Abstract
Uncertain time series analysis aims to explore how the current observation is affected by the disturbance terms and past imprecise observations characterized as uncertain variables. For the case that the current observation is affected by a single past disturbance term, the 1-order uncertain moving average (UMA) model has been tentatively explored. While for the situation that the current observation is affected by multiple past disturbance terms, this paper initiates a high-order UMA model to more accurately describe this relationship. By transforming the high-order UMA model into an uncertain autoregressive model via a backward shift operator, the unknown parameters are calculated through the least squares method. Then, a tth residual is defined to describe the properties of disturbance terms. Furthermore, the forecast value and the confidence interval are derived from the fitted model. Finally, two examples are presented to demonstrate the effectiveness of this method.
Acknowledgments
The authors gratefully acknowledge the financial support provided by Program for Young Excellent Talents in UIBE (No. 18YQ06).
Disclosure statement
No potential conflict of interest was reported by the author(s).
Additional information
Funding
Notes on contributors
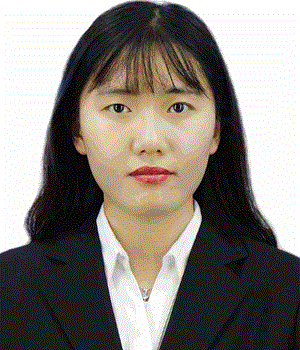
Yue Xin
Yue Xin received the B.S. degree from the Shandong University in 2019. She is currently working towards the Ph.D. degree with the School of mathematics, Renmin University of China. Her current research interests include uncertain time series analysis, uncertain differential equations, and uncertain regression analysis.
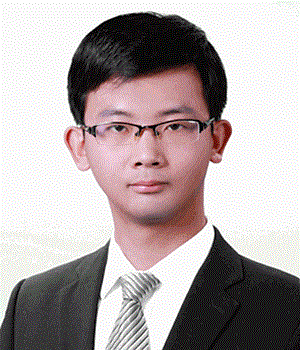
Xiangfeng Yang
Xiangfeng Yang received the B.S. degree from the South-Central University for Nationalities in 2011, the M.S. degree from Renmin University of China in 2014, and the Ph.D. degree from Tsinghua University in 2017. He is currently an associate professor with School of Information Technology and Management, University of International Business and Economics. His current research interests include uncertain game, uncertain time series, and uncertain differential equations.
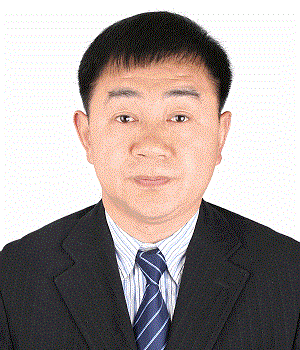
Jinwu Gao
Jinwu Gao received the B.S. degree in Mathematics from Shanxi Normal University in 1996, and the M.S. and Ph.D. degrees in Mathematics from Tsinghua University in 2005. He is currently a professor with School of Economics, Ocean University of China. His current research interests include fuzzy systems, uncertain systems and their application in optimization, game theory, and finance. He has been the Editor-in-Chief of the Journal of Uncertain Systems since 2011, and Associate Editor of Soft Computing since 2017. He has been a Fellow of the Asia Pacific Industrial Engineering and Management Society since 2015, the President of Intelligent Computing Chapter of Operations Society of China since 2015, and the Vice President of International Consortium for Uncertainty Theory since 2013.