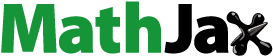
Abstract
The Poisson distribution is here used to illustrate Bayesian inference concepts with the ultimate goal to construct credible intervals for a mean. The evaluation of the resulting intervals is in terms of “mismatched” priors and posteriors. The discussion is in the form of an imaginary dialog between a teacher and a student, who have met earlier, discussing and evaluating the Wald and score confidence intervals, as well as confidence intervals based on transformation and bootstrap techniques. From the perspective of the student the learning process is akin to a real research situation. The student is supposed to have studied mathematical statistics for at least two semesters.
Keywords:
1. Introduction
For illustration of statistical theory and practice the Poisson distribution has proved to be of great value, due to its properties and simplicity, see e.g. Casella and Berger (Citation2002) and Hogg, McKean, and Craig (Citation2005). This article addresses Bayesian issues by way of a discussion between a teacher and a student. They meet on three occasions, when the student is gradually introduced to basic concepts. This ultimately leads to an understanding of the construction of credible intervals and their properties for, in this instance, a Poisson mean.
It is to be understood that during the meetings the teacher and student have access to a whiteboard to facilitate the interaction between them.
2. The first meeting
Teacher: Once again we meet to discuss interval estimation and as before we are going to make use of the Poisson distribution for illustrative purposes. This time however we will not focus on confidence interval constructions, involving various types of approximations and transformations, but instead primarily deal with an altogether different approach, involving Bayesian credible intervals.
Actually, we can start our investigation of Bayesian ideas by simply going back to our previous example about passing vehicles on a specific road, which were modeled as impulses of a homogenous Poisson process (see Andersson Citation2015, Citation2017). We aimed at the construction of a confidence interval for the intensity per hour of the process.
Student: I remember! First we made a theoretical comparison between the Wald, score and other confidence intervals and then we compared these intervals for given data.
Reply: Yes, and after we have gone through the Bayesian methodology, we will get back to this road example.
Student: I must admit that after our previous sessions I felt a certain weariness of confidence intervals in combination with the Poisson distribution. Though when you mention a Bayesian method, I become curious! Some of my recent courses included Bayesian ideas, but not in a very deep and systematic way. Primarily we studied procedures under normal distribution assumptions. As I understand it, the teachers were not fully committed Bayesians.
Reply: I believe that some scholars have fully adapted the Bayesian methodology and others consider themselves pragmatic and use Bayesian methods where they seem useful. There are also the” anti-Bayesians” who believe that the concept is fundamentally wrong, mostly due to its elements of subjectivity. A major complication for us now is that comparisons with our previous results using the classical so called frequentist approach are difficult to make, due to the fundamentally different interpretation of the unknown Poisson parameter θ from a Bayesian point of view.
Student: Well, that much I have understood. A Bayesian treats θ as the outcome of a random variable, say Θ, with a prior distribution, which is updated when we obtain data, thus arriving at a posterior distribution. The resulting inference is then conditional on observed data, but is that not always the case?
Reply: This is true enough, but a “frequentist” is of course not able to make a probabilistic statement about the resulting confidence interval in terms of θ. Now, to get things going, let us start with the fundamental idea of Bayesian inference, which is the following application of Bayes’ rule:
where
represents the joint marginal distribution of
the prior distribution of θ,
the conditional distribution of X given θ and
the conditional posterior distribution of θ given x.
Student: So here we assume a random sample where
Thus
Reply: Yes, so far this is all we can say. But now we have the flexibility to choose a prior Actually, we can start with a situation where we want to be neutral.
Student: I guess that a natural candidate as a prior of θ would then be a uniform continuous distribution, but in that case I must decide on an upper bound for the support.
Reply: Already we have another decision to make! But, as it turns out, does not have to be a proper probability density function (pdf).
Student: In that case, I simply let
Reply: This will work! We can furthermore note that represents the so called mixed discrete continuous joint pdf
Also,
is an example of a flat and improper prior.
Student: But will be a proper pdf if
is not a proper pdf? Do not say anything, I will check it!
and
I can integrate by parts repeatedly. I recall from a math course on Fourier series something called the Kronecker lemma, which leads to
So
Thus
From the integration just performed I can see that this is indeed a proper posterior pdf!
Reply: Good! Do you recognize the posterior distribution?
Student: Not immediately, but let me see (checking a list of distributions). It is a gamma with
and
Reply: Correct. By the way, you used Kronecker’s lemma (Berlin sitzungsberichte 1885 and 1889!) for the integration, but you might instead have considered manipulating the pdf of a gamma distribution.
Student: Of course, that old trick!
If I convert the notation to our situation: so
Reply: You can well imagine that integration to obtain a density soon gets really complicated for more complex situations, such as when we construct so called hierarchical models, where priors for hyperparameters, like α and β for a gamma distribution, are taken into account. Computer intensive methods like MCMC (Markov Chain Monte Carlo) to simulate samples from distributions are then of great help.
If we continue to consider the gamma distribution, what happens if we let ? (α and β are assumed to be known.)
Student: When was flat, the result was a gamma distribution, so a not very wild guess would be that a gamma prior leads to a gamma posterior!
Reply: Correctly “guessed”! What are the resulting gamma parameters?
Student: If I start with the prior
I do not need to evaluate
since it does not depend on θ and is therefore not contributing to information about the parameters. The posterior is then
Reply: You have illustrated that the family of gamma distributions is the conjugate family of distributions for the Poisson distribution. It is not unproblematic though to choose values or prior distributions of α and β for a gamma prior, unless we have vast experience and/or access to a great amount of previous data. A compromise between the flat and gamma priors is given by This could be reasonable if we tend to believe more in lower than in higher values of θ. Furthermore, we do not have to choose specific values of parameters. What is the posterior distribution given this prior?
Student:
I trust that this is enough, verifying that we once again arrive at a gamma distribution, this time with
and
a result close to our first case with the flat prior.
Reply: I agree! Actually, this prior belongs to the class of Jeffreys’s priors, where and where
is the Fisher information number. Check that!
Student: All right, but since the Poisson distribution belongs to an exponential family of distributions, we also have that
Here
which means that
and
so
Reply: Good! Furthermore we can observe that the class of Jeffreys’s priors belongs to a type of priors which are said to be noninformative, in the sense that we have invariance under reparameterization.
Student: You say that the prior does not need to be flat in order to be called noninformative? What does the invariance property signify here?
Reply: Yes and the invariance property means that if we let where u is a one-to-one function, then
implies
Can you show this?
Student: The density for according to the general transformation” formula”, should be
and
A lot of brackets!
Reply: Well, you need all of them!
Student: Then, using the chain rule I get and since
I am done!
Reply: Excellent. We have not yet discussed the issue of constructing credible intervals for θ, but before doing that, let us briefly look at the expected value for the three posterior distributions that you have derived.
Student: Generally for a the expected value is
so
So, with respect to expectation the influence of the parameters on the priors decreases with increasing values of n, since all three expressions eventually get close to
This makes sense!
Reply: Yes indeed! We can also observe that it seems relevant to call the first two priors uninformative. Furthermore the expectations can be seen as functions of the prior mean and the maximum likelihood estimate
Student: That escaped me regarding the last expression, but let me try and rewrite it:
This is elegant! We get a weighted average of the sample mean
and the prior mean
where the first weight tends to 1 and the second weight to 0 when
Reply: The conditional expectation is actually a Bayes’s point estimate of θ. It is formally derived as the decision function
which minimizes the conditional expectation of the loss function
(Ideally we should perhaps call this a predicted value of Θ rather than an estimated value of θ.)
We are now finally prepared for the Bayesian interval estimates of θ. They are called credible intervals and are constructed as posterior prediction intervals for Θ.
Student: Then if the posterior distribution is known, we can find, say, a and b so that
(Intentionally I avoided the use of α at the right hand side!)
Reply: Yes, and it is important to acknowledge that a and b depend on x.
Student: And on α and β for the gamma prior!
Reply: True! As usual we can further choose, for the sake of uniqueness and symmetry, a and b so that
Bayes’s interval estimation is really more straightforward than Bayes’s point estimation, where in the latter situation we have to specify a loss function.
It is also worth pointing out that is a sufficient statistic for the Poisson parameter θ, so we can condition on
instead of x.
Now I think it is time to study the behavior of credible intervals based on the prior and posterior distributions we have considered here. This could be accomplished analytically or by a simulation study, where you can labor with different scenarios. There will be many degrees of freedom for you!
Student: I accept the challenge!
Reply: Good! We will meet again in a few days and discuss how to accomplish this.
3. The second meeting
So, have you given this enough thought, do you think?
Student: Well, at first it seemed a bit strange to evaluate credible intervals, since what we get in the end is an interval with a prespecified probability of coverage. Could the length be something to consider?
Reply: Impolitely I will reply to your question with another. Did you consider comparisons with confidence intervals for a fixed parameter?
Student: That was tempting and I have been looking into what Casella and Berger have to say about this very case with the Poisson distribution parameter in their “Statistical Inference”. They make comparisons between confidence and credible intervals and I guess the main conclusion is that a confidence interval evaluated as a credible interval can perform poorly and vice versa. Is comparing these two concepts like comparing apples with oranges?
Reply: Not even that! I have a colleague who says that it is like comparing an apple with a lorry! It is really not very fruitful to make such comparisons, since the underlying concepts differ so much.
Student: Evaluating cases where we use the “wrong” prior might be something?
Reply: It is indeed problematic to discuss in terms of “right” and “wrong” in a Bayesian context, but it could be a way to illustrate the influence of the prior on the posterior. We must not forget though that the prior is the choice of the researcher and thus subjective.
Student: In that case I should first compute and
given whatever p and prior I have and then use these limits to compute the probability of coverage using a” wrong” posterior? I take it that since the first two priors we have discussed are similar, I may consider only two of them?
Reply: Yes, and do not forget to try different sample sizes to observe how the effect of a “wrong” prior changes.
Student: Another thing, I have been thinking about the derivation of the posterior for the flat prior, where we obtained a gamma posterior. When I generate a random number I want to use a uniform distribution with some upper bound for the support and then the posterior will not exactly be a gamma distribution?
Reply: No, but in this case we can approximate with a The density function for the true posterior will have an adjustment factor, which is quite close to 1 even for moderate sizes of n.
Student: I think I know what to do now, so I will get back to my computer and MATLAB and hopefully return with some interesting results!
Reply: Good luck, see you next week!
4. The third meeting
Teacher: Now I am curious about your results!
Student: I find them quite interesting even though they are not dramatic.
Reply: Something undramatic can be important too!
Student: Maybe! Anyway, there are some nice functions in MATLAB for generating values from distributions and computing probabilities and quantiles, so programing this was not hard work. For the flat prior I have used a uniform distribution on (0, 100) to cover a wide enough range of possible values of θ. For the “wrong” posterior as a first step I chose different combinations of α and β in such a way that the expected value equaled that of the prior (in this case 50). I have used the same p = 0.95 throughout and the sample sizes n = 10, 50 and 100. 10 000 Poisson samples were generated for each setup.
I could perhaps show the results of these cases first before I move on describing the other scenarios?
Reply: Please do!
Student: So, for the sake of completeness I include the results for all my chosen sample sizes, even if the level of inclusion is close to 0.95 already for n = 10.
Reply: By an inclusion probability you mean
where
is a posterior based on a “wrong” prior?
Student: Yes, I admit I have not defined it properly, but I think inclusion is a proper expression, since θ is supposed to be random. Coverage does not seem suitable here.
Reply: I agree. Actually, in design-based survey sampling theory the inclusion probability equals the probability that a unit in the population is included in the random sample.
Looking at your results, in these cases there is not much penalty for assuming the “wrong” prior. Maybe you thought that we should get gradually deteriorating results for decreasing values of α?
Student: Yes, since the skewness coefficient for a is
I expected that, but then I realized that one should also consider variances:
for the “true” uniform prior and
for the first gamma combination (α = 5, β = 10) and 1000 for the second.
Reply: Yes, that could very well be the reason why we get this pattern of inclusion probabilities. Did you try some “uglier” scenarios?
Student: Well, the next setup was letting with the following results:
Reply: Still high inclusion probabilities!
Student: Next I tried
Finally something substantially lower than 0.95!
Reply: However, you have to be pretty far off the mark with respect to the expected value to get this effect and then only for n = 10. There is clearly some stability here.
Student: After this I turned to the opposite situation, where the “true” prior is a while “falsely” assuming a flat improper prior. I started with the same pairs of α and β values as for the previous situation. Here is what happened for n = 10:
There is a tendency of decreasing inclusion probabilities for decreasing values of α, so then I tested which gave me the inclusion probability 0.924 and the combination
resulted in 0.917. Still the inclusion probabilities are higher than 90%, but for
the result was 0.780. The same combination with n = 50 yielded 0.808 and with n = 100 I got 0.820. Thus, this is an example of a situation where we need a lot of information from the sample to “correct” for a misspecified prior.
Reply: You have clearly shown that one can construct examples of “wrong” priors, which lead to unreliable credible interval limits in terms of reduced inclusion probabilities. However, as we have mentioned earlier, the discussion of “right” or “wrong” priors is conceptually problematic. In practice the prior is chosen using information from previous similar situations and/or your own belief which we consider to be subjective. What you also could have studied is how the outcome of the prior affects your empirical inclusion probabilities, that is, what happens locally if you get a value of θ far away from its expectation?
Student: I guess there is some degree of subjectivity in all types of approaches including the frequentistic.
Reply: Yes, you can say that already in the choice of the approach to be used you are actually being subjective.
Before we part, let us apply one of your derived posteriors to the road example.
Student: Yes, that would be interesting. I could try using the flat prior assuming that we do not have access to reliable previous traffic data.
The observed value of passing vehicles in one hour’s time was x = 28, so I get the posterior since n = 1. Using the gaminv function I arrive at the 95% credible interval
Reply: Good. Finally, let us compare this interval numerically with the Wald and score intervals for this case.
Student: The Wald interval was and the score interval
!
Reply: Well, that was a bit of a surprise, though I suspected that the credible interval would be closer to the score interval than to Wald’s.
Student: Is the main connection between the Poisson and its conjugate distribution, which is the gamma, that they are both positively skewed?
Reply: Yes, that was my thought. However, we must not forget the difference in interpretation between the interval concepts. You remember the comparison between an apple and a lorry?
Student: Absolutely! This has really been an interesting scientific journey for me! It is amazing how much you can illustrate in terms of statistical methodology just using the Poisson distribution.
Reply: It is certainly a very useful distribution. I am glad you have appreciated these excursions to “Poissonland”!
5. Summary
Once again the Poisson distribution and its parameter θ turn out to be very useful for the illustration of statistical inference theory. Particularly when working within the Bayesian approach where several technical steps are required, we need a distribution which is simple to work with, yet inhabiting interesting properties. This article is aimed at the construction of credible intervals and their properties when assuming “wrong” priors in some simple situations.
Table 1. Empirical inclusion probabilities for credible intervals of a Poisson mean given p = 0.95 for the uniform (0, 100) prior combined with the” wrong” gamma prior where
Table 2. Empirical inclusion probabilities for credible intervals of a Poisson mean given p = 0.95 for the uniform (0, 100) prior combined with the “wrong” gamma prior where
Table 3. Empirical inclusion probabilities for credible intervals of a Poisson mean given p = 0.95 for the uniform (0, 100) prior combined with the “wrong” gamma prior where
Table 4. Empirical inclusion probabilities for credible intervals of a Poisson mean given p = 0.95 for a prior combined with the “wrong” assumption of a flat improper gamma prior.
References
- Andersson, P. G. 2015. A classroom approach to the construction of an approximate confidence interval of a Poisson mean using one observation. The American Statistician 69 (3):160–4. doi: 10.1080/00031305.2015.1056830.
- Andersson, P. G. 2017. A classroom approach to illustrate transformation and bootstrap confidence interval techniques using the Poisson distribution. International Journal of Statistics and Probability 6 (2):42–53. doi: 10.5539/ijsp.v6n2p42.
- Casella, G., and R. L. Berger. 2002. Statistical inference. 2nd ed. Pacific Grove, CA: Duxbury Press.
- Hogg, R. V., J. W. McKean, and A. T. Craig. 2005. Introduction to mathematical statistics. 6th ed. Upper Saddle River, NJ: Pearson - Prentice Hall.