Abstract
It is more difficult to manipulate a set-valued decision information system (SVDIS) than common information system due to its strong uncertainty. D–S evidence theory can accurately express the unknowness and uncertainty of knowledge and information, so it has a strong ability to deal with uncertainty. This paper studies the measurement of uncertainty based on D–S evidence theory in an SVDIS. First, a novel pseudo-distance between two objects in an SVDIS considering decision attributes is proposed, Second, the tolerance relation based on the pseudo-distance is established. And then, the belief and plausibility are defined on the basis of the tolerance relation. Experimental and statistical analysis show that the defined belief and plausibility work well in measuring the uncertainty of an SVDIS. Furthermore, λ-belief, λ-belief significance, λ-plausibility and λ-plausibility significance reduction algorithms based on the defined belief and plausibility are proposed and the equivalence between λ-belief reduction algorithm and λ-plausibility reduction algorithm is proved. Experimental results and statistical tests on six data sets with missing values from UCI show that the proposed reduction algorithms are statistically superior to some state-of-the-art algorithms in classification accuracy. These findings will provide a wider perspective on the uncertainty of an SVDIS.
Acknowledgements
The authors would like to thank the editors and the anonymous reviewers for their valuable comments and suggestions, which have helped immensely in improving the quality of the paper.
Disclosure statement
No potential conflict of interest was reported by the authors.
Additional information
Funding
Notes on contributors
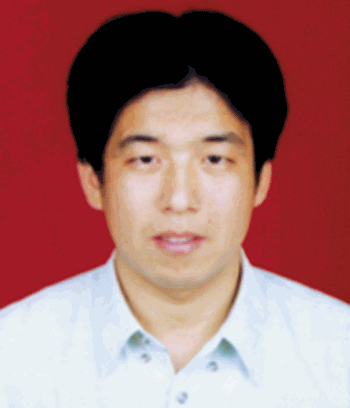
Qinli Zhang
Qinli Zhang received the Ph.D. degree from the School of Information Engineering, Jiangnan University, Wuxi, China, in 2009. He is currently a Professor with the School of Big Data and Artificial Intelligence, Chizhou University, Chizhou, China. His main research interests include fuzzy set theory, rough set theory and bioinformatics.

Lulu Li
Lulu Li received the M.Sc. degree in Computational Mathematics from Dalian University of Technology, Dalian, China, in 2009. He is currently an Associate Professor with the School of Computer Science and Engineering,Yulin Normal University, Yulin, China. His current research interests include machine learning, data mining and rough set theory.