travel costs and detour-time incurred by the other parties. Making a detour beneficial for all passengers has a significant impact on the future possibly of participation (Furuhata et al. Citation2013). Yet, it is difficult to determine if joint agreements of intra-vehicle participants can be reached. By studying the psychology and social behavior of all passengers, we are able to analyze their opinions regarding a detour using the friendship and trust relations extracted and thus maximize the satisfaction of passengers sharing a trip in the case of detour ridesharing.
Beyond designing novel mechanisms for desirable ridesharing services, travel-related information delivery between pairs often necessitates dealing with the unreliable communication links. We may consider that information delivery will impact service quality and matching reliability. For instance, both drivers and passengers may waste a lot of time when there is a traffic jam on the way to pick up passengers, and this may induce some participants into giving up the ridesharing arrangement (Zhao et al. Citation2014). However, traditional data-disseminating mechanisms are not perfect. First, the movement patterns (for example, speed, direction, and the stages of rest) of many vehicles can affect the quality of information delivery. Second, high traveling speeds can alter the traffic-flow density and affect the transfer of data. Finally, when the communication equipment is not running or road obstructions cause traffic to divert, the transmission link may experience short-term congestion. One approach to solve this issue would be the use of intermediate vehicles that serve as store-and-forward message switches when transferring travel-related information opportunistically.
The questions of the ethical, privacy, and security implications of aggregating social media data remain open. Presumably, using information derived from social media should be unproblematic because it is already in the public domain. However, it is unusual to put the physical location of the home and work on social media in the interests of personal security and a degree of anonymity. In the ridesharing application, the user would be physically present and frequent regular trips would allow their home and work addresses to be discovered easily. Hence, the aggregated data proposed here, perhaps even combined with psychological data, has the potential to be used by unknown people for purposes different from those intended by the user. This does not mean that social media data should not be used or aggregated, but there is a need for careful consideration of the data that would be collected and stored, individuals with access to it, secure storage, to ensure the privacy and security of users.
6. Conclusions
As a prospective shared mode of travel, ridesharing has recently been attracting increased attention from both the research and industrial communities. The main issue for ridesharing is to how to assign the passengers to drivers and identify each vehicle’s route to meet the participant’s trip demands and preferences. Such particular needs make geo-location data source and harvesting technologies inefficient in identifying subjective perceptions of the experience of ridesharing. In this survey, we presented five general attributes of a ridesharing service and defined the service model to view shared rides across an ecosystem of transportation services. Then, we presented a framework that highlights the role of information use in ridesharing and identified the needs and harvesting technologies for social data in ridesharing services. Finally, we described some possible directions for using social media data in developing ridesharing systems.
Acknowledgements
We thank LetPub (www.letpub.com) for its linguistic assistance during the preparation of this manuscript.
Disclosure statement
No potential conflict of interest was reported by the authors.
Additional information
Funding
References
- Agatz, N., A. Erera, M. Savelsbergh, and X. Wang. 2012. “Optimization for Dynamic Ride-sharing: A Review.” European Journal of Operational Research 223 (2): 295–303. doi:10.1016/j.ejor.2012.05.028.
- Alexander, L. P., and M. C. González. 2015. “Assessing the Impact of Real-time Ridesharing on Urban Traffic Using Mobile Phone Data.” 4th International Workshop on Urban Computing (UrbComp 2015).
- Basiouka, S., and C. Potsiou. 2014. “The Volunteered Geographic Information in Cadastre: Perspectives and Citizens’ Motivations Over Potential Participation in Mapping.” GeoJournal 79 (3): 343–355. doi:10.1007/s10708-013-9497-7.
- Bellemans, T., S. Bothe, S. Cho, F. Giannotti, D. Janssens, L. Knapen, C. Körner, et al. 2012. “An Agent-based Model to Evaluate Carpooling at Large Manufacturing Plants.” Procedia Computer Science 10: 1221–1227. doi:10.1016/j.procs.2012.08.001.
- Berlingerio, M., B. Ghaddar, R. Guidotti, A. Pascale, and A. Sassi. 2017. “The GRAAL of Carpooling: GReen And sociAL Optimization from Crowd-sourced Data.” Transportation Research Part C: Emerging Technologies 80: 20–36. doi:10.1016/j.trc.2017.02.025.
- Bollen, J., H. Mao, and A. Pepe. 2011. “Modeling Public Mood and Emotion: Twitter Sentiment and Socio-economic Phenomena.” Proceedings of the 5th International AAAI Conference on Weblogs and Social Media, 450–453.
- Borcuch, A. 2016. “The Sharing Economy: Understanding and Challenges.” International Journal of Humanities and Social Science Studies 2 (5): 77–85.
- Butzin, B., F. Golatowski, and D. Timmermann. 2016. “Microservices Approach for the Internet of Things.” 2016 IEEE 21st International Conference on Emerging Technologies and Factory Automation (ETFA), IEEE. doi:10.1109/ETFA.2016.7733707.
- Campana, M. G., F. Delmastro, and R. Bruno. 2016. “A Machine-learned Ranking Algorithm for Dynamic and Personalised Car Pooling Services.” 2016 IEEE International 19th International Conference on Intelligent Transportation Systems (ITSC), IEEE. doi:10.1109/ITSC.2016.7795857.
- Chan, N. D., and S. A. Shaheen. 2012. “Ridesharing in North America: Past, Present, and Future.” Transport Reviews 32 (1): 93–112. doi:10.1080/01441647.2011.621557.
- Cici, B., A. Markopoulou, E. Frías-Martínez, and N. Laoutaris. 2013. “Quantifying the Potential of Ride-sharing Using Call Description Records.” Proceedings of the 14th Workshop on Mobile Computing Systems and Applications, ACM. doi:10.1145/2444776.2444799.
- Cici, B., A. Markopoulou, E. Frías-Martínez, and N. Laoutaris. 2014. “Assessing the Potential of Ride-sharing Using Mobile and Social Data: A Tale of Four Cities.” Proceedings of the 2014 ACM International Joint Conference on Pervasive and Ubiquitous Computing, ACM, pp. 201–211. doi:10.1145/2632048.2632055.
- Çolak, S., L. P. Alexander, B. G. Alvim, S. R. Mehndiratta, and M. C. González. 2015. “Analyzing Cell Phone Location Data for Urban Travel: Current Methods, Limitations, and Opportunities.” Transportation Research Record: Journal of the Transportation Research Board 2526: 126–135. doi:10.3141/2526-14.
- Collins, C., S. Hasan, and S. V. Ukkusuri. 2013. “A Novel Transit Rider Satisfaction Metric: Rider Sentiments Measured from Online Social Media Data.” Journal of Public Transportation 16 (2): 21–45. doi:10.5038/2375-0901.16.2.2.
- Correia, G., and J. M. Viegas. 2010. “Applying a Structured Simulation-based Methodology to Assess Carpooling Time–Space Potential.” Transportation Planning and Technology 33 (6): 515–540. doi:10.1080/03081060.2010.505053.
- Correia, G., and J. M. Viegas. 2011. “Carpooling and Carpool Clubs: Clarifying Concepts and Assessing Value Enhancement Possibilities Through a Stated Preference web Survey in Lisbon, Portugal.” Transportation Research Part A: Policy and Practice 45 (2): 81–90. doi:10.1016/j.tra.2010.11.001.
- Deakin, E., K. Frick, and K. Shively. 2010. “Markets for Dynamic Ridesharing? Case of Berkeley, California.” Transportation Research Record: Journal of the Transportation Research Board 2187: 131–137. doi:10.3141/2187-17.
- de Almeida Correia, G. H., J. de Abreu e Silva, and J. M. Viegas. 2013. “Using Latent Attitudinal Variables Estimated Through a Structural Equations Model for Understanding Carpooling Propensity.” Transportation Planning and Technology 36 (6): 499–519. doi:10.1080/03081060.2013.830894.
- d’Orey, P. M., and M. Ferreira. 2015. “Can Ride-sharing Become Attractive? A Case Study of Taxi-sharing Employing a Simulation Modelling Approach.” IET Intelligent Transport Systems 9 (2): 210–220. doi:10.1049/iet-its.2013.0156.
- Elbery, A., M. ElNainay, F. Chen, C. T. Lu, and J. Kendall. 2013. “A Carpooling Recommendation System Based on Social VANET and Geo-social Data.” Proceedings of the 21st ACM SIGSPATIAL International Conference on Advances in Geographic Information Systems, ACM, 556–559. doi:10.1145/2525314.2525327.
- Furuhata, M., K. Daniel, S. Koenig, F. Ordóñez, M. Dessouky, M. E. Brunet, L. Cohen, and X. Wang. 2015. “Online Cost-sharing Mechanism Design for Demand-Responsive Transport Systems.” IEEE Transactions on Intelligent Transportation Systems 16 (2): 692–707. doi:10.1109/TITS.2014.2336212.
- Furuhata, M., M. Dessouky, F. Ordóñez, M. E. Brunet, X. Wang, and S. Koenig. 2013. “Ridesharing: The State-of-the-art and Future Directions.” Transportation Research Part B: Methodological 57: 28–46. doi:10.1016/j.trb.2013.08.012.
- Galland, S., L. Knapen, A. U. H. Yasar, N. Gaud, D. Janssens, O. Lamotte, A. Koukam, and G. Wets. 2014. “Multi-agent Simulation of Individual Mobility Behavior in Carpooling.” Transportation Research Part C: Emerging Technologies 45: 83–98. doi:10.1016/j.trc.2013.12.012.
- Gargiulo, E., R. Giannantonio, E. Guercio, C. Borean, and G. Zenezini. 2015. “Dynamic Ride Sharing Service: Are Users Ready to Adopt it?” Procedia Manufacturing 3: 777–784. doi:10.1016/j.promfg.2015.07.329.
- Giannopoulos, G. A. 2004. “The Application of Information and Communication Technologies in Transport.” European Journal of Operational Research 152 (2): 302–320. doi:10.1016/S0377-2217(03)00026-2.
- Grant-Muller, S. M., A. Gal-Tzur, E. Minkov, T. Kuflik, S. Nocera, and I. Shoor. 2015. “Transport Policy: Social Media and User-generated Content in a Changing Information Paradigm.” In Social Media for Government Services, edited by S. Nepal, C. Paris, and D. Georgakopoulos, 325–366. Berlin: Springer. doi:10.1007/978-3-319-27237-5_15.
- Grant-Muller, S. M., A. Gal-Tzur, E. Minkov, S. Nocera, T. Kuflik, and I. Shoor. 2014. “Enhancing Transport Data Collection Through Social Media Sources: Methods, Challenges and Opportunities for Textual Data.” IET Intelligent Transport Systems 9 (4): 407–417. doi:10.1049/iet-its.2013.0214.
- Guo, B., Z. Yu, X. Zhou, and D. Zhang. 2014. “From Participatory Sensing to Mobile Crowd Sensing.” 2014 IEEE International Conference on Pervasive Computing and Communications Workshops (PERCOM WORKSHOPS), IEEE. doi:10.1109/PerComW.2014.6815273.
- Hall, J. V., and A. B. Krueger. 2016. “An Analysis of the Labor Market for Uber’s Driver-partners in the United States.” NBER Working paper No. 22843. National Bureau of Economic Research, Cambridge, MA. doi:10.3386/w22843.
- He, F., and Z. J. M. Shen. 2015. “Modeling Taxi Services with Smartphone-based E-hailing Applications.” Transportation Research Part C: Emerging Technologies 58: 93–106. doi:10.1016/j.trc.2015.06.023.
- Hussain, I., L. Knapen, T. Bellemans, D. Janssens, and G. Wets. 2015. “An Agent-based Negotiation Model for Carpooling: A Case Study for Flanders (Belgium).” 94th Annual Meeting of the Transportation Research Board, Washington, DC, January.
- Jin, P. J., M. Cebelak, F. Yang, B. Ran, and C. M. Walton. 2014. “Location-based Social Networking Data: An Exploration into the Use of a Doubly-constrained Gravity Model for Origin-destination Estimation.” 93rd Annual Meeting of the Transportation Research Board, Washington, DC, January.
- Kamar, E., and E. Horvitz. 2009. “Collaboration and Shared Plans in the Open World: Studies of Ridesharing.” Proceedings of the 21st International Joint Conference on Artificial Intelligence (IJCAI-09), IJCAI Organization.
- Kanhere, S. S. 2011. “Participatory Sensing: Crowdsourcing Data from Mobile Smartphones in Urban Spaces.” 2011 IEEE 12th International Conference on Mobile Data Management (MDM), IEEE. doi:10.1109/MDM.2011.16.
- Karimi, H. A. 2011. “Me-friends-web (MFW): A Model for Navigation Assistance Through Social Navigation Networks.” 2011 7th International Conference on Collaborative Computing: Networking, Applications and Worksharing (CollaborateCom), IEEE. doi:10.4108/icst.collaboratecom.2011.247153.
- Kuflik, T., E. Minkov, S. Nocera, S. Grant-Muller, A. Gal-Tzur, and I. Shoor. 2017. “Automating a Framework to Extract and Analyse Transport Related Social Media Content: The Potential and the Challenges.” Transportation Research Part C: Emerging Technologies 77: 275–291. doi:10.1016/j.trc.2017.02.003.
- Lan, K. C., and W. Y. Shih. 2014. “An Intelligent Driver Location System for Smart Parking.” Expert Systems with Applications 41 (5): 2443–2456. doi:10.1016/j.eswa.2013.09.044.
- Lee, J. H., S. Gao, and K. G. Goulias. 2016. “Can Twitter Data be Used to Validate Travel Demand Models.” 95th Annual Meeting of the Transportation Research Board, Washington, DC, January.
- Lee, D., and S. H. L. Liang. 2011. “Crowd-sourced Carpool Recommendation Based on Simple and Efficient Trajectory Grouping.” Proceedings of the 4th ACM SIGSPATIAL International Workshop on Computational Transportation Science, ACM, pp. 12–17. doi:10.1145/2068984.2068987.
- Li, Y., R. Chen, L. Chen, and J. Xu. 2017. “Towards Social-aware Ridesharing Group Query Services.” IEEE Transactions on Services Computing 10 (4): 646–659. doi:10.1109/TSC.2015.2508440.
- Lin, J., W. Yu, X. Yang, Q. Yang, X. Fu, and W. Zhao. 2017. “A Real-time En-route Route Guidance Decision Scheme for Transportation-based Cyberphysical Systems.” IEEE Transactions on Vehicular Technology 66 (3): 2551–2566. doi:10.1109/TVT.2016.2572123.
- Liu, W., F. Zhang, and H. Yang. 2017. “Modeling and Managing Morning Commute with Both Household and Individual Travels.” Transportation Research Part B: Methodological 103: 227–247. doi:10.1016/j.trb.2016.12.002.
- Lv, Y., Y. Duan, W. Kang, Z. Li, and F. Y. Wang. 2015. “Traffic Flow Prediction with Big Data: A Deep Learning Approach.” IEEE Transactions on Intelligent Transportation Systems 16 (2): 865–873. doi:10.1109/TITS.2014.2345663.
- Ma, S., and O. Wolfson. 2013. “Analysis and Evaluation of the Slugging form of Ridesharing.” Proceedings of the 21st ACM SIGSPATIAL International Conference on Advances in Geographic Information Systems, ACM, 64–73. http://dx.doi.org/10.1145/2525314.2525365.
- Ma, S., Y. Zheng, and O. Wolfson. 2013. “T-share: A Large-scale Dynamic Taxi Ridesharing Service.” 2013 IEEE 29th International Conference on Data Engineering (ICDE), IEEE. doi:10.1109/ICDE.2013.6544843.
- Maghrebi, M., A. Abbasi, T. H. Rashidi, and S. T. Waller. 2015. “Complementing Travel Diary Surveys with Twitter Data: Application of Text Mining Techniques on Activity Location, Type and Time.” 2015 IEEE 18th International Conference on Intelligent Transportation Systems (ITSC), IEEE. doi:10.1109/ITSC.2015.43.
- Miller, M., and C. Gupta. 2012. “Mining Traffic Incidents to Forecast Impact.” Proceedings of the ACM SIGKDD International Workshop on Urban Computing, ACM, pp. 33–40. doi:10.1145/2346496.2346502.
- Minkov, E., R. C. Wang, and W. W. Cohen. 2005. “Extracting Personal Names from Email: Applying Named Entity Recognition to Informal Text.” Proceedings of the Conference on Human Language Technology and Empirical Methods in Natural Language Processing, Association for Computational Linguistics, 443–450. doi:10.3115/1220575.1220631.
- Mutabazi, M. I., and A. Ackbaralib. 2010. “School Travel Characteristics and Attitude Towards Ride Sharing: A Case Study of St. George East School District.” Trinidad. The West Indian Journal of Engineering 32 (1&2): 60–68.
- NAIC (National Association of Insurance Commissioners). 2015. “Transportation Network Company Insurance Principles for Legislators and Regulators.” Command Paper. National Association of Insurance Commissioners, Kansas City.
- NASEM (National Academies of Sciences, Engineering, and Medicine). 2016. “Between Public and Private Mobility: Examining the Rise of Technology-enabled Transportation Services.” The National Academies Press, Washington, DC. doi:10.17226/21875.
- Ni, M., Q. He, and J. Gao. 2017. “Forecasting the Subway Passenger Flow under Event Occurrences with Social Media.” IEEE Transactions on Intelligent Transportation Systems 18 (6): 1623–1632. doi:10.1109/TITS.2016.2611644.
- Oppenheim, N. 1979. “Carpooling: Problems and Potentials.” Traffic Quarterly 33 (2): 253–262.
- Pelzer, D., J. Xiao, D. Zehe, M. H. Lees, A. C. Knoll, and H. Aydt. 2015. “A Partition-based Match Making Algorithm for Dynamic Ridesharing.” IEEE Transactions on Intelligent Transportation Systems 16 (5): 2587–2598. doi:10.1109/TITS.2015.2413453.
- Pender, B., G. Currie, A. Delbosc, and N. Shiwakoti. 2013. “Social Media Utilisation During Unplanned Passenger Rail Disruption—What’s not to ‘Like’?” Proceedings of Australasian Transport Research Forum 2013 Proceedings, Australasian Transport Research Forum.
- Pereira, F. C., A. L. C. Bazzan, and M. Ben-Akiva. 2014. “The Role of Context in Transport Prediction.” IEEE Intelligent Systems 29 (1): 76–80. doi:10.1109/MIS.2014.14.
- Pereira, F. C., F. Rodrigues, and M. Ben-Akiva. 2013. “Text Analysis in Incident Duration Prediction.” Transportation Research Part C: Emerging Technologies 37: 177–192. doi:10.1016/j.trc.2013.10.002.
- Pereira, F. C., F. Rodrigues, E. Polisciuc, and M. Ben-Akiva. 2015. “Why So Many People? Explaining Nonhabitual Transport Overcrowding with Internet Data.” IEEE Transactions on Intelligent Transportation Systems 16 (3): 1370–1379. doi:10.1109/TITS.2014.2368119.
- Rayle, L., D. Dai, N. Chan, R. Cervero, and S. Shaheen. 2016. “Just a Better Taxi? A Survey-based Comparison of Taxis, Transit, and Ride-sourcing Services in San Francisco.” Transport Policy 45: 168–178. doi:10.1016/j.tranpol.2015.10.004.
- Ritchie, J., J. Lewis, C. M. Nicholls, and R. Ormston, eds. 2013. “Qualitative Research Practice: A Guide for Social Science Students and Researchers.” Interface – Comunicação, Saúde, Educação. 14: 705–707. doi:10.1590/S1414-32832010000300019.
- Santani, D., J. Njuguna, T. Bills, A. W. Bryant, R. Bryant, J. Ledgard, and D. Gatica-Perez. 2015. “Communisense: Crowdsourcing Road Hazards in Nairobi.” Proceedings of the 17th International Conference on Human-computer Interaction with Mobile Devices and Services, ACM, 445–456. doi:10.1145/2785830.2785837.
- Santos, A., N. McGuckin, H. Y. Nakamoto, D. Gray, and S. Liss. 2011. Summary of Travel Trends: 2009 National Household Travel Survey. Washington, DC: USDOT, FHWA.
- Schulz, A., P. Ristoski, and H. Paulheim. 2013. “I see a Car Crash: Real-time Detection of Small-scale Incidents in Microblogs.” In The Semantic Web: ESWC 2013 Satellite Events, edited by P. Cimiano, M. Fernández, V. Lopez, S. Schlobach, and J. Völker, 22–33. Berlin: Springer. doi:10.1007/978-3-642-41242-4_3.
- SFMTA (San Francisco Municipal Transportation Agency). 2015. “SFMTA Proposes New Shuttle Rules.” Accessed September 15th, 2017. https://www.sfmta.com/about-sfmta/blog/sfmta-proposes-new-shuttle-rules.
- Shaheen, S., A. Cohen, and I. Zohdy. 2016. Shared Mobility: Current Practices and Guiding Principles. Washington, DC: US Department of Transportation, Federal Highway Administration.
- Shin, E. J. 2017. “Ethnic Neighborhoods, Social Networks, and Inter-household Carpooling: A Comparison Across Ethnic Minority Groups.” Journal of Transport Geography 59: 14–26. doi:10.1016/j.jtrangeo.2017.01.002.
- Smith, L., R. Beckman, D. Anson, K. Nagel, and M. Williams. 1995. “TRANSIMS: Transportation Analysis and Simulation System.” Proceedings of the 5th National Transportation Planning Methods Applications Conference. doi:10.2172/88648.
- Sottini, F., S. Abdel-Naby, and P. Giorgini. 2006. Andiamo: A Multiagent System to Provide a Mobile-based Rideshare Service. Trento, Italy: University of Trento.
- Tang, L. A., Y. Zheng, J. Yuan, J. Han, A. Leung, W. C. Peng, and T. L. Porta. 2013. “A Framework of Traveling Companion Discovery on Trajectory Data Streams.” ACM Transactions on Intelligent Systems and Technology 5 (1). doi:10.1145/2542182.2542185.
- Tiwari, S., and S. Kaushik. 2014. “Information Enrichment for Tourist Spot Recommender System Using Location Aware Crowdsourcing.” 2014 IEEE 15th International Conference on Mobile Data Management (MDM), IEEE. doi:10.1109/MDM.2014.59.
- Wang, X., M. Dessouky, and F. Ordonez. 2016. “A Pickup and Delivery Problem for Ridesharing Considering Congestion.” Transportation Letters 8 (5): 259–269. doi:10.1179/1942787515Y.0000000023.
- Waraich, R. A., D. Charypar, M. Balmer, and K. W. Axhausen. 2009. “Performance Improvements for Large Scale Traffic Simulation in MATSim.” 9th STRC Swiss Transport Research Conference: Proceedings, Swiss Transport Research Conference. doi:10.3929/ethz-a-005864320.
- Zhao, W., Y. Qin, D. Yang, L. Zhang, and W. Zhu. 2014. “Social Group Architecture Based Distributed Ride-sharing Service in VANET.” International Journal of Distributed Sensor Networks 10 (3). doi:10.1155/2014/650923.
- Zheng, K., Y. Zheng, X. Xie, and X. Zhou. 2012. “Reducing Uncertainty of Low-sampling-rate Trajectories.” 2012 IEEE 28th International Conference on Data Engineering (ICDE), IEEE. doi:10.1109/ICDE.2012.42.
- Zhu, M., X. Y. Liu, F. Tang, M. Qiu, R. Shen, W. Shu, and M. Y. Wu. 2016. “Public Vehicles for Future Urban Transportation.” IEEE Transactions on Intelligent Transportation Systems 17 (12): 3344–3353. doi:10.1109/TITS.2016.2543263.