ABSTRACT
Understanding the value of travel time for mobility concepts based on self-driving vehicles is crucial to accurately value transport investments and predict future travel patterns. In this paper, we carry out a morphological analysis to illustrate the diversity of mobility concepts based on self-driving vehicles and the complexity of determining the value of travel time for such concepts. We consider four categories of parameters that directly or indirectly impact the value of travel time: (i) vehicle characteristics, (ii) operating principles, (iii) journey characteristics and (iv) traveler characteristics. The parameters and respective attributes result in a morphological matrix that spans all possible solutions. Out of these, we analyze five plausible solutions based on the implications of the concept characteristics on the total value of travel time. We conclude by suggesting an alternative approach to differentiate value of travel time based on travel characteristics rather than the usual decomposition into transport modes.
1. Introduction
The value of travel time savings is often the key benefit considered in a cost–benefit analysis of transportation projects and policies (Mackie, Jara-Diaz, and Fowkes Citation2001; Hensher Citation2011). The value of travel time (henceforth VTT) is also used in transportation models as part of the generalized cost of travel, which is important when modelling travel behavior. Thus, having valid estimates of VTT is crucial for forecast, planning and appraisal purposes. Traditionally, one of the main parameters that determine VTT is the mode of travel, which for example is segmented as travel by car, bus or train as well as cycling and walking. The purpose of this paper is to show that a shift to self-driving vehicles (SDVs) will blur the lines between currently established modes, such as private car travel and travel by public transport and to suggest that this might be handled by segmenting VTT in a new way. The introduction of self-driving vehicles will not only mean changing manually driven cars to self-driving cars and manually driven buses to self-driving busses but also enable existing and new transport providers to deploy SDVs under new business and service models (Townsend Citation2014). Taking this into account, we carry out a morphological analysis in order to identify and analyze possible SDV mobility concepts based on aspects that impact VTT. There is a range of characteristics that impact VTT for the identified SDV mobility concepts, which in turn support that there is a relative variation in VTT and that this variation can be represented by the characteristics as such rather than specific modes.
A morphological analysis can be seen as a structuring tool that allows for systematic identification and structuring of all possible aspects of a solution to a complex problem (Álvarez and Ritchey Citation2015). The starting point of the analysis is formulating the problem and identifying the parameters and possible states per parameter that represent the components and possible outcomes of the situation. This yields a full problem space, the so-called morphological box that contains all possible solutions. In our case, each of these solutions represents one SDV mobility concept. Usually, the analysis results in a final solution space, which includes all solutions that are logically and empirically consistent. However, the aim of this paper is not to apply morphological analysis to identify all the final or the ‘best solutions’, i.e. the most probable or desirable scenarios. Neither is it to lay out the specifications for all future self-driving vehicle concepts in detail. Rather, we strive to showcase the diversity of possible self-driving vehicle applications and the complexity of modelling a VTT for these applications.
To our knowledge, the types of SDVs (and thus, the various possible concepts) discussed so far in the literature on VTT for SDVs have been limited (Kolarova et al. Citation2018; Steck et al. Citation2018). Thus, we believe that discussing the proper segmentation of automated vehicles into modes has largely been neglected. So far, the literature mostly considers new SDV-based modes, some of which are assumed to operate in parallel with ‘traditional’ modes. Arguably, this will complicate the picture of accurately modelling the complete, multi-modal transportation system. Hence, based on our analysis, we suggest that future transport research should consider moving away from specifying VTT for each existing and potential travel mode. Instead, we argue that VTT models should employ a range of travel and service characteristics (self-driving/manually-driven shared/non-shared, private/non-private, etc.) to reflect the heterogeneity of VTT in a context of rapid technological advancements. While this does not guarantee that estimating values of time for SDVs will be methodologically simpler, it is an approach that should at least be considered as an alternative to using the current mode-based segmentation of VTT based on a transport paradigm that might soon be transformed.
The paper is structured as follows. In section 2, we describe morphological analysis as a tool for scenario modelling. In section 3, we briefly present the notion of self-driving vehicles and the concept of travel time as it is understood in transport economics. In section 4, we present and discuss the specific parameters we have considered as being relevant for the VTT in our analysis. In section 5, we present five possible transport concepts based on scenarios we have identified in our analysis. Section 6 is a discussion based on these concepts and section 7 concludes.
2. Morphological analysis and scenario modelling
Though there are a number of scenario modelling techniques in the literature (Börjeson et al. Citation2006; Amer, Daim, and Jetter Citation2013), we have chosen to carry out a morphological analysis as an explorative tool. Developed for scenario modelling in defense planning and used by researchers in the field of futures studies and technological forecasting, it can be used to visualize elements and dimensions to develop raw scenarios for the future (Amer, Daim, and Jetter Citation2013). In this context, a scenario is defined as a description of a possible state. It is not intended as a prediction of future events, rather the intention is to present a set of alternatives against which different courses of action can be considered. Morphological analysis allows us to showcase the diversity of possible future SDV-mobility concepts by presenting the solution space in which each solution represent one SDV mobility concept.
The purpose of a morphological analysis is to identify and structure all possible aspects and solutions in situations where the problem space is complex and non-reducible (Johansen Citation2018).
There are multiple advantages to the morphological approach, such as it being a structured and transparent process, the identification of the entire solution space rather than focusing on a few scenarios and the possibility to define solutions beyond those that are most likely and mainstream (Johansen Citation2018). However, the full analysis is very resource consuming and can be claimed to rest on judgmental evaluations, though this is exactly what can be avoided through a well-structured process (ibid). The steps of the analysis are described in .
As noted in step 4, the morphological box contains the entire solution space and some of it consists of so-called ‘noise’, i.e. solutions that are inconsistent or impossible. Therefore, it is crucial to carry out an analysis of the solution space to reduce the amount of noise, i.e. step 5. The end-result of the analysis is a solution space where all solutions are both logically and empirically consistent. The former refers to the internal relationship between concepts not being mutually contradicting while the latter refers to the sense that solutions do not rely on empirically impossible or highly improbable assumptions.
The intention of applying morphological analysis in this paper is neither to identify the ‘best solutions’ nor to identify a solution space fully free from noise. Instead, we have selected and further analyzed five plausible solutions. We have stopped the morphological analysis short of the last step for two reasons. First, it is unclear which conditions or methods one should use to identify the so-called final solutions. Due to the size of the morphological box with this particular problem formulation, judgmental evaluations would not be avoidable. Second, and most important for our purposes, we aim to showcase the diversity and complexity of SDV mobility concepts. To do this, it is sufficient to showcase the full solution space and to discuss a selection of plausible and probable solutions without claiming that they are the final or most likely ones.
3. Background
3.1. Self-driving vehicles
In this paper, a self-driving vehicle (SDV) is defined as a road vehicle for which the full driving task is automated in some or all operational domains (equivalent to SAE level 4 and 5Footnote1). For the purpose of analysis in the remainder of this paper, an umbrella term for different SDV-related sub-modes or services is needed. The term SDV mobility concept is used to denote an idea underlying and governing the design of a mobility solution in which some type of SDV is used. Hence, the term concept encompasses characteristics including, but not limited to, SDV vehicle type and characteristic, service model, vehicle ownership, operational principles, etc. An SDV mobility concept should be understood as a specific and distinct combination of such characteristics, i.e. one solution from the morphological box.
The development and impacts of SDVs are subject to significant uncertainty and to what extent and how SDVs will be used is not clear. The role SDVs will play in the future is determined by a range of sociotechnical factors that are influenced by broader concurrent societal developments (Engholm, Kristoffersson, and Brenden Citation2018; Skeete Citation2018). Future transport systems with SDVs will likely consist of multiple complementing and competing SDV mobility concepts. Ongoing pilot projects and commercial initiatives include applications of SDVs for taxi services, ride sharing, public transport shuttles and private cars (Hawkins Citation2019; iMove Citation2019; Kane Citation2019; Wiggers Citation2019). There are examples in the literature of many different future scenarios for transport systems ranging from luxurious self-driving private cars to shabby DIY transit network with self-driving minivans. There are also examples of both traditional transport service models and yet unseen service and business models for SDVs (Townsend Citation2014). All in all, the spectrum of future potential mobility concepts is broader than the classical distinction between private cars and public transport. There are numerous emerging forms of car sharing, ride sharing and combined mobility concepts with SDVs (Laine et al. Citation2018) in between those categories.
3.2. Value of travel time (VTT)
The notion of travel time value stems from time allocation models with origins in consumer theory (Hensher Citation2011). In mainstream economics, it is generally assumed that individuals seek to maximize their happiness or satisfaction with life. This is assumed to depend on the consumption of various goods and services as well as on the activities individuals carry out during the available time they have. Travel is usually viewed as a derived demand; we travel only to be able to reach the locations needed to carry out activities that yield value for us. Consequently, the value of free time (non-working time) has been obtained from analysis of choices individuals made when having to choose between a time-consuming but cheap alternative and a more expensive but less time-consuming alternative, such as taking the slow but cheap train versus taking the expensive express train. The generally accepted method consists in identifying the marginal rate of substitution between the travel time and travel cost using discrete choice models based on random utility theory. Individuals’ willingness to pay to reduce travel time is interpreted as the subjective VTT. For travel time in particular, the value of saving travel time is composed of two effects – the benefit of being able to allocate time to other activities and the benefit of reduction of time spent on an unpleasant activity (traveling). Notably, for car travel, it is typically the willingness to pay to reduce travel time of the car driver that is seen as the relevant measure. Insofar as car passengers have been considered, it is mostly from the perspective of how they impact the valuation of travel time of the car driver (Hensher Citation2008).
Generally, there are multiple factors that may have an impact on the VTT (Shires and De Jong Citation2009; Small Citation2012). For example, it is common to distinguish between types of trips based on their purpose, such as if the travel is done for business purposes or other purposes (including commuting). The travel mode, such as if the travel is by car, bus and train and so on, also affects the VTT. This reasoning results in varying values of travel time, depending on the characteristics of the travel. The characteristics of the journey are also considered, such as if it is crowded, how reliable the travel mode is and comfort during the trip (Wardman Citation2014). There are a multitude of travel parameters that impact the VTT and it has been claimed that the average effect of the unobserved attributes differs significantly across the various public transport modes (Varela, Börjesson, and Daly Citation2018).
In the context of SDVs, there intuitively seems to be an inclination to assume lower VTT: ‘more travel comfort, enhanced travel safety, higher travel time reliability, and the possibility to perform activities other than driving (like working, meeting, eating, or sleeping) while on the move will likely lead to lower values of time’ (Milakis, van Arem, and van Wee Citation2017, p. 329). Simply put, having SDVs at their disposal is assumed to enable people to travel more, both longer distances and for longer periods of time. For example, the value of in-vehicle travel time in a shared electric SDV is modeled at 35% of the in-vehicle travel time in a non-self-driving privately owned vehicle which is said to be equivalent of travel time as in (rail-based) public transport (Chen and Kockelman Citation2016). To a certain extent, the lower VTT for SDVs has also been confirmed by current empirical evidence (Cyganski, Fraedrich, and Lenz Citation2015). However, the current literature has to a large extent continued with the practice of segmenting on the basis of mode of travel, adding additional ‘SDV-modes’. The main distinction that is made is between privately owned SDVs and shared SDVs (Steck et al. Citation2018; Kolarova, Steck, and Bahamonde-Birke Citation2019) as well as SDVs with a so-called office interior and AVs with a leisure interior (Correia et al. Citation2019). Due to lack of empirical data from real-world SDV implementations, most of the current research is based on stated preference surveys, which are vulnerable to hypothetical bias. To address this, some authors compare stated preference for SDVs with actual usage of chauffeur driven cars (Wadud and Huda Citation2019). While some work relates to shared SDVs (Chen and Kockelman Citation2016), the actual characteristics of the travel mode do not seem to be taken into consideration. For example, though the notion of crowding does not apply in shared SDVs, one of the suggested drivers of the dissatisfaction does, namely the physical closeness of other travelers per se (Haywood, Koning, and Monchambert Citation2017).
Furthermore, the notion of lower values of travel time hinges on an assumption of travel time being ‘freed’, which has been disputed both on theoretical and empirical grounds (Singleton Citation2019). As a study by de Almeida Correia et al. (Citation2019) shows, the VTT for a SDV with a specific ‘leisure interior’ was not lower compared to a conventional car. At the very least, the remarkable advancement in transportation technologies requires that the assumed benefits are closely studied and not just taken for granted (Mokhtarian Citation2018).
4. Parameters determining the value of travel time
As briefly mentioned in the previous section, the literature supports that multiple aspects of a journey are assumed to affect the VTT. These are aspects such as mode of travel, the time at which the journey is made, the characteristics of the journey as well as the purpose and length of the journey. For the purpose of our analysis, we have considered four categories of parameters that we find relevant to the VTT for SDV mobility concepts: (i) vehicle characteristics, (ii) operating principles, (iii) journey characteristics and (iv) traveler characteristics. The intent is to cover aspects mentioned in the paragraph above as well as to consider parameters that provide a comprehensive picture of potential SDV mobility concepts. Thus, we cover both parameters that directly impact the VTT and parameters that outline the design of SDV mobility concepts that indirectly impact the VTT. Additionally, there are multiple context-dependent circumstances that might influence in-vehicle travel time perception and time use (Kolarova, Cyganski, and Lenz Citation2019). In our analysis, we have not made any assumptions on how travelers will actually spend their time while traveling using different SDV mobility concepts even though the desire to spend time in a particular way while traveling can influence one’s travel choices. In addition, we have not distinguished our analysis for different trip purposes nor do we distinguish for different social groups (including different income levels). Similarly, while the VTT is also assumed to depend on trip purpose, multiple SDV mobility concepts could be utilized for travel with multiple purposes. Hence, we do not consider travel purpose in this paper.
Given the categories of parameters established above, we will below briefly discuss each category and highlight parameters that deserve most recognition. The first two categories of parameters we consider are vehicle characteristics and operating principles. To a large extent, the vehicle parameters define the comfort of the vehicle since this category includes driving style, interior design focus as well as vehicle form and capacity. The operating principles in turn include parameters such as vehicle ownership model and service model, as well as if the travel is shared or private. We consider the possibility to use a remote human operator to monitor vehicle operations as such an option may influence the perceived level of safety (Zhao, Darwish, and Pernestål Citation2019). Traditionally, in VTT estimations, matters related to comfort are seen as up to the individual car traveler to decide (since the levels of comfort are ultimately up to each car-owner) whereas they are to some extent considered for public transport. The most prominent qualitative aspect of public transport is crowding, with a higher VTT for journeys under crowded conditions (Li and Hensher Citation2011; Batarce, Muñoz, and de Dios Ortúzar Citation2016) since a lack of seating is expected. Other aspects include seating layout and position (Wardman and Murphy Citation2015) as well as comfort, interior design, temperature control and legroom. In our analysis, we have considered interior design and interior design focus, as well as driver seat design, as part of the vehicle characteristics parameters. A potentially important factor for SDV mobility concepts when vehicles and/or rides are shared is the risk of trip rejection, meaning that a traveler’s trip request will be declined due to insufficient vehicle availability. The service provider can to some extent decide an acceptable rejection rate by adjusting the fleet size for a given demand although the temporal and spatial stochasticity in demand likely make it unfeasible to fully eliminate the risk of rejection (Bösch, Ciari, and Axhausen Citation2018).
The third category of parameters in our analysis is journey characteristics, which we have defined, based on a door-to-door perspective of travel. This is important since the value of in-vehicle time is considered separate from waiting time, access and egress time (which is significantly higher). In the context of SDVs, the usual assumptions regarding waiting and access time have to be reconsidered. Compared to traditional car travel, where there might be short walk time to access the car (if it is parked inconveniently) but no wait time at the start of the journey, a personal self-driving car might park itself far from the travel destination. Thus, there will be no access time but rather waiting time at the start of the journey since the car has to drive itself back. Ideally, you will be able to ‘call’ the car in advance but this will require some planning. Depending on the parking arrangements, access to the car (even when it is privately owned) might not be instant. This is also related to notions of reliability and variability of travel time, since the car can get stuck in traffic driving itself back from parking.
Another aspect that significantly impacts the experience of a journey and thus VTT is reliability. There is sometimes a difference between how reliability is considered depending on mode, such as for private car transport compared to public transport. For the former, the notion of variability, defined as the variation of travel time for a particular distance, is used rather than the term reliability, which is used in the context of the latter. This is because public transportation modes generally adhere to a schedule; there is a frequency of service to take into consideration. A public transport mode following a schedule can be late and the VTT for delays is high compared to regular in-vehicle time. For regular car travel, the travel time can vary but is not necessarily considered to be a delay in transport appraisal (Trafikverket Citation2018, chap. 8), even if arriving late can be perceived as a delay by the traveler. The reasoning is that since there is no schedule and thus no reference point, measuring a delay is difficult for practical reason and would be meaningless. However, for SDV mobility concepts, maintaining that the concept of reliability is only applicable to public transport (assuming we would be able to divide the concepts into public and non-public modes) seems limiting. This brings us to the parameter we have chosen to call scheduling type. We claim that the central aspect concerning how reliability should be considered is related to the way the travel is perceived from a scheduling perspective. Namely, if the travel is planned according to an estimated time of arrival (based on the ETA-principle) or based on departure time. Given the premise of travel being with the purpose of arriving at a desired destination, one can assume that ‘Uber-like’ SDV mobility concepts will offer travel booking based on a specific arrival time. If this is the case, we argue that delay should be taken into account for concepts that resemble the traditional car mode similarly to how it is taken into account for public transportation modes. While travel time uncertainty has been considered for ride-sharing with the conclusion that it is important to consider (Long et al. Citation2018), it has thus far not been discussed in detail for other concepts based on SDVs.
The last category of parameters we have considered is traveler characteristics, including travelers’ risk attitudes. We have broadly assumed that some travelers will be risk averse and others will be risk accepting regarding travel time, for example travelers accepting a higher risk of rejections being ‘risk accepting’. Additionally, we consider perceived safety as part of the attitude towards the travel concept. This is closely related to the level of trust passengers will have towards the new technology (Bjørner Citation2019). We assume that the VTT will depend on the perception of safety with a ‘safe’ journey being valued lower compared to a concept that is perceived to be less safe and thus has a higher VTT.
5. The SDV mobility concepts and VTT implications
While the full solution space of the morphological analysis is presented in , we have chosen to highlight a selection of five plausible and probable future SDV mobility concepts that have been discussed in the SDV literature. This selection of SDV mobility concept is not intended to be exhaustive nor to represent the SDV mobility concepts most likely to emerge. Instead, the selection is intended to show the broad range of potential concepts with different implications on the VTT. Each of these SDV mobility concepts represent one feasible solution of the morphological matrix (). In , the full set of attributes for the five SDV mobility concepts is presented.
Figure 2. The morphological box which illustrates the solution space given the selected set of parameters and attributes.
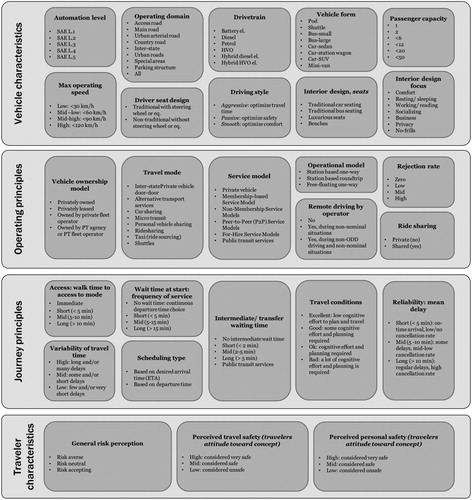
Below, we give a broad description and discuss the main characteristics for each concept. Further, we consider the implications for VTT in order to illustrate how different combinations of attributes may influence the VTT per concept.
5.1. Feeder shuttle
The purpose of the feeder shuttle concept is to improve the quality of public transport in urban areas by solving the first/last-mile issue by connecting high-capacity public transport hubs with workplaces, services or residential areas (Pernestål and Kottenhoff Citation2018). There are several pilots and commercial implementations of self-driving shuttles used to complement existing high-capacity public transport (S3 Project Citation2018; iMove Citation2019; ITRL Citation2019). The feeder shuttle is operated by or on behalf of a public transport authority and is integrated in the regular public transport service. It operates on a fixed route with fixed stops, similarly to many public transport bus routes in urban areas. However, the feeder shuttle route tends to be short with a typical trip on the shuttle taking less than 15 min. The service is monitored by a control center with remote human operators that can operate the shuttle in non-nominal driving conditions.
The feeder shuttle typically has a capacity of around 15 passengers and is powered by a battery-electric drivetrain. The interior design is similar to a small tram with a few passenger seats and space for standing passengers. To minimize risks for standing passengers, the shuttle operates at low speeds. Comfort is not prioritized since trips are short and high utilization of the interior space and cost-efficient cleaning and maintenance is crucial for the operator. The frequency of service is high due to the low operational costs. Usually, the feeder shuttle route has many stops and the average walking time to access the service is short.
5.1.1. Implications for VTT
The feeder shuttle can be assumed to have a short access time and a mid to long wait time at start (frequency of service). Considering the trip is usually short, the mean late time will also be short and thus the reliability will be high and the variability will be low since few or very short delays are expected. From the perspective of reliability and variability, the VTT should be lower than for a regular public transport bus. However, the qualitative conditions of a feeder shuttle will generally be lower than on a regular bus making it impossible to spend the travel time productively by e.g. performing work. The trip is short there is little need for comfort. Thus, from the perspective of comfort, the VTT should be higher than for a regular bus. Moreover, considering the feeder shuttle moves at low speeds, there is a possibility of it being perceived as stressful due to being slow (related to the perception of travel in congested conditions being more stressful). Based on the analysis, we do not believe it is warranted to assume the VTT of a feeder shuttle to be lower than for a regular bus nor it warranted to assume it to be higher without further studies.
5.2. Public transport shuttle on-demand in rural areas
The public transport shuttle on-demand is a mobility concept for enhancing the availability and quality of public transport services in rural and non-urban areas with relatively low population density. It has been suggested that using self-driving shuttles for public transport services in rural areas will be significantly more cost efficient than current solutions and also have lower implementation thresholds than in urban settings (Bernhart et al. Citation2018). The on-demand shuttle is operated by or on behalf of a public transport authority and the traveler needs to book a trip in advance with the service through an app or any other digital interface. The shuttle can be used either for making door-to-door trips or to access other public transport services (i.e. acting as a feeder). Trips with the shuttle tend to be long since they are made in sparsely populated areas. The shuttle route is not fixed but dynamically optimized by the operator given current demand in the area. Thus, some trips might be private for the traveler and other trips are shared with other passengers for parts of the journey.
The vehicle used for the shuttle on-demand service is very similar to the feeder shuttle. However, the shuttle on-demand operates at slightly higher speeds since its passengers are seated and it needs to cover longer distances. Also, it is equipped with slightly more comfortable seating.
5.2.1. Implications for VTT
The public transport on-demand shuttle is envisioned to be operating in rural areas. Thus, even if it is ‘on demand’, the wait time from wanting to take the shuttle to it arriving is assumed to be long. In practice, the ‘demand’ or booking in advance will probably have to be made with fairly long notice and it is unreasonable to include the time from when the vehicle is booked to when it arrives, even from a ‘door-to-door’ perspective. On the one hand, the time from ‘booking’ to ‘start of trip’ should not impact the value of in-vehicle time. On the other hand, the concept is clearly less flexible than a privately owned option which should be reflected in the total assessment. Given that the concept operates in rural areas, we assume that it is reliable with low variability of travel time. However, if the service is to pick other passengers along the trip, there could be unexpected intermediate wait time. Here, we assume that such a service would wait for passengers that have booked the service but were not at the place of pick-up at the time the shuttle arrives. While the wait time could be regulated, it could still be a source of delays.
While the concept is technically ‘on demand’, this is a case of travel being made with an estimated time of arrival in mind and thus, the mean delay and the variability of the delay should be considered, even if the actual vehicles are ‘car-like’ in their operational principles. The vehicle is assumed to be shared but given the rural context, it is probably rarely crowded. While the characteristics of shared travel will impact the sense of privacy and potentially comfort, the extent of the impact should be evaluated further.
5.3. Private premium self-driving car
Private ownership of self-driving cars is similar to privately owned conventionally driven cars in many ways. However, there are a few aspects of self-driving cars that might differ from the practices of conventionally driven cars, especially from a door-to-door perspective. The private premium self-driving car is capable of parking itself after dropping of the traveler and driving itself back from parking. Thus, instead of walking to the parking lot as in the case with a conventionally driven car, the traveler will wait at the trip origin. Another potential feature of private premium self-driving cars is that they might use supportive digital tools that automatically do the travel planning. The traveler could use an app to state the trip destination and preferred time of arrival and the self-driving car (or a connected IT-system) calculating time of departure based on prognosticated travel conditions and making sure to have the self-driving car ready in time.
5.3.1. Implications for VTT
Compared to a non-automated vehicle, it has been assumed that the in-vehicle time in a private premium self-driving car can be utilized better by the traveler and thus the VTT is lower compared to the current mode ‘car’. However, as noted above, the concept is different from a non-automated car in several ways. Given that the vehicle drives itself to its traveler, there is no access time and the wait time is assumed to be short. However, the traveler will also have the option of walking to the car. Depending on the circumstances (such as the weather), a short walk can be preferred to wait time, especially since the drive back from parking can be a source of unreliability. All in all, the traveler will have flexibility and will be able to plan travel with little cognitive effort.
Even though the concept is close to a traditional car, there is reason to consider reliability as well as variability. As suggested above, the traveler could choose to plan the travel based on an estimated time of arrival. In a setting where the vehicle then suggests the optimal departure time, a potential delay at the end destination should constitute a delay in the same sense as for ‘schedule-based’ modes. Nevertheless, the high flexibility of the concept would warrant a high reliability.
5.4. Shared self-driving taxi
An alternative type of self-driving taxi service is a shared ride service. The idea is that by sharing rides with other travelers with similar routes and times for their trips, costs can be shared between several travelers, thus making the service cheaper than a private self-driving taxi. Travelers order rides in the same way as for the private self-driving taxi and the operator’s IT-system automatically analyzes and pairs the trips with other travelers for which the ride will be shared. Shared rides typically have longer travel times since small detours may be required to pick up fellow travelers and stopping to pick up and/or drop off other travelers increases the risk of delays.
5.4.1. Implications for VTT
As noted above, while the access time and wait time are similar to private driverless taxis, other passengers will inevitably impact the reliability and variability of travel. Even if the intermediate wait time is short, every new passenger that is picked up can be a potential delay. Since the service will do detours, the variability can be affected by high unpredictability.
The in-vehicle time will be less private compared to non-shared rides. Here, the sources of discomfort in crowded environments can be applicable even though the car isn’t ‘crowded’ per se. Moreover, the sense of safety will be important to the in-vehicle experience. Even with surveillance which can be assumed will be implemented and itself being a potential privacy concern, you are sharing a small space with strangers. Compared to traditional taxis where the drivers commonly have a license and can be identified, the amount of information and traceability of the fellow travelers is unknown. Hence, the level of perceived personal safety can be assumed to be lower than for private travel and travel with a driver. Thus, the VTT can be higher than for such alternatives (all else equal). In summary, while the VTT can be lower compared to traditional car traveled, the lack of privacy and safety concerns as well as higher levels of variability make it difficult to assume that the VTT can be compared to other, non-shared modes without further investigations.
5.5. No-frills self-driving service
It has been suggested that private self-driving taxi service providers (at least initially) might focus on wealthy urban areas to maximize profits and not offer the service in other parts of the city where the payment ability of the inhabitants is lower. This might create a market opportunity for emerging low-cost self-driving services focusing on lower income groups or areas (Townsend Citation2014; Litman Citation2018).
This SDV mobility concept can be compared to low-cost airlines with a no-frills offering. The no-frills self-driving service is offered by a fleet operator that is commercial or community-owned and operated. Travelers order trips through an app. The SDVs used for the service could be old mini vans that have been retrofitted with driving automation technology or second-hand self-driving shuttles that previously were used for other services. The SDVs are equipped with an interior of hard plastics, which is durable and simple and cheap to clean but offers no comfort. The vehicles are often packed with passengers and often suffer from standstills due to technical faults due to old components and poor maintenance, which causes delays.
5.5.1. Implications for VTT
A no-frills service operates at low levels of convenience. As such, passengers can be required to walk to a point of pick-up (access time) and wait until the vehicle arrives. While it will not wait for other passengers for long (making the intermediate wait time short), there are still plenty of sources of delays and hence variability of travel time. One such source is the possibility of rejections, meaning that the service is not available when needed. A high rejection rate heavily impacts reliability of the service in general as well as the VTT directly since the availability of the service cannot be trusted. Moreover, the concept is assumed to be ‘departure’ based and does not allow for travel to be planed according to estimated arrival time. Additionally, the in-vehicle level of comfort is presumed to be low. All in all, even though the service is self-driving, the VTT is comparable to low-cost bus travel, at best.
6. Discussion
Given the above exposition of SDV mobility concepts (), we observe that some of the concepts are similar from the perspective of VTT characteristics to traditional modes while others are novel and do not have a traditionally operated equivalent. On the one hand, a privately-owned self-driving vehicle is probably similar in many ways (except for the self-driving aspect) to a privately owned traditionally operated car and private self-driving taxis are similar to traditionally operated taxis. On the other hand, the ‘traditional’ equivalent mode of a feeder shuttle or a shared is not obvious. While a rural public transport shuttle and a no-frills shuttle could be viewed as instances of ‘public transport’, these concepts do not share many characteristics with a traditional bus or train.
Furthermore, there are recurring aspects related to the implication for VTT for the respective concepts that require further consideration. First, considering only in-vehicle time for SDVs is insufficient since the operating principles and ultimately the journey characteristics will impact the entire door-to-door travel experience. This is particularly true for the privately-owned SDVs where the self-parking capabilities can impact both waiting time and lead to a higher level of uncertainty since the vehicle is dependent on the traffic situation while driving itself to the pickup location. These aspects are new compared to traditionally operated privately owned cars and would seemingly increase the VTT, something that has to be put in perspective to the commonly accepted assumption of lower travel time for privately-owned SVDs. Second, the current practice of only considering public transport (i.e. schedule-based) modes as potentially being delayed and thus considering variability rather than reliability can be questioned in the context of SDV mobility concepts. We claim it is reasonable to assume that concepts similar to traditional cars with regards to vehicle parameters can be operated on a schedule basis. Moreover, technology enables travelers to plan their journeys on a basis of estimated time of arrival (ETA). If ETAs for car travel can be known, actual delays as they are experienced by the travelers can be observed. In light of these considerations, segmenting concepts into modes to either consider reliability or variability is limiting. Lastly, understanding the difference in the perception of the VTT between private and shared travel is crucial. Aspects of privacy, risk and comfort need to be further studied. A vehicle that is car-like in its design but shared can be experienced as ‘crowded’ with higher levels of discomfort.
This analysis can provide insight into how to approach the characteristics of SDV mobility concepts to model the values of travel time. As noted in Section 4, the common method in the literature has been to add new modes to existing models, such as adding ‘privately owned SDVs’ as a new mode. Mapping the concepts discussed here into modes is a possible way forward. However, the complexities showcased and the aspects discussed above indicate that this mapping can be complicated. This makes us question the meaningfulness and value of segmenting travel time value based on modes. The morphological analysis in this paper covers a wide range of variables that may affect the VTT for SDV mobility concepts. However, it is practically infeasible to model and assess the VTT for all available concepts in the solution space. In addition, it can be expected that the individual, direct effect of several of these variables on the VTT is low, and potentially practically impossible to empirically identify. An alternative method would be to define the main parameters that influence the VTT as the underlying basis of segmentation. This could be extended by taking into account context dependent factors such as they have been outlined by Kolarova, Cyganski, and Lenz (Citation2019). With this approach, VTT could depend on whether the journey is shared or private, if it is based on departure time or estimated time of arrival and if it is on-demand or schedule based. Using this segmentation, a traditionally operated car would be private, on-demand and departure time based while a traditional bus would be shared, schedule-based, and based on estimated time of arrival. While more research needs to be done to see if these particular parameters sufficiently capture the differences in VTT, they do seem to capture the difference between traditional modes and are more flexible with regards to SDV mobility concepts.
7. Conclusions
The results of the morphological analysis carried out in this paper provide further insight into the complexity of VTT for self-driving vehicles. In particular, the findings help to illuminate the multivariate nature of variables interactively influencing the VTT for different SDV concepts.
The emerging landscape of driving automation technology indicates that SDVs will be used for different types of mobility concepts. Many of these do not fit into the traditional classification of transport modes used for VTT estimation and transport modelling. While our analysis shows that some SDV concepts have VTT characteristics similar to existing modes (although we cannot know for certain that the VTT estimate is similar), other SDV concepts bear few similarities with any of the existing modes and may require a new way of understanding and empirically estimating VTT. Hence, we believe that we have provided sufficient reason to reconsider viewing self-driving vehicles as new additional modes. Given the complexities, future research on the VTT for travel concepts that rely on SDV technology has to be more developed. Without further studies, we cannot be certain of the magnitude of variations between the values of time for the concepts we have analyzed. However, we show that the possible solutions differ significantly in characteristics that are relevant for the VTT.
The research presented in this paper can serve to guide future theoretical and empirical research on the VTT of SDV mobility concepts. The parameter set used for the morphological analysis highlight aspects that require consideration and the presented SDV mobility concepts can be used as a starting point for VTT studies going beyond the traditional transport mode segmentation. Finally, we believe that the proposed approach to focus on a set of key parameters instead of transport mode classification may be a more prosperous approach to valuing travel time going forward.
Acknowledgment
This work was partly supported by the Swedish Transport Administration (Trafikverket) under grant TRV 2017/22806.
Disclosure statement
No potential conflict of interest was reported by the author(s).
Additional information
Funding
Notes
1 See SAE International (Citation2018)
References
- Almeida Correia, Gonçalo Homem de, Erwin Looff, Sander van Cranenburgh, Maaike Snelder, and Bart van Arem. 2019. “On the Impact of Vehicle Automation on the Value of Travel Time While Performing Work and Leisure Activities in a Car: Theoretical Insights and Results from a Stated Preference Survey.” Transportation Research Part A: Policy and Practice 119: 359–382.
- Álvarez, Asunción, and Tom Ritchey. 2015. “Applications of General Morphological Analysis.” Acta Morph Gen 4 (1): 1–40.
- Amer, Muhammad, Tugrul U. Daim, and Antonie Jetter. 2013. “A Review of Scenario Planning.” Futures 46: 23–40.
- Batarce, Marco, Juan Carlos Muñoz, and Juan de Dios Ortúzar. 2016. “Valuing Crowding in Public Transport: Implications for Cost-Benefit Analysis.” Transportation Research Part A: Policy and Practice 91 (September): 358–378. doi:10.1016/j.tra.2016.06.025.
- Bernhart, Wolfgang, Hitoshi Kaise, Yuzuru Ohashi, Tobias Schönberg, and Laurianne Schielles. 2018. Reconnecting the Rural - Autonomous Driving as a Solution for Non-Urban Mobility. Frankurt am Main: Roland Berger.
- Bjørner, Thomas. 2019. “Driving Pleasure and Perceptions of the Transition from No Automation to Full Self-Driving Automation.” Applied Mobilities 4 (3): 1–16.
- Boesch, Patrick M., Francesco Ciari, and Kay W. Axhausen. 2016. “Autonomous Vehicle Fleet Sizes Required to Serve Different Levels of Demand.” Transportation Research Record: Journal of the Transportation Research Board 2542 (January): 111–119. doi:10.3141/2542-13.
- Börjeson, Lena, Mattias Höjer, Karl-Henrik Dreborg, Tomas Ekvall, and Göran Finnveden. 2006. “Scenario Types and Techniques: Towards a User’s Guide.” Futures 38 (7): 723–739. doi:10.1016/j.futures.2005.12.002.
- Bösch, Patrick M, Felix Becker, Henrik Becker, and Kay W. Axhausen. 2018. “Cost-Based Analysis of Autonomous Mobility Services.” Transport Policy 64: 76–91. http://dx.doi.org/10.1016/j.tranpol.2017.09.005.
- Chen, T Donna, and Kara M Kockelman. 2016. “Management of a Shared Autonomous Electric Vehicle Fleet: Implications of Pricing Schemes.” Transportation Research Record: Journal of the Transportation Research Board 2572 (1): 37–46.
- Correia, Goncalo, Erwin Looff, Sander van Cranenburgh, Maike Snelder, and Bart van Arem. 2019. “On the Impact of Vehicle Automation on the Value of Travel Time While Performing Work and Leisure Activities in a Car: Theoretical Insights and Results from a Stated Preference Survey.” 119: 359–382. doi:10.1016/j.tra.2018.11.016.
- Cyganski, Rita, Eva Fraedrich, and Barbara Lenz. 2015. “Travel-Time Valuation for Automated Driving: A Use-Case-Driven Study.” Proceedings of the 94th annual meeting of the Transportation Research board, Washington DC.
- Engholm, Albin, Ida Kristoffersson, and Anna Pernestål Brenden. 2018. “Is the Driverless Future Sustainable? - Strategic Uncertainties and System Impacts.” World conference on Transport Research WCTR 2019, Mumbai.
- Hawkins, Andrew J. 2019. “Waymo’s Self-Driving Cars Are Now Available on Lyft’s App in Phoenix.” The Verge. 7 May 2019. https://www.theverge.com/2019/5/7/18536003/waymo-lyft-self-driving-ride-hail-app-phoenix.
- Haywood, Luke, Martin Koning, and Guillaume Monchambert. 2017. “Crowding in Public Transport: Who Cares and Why?” Transportation Research Part A: Policy and Practice 100: 215–227.
- Hensher, David A. 2008. “Influence of Vehicle Occupancy on the Valuation of Car Driver’s Travel Time Savings: Identifying Important Behavioural Segments.” Transportation Research Part A: Policy and Practice 42 (1): 67–76. doi:10.1016/j.tra.2007.06.010.
- Hensher, David A. 2011. “Valuation of Travel Time Savings.” In A Handbook of Transport Economics, edited by André de Palma, et al. Cheltenham: Edward Elgar Publishing. https://ideas.repec.org/h/elg/eechap/12679_7.html.
- iMove. 2019. “Smart Mobility Projects and Trials Across the World.” IMOVE Australia | Transport R&D. 2019. https://imoveaustralia.com/smart-mobility-projects-trials-list/.
- ITRL. 2019. “MMiB Modern Mobility in Barkaby.” KTH. August 6. https://www.itrl.kth.se/research/ongoingprojects/mmib-modern-mobility-in-barkaby-1.917931.
- Johansen, Iver. 2018. “Scenario Modelling with Morphological Analysis.” Technological Forecasting and Social Change 126 (January): 116–125. doi:10.1016/j.techfore.2017.05.016.
- Kane, Mark. 2019. “Scania And Nobina Announce Autonomous EV Bus Trials In Sweden.” InsideEvs.Com. March 28. https://insideevs.com/news/343628/scania-and-nobina-announce-autonomous-ev-bus-trials-in-sweden/.
- Kolarova, Viktoriya, Rita Cyganski, and Barbara Lenz. 2019. “Advances in Transport Policy and Planning.” In Advances in Transport Policy and Planning 3: 171–206. doi:10.1016/bs.atpp.2019.07.002.
- Kolarova, Viktoriya, Felix Steck, and Francisco J. Bahamonde-Birke. 2019. “Assessing the Effect of Autonomous Driving on Value of Travel Time Savings: A Comparison between Current and Future Preferences.” Transportation Research Part A: Policy and Practice 129 (November): 155–169. doi:10.1016/j.tra.2019.08.011.
- Kolarova, Viktoriya, Felix Steck, Rita Cyganski, and Stefan Trommer. 2018. “Estimation of the Value of Time for Automated Driving Using Revealed and Stated Preference Methods.” Transportation Research Procedia 31: 35–46. doi:10.1016/j.trpro.2018.09.044.
- Laine, Anna, Tommi Lampikoski, Tuukka Rautiainen, Marika Bröckl, Christian Bang, Nina Stokkendal Poulsen, and Anders Kofoed-Wiuff. 2018. Mobility as a Service and Greener Transportation Systems in a Nordic Context. TemaNord. Nordic Council of Ministers. doi:10.6027/TN2018-558.
- Li, Zheng, and David A. Hensher. 2011. “Crowding and Public Transport: A Review of Willingness to Pay Evidence and Its Relevance in Project Appraisal.” Transport Policy 18 (6): 880–887. doi:10.1016/j.tranpol.2011.06.003.
- Litman, Todd. 2018. Autonomous Vehicle Implementation - Implications for Transport Planning. Victoria, BC: Victoria Transport Policy Institute.
- Long, Jiancheng, Weimin Tan, W. Y. Szeto, and Yao Li. 2018. “Ride-Sharing with Travel Time Uncertainty.” Transportation Research Part B: Methodological 118: 143–171.
- Mackie, P. J., S. Jara-Diaz, and A. S. Fowkes. 2001. “The Value of Travel Time Savings in Evaluation.” Transportation Research Part E: Logistics and Transportation Review 37 (2–3): 91–106. doi:10.1016/S1366-5545(00)00013-2.
- Milakis, Dimitris, Bart van Arem, and Bert van Wee. 2017. “Policy and Society Related Implications of Automated Driving: A Review of Literature and Directions for Future Research.” Journal of Intelligent Transportation Systems 21 (4): 324–348. doi:10.1080/15472450.2017.1291351.
- Mokhtarian, Patricia L. 2018. “The Times They Are A-Changin’: What Do the Expanding Uses of Travel Time Portend for Policy, Planning, and Life?.” Transportation Research Record: Journal of the Transportation Research Board 2672 (47): 1–11.
- Pernestål, Anna, and Karl Kottenhoff. 2018. “Self-Driving Shuttles as a Complement to Public Transport.” Proceedings of 7th Transport Research arena TRA 2018, Vienna.
- S3 Project. 2018. “S3project.Se.” S3project. 2018. https://s3project.se/en/start-2/.
- SAE International. 2018. Taxonomy and Definitions for Terms Related to Driving Automation Systems for On-Road Motor Vehicles. J30316. SAE International.
- Shires, Jeremy D, and Gerard C De Jong. 2009. “An International Meta-Analysis of Values of Travel Time Savings.” Evaluation and Program Planning 32 (4): 315–325.
- Singleton, Patrick A. 2019. “Discussing the “Positive Utilities” of Autonomous Vehicles: Will Travellers Really Use Their Time Productively?” Transport Reviews 39 (1): 50–65. doi:10.1080/01441647.2018.1470584.
- Skeete, Jean-Paul. 2018. “Level 5 Autonomy: The New Face of Disruption in Road Transport.” Technological Forecasting and Social Change 134 (September): 22–34. doi:10.1016/j.techfore.2018.05.003.
- Small, Kenneth A. 2012. “Valuation of Travel Time.” Economics of Transportation 1 (1-2): 2–14.
- Steck, Felix, Viktoriya Kolarova, Francisco Bahamonde-Birke, Stefan Trommer, and Barbara Lenz. 2018. “How Autonomous Driving May Affect the Value of Travel Time Savings for Commuting.” Transportation Research Record: Journal of the Transportation Research Board April, 036119811875798. doi:10.1177/0361198118757980.
- Townsend, Anthony. 2014. Re-Programming Mobility - The Digital Transformation of Transportation in the United States. NYU Wagner Rudin Center for Transportation Policy and Management. http://reprogrammingmobility.org/wp-content/uploads/2014/09/Re-Programming-Mobility-Report.pdf.
- Trafikverket. 2018. “Analysmetod Och Samhällsekonomiska Kalkylvärden För Transportsektorn: ASEK 6.1.” (In Swedish).
- Varela, Juan Manuel Lorenzo, Maria Börjesson, and Andrew Daly. 2018. “Public Transport: One Mode or Several?” Transportation Research Part A: Policy and Practice 113: 137–156.
- Wadud, Zia, and Fuad Yasin Huda. 2019. “Fully Automated Vehicles: The Use of Travel Time and Its Association with Intention to Use.” Proceedings of the Institution of Civil Engineers - Transport, February, 1–15. doi:10.1680/jtran.18.00134.
- Wardman, Mark. 2014. “Valuing Convenience in Public Transport”.
- Wardman, Mark, and Paul Murphy. 2015. “Passengers? Valuations of Train Seating Layout, Position and Occupancy.” Transportation Research Part A: Policy and Practice 74: 222–238.
- Wiggers, Kyle. 2019. “Pony.Ai Tests Robo-Taxi Program on Its Employees, Promises Self-Driving Trucks Next.” VentureBeat (blog). 19 April 2019. https://venturebeat.com/2019/04/19/pony-ai-launches-robotaxi-employee-program-ponypilot-says-its-developing-self-driving-trucks/.
- Zhao, Xiaoyun, Rami Darwish, and Anna Pernestål. 2019. “Automated Vehicle Traffic Control Tower - the Bridge to next Level Automation.” Integrated Transport Research Lab, KTH Royal Institute of Technology, Stockholm.