Abstract
Unfamiliarity with the economic structure of the game ranching sector is regarded as one of the reasons why questions are raised with regard to the potential economic impact of continued growth in the sector. This study employs structural path analysis to provide a better understanding and subsequently improves the ability to conceptualise the potential economic impact of the sector. Amongst others, results revealed strong economic links between the community, social and personal, chemicals and chemical products, manufacturing and equipment, transport equipment, business services sector, and so forth, either directly or indirectly, and the game ranching sector. The same accounts for income distribution, with households receiving income from production activities in other sectors that are directly or indirectly generated by stimulus within the game ranching sector. Finally, results underline the fact that game ranching has the potential for making a meaningful contribution towards economic and socioeconomic challenges in South Africa.
1. Introduction
Changes with regard to game ranching in South Africa started in the late 1960s. Up until the early 1960s it was accepted wisdom that wild animals and domestic livestock needed to be separated because they compete with each other for grazing and because transmittable wildlife diseases threaten the health of livestock. It was thus assumed that in order for modern agricultural to prosper, game should be exterminated to make way for livestock. Agricultural and game departments acted in accordance to this belief for the biggest part of the early twentieth century (Carruthers, Citation2008).
Following the pre-1960s wisdom, a process began that returned the rights over wildlife to private land owners, and which at the same time increased its commercial value. Some people regard this period as ‘one of the great agricultural transformations in history, comparable to the adoption of maize and cattle’ (Nell, Citation2003:96; Jarrell, Citation2003:324). Others described it as ‘a conservation revolution in South Africa’ (Bothma et al., Citation2009). However, the actual expansion of the industry only took effect in the early 1990s with the granting of conditional ownership of game animals to private land owners. Eloff (Citation2002) reports that the since the granting of private ownership in the early 1990s, the game industry has grown at an average annual rate of 5.6% in terms of area exempted for game ranching. This growth rate was predominantly spurred by economic and ecological benefits from common/plain game species, with many farmers switching from livestock to game ranching (Dekker, 1998, as cited by Van der Waal & Dekker, Citation2000). However, an increase in intensive breeding practices, especially of higher value animals since the mid-2000s, resulted in a further acceleration to a growth rate of 6.75% per annum (Cloete, Citation2012). As a result, today, an estimated 10 000 game ranchers utilised more than 20 million hectares for ranching activities (Cousins et al., Citation2010; Dry Citation2010, Citation2013). This makes the game ranching sector one of the fastest growing agricultural sectors in South Africa, resting on several consumable and non-consumable product pillars.
Although the evolution of the South African game ranching industry in terms of conservation and development is well documented, the opposite is true in terms of its size and economic contribution. Child et al. (Citation2012:16) are of a similar view, arguing that the shift from livestock to wildlife and, to an extent, the growth in the game ranching industry, ‘is well documented but not reliably quantified’. Moreover, a significant number of discrepancies exist in the literature as far as the size and/or economic contribution of the game industry goes. This discrepancy is evident from, amongst others, the studies by Du Toit (Citation2007), Cousins et al. (Citation2008, 2010), Dry (Citation2010), Dlamini & Fraser (Citation2010), Child et al. (Citation2012). Although different results are reported in terms of the economic size and/or contribution of the industry, all of these studies suggest that the South African game ranching sector has a notable monetary size and, more specifically, makes a meaningful contribution towards national imperatives such as economic growth, employment creation, poverty reduction and, subsequently, food security.
Nonetheless, questions still remain with regard to the impact of continued growth in the game ranching sector on national imperatives. This is evident from statements such as that made by the Department of Environmental Affairs and Tourism (Citation2005), which is concerned that the level of transformation of previously disadvantage people in certain spheres of the game industry is hampering rather than contributing towards achieving national imperatives.
Booth (Citation2010) suggests that unfamiliarity with the economic structure of the wildlife ranching sector contributes to questions being raised. In other words, people do not understand the way in which a stimulus in the wildlife ranching sector impacts on the rest of the economy. This unfamiliarity could be captured through an in-depth analysis of the entire network of paths through which structural relationships in an economy lead to their ultimate affects (i.e. mapping the network of transmission channels between wildlife and other sectors).
Therefore, the aim of this study is to shed light on the paths and linkages (mechanisms) through which a stimulus in the wildlife ranching sector will influence the rest of the economy by making use of structural path analysis (SPA). Expectantly, this will provide a better understanding of the economic structure and subsequently improve the ability to conceptualise the contribution or potential contribution of the sector.
The following sections provide a detailed discussion on the methodological approach and data used, followed by results, discussion and, finally, concluding remarks.
2. Methodology and data used
2.1 Structural path analysis method
The SPA method of decomposing multipliers from a social account matrix (SAM) of South Africa, compiled by Conningarth Economists (Citation2006) and made available through the Development Bank of Southern Africa, will be applied. The first step involves the calculation of accounting multipliers, which are reflected by the Leontief inverse matrix. These multipliers can be used to identify key sectors within an economy, particularly those that have the potential for generating high demand-led multiplier effects (Ngandu et al., Citation2010). Following the calculation and decomposition of the accounting multipliers, SPA can be conducted.
SPA focuses on how individual elements lead to the global influence we obtain from conventional SAM-based multipliers by tracing the transmission of influence within an economic structure and thus connecting the spider web and the relative strength of its connections. It is a means of identifying the paths through which structural relationships in an economy lead to ultimate effects on endogenous variables, and reveals aspects of an economy that are not apparent from an examination of either direct transactions between accounts or an examination of the global influence that is the solution of conventional decompositions (Roberts, Citation2005). SPA aims to identify the most important interactions or paths within an economic system and ultimately to identify which individual poles (sectors, factors or households) are important transmitters of economic influence (Ngandu et al., Citation2010).
Accordingly, SPA was designed to provide a more detailed picture of the effects of shocks to exogenous accounts. SAM multipliers measure the cumulative effects from a shock, whereas SPA not only decomposes these multipliers into direct and indirect components but also reveals the network of transmission channels. The SPA decomposition is, in this context, useful in coming to grips with the nature and strength of linkages that work through the economic sector.
In order for the results of multipliers and SPA to strictly apply to the South African economy, two important assumptions must hold simultaneously. First, supply-side constraints to economic expansion are not binding. As a result, the level of demand determines the level of economic activity. Second, one must either assume that prices are constant or that preferences and technology are of the Leontief form. In other words, consumers consume in fixed proportions and producers use inputs in fixed proportions either because fixed relative prices provide no incentives to change those proportions or because preferences and technology are specified in that way.
Ordinarily, these assumptions are considered highly difficult. Multiplier analysis is often viewed as providing useful insights into demand forces on the economy that may be important for the nature and rate of economic growth. However, the interpretation of results is usually tempered by a more realistic view of supply side, prices, preferences, and technology. However, in the context of the past and more recent economic downturn, these assumptions become more plausible. With an economic contraction, supply-side constraints are clearly less problematic. At the same time, while prices, preferences, and technology almost surely maintain some flexibility, fixed proportion preferences and technology provide a valuable first-order approximation to the effects of demand shifts within the South African economy (Ngandu et al., Citation2010).
The value of SPA depends crucially upon whether the aforementioned underlying assumptions are met. There are, however, circumstances when they are not. If the economy is constrained or faces bottlenecks in any sector, in the supply of goods or services, or in key factors of production, then the SPA needs to be viewed with caution. Also, SPA results are only useful in examining the real side effects of quantity-based shocks; they are not so useful at handling price shocks or ascertaining price effects (Round, Citation2003). However, despite such limitations, one should keep in mind that the main benefit of SPA is its simplicity and transparency.
2.2 Data used
This paper uses a 2006 SAM for South Africa. Multiplier analysis in a SAM framework permits incorporation of feedback effects from household consumption. In addition, the detailed representation of households present in the 2006 SAM for South Africa allows one to consider the distributional impacts of various types of demand injections.
The 2006 SAM includes a total of 209 accounts, which can be divided into endogenous and exogenous accounts. The endogenous accounts include 48 activities, 48 commodities, 48 factors (labour and capital), and 52 institutions (enterprises and households). The exogenous accounts include five types of taxes and subsidies, three levels of government (national, provincial and local), two capital accounts (government, corporate sector and households), savings–investment balance and the rest of the world. In a multiplier decomposition, as proposed by Pyatt & Round (Citation1979) and Defourny & Thorbecke (Citation1984), the effect of injections from exogenous accounts can be traced in the economy through the endogenous accounts. Under the assumptions mentioned above (fixed prices and no supply restrictions), multiplier analysis and SPA can reveal the interaction between and across production activities, factors of production, and institutions.
In performing the SPA, data from the SAM relating to both skills levels and income groups have been aggregated according to the following schemes in order to provide a more condensed view of the results. The 44 labour skills/occupation categories were aggregated to represent three major groups: low-skilled or unskilled (LABLS – elementary occupations, domestic workers), semi-skilled (LABSK – clerks, service workers, skilled agricultural workers, craft workers, plant and machine operators), and high-skilled or skilled workers (LABHI – legislators, professionals, technicians). Also, while data for all 48 household categories are reflected in the SAM, the data for the purposes of this discussion have been summarised and presented for three household expenditure categories (in terms of percentiles as shown in Table A1 in Appendix A) across all population groups. The aggregation was performed on the following basis: low-income households (HHDLOW – percentiles P1 and P2), middle-income households (HHDMID – percentiles P3 and P8), and high-income or wealthy households (HHDWHI – percentiles P9 and P12).
3. Results
3.1 Multiplier decomposition analysis
This section will start with the conventional multiplier decomposition analysis as suggested by Pyatt & Round (Citation1979) and Defourny & Thorbecke (Citation1984), which generates the multipliers in a non-transparent way, producing the foundation for SPA that exposes the network of channels through which economic influence flows in the economy. The first step is to calculate the accounting multipliers (see ), which measure the global effect of an exogenous increase in the demand of a sector.
Table 1: Selected accounting multipliersa,b
When game ranching is stimulated via, for example, growth (transition of land previously used for livestock to game), demand for intermediate inputs used by game ranching expands. The additional intermediate inputs are reflected by the shaded row in . For example, assuming the game ranching sector expands with a hypothetical R1.1 billion, which is more or less similar to the expansion experienced between 2007 and 2009 (Du Toit, Citation2007; Dry Citation2010), the accounting multiplier for the chemical and chemical product (Chem) sub-sector (0.31) indicates that sales in the specific sub-sector are likely to increase with an estimated R341 million. Analogous, the additional chemicals and chemical products required are either produced domestically, imported or a combination of the two. While the share of domestic purchases will remain in the system, the financial flows to imports and subsequently to taxes are examples of flows to exogenous accounts that are ‘leakages’ from the system. The increase in demand for chemicals and chemical products, whether produced locally or imported, will results in ‘further stimulates’ in sectors intermediate to the Chem sub-sector.
From the above, an expansion of the game ranching sector appears to have some economic benefits for the local economy. However, these positive economic impacts need to be carefully weighed against the opportunity cost of land use. For example, an expansion of the game ranching sector may require a decline in livestock or agricultural production, which in turn may adversely affect other (linked) sectors. The development of game ranching should therefore be carefully planned, with due attention taken for possible negative spillover effects and trade-offs.
At the same time, expansion of the game ranching sector requires increased use of factors of production (i.e. labour and capital). These factor earnings are passed to households, although some of the incremental income leaks to factor taxes, corporate taxes, and retained earnings. For example, the total production cost (factor usages) of the game ranching sector accounts for an estimated 43% of the total input cost (calculated from the SAM database). However, the stimulus to factors amounts to 1.82Footnote3 once the direct and indirect effects are accounted for. In other words, an additional 82 cents worth of inputs (added value to input supplying sectors) will be required for every R1 of additional output in the game ranching sector. However, the spillover to households is less at 0.83 (83 cents for every R1 increase in production in the input supplying sectors) due to the leakages mentioned above; that is, factor taxes, corporate taxes and retained earnings.
Considering earnings in the game ranching sector itself, the sector is reasonably capital intensive with a share of capital in value of 31%. With this share greater than the economy-wide average capital intensity of 24%, the share also implies that significant payments are directed to capital. Secondly, payments to capital are subject to corporate and factor taxes as well as retained earnings. These leakages represent nearly 50% of capital income (calculated from the SAM database). In other words, income received by households in the game ranching sector is more from interest on capital than from salaries and wages for labour. This is also evident in the distribution of additional income to the different enterprises/households with lower-income households that benefit from a 0.04 stimulus compared with the 0.78 for higher-income households (Table A2 in Appendix A). In other words, lower-income households will only receive an estimated 4 cents of the additional 83 cents worth of income. Although this is not a favourable distribution, considering the poor, only a few sectors are likely to provide a larger stimulus to lower-income households due to the ‘features’Footnote4 of the South African economy.
3.2 SPA results
This section presents the results of the SPA, which goes a step further than the multiplier decomposition presented in the previous section. The previous multiplier decomposition gave us the macro influences that exist between the wildlife sector and other poles within the economy. Using SPA, one can now proceed to identify various paths, through which economic influence is transmitted from one sector, in this case from the game ranching sector (the sector of origin), to other sectors in the economy. These destination sectors have been chosen on account of: their close relationship with the game ranching sector as sources of input; the strength of the path associated with its contribution to the total multiplier effect of a shock; the percentage of the multiplier carried along the most important path (i.e. the concentration of transmission channels) being higher for the chosen sectors; and the machinery and equipment (M&E), and the business services (BusServ) sectors displaying a structure with longer (more indirect) paths that are more important than in the transport (Trnsp) and finance and insurance (Fin) sectors. The results for the SPA in to only show up to the 10 most important paths in each case. These are not exhaustive since, in any given SAM, there are thousands of possible paths.
Table 2: SPA for selected activities
Table 3: SPA for factors
Table 4: SPA for institutions
3.2.1 Production activities
shows the path analysis of game ranching on other production activities. For Case I, shows the impact of an injection in game ranching on the Chem sector. From column 3, Case I – an injection of R1.1 billion into the game ranching sector – generates an additional R341 million worth of sales in the Chem sector. SPA reveals that approximately 52.6% (column 8) of this additional production is caused directly by the demand for chemicals and chemical product inputs by the game ranching sector through the path linking the two sectors. The other paths shown under Case I in reveal that a significantly smaller part of the influence of game ranching on the Chem sector is exercised indirectly through the demand for transport (5.5%) of chemicals and chemical products, 2.6% from owners of capital inputs (HHDWHI – high-income households) from public business enterprises (PBE) in the Chem sector, and so forth.
Case III in is interesting and demonstrates that sometimes indirect paths can transmit a significant influence compared with the direct paths between two sectors. For example, 11.6% of the influence is actually transmitted by an indirect path via the owners of capital inputs (HHDWHI – high-income households) that reinvest their gains in the manufacturing of transport equipment (MoTE) sector. Households emerge to be an important transmitter of influence in this instance.
3.2.2 Factors
From , the influence of a stimulus in the game ranching sector on factors (capital and labour) can be seen. With an accounting capital multiplier of 0.75 (column 3, Cases I and II), an estimated R825 million worth of capital would have been required for the game ranching industry to expand with a hypothetical R1.1 billion. Of the R825 million, 76% would have been accounted for by the game ranching sector; that is, 26% from public enterprises (GOS – PE) and 49.9% from role players within the public business enterprise (GOS – PBE) segment. The rest of the influence is transmitted indirectly through paths that flow past other production activities with links to Trnsp, community, social and personal services (CS&PS), and trade (Trade), amongst others (Cases I and II, ).
Case III in shows the influence creation of additional employment in the low-skilled workers (LABLS) segment. The results show that 44% of all additional employment will come directly from the game ranching sector itself and the rest from other sectors, with community, CS&PS (7.7%), Trade (3.8%) and Trnsp sectors being some of the main contributors to the increase in employment. In other words, community, and CS&PS, besides the game ranching sector itself, play the most important role in transmitting the economic influence of changes in the game ranching sector to LABLS segment.
With respect to skilled (LABSK) and highly skilled (LABHI) labour, the CS&PS sector stands out (Case V in ); that is, of all the additional LABSK and LABHI employment opportunities created by a stimulus in the game ranching sector, 20.7% will be accounted for in the CS&PS sector compared with the 17.2% in the game ranching sector itself. Beyond these two sectors, the sources of additional employment for LABSK and LABHI labour seem to be evenly distributed without any one sector dominating as an important transmitter of influence.
3.2.3 Institutions
Lastly, path analysis can also give us a better sense of the distribution of incremental income for institutions (households and enterprises). Cases III, IV and V in show us that the income of all households comes from very few sectors. Moreover, incremental income of middle-income households (HHDMID) mainly comes from three sources, namely the CS&PS, Trade and Chem sectors. This is revealed by the fact that these sectors appear an equal number of times in the paths that connect game ranching and HHDMID. Path analysis also shows that PBE receive most of their income through the direct path via capital (Case II in ), which explains 49.9% of the global influence. The remaining share comes indirectly through capital but initially going from game to CS&PS (5.5%), Trade (3.2%), Trnsp (1.7%), Fin (1.6%), and Chem with 1.3%.
HHDLOW (Case III in ) receive 8.5% of the income generated through LABLS and 7.2% from LABSK. They also receive 20.8% of the income generated by game ranching, through capital invested in GOS – PE. HHDMID (Case IV in ) followed a similar pattern, but with a more even distribution among the paths explained above, where the income coming from LABLS labour represents 5.9% of the multiplier. Lastly, HHDWHI (Case V in ) receive 21.3% of their income through GOS – PBE, followed by 8.3% from LABHI. Production activities that consistently participate in the paths of income generation for enterprises and households are CS&PS, Trade, Trnsp, Fin, and Chem. These results show that South African households have relatively strong links to the services sector, and that many of the sectors involved in wildlife ranching hire labour with different sets of skills.
3.2.4 A graphical representation
The analyses and results discussion presented above relied only on the quantitative results generated by SPA, which are not as straightforward to visualise. As SPA is a construct of relationships within the economy, a graphical representation allows for a potentially more straightforward interpretation of this construct. Accordingly, to showcase the 10 most important paths based on the SPA results in the previous section. The thickness of the lines connecting the accounts represents the strength of the connection between the corresponding accounts: the thicker the line, the greater the relative volume of flow along that path. It is, however, important to note that the thickness is not related to income magnitudes. Rather, the importance of the path relates to both its influence and the path multiplier of adjacent circuits.
Figure 1: Wildlife – Structural path to all institutions

portrays the movement of the influence generated by a stimulus (growth) in the game ranching sector. The three sectors below game ranching are sectors with paths connected to more than one factor. For example, CS&PS uses labour at all skill levels (together with game), while Trade mainly uses LABSK and LABHI. LABLS labour receives income from two out of the three production activities, and generates income for all households. LABSK labour is similarly benefited, whereas LABHI labour is the least connected factor. It is also interesting to note that all household income groups benefit significantly through capital gains via PBE (connected to the game ranching sector), and to a lesser extent from capital gains from Trnsp activity (supplied to the game ranching sector) via public enterprises (PE).
To better understand the distributional effects caused by a stimulus in the game ranching sector, we generate three sets of figures (showing up to four arcs) with corresponding effects on low-income (HHDLOW, ), middle-income (HHDMID, ), and high-income (HHDWHI) households (). Different from what was expected, HHDLOW () receive the bulk of their income from PBE. Nonetheless, they also benefit from strong ties with both LABLS and LABSK workers. The LABLS and LABSK labour in HHDLOW obtained their incremental income primarily from the game ranching sector and to a lesser extent from Trade and CS&PS.
Figure 2: Wildlife – Structural path to lower-income households
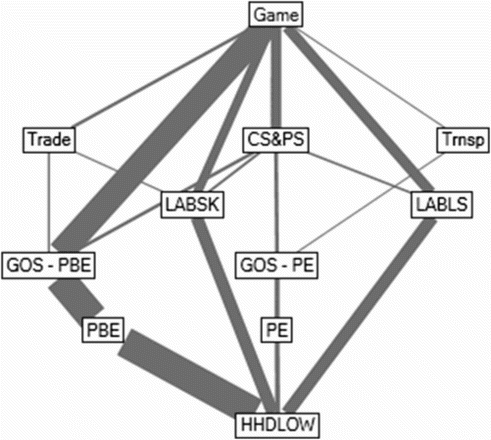
Figure 3: Wildlife – Structural path to middle-income households

Figure 4: Wildlife – Structural path to higher-income households
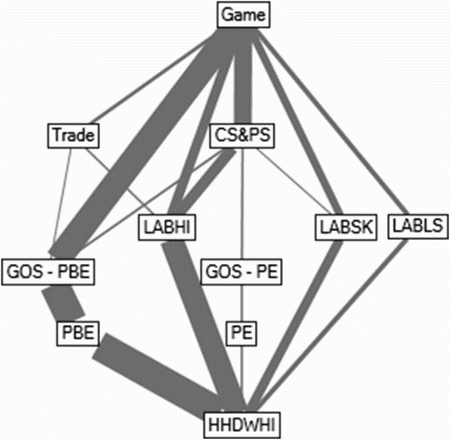
In the case of HHDMID (), there was a large flow between game ranching and PBE. Invested capital in PBE, as well as the use of LABSK workers in the sector, created a huge amount of income activity with this household. Only two production activities are present in the top 10 paths, with CS&PS being the most connected sector. This household enjoyed a more evenly distributed influence from all factors.
showcases how income travels to HHDWHI. This household is highly connected to PBE, CS&PS and LABHI labour (both directly and indirectly through CS&PS). The structure of this case resembles that observed by HHDMID, but with the CS&PS sector having greater importance. Enterprises in this case also receive income from all three activities present, including game, Trade, and CS&PS.
The results from the SPA show that an investment that generates income in the game ranching sector generates movement in a limited number of production activities, factors and institutions. The CS&PS sector appears to be an important factor in this economy, together with the Trade sector (which includes retail and wholesale trade activities). Income received by HHDLOW households comes from a more diverse set of activities, and all household income groups received major capital rents from a smaller set of activities, which is thereafter invested in enterprises.
4. Conclusions
Results from the multiplier decomposition revealed that a stimulus in the wildlife ranching sector has a significant impact throughout various other sectors of the South African economy. Foremost among these are the notable expansions in the monetary size of the community, social and personal services, chemicals and chemical products, financial and transport sub-sectors. For instance, 83 cents worth of additional inputs will be required from the input supply sectors for every R1 of additional output in the wildlife ranching sector. The leakages and distribution of benefits resulting from the additional production across households were not as favourable, with lower-income households receiving only a small percentage of the benefit compared with middle-income or upper-income households. However, this is more a featureFootnote5 of the South African economy than of the wildlife sector, with relatively few of the other agricultural sectors (as substitutes for land use) providing a greater stimulus to overall household income than wildlife ranching and even fewer providing a larger stimulus to lower-income households.
In addition, the paper applied SPA to decompose the cumulative effects as summarised above; that is, to identify the various paths through which economic influence is transmitted from one sector to other sectors in the economy. Results revealed that the strongest links exist between wildlife ranching, the community, social and personal services, chemicals and chemical product, manufacturing and equipment, manufacturing and transport equipment, and business services sectors. For instance, the impact of a hypothetical R1.1 billion expansion in the wildlife ranching sector, which is similar to the expansion from 2007 to 2009, ranges from an R88 million increase in the economic value/size of the manufacturing and equipment sectors to R430 million in the community, social and personal services sectors. The increase in the value of these sectors is due to the direct and indirect demand created by the stimulus in the wildlife ranching sector. For instance, 55.4% of the additional R88 million worth of sale in the manufacturing and equipment sector will be accounted for by the direct demand for equipment, while a smaller part (6.5%) is due to the additional demand for chemicals and chemical products used in the manufacturing process.
Results also revealed that indirect paths can transmit a significant influence compared with the direct paths between two sectors, as is the case between the wildlife ranching sector and the manufacturing of transport equipment; that is, 11.6% of the total influence is transmitted via the owners of capital inputs, mainly wealthy or high-income households that reinvest their gains in the manufacturing of transport equipment sector.
At the same time, a significant influence is reported in terms of factor usages (capital and labour). In terms of capital, 75 cents (global influence = 0.75) of capital is required for every R1 worth of additional output produced in the wildlife ranching sector. The majority of this will be accounted for by the wildlife ranching sector itself (76%); that is, 26% of the capital investments will be accounted for by public enterprises, with the remaining 50% by public business enterprises within the sector.
Although the wildlife ranching sector is responsible for most of the additional low-skilled employment opportunities created, results revealed significant indirect effects with other sectors such as community, social and personal services, trade and transport, and so forth also being able to create additional low-skilled employment opportunities due to the original stimulus in the wildlife ranching sector. On the contrary, of all the additional skilled and highly skilled employment opportunities that will be created, community, social and personal services will account for the majority (20.7%), followed by the wildlife ranching sector with 17.2%.
Moreover, results revealed that income generated in the wildlife ranching sector, either through capital gains or employment (factor payments), generates momentum in the economy that reaches all households through other production activities that are directly or indirectly related to the wildlife ranching sector. Within the production activities, community, social and personal services, trade, transport, financial and insurance services, and chemicals and chemical products are some of the most important sectors through which income is transmitted to households via factor payments.
Finally, it is clear that the contribution of a specific sector should not be viewed in isolation from its structural paths/linkages to other sectors in a specific economy. This paper not only provides insights into the linkages of the wildlife ranching sector with the rest of the economy; it also supports previous findings which suggest that the sector makes a significant contribution, be it through direct or indirect linkages, towards the overall economy and subsequently national imperatives.
Notes
3The direct + indirect multiplier value (1.82) is calculated from Table A2 in Appendix A by subtracting the induced effect multiplier value (1.02) from the total production multiplier value (2.84) shown in column 2 of Table A2.
4The low multipliers for lower-income households reflect a high degree of dependence of these households on government transfers as a source of income. For households in the lower 50% of the income distribution, government transfers represent slightly less than 33% of income. Thus, the small shares of factor earnings garnered by lower-income households imply relatively low multipliers for these households.
5See note 3.
References
- Booth, VR, 2010. The Contribution of Hunting Tourism: How Significant is this to National Economies. Joint publication of FAO and CIC, Budapest.
- Bothma, J, Du, P, Suich, H & Spenceley, A, 2009. Extensive wildlife production on private land in South Africa. In Suich, H, Child, B & Spenceley, A (Eds.), Evolution and Innovation in Wildlife Conservation – Parks and Game Ranches to Transfrontier Conservation Areas. Earthscan, London, 163–84.
- Carruthers, J, 2008. ‘Wilding the farm or farming the wild’? The evolution of scientific game ranching in South Africa from 1960s to present. Transactions of the Royal Society of South Africa 63(2), 160–81. doi: 10.1080/00359190809519220
- Child, BA, Musengezi, J, Parent, GD & Child, GFT, 2012. The economics and institutional economics of wildlife on private land in Africa. Pastoralism: Research, Policy and Practice 2(18), 1–32.
- Cloete, PC, 2012. Investment in change: Wildlife versus agricultural development. Keynote address delivered at the ABSA Annual Wildlife Conference, 31 May, Port Elizabeth, South Africa.
- Conningarth Economists, 2006. Disaggregated social accounting matrix for South Africa. Unpublished report delivered as part of a research project commissioned by the office of the premier, North-West Province, Mafikeng, p. 79.
- Cousins, A, Sadler, JP & Evans, J, 2008. Exploring the role of private wildlife ranching as a conservation tool in South Africa: Stakeholder Perspectives. Ecology and Society 13(2), 1–17.
- Cousins, JA, Sadler, JP & Evans, J, 2010. The challanges of regulating private wildlife ranches for conservation in South Africa. Ecology and Society 15(2), 28.
- Defourny, J & Thorbecke, E, 1984. Structural path analysis and multiplier decomposition within a social accounting matrix. Economic Journal 94, 111–36. doi: 10.2307/2232220
- Department of Environmental Affairs and Tourism, 2005. Panel of experts on professional and recreational hunting in South Africa. Report to the Minister of Environmental Affairs and Tourism, DEAT, Pretoria.
- Dlamini, TS & Fraser, G, 2010. Economics of meat production from the Springbuck in the Eastern Cape Karoo. Paper presented at the joint 3rd AAAE and 48th AEASA Conference, 19–23 September, Cape Town.
- Dry, G, 2010. Commercial wildlife ranching contribution to the green economy. Green Economy Summit, 19 May. Pretoria, South Africa.
- Dry, G, 2013. Wildlife ranching in perspective. African Indaba. http://www.africaindaba.co.za/Newsletter/14htm Accessed 12 September 2013.
- Du Toit, JG, 2007. Report: Role of the Private Sector in the Wildlife Industry. Wildlife Ranching SA/Du Toit Wilddienste, Pretoria.
- Ellof, T, 2002. The economic realities of the game industry in South Africa. In Ebedes, H, Reilly, B, Van Hoven, W & Penzhorn, B (Eds.), Sustainable Utilisation – Conservation in Practice. South African Game Ranchers’ Organisation, Pretoria, 78–86.
- Jarell, R, 2003. A giant amongst ecologist: Raymond F. Dasmann, 1919–2002. Wildlife Society Bulletin 31(1), 324.
- Nell, DD'A, 2003. The development of wildlife utilization in South Africa and Kenya, c. 1950–1990. PhD thesis, University of Oxford.
- Ngandu, S, Garcia, AF & Arndt, C, 2010. The economic influence of infrastructural expenditure in South Africa: A multiplier and structural path analysis. Paper presented at the TIPS/DPRU Conference, 27 October, Johannesburg.
- Pyatt, G & Round, JI, 1979. Accounting and fixed price multipliers in a SAM framework. Economic Journal 89, 850–73. doi: 10.2307/2231503
- Roberts, D, 2005. The role of households in sustaining rural economies: A structural path analysis. European Review of Agricultural Economics 32, 393–420. doi: 10.1093/eurrag/jbi014
- Round, J, 2003. Social accounting matrices and SAM-based multiplier analysis. In Bourguignon, F & Pereira da Silva, LA (Eds.), Techniques and Tools for Evaluating the Poverty Impact of Economic Policies. World Bank and Oxford University Press, Oxford, 301–24.
- Van der Waal, C & Dekker, B, 2000. Game ranching in the Northern Province of South Africa. South African Journal of Wildlife Research 30, 151–6.
Appendix A