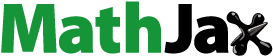
ABSTRACT
The number of agricultural interventions promoting biofortified crops as a strategy to combat micronutrient malnutrition have increased significantly during the past decade. These interventions aim to improve the intake of key nutrients and sometimes other nutrition outcomes. In this study, we assess the impact of a market-oriented gender-sensitive agriculture-health project on household food consumption and food security. We use gender-disaggregated data from 842 sweetpotato farmers and a series of econometric techniques to assess the project’s impact on food consumption household food security. The study finds unambiguous and strong impact of the project on both household food consumption and food security. Moreover, female-headed participant households benefitted much more from participation than did male-headed households. We further find that nonparticipant households would also have benefitted had they participated. The findings imply the importance of designing market-focused projects with a gender lens. They also imply that market-oriented agriculture-sensitive interventions do not necessarily harm the nutritional status of farm households.
Introduction
Agricultural interventions are an important avenue for improving household food and nutrition security of farm families. Adoption of improved agricultural technologies can be a powerful strategy for increasing food availability, hence consumption, and income from surplus sales (Feleke, Kilmer, and Gladwin Citation2005). Incomes from surplus sales can be used by farm households to buy other foods thus diversifying diets or meeting other urgent household needs.
In developing countries, women contribute a significant share to agricultural labor and engaged in various activities that enhance productivity (Okonya et al. Citation2019). However, gender differences in the agricultural sector, due to differences in resource access, inherent gender norms and lack of decision-making power by women impede agricultural productivity (Farnworth et al., Citation2019; Labintan & Ding, Citation2012; Mudege et al. Citation2016). Consequently, recent interventions are increasingly targeting women with the aim of ensuring that they participate substantively in projects designed to improve household welfare. Such projects include those designed to improve productivity, production, and consumption of nutritious foods, participation in commercial value chains (Low et al., Citation2017). To date, there is still very limited understanding of the impacts of gendered agricultural interventions targeting food and nutrition security on rural vulnerable farm households. In particular, over the last decade, there has been a surge in interventions that deploy nutritious biofortified staple food crops along with differing degrees of nutrition education targeting such households. Some of these interventions use food-basket and nutrition education approaches geared at increasing the consumption of diverse diets that incorporate the nutritious biofortified crops (Mutiso et al. Citation2018; Okello et al. Citation2019). Others focus on the development of commercial value chains in which smallholder farmers are linked to private sector processing firms to boost their incomes and improve household welfare (Okello et al. Citation2019). The majority of these interventions target vulnerable rural farm households, especially female and male caregivers of young children pregnant women and breastfeeding mothers, with the goal of tackling the often high micronutrient deficiency among such household members (Shikuku et al. Citation2019) or avoiding the exclusion of women in the value chain as it commercializes (World Bank, Citation2012; Katungi, Kabungo, and Mukankusi Citation2019). Such interventions tend to assume that the nutrition-sensitive agricultural intervention will improve food availability and incomes and stimulate changes in food consumption and other nutrition outcomes among vulnerable populations, and ultimately contribute to the alleviation of overall food insecurity among rural households.
This study rigorously investigates the impact of a gender-sensitive agricultural intervention on food consumption and food insecurity among rural farm households in Rwanda. It also examines the factors explaining differences in food consumption and food insecurity among female and male-headed households. The study was conducted in rural communities exposed to an agricultural intervention that targeted female and male-headed households with nutritious biofortified orange-fleshed sweetpotato (OFSP). The main aim of the intervention was to provide participating households with access to a new market for biofortified orange-fleshed sweetpotato roots by linking them to a root processor. Surplus production or unmarketed produce was expected to boost household food availability while income from OFSP sales was to boost market purchases of other foods, ultimately jointly improving food consumption and reducing food insecurity. Targeted households received minimal nutrition education on the benefits of consuming OFSP along with agronomic training focusing on recommended practices for growing this relatively new type of sweetpotato.
The data used in this paper were collected from different categories of households, namely project participating, spillover, and control households (defined in the next section). To check the robustness of our findings, we employed different regression techniques. Specifically, the paper applied propensity score matching, endogenous switching and inverse probability weight-adjusted regression models. Recent literature has tended to apply multiple techniques in assessing impacts of agricultural interventions usually with the aim of checking the robustness of the estimates because of the difficulties of controlling for confounding endogenous effects (Ochieng et al. Citation2019; Shikuku et al. Citation2019; Wossen et al. Citation2017). The study focused on sweetpotato producing farm households in five districts of Rwanda, namely Rulindo, Gakenke, Muhanga, Kamonyi, and Burera
The study context
Rwanda is one of the smallest countries in Africa, with a total area of 26,334 square km, and a population of about 12 million people; and hence, has one of the highest population densities (i.e. 460 persons per square meter) in the region (Muhinda, Citation2013). Agriculture is the main economic activity, contributing 34% of the gross domestic product, 80% of the employment, and generating 70% of the foreign earnings (Muhinda, 2013). It is also the main source of food for a rapidly increasing population. Food insecurity and malnutrition are major challenges. It is estimated that 22% of the rural population suffered from chronic food insecurity and that 35% of the children under five years of age were malnourished in 2018 (World Food Programme (WFP) Citation2018). Gender difference in agricultutral productivity and women’s poor access to productive resources exacerbate these problems, despite women contributing to about one-half of the agricultural workforce in Rwanda (Alkire et al. Citation2013; Daroque and Gasson Citation1991; Galie, Jiggins, and Struik Citation2013; Kokeye et al. Citation2013).
Sweetpotato is one of the main staple food crops in Rwanda. On average, the Rwandese annually consume 88 kg per capita.Footnote1 The crop accounts for 7.1% of the land under crop cultivation (NISR, Citation2015). The share of sweetpotato in the total production is the highest nationally (18.3%), and is followed by beer banana (17.2%). Statistics show that sweetpotato production quadrupled in Rwanda between 1960 and 2014 (Food and Agriculture Organization (FAO), Citation2013). Thus, sweetpotato is an important staple and food security crop. However, majority of the households grow white/cream and yellow-fleshed varieties, which are typically very low in beta-carotene, a precursor for vitamin A. Vitamin A deficiency a major public health problem in most developing countries and results mainly from inadequate intake of vitamin A in commonly consumed diets (Low et al., 2018).
To contribute to the fight against malnutrition and food insecurity, the International Potato Center (CIP) and its partners, namely the Rwanda Agriculture Board (RAB), Catholic Relief Services (CRS), Young Women Christian Association (YCWA), and Imbaraga Farmers Association (IFA), implemented a nutrition-sensitive market-focused project targeting households at the greatest risk of micronutrient malnutrition. Known as the Rwanda Superfoods project, the project lasted from 2010 to 2014. It targeted households with young children (i.e. under 5 years old), pregnant women, and breastfeeding mothers. CIP and RAB were responsible for establishing the sweetpotato seed system, while CIP and its nongovernmental organization partners (namely, YWCA, CRS and IFA) facilitated linkages between the agro-processor, Urwibutso Enterprises Limited, and the participating farmers' groups. The partners disseminated planting material of nutritious biofortified sweetpotato (i.e., the OFSP), conducted trainings on good sweetpotato agronomic practices, and on how to utilize the OFSP at home.
The major objective of the Rwanda Superfoods project was to enhance the income of both women and men through production and sale of OFSP to an agro-processor. It secondarily aimed to improve household food availability and OFSP consumption which were expected to reduce the incidence of food insecurity. Given that there was no major nutrition education component, a key research question is whether the focus on income generation improved dietary practices at the household level. A second research question is whether, by improving household food availability, the project improved the food security status of vulnerable households. That is, can such a market-oriented project lead to improved food intakes by households? Moreover, the project was conducting the first pilot in Africa to develop a commercial product, in this case, a biscuit, using orange-fleshed sweetpotato purée as a key ingredient. Evidence from Kenya indicates that men can displace women from growing traditionally women-dominated crop when its production becomes commercialized (Dolan Citation2001; Nyabaro, Mburu, and Hutchinson Citation2019). Dolan (Citation2001), for instance, found that the introduction of export-oriented green beans into hitherto women-dominated bean farming led to the displacement of women by men as the industry became more commercialized. Consequently, the project specifically set and achieved a target that 75% of the farmers linked to the agro-processor would be women. Thus, the third research question is whether the nutrition outcomes of the project differ between female and male participants.
Study methods
Study area, data and sampling
This study uses primary data collected in the four study districts targeted by the Rwanda Superfoods project. In order to obtain a comparison group, one additional district, namely Burera (just north of Gakenke), was included in the study. Control households were recruited from this fifth district.
The data used were collected in September 2014 by trained enumerators using a pre-tested and validated questionnaire. The pretests were conducted prior to the main survey and were aimed at refining the questions based on local context and improving the fluency of data collection teams in administering the surveys. Data were collected on crop production; input usage; marketing/sales; knowledge, attitudes, and practices; household food security; food consumption by the household and key members including children under 5 years of age and their caregivers; and household asset endowments.
The sampling process proceeded as follows. First, the four project districts and one control district were purposively selected. Second, in each project district, a list of households that benefited directly from the project interventions (i.e. were reached directly by the project staff or project’s implementing partners) was compiled with the help of the project team and a random sample of households drawn using probability proportion to size sampling technique. That is, more respondents were sampled from districts with more households, and vice versa. Third, during the validation of the list of sampled directFootnote2 beneficiary households, each beneficiary was asked to identify households they shared sweetpotato vines with through farmer-to-farmer exchange, and a list of these indirect beneficiaries compiled. A random sample of households generated through this process was also taken and interviewed as “spillovers” households. Lastly, a list of households in the control district (who did not benefit at all from the project) was compiled with the help of project teams and local government administrators and a random sample drawn and interviewed as nonparticipants (i.e. nonbeneficiaries) of the project.
Questions administered to the three categories of respondents (direct beneficiaries, spillovers, and nonparticipants) included screening questions that validated their actual status. This allowed the research team to assign the respondents to the appropriate category. A total of 856 households were sampled and interviewed through this process. However, out of 856 households, 6 reported that they did not grow sweetpotato during the year preceding the survey and were therefore excluded from the analysis, resulting in 846 usable responses. A summary of respondents interviewed in each of the districts (by participation type) is presented in . Overall, 69% of the respondents interviewed in the entire sample were women; 24% were nonparticipants, 39% project participants, and 37% spillover households. The large proportion of women in the sample was due to the specific targeting done by the project and the fact that women are dominant in sweetpotato production in Rwanda.
Table 1. Distribution of sampled households (counts) by districts and gender of the household head
Empirical methods
The sweetpotato project under study specifically targeted poor households with children under 5 years of age, pregnant women, or breastfeeding women, that is, households at the greatest risk of vitamin A deficiency. The Imbaraga Farmers Association supported smallholder market-oriented farmers, hence the farmers they supported were not likely to be the poorest of the poor. YWCA, on the other hand, backstopped vulnerable households, the majority of whom were female-headed. Therefore, participation in the sweetpotato project by farm households was clearly not random. The resulting selection bias is expected to confound the effect of the intervention (i.e. the project) on outcome variables making the coefficients of the simple Ordinary Least Square (OLS) model imprecise. Identifying the relationship between the intervention and the outcome variables, therefore, requires the use of specific techniques that control for the selection bias by accounting for the observed and unobserved characteristics likely to confound the effects of the intervention. In this study, we control for observable and unobservable confounders by using Endogenous Switching Regression (ESR), Propensity Score Matching (PSM), and the Inverse Probability Weight-Adjusted Regression (IPWAR) techniques. We apply these three techniques, in addition to the OLS estimation, to test the robustness of the results and estimates.
The key question we address in this study is how the welfare, in terms of household food consumption and food security, of the women and men-headed project participating households (i.e. direct beneficiaries and spillovers (i.e. indirect beneficiaries)) have changed had they not participated in the project in any way. If denotes the net benefit from participation in the project,
can be expressed in terms of explanatory variables assumed to affect the net benefits as:
where is a vector of variables assumed to determine participation in the project or otherwise,
is a parameter to be estimated and
is the error term in the selection equation,
and
are the expected outcomes when the latent variable Ai occurs or otherwise, respectively;
is a vector of explanatory variables assumed to affect the outcome variables. Variable
include household characteristics (such as sex, age, education, family size), institutional factors (such as distance to market and health facility, access to extension service, trainings).
and
will not be observed simultaneously since the covariance of the error terms
and
is undefined. However, ɛ1i, and
are internally correlated via the first selection equations. Therefore, the decision to participate in the project or not (selection equation) depends on the covariance between
and
.
In the absence of selection bias, the effect of the project on the selected outcome indicators can be measured by including an intervention dummy in an OLS regression model. The coefficient of the dummy measures the program effect. However, with selection bias, the estimated OLS coefficient of the intervention dummy will either under or overestimate the effect of the intervention on the outcome variables because the participation and the outcome variables are strongly correlated.
The ESR model is used to obtain more precise measures of intervention effects on outcome variables in the presence of selection bias. It is specifically applied in the estimation of program effects where unobservable confounders are expected to be present (Alene and Manyong Citation2007; Asfaw et al. Citation2012b; Kleemann & Abdulai, Citation2013; Khone et al. Citation2015; Kanburi Bidzakin et al. Citation2019). It allows for the estimation of the effects of participation in the project on the desired outcome variables, namely, food consumption and food security, after accounting for heterogeneity as outlined in . The row difference measures participation effects when a heterogeneity is controlled while the column differences measure the participation effect on participant households and nonparticipant, under actual and counterfactual conditions. The diagonal elements (a) and (d) represent the expected outcomes in the sample dataset for participant and nonparticipant households, respectively. Similarly, cells (b) and (c) represent the counterfactual outcomes of participation and nonparticipation, respectively. The ESR estimates the effect of adoption in two steps: In the first step decision is made to received treatment or not; hence, a Probit regression model is estimated. In the second step, the relationship between outcome and treatment variables with other set of variables is estimated. The results of the ESR model can, however, be sensitive to assumptions made about the instruments used in the selection equation (Khone et al. Citation2015).
Table 2. The effect of the intervention on expected outcome variables of treated and control households
The PSM technique is, on the other hand, applied in circumstances where selection bias is expected to arise result from observable confounders. It essentially matches participant households to the nonparticipant counterparts that are similar in all aspects, except for the intervention effect; hence, the observed differences in outcomes between the two groups can be attributed to the intervention effect (Ogutu, Okello, and Otieno Citation2014; Wainaina, Okello, and Nzuma Citation2014; Zingiro, Okello, and Guthiga Citation2014). The technique estimates the average treatment effect on the treated (ATT) defined as (Heckman, Ichimura, and Todd Citation1997; Takeshi and Barrett Citation2013):
where and
, denote the outcome variable of household
if it received treatment
, or otherwise
, respectively. As before,
and
cannot be observed simultaneously. Instead, one can only observe the outcome variable of the treated households,
. The PSM technique relies on two key assumptions. The first is the conditional independence assumption where conditional on of being treated, and given a set of observed covariates, an outcome of participation,
, and the probability of participation,
, are assumed to be statistically independent. The second assumption is that of common support and requires strong similarity in the covariates between the participant and nonparticipant households. The participant and nonparticipant households being compared should have a common probability of being in the intervention and nonintervention groups. The PSM has the major weakness of failing to account for unobservable characteristics/confounders and therefore, results may not be robust when the assumption of the absence of unobserved confounders is violated. Its misspecification can lead to biased estimates of ATT (Robins, Sued, Lei-Gomez, & Rotnitzky, Citation2007; Wooldridge, Citation2007).
More recently, the use of inverse probability weight-adjusted regression (IPWAR) and Augmented Inverse Probability Weight-adjusted regression (AIPWAR) techniques to estimate program effects has gained prominence in the literature (Forsman et al. Citation2016; Kebebe and Shibru Citation2017; Nin-Pratt & McBride, Citation2014; Wossen et al. Citation2017). This technique improves the precision of the estimated PSM coefficients by employing probability weighting, where the weights are the estimated inverse probabilities of treatment effects. However, even though IPWAR and AIPWAR can overcome misspecification bias, they do not eliminate the bias resulting from the unobservable variables (or heterogeneity).
Measurement of food consumption score
We use the food consumption score (FCS) to capture the consumption of various foods by the respondent’s households. Following the World Food Program (WFP) guidelines, we measured FCS by grouping the food items consumed by the households into 12 groups and summing up the number of times a food group is consumed per day over the seven days of recall period (World Food Programme (WFP) Citation2018). The food groups are weighted to reflect the nutritional contents of the food groups. Mathematically, Food Consumption Score (FCS) can be computed as follows:
where is the number of days ith food category was consumed by household in the past 7 days and
is the weight assigned to ith food category. The weights assigned are: cereals and tubers (3), pulses (3), vegetables (1), fruits (1), meat and fish (4), milk (4), sugar (0.5), oil (0.5); and condiments (0). Based on this computation, the maximum food consumption score is 112 if the household consumed all the food groups the whole week.
Measurement of food security
Food security is measured in this study using self-reported response to the question: “In the past month (i.e. 30 days), did you have enough food to eat or money to buy food.” The variable takes the value 1 if the household was food insecure, and 0 if otherwise (i.e. the household was food secure).
Results and discussion
Descriptive analysis of sampled household characteristics
presents the results of the analysis by respondent category: nonparticipant, participant, and spillover. Except for household size and the number of female adults, there is no statistically significant difference between male and female respondents among nonparticipant households. For the participant households, results show a significant difference between male and female respondents in terms of education of the head and number of male and female adult members. In this category of households, male respondents were more educated ().
Table 3. Summary statistics of households by gender and project participation
Among the spillover households, females were less endowed with livestock assets (as shown by the lower tropical livestock units ()), more likely to receive remittances, and less educated than their male counterparts. The latter finding suggests that more female caregivers are likely to have spouses that work and live away from home and therefore maintain families through remittances. These remittances are a source of income for the household and can influence food consumption and food insecurity. The results further indicate that female household heads in spillover households were less educated than their male counterparts. Spillover households also had a higher number of female adult members (). Furthermore, the results in revealed a clear relationship between education, food consumption, food insecurity, and wealth status. They also show that the nutrition outcome variables (namely, food consumption score and food security) differ considerably depending on the size of farm owned by households.
Table 4. Comparison of outcomes across the level of formal education of the household head and farm size endowments
Determinants of food consumption and food insecurity after matching
presents the determinants of food consumption and food insecurity based on the results of the propensity score matching estimation used to generate the propensity score for matching project participants to nonparticipants of similar characteristics. We include a variable treatment in the model to test the effect of participation in the project on the outcome variables, namely, food consumption and food insecurity. Food consumption was proxied by food consumption score which ranged from 0 to 112. It is therefore a discrete variable, hence, was estimated using the Tobit regression model. Food insecurity was measured using a binary variable equal to 1 if the household did not have enough food from its own sources or purchases to eat (i.e. food secure), and 0 otherwise. The food insecurity model was therefore estimated using the Logit regression.
Table 5. Determinant food consumption score and food insecurity after matching
Staring with the food consumption model, the results show that participation in the project had a strong significant effect on household food consumption. This finding indicates that participation in the Rwanda Superfoods project increased the consumption of diverse types of food. Results also show that education of the household head, number of varieties of sweetpotato planted, involvement in informal business activities, and livestock endowment all increase the diversity of foods consumed, as measured by food consumption score. The findings regarding the positive effect of the head’s education corroborates the finding in . These findings also indicate that access to own produced foods (captured by the number of sweetpotato varieties grown – a proxy for crop diversification) and livestock ownership and endowment with financial capital (i.e. income from various activities) improve household food consumption. The study however finds a negative effect between age and food consumption. Specifically, the findings suggest that more senior members of the community are less likely to consume a wider range of foods. In the context of this study, the more senior people interviewed were caregivers of children under 5 years of age.
The results of the food insecurity model are presented in the last two columns of . Participation in the project had a negative, but statistically insignificant effect on food insecurity. However, food insecurity is influenced by demographic characteristics, asset ownership, and geographic location. In particular, the education level of the household head, household size, the number of sweetpotato varieties planted (indicator for crop diversification), involvement in informal business activities, and livestock ownership decrease the likelihood of food insecurity. Notably, an increase in household size by one unit increases the likelihood of food insecurity by 0.86 units, other things held constant. The results also show that involvement in business activities (source of income), livestock ownership, and diversification of sweetpotato production reduces the likelihood of an incidence of food insecurity. Moreover, the estimated coefficient of education on food insecurity is negative and statistically significant, indicating that higher levels of education of the household heads reduce the likelihood of food insecurity. It specifically indicates that an increase in years of education by one year reduces food insecurity by 5%, other things held constant. The finding that livestock ownership, a proxy for wealth, affects both food consumption and food insecurity suggests the importance of wealth effects on household welfare.
We included district dummies in both estimated models to control for district-fixed effects. As shown, except for the Muhanga district, all the other district dummies have no effect on both food consumption and food insecurity. For food insecurity, the findings indicate that being in the Muhanga district increases the likelihood of food insecurity by 64%.
Results of endogenous switching regression analysis
The results of the endogenous switching regression (ESR) model estimated to predict the impact of participation in the project on male and female-headed households are presented in . ESR regression provides the estimated effects of the intervention on outcome variables considering the heterogeneity of the study household members. The row differences in measures the effect of the intervention on food security and food consumption scores of households differentiated by the intervention type and gender, and accounting for the heterogeneity effects. The column difference, on the other hand, measures the effects of the intervention on food consumption and food insecurity for participant households under treatment/intervention (i.e. participation) and counterfactual/control households. Similarly, the diagonal elements of the table indicate the cases for the intervention and control households. Thus, A1 and B2 in represent the expected average food insecurity while A3 and B4 represent expected average food consumption by intervention and control households under actual conditions, respectively. Similarly, A2 and B1 present the expected food insecurity of intervention and control under the counterfactual condition. The lowercase a and b represent the actual and counterfactual cases for the female and male-headed households within intervention and control households.
Table 6. Endogenous Switching regression-based average treatment effects on treated and nontreated households
The result in shows that participation in the project improved food consumption and food security. Moreover, the intervention had a more pronounced impact on participant females (reduced food insecurity by 48 percentage point, and increased food consumption score by 25 points) than nonparticipants/control females (where food insecurity could have been reduced by 16% point and food consumption could have been increased by 6.6 points). Similarly, the intervention benefited more male participants (reduced food insecurity by 41 percentage points, and food consumption score increased by 23 points) than male nonparticipants/control (where food insecurity could have reduced by 12% point, and food consumption increased by 9 points). Clearly, these findings indicate that the project had an impact on the welfare of the participating household members in terms of food consumption and reduction in food insecurity.
Comparing the effects of intervention within the participant groups: Female-headed households benefited more in terms of reduction in food insecurity and increase in food consumption compared to their male counterparts. Notably, results indicate that the intervention did not have a significant differential impact on food insecurity and food consumption for male and female in the control. In general, the average food insecurity for the treated groups is 42%, and 39% for the control group, indicating that the control group would have been better off had they received the intervention. However, without intervention, participant households could have been 88% more food insecure. This shows that nonparticipant household members were actually less food insecure compared to the participating household members even without intervention. Further, a comparison of food consumption score for control and intervention households revealed the average effect of the intervention on participant households is 24.26 points while the average treatment effect on control households could have been 7.74 points if they had participated in the project. Thus, project participation accounts for 16.52 of the heterogeneity.
Robustness checks of the results using alternative impact assessment models
presented the results of the analysis of the impact of the project on food consumption and food insecurity on male and female-headed households based on alternative estimation techniques. The first major column of the table presents the effect of the intervention on food insecurity for female-headed beneficiary households using Ordinary least square (OLS), Propensity Score Matching (PSM), and Inverse Probability-Weight Adjusted Regression (IPWAR), Inverse Probability-Weighted Average Regression and Augmented Inverse Probability Weight-adjusted Regression (AIPWAR) techniques.
Table 7. Result robustness check of the effect of the intervention on food insecurity and food consumption
Considering the entire participant group (i.e. both direct beneficiaries and spillovers), the PSM results indicate that participation in the project reduces the likelihood of being food insecure by 25% (p < .000) using OLS and 8% (p < .000) under. This effect was through male participants among whom participation significantly reduced the likelihood of food insecurity by 12%. However, IPWAR and AIPWAR techniques yielded no significant effect of the intervention on food insecurity.
Regarding food consumption, results indicate that the project, overall, significantly increased food consumption score by 3.5 points under OLS, 5.00 points under PSM, and 3.7 both under both APWAR and AIPW. Moreover, the results of the PSM estimation show that the project increased the food consumption score by nearly 5 points. Results further show that the project significantly increased food consumption among both male and female-headed households. Clearly, these findings indicate that the project had a robust and unambiguous (positive) effect on food consumption among targeted households irrespective of the gender of the head. The effect of the project on household food insecurity is however not robust.
Summary, conclusions, and policy implications
This study examined the impact of a project-based intervention that promoted the production of biofortified orange-fleshed sweetpotato by smallholder farmers for commercial processing in Rwanda, focusing on food consumption and food insecurity of men and women-headed household participants. It uses data collected from 846 households stratified by intervention type (i.e. participant versus nonparticipant) and gender of the head (men versus women) and different regression techniques to assess the impact of the intervention on food consumption and food insecurity and the robustness of findings.
The study finds that the intervention increased food consumption of both men and women-headed households. The improvement in food consumption is robust across the different estimation techniques used. This implies that the Rwanda Superfoods project unambiguously resulted in significantly higher food consumption among participant households. In addition, the magnitude of the impact is higher for women-headed households than their men-headed counterparts. The results also indicate that intervention households could have consumed less food groups had they not participated in the intervention. Further, the nonparticipants could have significantly benefitted from the project intervention had they participated, but the magnitude of the effect on nonparticipants would have been lower. Evidence of the impact of the intervention on household food insecurity was similarly unambiguous, but not robust. While the ESR and PSM techniques found a moderate effect, IPWAR and AIPWAR techniques found no effect on food insecurity. In general, the findings indicate that there is evidence that the project did reduce the incidence of food insecurity among participant households, despite the evidence not being robust.
Overall, the study concludes that the Rwanda Superfoods project improved the welfare of households by increasing food consumption. It also concludes that there is evidence, albeit nonrobust, that the project improved household food security status. Moreover, it concludes that female-headed households benefitted much more from the intervention than their male-headed counterparts in terms of improvement in food consumption. This finding is likely to be due to the project design which intentionally targeted women. Nonetheless, the significant and higher impact of the project on food consumption could be related to the fact that women tend to undertake most of the food preparation activities in the household, hence are likely to apply what they learn in the household’s food provisioning. The second key finding of this study is that significant improvement was seen in food consumption and food security at the household level without an intensive nutrition education component. As noted earlier, the focus was on market development, with just minimal information provided on the nutritional benefits of orange-fleshed sweetpotato.
The finding that the project improved food consumption for female-headed households more than their male-headed households implies that projects that are designed with gender in mind are likely to benefit female-headed households much more. This is especially important in cases where control of the crop is likely to be taken over by men as the value chain becomes commercialized, as in the case of sweetpotato. Consequently, market-focused projects should be designed with gender considerations in mind. The findings also imply that interventions that aim to link rural farm households to commercial value chains can actually improve their welfare, especially household nutrition outcomes.
This study has two limitations. First, the findings relate to relatively smaller regions of Rwanda, and hence are context specific. Moreover, because the project targeted vulnerable households in communities with a higher incidence of malnutrition, the study findings should be interpreted with these design aspects in mind. Second, our measure of food insecurity relies on a narrow qualitative and static proxy of household food availability assessment by the respondent. The assessment of food security, therefore, applies only to the time of the year and season the study was conducted.
Additional information
Funding
Notes
2. A direct beneficiary is a household that received the intervention (e.g. OFSP vines) directly from the project or its implementing partners. By comparison, indirect beneficiary household (also called spillovers) received the intervention through farmer-to-farmer exchange.
References
- Alary, V., C. Corniaux, and D. Gautier. 2011. Livestock’s Contribution to Poverty Alleviation: How to Measure It? World development 39:1638–48. doi:10.1016/j.worlddev.2011.02.008.
- Alene, A. D., and V. M. Manyong. 2007. The effects of education on agricultural productivity under traditional and improved technology in northern Nigeria: An endogenous switching regression analysis. Empirical economics 32 (1):141–59. doi:10.1007/s00181-006-0076-3.
- Alkire, S., R. Meinzen-Dick, A. Peterman, A. Quisumbing, G. Seymour, and A. Vaz. 2013. The Women’s Empowerment in Agriculture Index. World development 52:71–91.
- Asfaw, S., B. Shiferaw, F. Simtowe, and L. Lipper. 2012b. Impact of modern agricultural technologies on smallholder welfare: Evidence from Tanzania and Ethiopia. Food Policy 37:283–95. doi:10.1016/j.foodpol.2012.02.013.
- Asfaw, S., M. Kassie, F. Simtowe, and L. Lipper. 2012a. Poverty reduction effects of agricultural technology adoption: A micro-evidence from rural Tanzania. Journal of Development Studies 48 (9):1288–305. doi:10.1080/00220388.2012.671475.
- Daroque, M. B., and R. Gasson. 1991. Changing Gender Relations in Agriculture: An International Perspective. Journal of rural studies 7.
- Dolan, C. 2001. The’good wife’: Struggles over resources in the Kenyan horticultural sector. Journal of Development Studies 37 (3):39–70. doi:10.1080/00220380412331321961.
- FAO. 2013. Country Programming Framework for Rwanda: 2013–2018. http://www.fao.org/3/a-bp633e.pdf
- Farnworth, C. R., Jafry, T., Lama, K., Nepali, S. C., and Badstue, L. B. 2019. From Working in the Wheat Field to Managing Wheat: Women Innovators in Nepal. The European Journal of Development Research, 31 (2):293–313.
- Feleke, S. T., R. L. Kilmer, and C. H. Gladwin. 2005. Determinants of food security in Southern Ethiopia at the household level. Agricultural Economics 33 (3):351–63. doi:10.1111/j.1574-0864.2005.00074.x.
- Forsman, H., L. Brännström, B. Vinnerljung, and A. Hjern. 2016. Does poor school performance cause later psychosocial problems among children in foster care? Evidence from national longitudinal registry data. Child abuse & neglect 57:61–71. doi:10.1016/j.chiabu.2016.06.006.
- Galie, A., J. Jiggins, and P. C. Struik. 2013. Women’s identity as farmers: A case study from ten households in Syria. NJAS - Wageningen Journal of Life Sciences 64–65:25–33. doi:10.1016/j.njas.2012.10.001.
- Heckman, J. J., H. Ichimura, and P. E. Todd. 1997. Matching As An Econometric Evaluation Estimator: Evidence from Evaluating a Job Training Programme. The Review of economic studies 64:605–54. doi:10.2307/2971733.
- Kanburi Bidzakin, J., S. C. Fialor, D. Awunyo-Vitor, I. Yahaya, and G. Aye. 2019. Impact of contract farming on rice farm performance: Endogenous switching regression. Cogent Economics & Finance 7 (1):1618229. doi:10.1080/23322039.2019.1618229.
- Katungi, E., C. Kabungo, and C. Mukankusi. 2019. Impact of improved bean adoption on commercialization in Southern Tanzania: Why women are excluded? Invited paper presented at the 6th African Conference of Agricultural Economists, September 23- 26, 2019, Abuja, NigeriaKASSALI, R. 2011. Economics of Sweet Potato Production. International Journal of Vegetable Science 17:313–21.
- Kebebe, E., and F. Shibru. 2017. Impact of alternative livelihood interventions on household welfare: Evidence from rural Ethiopia. Forest Policy and Economics 75:67–72. doi:10.1016/j.forpol.2016.12.001.
- Khone, M., J. Manda, A. D. Alene, and M. Kassie. 2015. Analysis of Adoption and Impacts of Improved Maize Varieties in Eastern Zambia. World development 66:695–706. doi:10.1016/j.worlddev.2014.09.008.
- Kleemann, L., and Abdulai, A. 2013. The impact of trade and economic growth on the environment: Revisiting the cross‐country evidence. Journal of International Development 25 (2):180–205 doi:10.1002/jid.v25.2
- Kokeye, S. E. H., J. A. Yabi, S. D. Tovigna, R. N. Yegbemey, and E. A. Nuppenau. 2013. Simultaneous modelling of the determinants of the partial inputs productivity in the municipality of Banikoara, Northern Benin. Agricultural systems 122:53–59. doi:10.1016/j.agsy.2013.08.002.
- Labintan, A. C., and Ding, S. 2012. An assessment of agricultural productivity and major driving factors in the republic of Benin. 5 (4):470–476.
- Low, J. W., Mwanga, R. O., Andrade, M., Carey, E., and Ball, A-M. 2017. Tackling vitamin A deficiency with biofortified sweetpotato in sub-Saharan Africa. Global food security 14: 23–30.
- Mudege, N. N., T. Chevo, T. Nyekanyeka, E. Kapalasa, and P. Demo. 2016. Gender norms and access to extension services and training among potato farmers in Dedza and Ntcheu in Malawi. The Journal of Agricultural Education and Extension 22 (3):291–305.
- Muhinda, J.J. Mbonigaba. 2013. Rwanda Agricultural Sector and Its Impact on Food Security and Economy Workshop on Asian Lessons and Agriculture Transformation in Rwanda Kigali, 31 Jan 2013. Kigali. https://docplayer.net/20628972-Rwanda-agricultural-sector-and-its-impact-on-food-security-and-economy.html
- Mutiso, J. M., J. J. Okello, C. J. Lagerkvist, P. Muoki, W. O. Kosura, and S. Heck. 2018. Effect of nutrition education and psychosocial factors on child feeding practices: Findings of a field experiment with biofortified foods and different women categories. Ecology of food and nutrition 57 (4):346–71. doi:10.1080/03670244.2018.1492382.
- National Institute of Statistics of Rwanda (NISR). 2015. Seasonal Agricultural Survey 2013: Version 2. file:///E:/DOCUMENTS/Downloads/SAS_2013_Report_Version-2.pdf
- Nin-Pratt, A., and McBride, L. 2014. Agricultural intensification in Ghana: Evaluating the optimist’s case for a Green Revolution. Food Policy 48: 153–167.
- Nyabaro, V., J. Mburu, and M. Hutchinson. 2019. Factors enabling the participation of women in income sharing among banana (musa spp.) producing households in South Imenti, Meru County, Kenya. Gender, technology and development 23 (3):277–92. doi:10.1080/09718524.2019.1669104.
- Ochieng, J., B. Knerr, G. Owuor, and E. Ouma. 2019. Food crops commercialization and household livelihoods: Evidence from rural regions in Central Africa. Agribusiness 1–21. doi:10.1002/agr.21619.
- Ogutu, S. O., J. J. Okello, and D. J. Otieno. 2014. Impact of information and communication technology-based market information services on smallholder farm input use and productivity: The case of Kenya. World development 64:311–21. doi:10.1016/j.worlddev.2014.06.011.
- Okello, J. J., N. Kwikiriza, P. Muoki, J. Wambaya, and S. Heck. 2019. Effect of Intensive Agriculture-Nutrition Education and Extension Program Adoption and Diffusion of Biofortified Crops. Journal of Agricultural & Food Information 1–23.
- Okonya, J. S., W. Ocimati, A. Nduwayezu, D. Kantungeko, N. Niko, G. Blomme, J. Kroschel, and J. Kroschel. 2019. Farmer reported pest and disease impacts on root, tuber, and banana crops and livelihoods in Rwanda and Burundi. Sustainability 11 (6):1592. doi:10.3390/su11061592.
- Robins, J., Sued, M., Lei-Gomez, Q., and Rotnitzky, A. 2007. Comment: Performance of Double-Robust Estimators When “Inverse Probability” Weights Are Highly Variable. Statistical Science 22 (4): 544–559.
- Shikuku, K. M., J. J. Okello, S. Wambugu, K. Sindi, J. W. Low, and M. McEwan. 2019. Nutrition and food security impacts of quality seeds of biofortified orange-fleshed sweetpotato: Quasi-experimental evidence from Tanzania. World development 124:104646. doi:10.1016/j.worlddev.2019.104646.
- Takeshi, K., and C. B. Barrett 2013. The system of rice intensification and its impacts on household income and child schooling: Evidence from rural Indonesia. American.
- Wainaina, P. W., J. J. Okello, and J. M. Nzuma. 2014. Blessing or evil? Contract farming, smallholder poultry production and household welfare in Kenya. Quarterly Journal of International Agriculture 53 (4):319–40.
- Wooldridge, J. M. 2007. Inverse probability weighted estimation for general missing data problems. Journal of Econometrics 141(2):1281–1301.
- World Bank. 2012. The world development report 2012: Gender equality and development. Washington DC: The World Bank.
- World Food Program (WFP). 2018. Rwanda: Comprehensive Food Security and Vulnerability Analysis 2018. Via C.G. Viola 68, Parco dei Medici, 00148, Rome, Italy. http://www.wfp.org/food-security and www.statistics.gov.rw
- Wossen, T., T. Abdoulaye, A. Alene, M. G. Haile, S. Feleke, A. Olanrewaju, and V. Manyong. 2017. Impacts of extension access and cooperative membership on technology adoption and household welfare. Journal of rural studies 54:223–33. doi:10.1016/j.jrurstud.2017.06.022.
- Zingiro, A., J. J. Okello, and P. M. Guthiga. 2014. Assessment of adoption and impact of rainwater harvesting technologies on rural farm household income: The case of rainwater harvesting ponds in Rwanda. Environment, development and sustainability 16 (6):1281–98. doi:10.1007/s10668-014-9527-8.