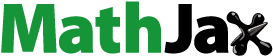
Abstract
In this paper, we introduce the notion of strongly generalized convex functions which is called as strongly η-convex stochastic processes. We prove the Hermite-Hadamard, Ostrowski type inequality, and obtain some important inequalities for above processes. Some previous results are special cases of the results obtained in this paper.
1. Introduction
Karamardian (Citation1969) introduced strongly convex functions. However, we can find some references (Merentes and Nikodem Citation2010; Nikodem and Pales Citation2011) citing Polyak (Citation1966) as being the pioneer to introduce this notion. It is well known that every continuously differentiable function is strongly monotone if its Jacobian matrix is strongly positive definite (Karamardian Citation1969). Awan et al. (Citation2017) introduced the notion of strongly η-convex functions and obtained some new integral inequalities of Hermite-Hadamard and Hermite-Hadamard-Fejér type for strongly η-convex functions.
Practical applications of stochastic processes include areas of image processing, signal processing, control theory, information theory, computer science, cryptography, telecommunications, neuroscience, statistics, physics, biology, chemistry and ecology, see (Allen Citation2010; Kotrys Citation2012; Nikodem Citation1980; Sobczyk Citation2013) and their references. Nikodem (Citation1980) introduced some powerful properties of convex stochastic processes. Nikodem (Citation1980) proved that a convex stochastic processes is continuous if and only if for all
and
where
denotes the extended real line. It is well known that if a stochastic process
is convex and measurable, then it is continuous (Nikodem Citation1980). Kuhn (Citation2006) studied the convex stochastic programs with a generalized non convex dependence on the random parameters. Kuhn (Citation2006) proved that, under certain conditions, the saddle structure can be restored by adding specific random variables to the profit functions. These random variables are referred to as ‘correction terms’. Allen (Citation2010) introduced basic theory of stochastic processes and applying these methods to biological problems, such as enzyme kinetics, population extinction, the spread of epidemics and the genetics of inbreeding.
Kotrys (Citation2013) defined the strongly convex stochastic processes and derived the Hermite-Hadamard inequality, Jensen inequality, Kuhn and Bernstein theorem. A stochastic process is strongly
-convex with modulus
if and only if the stochastic process
defined by
is
-convex (Kotrys Citation2013). Further, Ibrahim (Citation2020) introduced the concept of strongly h-convex stochastic process. Jung et al. (Citation2021) introduced the notion of η-convex stochastic processes and established Jensen, Hermite-Hadamard and Ostrowski type inequalities. Further, Fu et al. (Citation2021) derived Hölder-İşcan and Improved power mean integral inequalities, and proved Hermite-Hadamard type integral inequalities for
polynomial stochastic processes. For more study of Hermite-Hadamard inequalities, we refer to (Karahan and Okur Citation2018; Omaba and Nwaeze Citation2020; Özcan Citation2019; Sharma, Mishra, and Hamdi Citation2020, Sharma et al. Citation2021).
Recently, Farid et al. (Citation2021) proposed refinements of fractional versions of the Hadamard inequalities for Caputo fractional derivatives using strongly convex functions. Tariq et al. (Citation2021) defined harmonic s-type convex functions and investigated the refinements of Ostrowski type inequalities for s-type convex functions. Further, Omaba, Omenyi and Omenyi (Citation2021) obtained the new Hadamard type inequalities for a class of s-Godunova-Levin functions of the second kind for fractional integrals. Iftikhar et al. (Citation2021) introduced some new generalized different types of kernels, development of new identities and new error bounds of Ostrowski type inequalities for first and second derivable mappings.
Stochastic convexity and its applications are very important in mathematics, probability, so researchers are attracting to develop generalized stochastic convexity. However, Hermite-Hadamard and some other integral inequalities for strongly η-convex stochastic processes have not been studied. So we propose a new class of convex functions known as strongly η-convex stochastic processes. The definition of strongly η-convex stochastic processes is motivated by the definition of η-convex, strongly convex stochastic processes and strongly η-convex functions. Thus, the purpose of this paper is to establish Hermite-Hadamard, Ostrowski and some other integral inequalities for strongly η-convex stochastic processes.
The organization of the paper is as follows: In Section 2, we recall some basic results that are necessary for our main results. In Section 3, we introduce the concept of strongly η-convex stochastic processes. Further, we prove the Hermite-Hadamard inequality, Ostrowski inequality and some other interesting inequalities for this paper. Some special cases of these results are also investigated in Section 3. In Section 4, we discuss the conclusions and future directions of this study.
2. Preliminaries
A stochastic process X(t) is a function which maps the index set T into the space S of random variable defined on
Definition 1.
(Sobczyk Citation2013) The stochastic process is called
continuous in probability in interval I, if for all
where
denotes the limit in probability;
mean square continuous in the interval I, if for all
where
denotes the expectation value of the random variable
increasing(decreasing), if for all
such that
monotonic if it is increasing or decreasing;
differentiable at a point
if there is a random variable
Definition 2.
(Sobczyk Citation2013) Suppose that is a stochastic process with
for all
and
is a partition of
and
for all
Further, suppose that
be a random variable. Then, it is said to be mean-square integral of the process X on
if for each normal sequence of partitions of the interval
and for each
we have
Then, we can write
Also, mean square integral operator is increasing, that is,
where
Theorem 1.
(Kotrys Citation2012) Let is convex and mean square continuous in the interval I. Then for any
we have
Definition 3.
(Kotrys Citation2013) Let denote a positive random variable. The stochastic process
is called strongly convex with modulus
if for all
and
the inequality
is satisfied.
Theorem 2.
(Kotrys Citation2013) Let be a stochastic process, which is strongly Jensen convex with modulus
and mean square continuous in the interval I. Then for any
we have
Definition 4.
(Jung et al. Citation2021) Let be a probability space and
be an interval, then
is an η-convex stochastic process if
Theorem 3.
(Fu et al. Citation2021; Hölder-İşcan integral inequality) Let be real stochastic process and
be mean square integrable on
If p > 1 and
then the following inequality holds almost everywhere:
Theorem 4.
(Fu et al. Citation2021; Improved power mean integral inequality) Let be real stochastic process and
be mean square integrable on
If
then the following inequality holds almost everywhere:
Lemma 1.
(Gonzales, Materano, and Lopez Citation2016) Let be a stochastic process which is mean square differentiable on I0. If
is mean square integrable on
where
with
then the following equality holds
Lemma 2.
(Fu et al. Citation2021) Let be a mean square differentiable stochastic process on I0 and
is mean square integrable on
, where
Then we have almost everywhere
Theorem 5.
(Jung et al. Citation2021) Suppose that is an η-convex stochastic process such that η is bounded above on
then the following inequalities hold almost everywhere:
3. Main results
In this section, we derive our main results.
Definition 5.
Let be a probability space and
be an interval. Let
denote a positive random variable, then
is said to be strongly η-convex stochastic process with respect to
and modulus
if
Remark 1.
If , then the definition of strongly η-convex stochastic process reduces to the definition of strongly convex stochastic process proposed by Kotrys (Citation2013). When
then above definition reduces to the definition of η-convex stochastic process (Jung et al. Citation2021).
Example 1.
Let be a stochastic process defined as
and
Then X is strongly η-convex stochastic processes with modulus 1.
Theorem 6.
A random variable is an strongly η-convex stochastic process with modulus
if and only if for any
with
we have
Proof.
Suppose that X is an strongly η-convex stochastic process and with
Then there exist
such that
This implies,
or
For the converse part, take with
Choose any
then we have
The above determinant is
This implies
□
Theorem 7.
Suppose that is an strongly η-convex stochastic process with modulus
, such that η is bounded above on
, then the following inequalities hold almost everywhere:
where
is an upper bound of η.
Proof.
Since X is strongly η-convex stochastic process, therefore
This implies,
Similarly,
By using the change of variable technique, we have
(1)
(1)
We now prove the right hand side of the theorem. Since X is strongly η-convex stochastic process with modulus we get
Integrating above inequality with respect to λ on both sides from 0 to 1, we have
This implies,
Similarly,
Therefore,
(2)
(2)
From EquationEquations (1)(1)
(1) and Equation(2)
(2)
(2) , we have
(3)
(3)
□
Remark 2.
When , then above theorem reduces to Theorem 5. If we consider
, then above theorem reduces to the classical Hermite-Hadamard inequality for convex stochastic process (Kotrys Citation2012).
Theorem 8.
If a stochastic process be an strongly η-convex with modulus
and integrable on
we have
Proof.
Since X is strongly η-convex stochastic process, therefore
(4)
(4)
and
(5)
(5)
From EquationEquations (4)(4)
(4) and Equation(5)
(5)
(5) , we obtain
Integrating above inequality from 0 to 1 on both sides with respect to we have
This implies,
□
Remark 3.
When X is strongly log-convex stochastic process, then above theorem reduces to Theorem 3 of (Tomar, Set, and Bekar Citation2014).
Theorem 9.
Let be a mean square stochastic process such that
is mean square integrable on
where
with
If
is an strongly η-convex stochastic process with modulus
on I and
for every t, then
Proof.
Recall Lemma 1:
Since is strongly η-convex stochastic process, therefore
□
Remark 4.
When , then above theorem reduces to Theorem 4.2 of (Jung et al. Citation2021).
Theorem 10.
Let be a mean square differentiable stochastic process on I0 and
be a mean square integrable on
, where
If
is an strongly η-convex stochastic process on
then we have almost everywhere:
Proof.
From Lemma 2, we have
Using the definition of strong η-convex stochastic process in above inequality, we have
□
Corollary 1.
When in above theorem, then we obtain the following inequality for η-convex stochastic processes:
Remark 5.
When and
then above theorem reduces to Corollary 5.3 of (Fu et al. Citation2021).
Theorem 11.
Let be a mean square differentiable stochastic process on I0 with
and assume that
be a mean square integrable on
, where
If
is an strongly η-convex stochastic process on
then we have almost everywhere:
Proof.
From Lemma 2, we have
Using Hölder’s inequality and the definition of strong η-convex stochastic process in above inequality, we obtain
□
Corollary 2.
When in above theorem, then we obtain the following inequality for η-convex stochastic processes:
Remark 6.
When and
then above theorem reduces to Corollary 5.5 of (Fu et al. Citation2021).
Theorem 12.
Let be a mean square differentiable stochastic process on I0 with
and assume that
be a mean square integrable on
, where
If
is an strongly η-convex stochastic process on
then we have almost everywhere:
Proof.
For we use the estimates from the proof of Theorem 10. Now we prove result for
From Lemma 2, we have
Using Hölder’s inequality and the definition of strong η-convex stochastic process in above inequality, we obtain
□
Corollary 3.
When in above theorem, then we obtain the following inequality for η-convex stochastic processes:
Remark 7.
When and
then above theorem reduces to Corollary 5.8 of (Fu et al. Citation2021).
Theorem 13.
Let be a mean square differentiable stochastic process on I0 with
and assume that
be a mean square integrable on
, where
If
is an strongly η-convex stochastic process on
then we have almost everywhere:
Proof.
From Lemma 2, we have
Using Hölder’s inequality and the definition of strong η-convex stochastic process in above inequality, we get
□
Corollary 4.
When in above theorem, then we obtain the following inequality for η-convex stochastic processes:
Remark 8.
When and
then above theorem reduces to Corollary 5.10 of (Fu et al. Citation2021).
Theorem 14.
Let be a mean square differentiable stochastic process on I0 with
and assume that
be a mean square integrable on
, where
If
is an strongly η-convex stochastic process on
then we have almost everywhere:
Proof.
For we use the estimates from the proof of Theorem 10. Now we prove result for
From Lemma 2, we have
Using improved power-mean integral inequality and the definition of strong η-convex stochastic process in above inequality, we get
□
Corollary 5.
When in above theorem, then we obtain the following inequality for η-convex stochastic processes:
Remark 9.
When and
then above theorem reduces to Corollary 5.13 of (Fu et al. Citation2021).
4. Conclusion
We have defined strong η-convexity for stochastic processes. We have established the Hermite-Hadamard, Ostrowski and some important inequalities for the above mentioned processes. The results obtained in this paper are the generalization of the previously known results. In the future, new integral inequalities for the other generalized convex stochastic processes can be obtained using similar methods in this study.
Acknowledgments
The authors are indebted to the anonymous reviewers for their valuable comments and remarks that helped to improve the presentation and quality of the manuscript.
Additional information
Funding
References
- Allen, L. J. 2010. An introduction to stochastic processes with applications to biology. Boca Raton, FL: CRC Press.
- Awan, M. U., M. A. Noor, K. I. Noor, and F. Safdar. 2017. On strongly generalized convex functions. Filomat 31 (18):5783–90. doi: 10.2298/FIL1718783A.
- Farid, G., A. U. Rehman, S. Bibi, and Y. -M. Chu. 2021. Refinements of two fractional versions of hadamard inequalities for caputo fractional derivatives and related results. Open Journal of Mathematical Science 5 (1):1–10.
- Fu, H., M. S. Saleem, W. Nazeer, M. Ghafoor, and P. Li. 2021. On Hermite-Hadamard type inequalities for n-polynomial convex stochastic processes. AIMS Mathematics 6 (6):6322–39. doi: 10.3934/math.2021371.
- Gonzales, L., J. Materano, and M. Lopez. 2016. Ostrowski-type inequalities via hconvex stochastic processes. JP Journal of Mathematical Sciences 16 (2):15–29.
- Ibrahim, A. 2020. On strongly h-convex stochastic processes. Journal of Quality Measurement and Analysis JQMA 16 (2):243–51.
- Iftikhar, M., A. Qayyum, S. Fahad, and M. Arslan. 2021. A new version of ostrowski type integral inequalities for different differentiable mapping. Open Journal of Mathematical Science 5 (1):353–9.
- Jung, C. Y., M. S. Saleem, S. Bilal, W. Nazeer, and M. Ghafoor. 2021. Some properties of η-convex stochastic processes. AIMS Mathematics 6 (1):726–36. doi: 10.3934/math.2021044.
- Karahan, V., and N. Okur. 2018. Hermite-Hadamard type inequalities for convex stochastic processes on n-coordinates. Turkish Journal of Mathematics and Computer Science 10:256–62.
- Karamardian, S. 1969. The nonlinear complementarity problem with applications, part 1. Journal of Optimization Theory and Applications 4 (2):87–98. doi: 10.1007/BF00927414.
- Kotrys, D. 2012. Hermite–Hadamard inequality for convex stochastic processes. Aequationes Mathematicae 83 (1–2):143–51. doi: 10.1007/s00010-011-0090-1.
- Kotrys, D. 2013. Remarks on strongly convex stochastic processes. Aequationes Mathematicae 86 (1–2):91–8. doi: 10.1007/s00010-012-0163-9.
- Kuhn, D. 2006. Generalized bounds for convex multistage stochastic programs. Vol. 548. Berlin: Springer.
- Merentes, N., and K. Nikodem. 2010. Remarks on strongly convex functions. Aequationes Mathematicae 80 (1–2):193–9. doi: 10.1007/s00010-010-0043-0.
- Nikodem, K. 1980. On convex stochastic processes. Aequationes Mathematicae 20 (1):184–97. doi: 10.1007/BF02190513.
- Nikodem, K., and Z. Pales. 2011. Characterizations of inner product spaces by strongly convex functions. Banach Journal of Mathematical Analysis 5 (1):83–7. doi: 10.15352/bjma/1313362982.
- Omaba, M., L. O. Omenyi, and L. Omenyi. 2021. Generalized fractional hadamard type inequalities for (qs)-class functions of the second kind. Open Journal of Mathematical Sciences 5(1):270–8.
- Omaba, M. E., and E. R. Nwaeze. 2020. Generalized fractional inequalities of the Hermite–Hadamard type for convex stochastic processes. Annales Mathematicae Silesianae 35 (1):90–104. doi: 10.2478/amsil-2020-0026.
- Özcan, S. 2019. Hermite-hadamard type inequalities for exponentially p-convex stochastic processes. Sakarya University Journal of Science 23 (5):1012–8. doi: 10.16984/saufenbilder.561040.
- Polyak, B. T. 1966. Existence theorems and convergence of minimizing sequences in extremum problems with restrictions. Soviet Mathematics - Doklady 7:72–5.
- Sharma, N., R. Mishra, and A. Hamdi. 2020. Hermite-Hadamard type integral inequalities for multidimensional general h-harmonic preinvex stochastic processes. Communications in Statistics-Theory and Methods 51:6719–40.
- Sharma, N., S. K. Singh, S. K. Mishra, and A. Hamdi. 2021. Hermite–Hadamard-type inequalities for interval-valued preinvex functions via Riemann–Liouville fractional integrals. Journal of Inequalities and Applications 2021 (1):1–15. doi: 10.1186/s13660-021-02623-w.
- Sobczyk, K. 2013. Stochastic differential equations with applications to physics and engineering. Vol. 40. Dordrecht: Springer.
- Tariq, M., S. I. Butt, and S. Butt. 2021. Some ostrowski type integral inequalities via generalized harmonic convex functions. Open Journal of Mathematical Sciences 5 (1):200–8.
- Tomar, M., E. Set, and N. O. Bekar. 2014. Hermite-hadamard type inequalities for strongly-log-convex stochastic processes. Journal of Global Engineering Studies 1:53–61.