ABSTRACT
The South African existing literature on poverty mainly adopted the money-metric approach to examine poverty levels and trends since the advent of democracy. In general, poverty increased until the end of the 1990s, before a downward trend took place. Despite the robust findings on the trends, poverty levels differed because of various reasons, ranging from the use of different poverty lines across the studies, to the adoption of different approaches to collect the income and expenditure information, and the presence of a high proportion of households reporting zero or unspecified income. This article aims to fill the existing research gap by explaining the possible factors accounting for the contrasting poverty levels across the eight commonly used South African censuses and household surveys between 1993 and 2012.
JEL CLASSIFICATION:
1. Introduction
To evaluate the extent to which a country achieved the objective of poverty reduction, reliable income and expenditure data are required. Since the advent of democracy, a major advance by Statistics South Africa (Stats SA) was the improvement of the Income and Expenditure Survey (IES) and the October Household Survey (OHS), as the sample was extended to all areas (the IES sample covered households in both metropolitan and non-metropolitan areas, while the OHS sample no longer excluded people residing in the Transkei–Bophuthatswana–Venda–Ciskei states). New surveys were conducted, such as the General Household Survey (GHS) introduced in 2002, the Labour Force Survey (LFS) which has been replacing the OHS since 2000, and the Quarterly Labour Force Survey which has been replacing the LFS since 2008. Institutions other than Stats SA have been conducting surveys, such as the Project for Statistics on Living Standards and Development (PSLSD) and the National Income Dynamic Study (NIDS) conducted by the Southern Africa Labour and Development Research Unit (SALDRU). Although the All Media Products Survey (AMPS) has been conducted by the South African Advertising Research Foundation since 1975, it has only been used for poverty analysis in recent years.
The South African money-metric poverty studies employed the above-mentioned datasets. Although different poverty lines were used, their findings generally reflected poverty increased until 2000, after which there was a continuous downward trend. Despite the robust findings on trends, poverty levels differed amongst the studies. It is always a problem to compare poverty levels because of various reasons. Firstly, the studies might have simply examined surveys from different years (e.g. study X examined IES 1995 and 2005, while study Y compared AMPS 1998 with 2008). Even if some studies used surveys taking place in the same year, different poverty lines were used (e.g. US$1 in one study but US$2 in another study). Furthermore, the income and expenditure data were captured differently across the surveys, thereby affecting the reliability and comparability of the ensuing poverty estimates. Finally, few studies adjusted the income and expenditure to derive a revised per-capita variable before estimating poverty levels.
This study aims to fill the existing research gap by examining how different the poverty levels are across the surveys and the factors accounting for these differences. Section 2 provides a literature review on the main factors affecting the comparability of income and expenditure information across the surveys as well as the recent studies examining money-metric poverty levels and trends. Section 3 discusses how the income and expenditure information was captured, and poverty levels and trends across all the surveys and censuses during 1993–2012 if the same poverty line is used. Section 4 concludes.
2. Literature review
2.1 Factors affecting the comparability of poverty estimates across surveys and censuses
A general argument for different poverty levels is that respondents report different income and expenditure amounts. Most rich countries use the income variable, as income mainly comes from salaries and wages and hence is easier to measure, while it is difficult to quantify the volumes and amounts of purchases when capturing expenditure. In poor countries, income is relatively harder to measure as much of it comes from self-employment, or own-account agricultural and informal activities associated with volatile earnings (Deaton & Grosh, Citation2000:93–4; Haughton & Khandker, Citation2009:30). Although random irregularities and seasonal patterns could be present in expenditure, they are normally smaller than those of income, because expenditure is less tied to seasonal and weather-related patterns in agriculture (Deaton & Grosh, Citation2000:93–4).
The ways in which the income and expenditure information is captured could affect the comparability of such information and the ensuing poverty estimates. In the South African surveys, the recall method was adopted – except in IES 2005/06 and 2010/11, which adopted the diary method to complement the recall method. Recall bias often happens under the recall approach, as the respondents could not remember income earned a long time ago and purchases long after they have been made (Deaton, Citation1997:24–5; Deaton & Grosh, Citation2000:109–10), leading to inaccurate guesses. This recall bias becomes more serious as the recall period increases.
The recall approach also leads to the telescoping phenomenon, as the respondents may include consumption events that took place before the beginning of the recall period, thereby resulting in overestimation of expenditure (Deaton & Grosh, Citation2000:110), and subsequently an underestimation of the poverty level. Deaton (Citation2005:16) suggests a shorter recall period, but many visits are required to ensure that data on high-frequency non-durable purchases are collected accurately, assuming the respondents’ memories of their consumption activities fade quickly. However, the increase of the interview frequency is time-consuming and costly. As the consumption of durable items only take place occasionally during the year, a longer recall period is required, yet this is associated with a greater likelihood of recall bias and telescoping.
Because of the drawbacks of the recall method, the diary method becomes an alternative approach. This is designed to reduce the likelihood of recall bias and telescoping, as consumption events are recorded on the diary as they take place or close to that time (Deaton & Grosh, Citation2000:109; Battinstin, Citation2003:2; Wiseman et al., Citation2005:395). However, diaries are less suitable where literacy levels are low because the diary keepers might not write down the purchase items correctly if given an unstructured diary.Footnote1 In other words, the data collected from the diaries might be biased towards the competent, literate diary keepers (Corti, Citation1993). A pictorial diary might be required to improve the accuracy of the responses of those with lower literacy levels. Since the participants were asked to keep the diaries for a very short period of time (e.g. four weeks in the IES), the diary method might work better for non-durable items with higher purchase frequency, but is not suitable to record the consumption of more durable items with low purchase frequency (Deaton & Grosh, Citation2000:119–22; Battinstin, Citation2003:2).
Recall bias and telescoping could still take place under the diary approach, as respondents may not report the consumption events immediately after the purchases took place (Deaton, Citation1997:24–5; Wiseman et al., Citation2005:398). A one-day effect could happen in the diary approach (Deaton & Grosh, Citation2000:119–22; Wiseman et al., Citation2005:395; Ahmed et al., Citation2006:9–10), as the first week of diary keeping shows higher reporting of consumption than the following weeks because the novelty of diary keeping wears off as time goes by, and the respondents feel exhausted of keeping records and become less detailed in their reporting.
Posel & Casale (Citation2005:10), Von Fintel (Citation2006:1) and Malherbe (Citation2007:25) argue that some respondents were reluctant to disclose the exact amount due to privacy concerns, while others really do not know this exact amount and hence could not specify how much they earned or spent. The interval approach permits the respondents to report income and expenditure with a margin of error, especially if they do not know the exact amounts earned or spent. However, the income or expenditure brackets need to be adjusted as survey years progress, or an increasing proportion of households fall in the higher-income or higher-expenditure categories due to the impact of inflation if the brackets are left unadjusted.
If the respondents are asked to declare the exact amounts earned or spent, the next issue to decide is whether to ask the respondents to declare the ‘one-shot’, single estimate (by asking questions such as ‘What is the total income you earned from all sources in the past 12 months?’) or to aggregate the amounts from sub-categories (i.e. by asking questions such as ‘How much do you earn from income source X?’). The single estimate approach, despite being relatively less time-consuming and costly, may confuse the respondents, as they are unsure about what items should be included as part of the total income or expenditure. This may result in a low response rate, and/or under-reporting of total income or expenditure (Deaton, Citation1997:27; Browning et al., Citation2002:7–10). Note that the interval approach already discussed also relates to the single estimate method, as the respondents were asked to declare the ‘one-shot’ amount by choosing the relevant category.
If the aggregation method is used, the appropriate level of disaggregation should be considered. Deaton (Citation2005:16) claims that the greater the degree of disaggregation of the number of items that are separately distinguished, the more accurate the measured income or expenditure in total. However, Browning et al. (Citation2002:12–18) and Deaton (Citation2005:16) suggest that, if the level of disaggregation is too high, it could be time-consuming and exhausting to both the interviewers and interviewees, and the latter might end up deliberately providing misleading amounts and even not answering some questions (i.e. item non-response). This leads to an inaccurate aggregate amount, compared with the single estimate method.
If the information was collected in bands, the data need to be made continuous. That is, the amount of each band needs to be determined. Commonly used approaches are the midpoint Pareto method, interval regression, the random midpoint method and the equal distribution method (Cloutier, Citation1988; Fields, Citation1989; Whiteford & McGarth, Citation1994; Gustavsson, Citation2004; Malherbe, Citation2007).
The accuracy of the income and expenditure information could be influenced by the number of intervals and the width of each band. For instance, if a household's exact monthly income is R8200 in nominal terms, this household would fall in the ‘R6401–R12 800’ category in CS 2007, ‘R5000–R9999’ interval in the GHS and ‘R8000–R9999’ interval in the AMPS. The derived income or expenditure amount would be estimated as R9600, R7500 and R9000 respectively in each survey. These different amounts could result in different poverty estimates across the surveys. There is a lack of both South African and international studies to investigate the impact of the number of intervals and width of each interval on poverty estimates, except that Seiver (Citation1979) found that fewer, wider brackets result in overestimation of inequality measures.
The presence of households reporting zero or unspecified income or expenditure could also affect reliability of poverty estimates. Ardington et al. (Citation2006) argue that if those with missing data fall excessively in the bottom of the income distribution, then poverty levels will be under-estimated if they are ignored. If non-response is higher among the affluent, poverty estimates are likely to be biased upwards. If the zero-income households are included unadjusted for the analyses, this leads to a serious over-estimation of measured poverty. Looking at the missing data in greater detail, unless the data are missing completely at random, ignoring these households would lead to biased poverty estimates. Commonly used methods to deal with missing data are case-wise deletion, available-case deletion, single imputation (e.g. cell mean substitution, hot deck imputation, cold deck imputation) and multiple imputation (Lacerda et al., Citation2008). For the latter approach, a commonly used approach when data are missing at random is the sequential regression multiple imputation (SRMI).Footnote2 This approach will be adopted in the forthcoming sections when investigating the comparability of poverty levels across surveys that include a high proportion of respondents with zero or unspecified income or expenditure.
Because of the various reasons discussed (e.g. fatigue, illiteracy, and recall bias), surveys are more likely to under-estimate income (expenditure), and subsequently over-estimate poverty levels. Some surveys might have under-estimated income more seriously, and this makes it difficult to compare poverty levels across the surveys. Hence, the distributional estimates of the survey data could be adjusted rightwards in line with the national accounts series for aggregate income, before poverty levels are re-estimated. That is, household survey means are replaced by the national accounts mean, but the distribution of the household survey is retained. This takes place under the following two assumptions (Deaton, Citation2001:135): the national accounts estimates are correct; and survey estimates of the mean are correct.
Person weights in the household surveys are post-stratified to the external population totals (for instance, the mid-year population estimates at the time of the survey, derived using the Census information), with the pre-census and post-census years' populations being calculated using exponential interpolation and extrapolation. However, there are concerns regarding the reliability of the post-stratification design weights, as the mid-year population estimates could be inconsistent over time; that is, temporal consistency is not considered (Branson & Wittenberg, Citation2014:20). This leads to problems when the cross-sectional data are used for time-series poverty analysis. Therefore, there is a need to re-weight the weight variables so that the population data and poverty estimates derived would become more time-consistent and comparable. One possible solution is the cross-entropy estimation approach,Footnote3 which adjusts the weights to conform to the race, gender and age distribution of the population estimates derived by the Actuarial Society of South Africa 2003 model, as the population data derived from this model are more time consistent.
Finally, other factors affecting the reliability of poverty estimates include the length of the questionnaire, quality of training received by the interviewers prior the start of the interviews, their experience and efforts devoted to capture information during the interviews.
2.2 Review of recent studies examining poverty levels and trends
There is an abundance of recent studies examining money-metric poverty levels and trends. The commonly used poverty lines are R250 and R322 per capita per month in 2000 prices and the international US$1 and US$2 a day. These studies could be categorised into two groups. First, various studies used the data unadjusted. For example, Hoogeveen & Özler (Citation2006), Özler (Citation2007), Yu (Citation2008) as well as Bhorat & Van der Westhuizen (Citation2012) examined the IES data; Meth & Dias (Citation2004) and Vermaak (Citation2005) used the OHS and LFS data; Van der Berg et al. (Citation2007a, Citation2008) looked at the AMPS data; and Agüero et al. (Citation2005) used a provincial dataset known as the KwaZulu-Natal Income Dynamics Study. Although different poverty lines were used, these studies found that poverty levels increased in the 1990s, before a downward trend took place.
The second group of studies considered the factors as discussed in Section 2.1 and hence adjusted the survey data, before poverty levels were estimated. For instance, Van der Berg & Louw (Citation2004) as well as Pauw & Mncube (Citation2007) were concerned that the rapid decline in household income between IES 1995 and IES 2000 was inconsistent with the increase of current household income as captured in the national accounts. Therefore, Van der Berg and Louw calculated mean incomes by race using national accounts and other sources of data, before applying these income values to the intra-group distributions of income in these IEs. Using the poverty line of R250 per month in 2000 prices, they found that the poverty headcount ratio was stable across the two surveys (from 0.39 to 0.38), but the absolute number of people living in poverty increased. Pauw & Mncube (Citation2007) imputed the food and tax expenditures in IES 2000 to replace unexpected missing or zero values or cases of obvious under-reporting. They assumed that for each household in both surveys, the larger of total income and expenditure was the correct measure to be used to derive the per-capita variable. In the end, they found that poverty levels increased moderately between 1995 and 2000.
Van der Berg et al. (Citation2005, Citation2007b) adjusted the AMPS distributional estimates of income rightwards to be consistent with the national accounts series for aggregate household income, and found that the poverty headcount ratio has been increasing slightly since 1993, before a moderate downward trend was observed in the 2000s. Leibbrandt et al. (Citation2006) estimated poverty with Census 1996 and Census 2001. If the data were used unadjusted, at a poverty line of R250 per month in 2000 prices, the poverty headcount ratio increased from 0.59 to 0.65. However, once the zero-income households were excluded, although the poverty trend remained the same, the poverty level was lower in both censuses (0.50 in 1996 and 0.55 in 2001). Yu (Citation2009) used the above two censuses and CS 2007, but he imputed the income of households with zero or unspecified income by SRMI. He found that the poverty trend was unchanged (poverty increased between 1996 and 2001, before a decline took place in 2007), but the poverty levels were lower in all three years after SRMI.
Leibbrandt et al. (Citation2010) was one of the rare studies using different data sources (PSLSD 1993, IES 2000 and NIDS 2008) to investigate poverty trends. Certain income items were excluded (e.g. imputed rent, agricultural income, sale of vehicles and property) so that the per-capita income derived across the data sources would be more comparable across the surveys. Using the revised per-capita income variable, they found that the poverty headcount ratio showed a negligent decline (0.56, 0.54 and 0.54 in 1993, 2000 and 2008 respectively using the R211 poverty line, and 0.72. 0.71 and 0.70 using the R322 poverty line). Simkins (Citation2004) also used different data sources to examine poverty (IES 1995 and 2000; Census 1996 and 2001). However, he did not explain clearly the decision rules he applied to impute the income of households with unspecified incomes, and did not specify the poverty lines used. Nonetheless, he found that the poverty headcount ratio increased between the two IESs and censuses.
To conclude, with the exception of a few studies, recent studies suggest that poverty increased following the advent of democracy, before a continuous decline took place from 2000. Since these studies examined surveys taking place in different years and/or used different poverty lines, it is difficult to compare the poverty levels amongst these studies.
3. South African poverty estimates: 1993–2012
3.1 How the income and expenditure information was captured in each survey
summarises the collection of income and expenditure information in each household survey and census.Footnote4 Income was collected in some surveys but expenditure was collected in others. Only the IESs, NIDS and OHS 1999 collected both income and expenditure. Respondents were asked to declare the actual amounts in some surveys (e.g. IESs, NIDS, OHS 1996–98), but the relevant interval in others (e.g. censuses and CS 2007, LFSs, GHSs, AMPSs). Looking at the former approach in detail, respondents were asked to declare a single-estimate amount in some surveys either in actual continuous amount (e.g. OHS 1999) or in interval terms (e.g. AMPSs), but in other surveys (IESs and NIDS) they were asked to report the amounts on each income or expenditure source, before these amounts were added to derive total household income or expenditure. in Appendix A shows the number of intervals and width of each interval in selected surveys. The nominal brackets have been left unadjusted, except between Census 2001 and CS 2007 and across the AMPSs.
Table 1. Availability of income and expenditure information in the 1993–2012 South African household surveys: a summary
IES 2005/06 and 2010/11 adopted the diary approach to complement the recall method to capture semi-durable and durable expenditure. Non-durable expenditure was captured exclusively by the diary method, while only the recall method was used to collect income information (Yu, Citation2008). To deal with the one-day effect, only households that completed the main questionnaire and at least two weekly diaries were accepted.Footnote5
Two further issues need to be taken into consideration. First, the Standard Trade Classification (STC) approach was adopted to categorise the income and expenditure items in IES 1995 and IES 2000, but the Classification of Individual Consumption According to Purpose (COICOP) approach was used in IES 2005/06 and 2010/11.Footnote6 Since COCIOP is different from STC, in order to have consistent income and expenditure variables across the four IESs there are two options: re-categorise the income and expenditure items in the 1995 and 2000 surveys, using the 2005 COICOP structure; or re-categorise the income and expenditure items in the 2005/06 survey using STC. Both approaches will be adopted when comparing the income and expenditure data and poverty estimates in the IESs.
NIDS was the only survey asking the respondents to declare income and expenditure by both the single estimate and aggregation approaches. Looking at the latter in greater detail, household expenditure was derived by adding the respondents’ answers on 32 categories of food spending, 54 categories of non-food spending and rent expenditure, while household income was derived by summing the respondents’ answers on seven broad components, namely wage income, government grant income, other government income, agricultural income, remittances income, investment income and implied rent income.
SALDRU was concerned about the low response rate to the single-estimate amount questions and that poverty would be over-estimated because the amount derived from the single estimate approach was lower, and decided to use the income and expenditure variables derived by the aggregation approach to conduct poverty analyses in the official NIDS reports (e.g. Argent et al., Citation2009; Finn et al., Citation2009; Finn & Leibbrandt, Citation2013). All households had specified aggregate income and expenditure as imputations were applied to deal with item non-response.
For the interval data, the midpoint Pareto method was used to approximate the total income or expenditure amount in each category. The proportion of households with zero income was 13.0%, 21.0%, 8.2% and 15.1% in Census 1996, Census 2001, CS 2007 and Census 2011 respectively, while the proportion of households with unspecified income was 11.5%, 16.4%, 11.1% and 0.1% respectively. The latter proportion was only ranged between 1.5 and 3.5% in the GHS expenditure data. Therefore, the SRMI approach at household levelFootnote7 would be applied to impute the income of households with zero or unspecified income in the three censuses and CS 2007 to obtain more reliable poverty estimates.
3.2 Comparison of survey income and expenditure estimates with national accounts income
The nominal income and expenditure amounts were converted into real amounts in constant 2013 prices using the South African Reserve Bank's monthly consumer price index series. in Appendix A presents the real total annual income and expenditure captured in each census and survey, as well as the census/survey amount as proportion of the national accounts income of the same year. Looking at the three censuses and CS 2007, this proportion was the highest in Census 2011 (71.3%) before SRMI but was the highest in CS 2007 (85.6%) after SRMI. As expected, after dealing with households with zero or unspecified income by SRMI, total income became higher.
With regard to the IESs, the 1995 survey best captured total income and expenditure. Under the STC approach, these amounts were equal to 95% of the national accounts income amount. One notable finding from Table A2 is that total income, expenditure and consumption experienced a sharp decline between 1995 and 2000, contradicting the upward trend in the national accounts total income between the two years.
Total expenditure was seriously under-captured in the OHSs, LFSs and GHSs, as it only amounted to 30 to 50% of the national accounts income (except in GHS 2009–12). The OHS 1999 income variable was an exception because total income amounted to 94.9% of the 1999 national accounts income. In almost all AMPSs, total income was approximately 55 to 65% of the national accounts income.
Comparing the results across the surveys in greater detail, for surveys capturing both income and expenditure the income was greater than expenditure in all surveys except IES 2005/06, as shown in . This contradicts the earlier argument in the literature review that expenditure is captured better in poorer countries.
Figure 1. Total income and expenditure (Rand million, 2013 prices) of surveys that collected both income and expenditure.
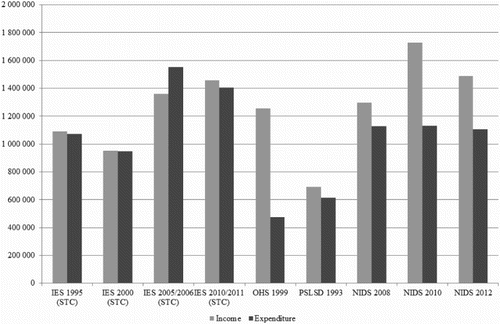
suggests that the interval approach leads to under-estimation of income and expenditure. For instance, the 1995 AMPS total income (captured in bands) was smaller than the 1995 IES total income (captured in exact amount), while in 2005 the GHS expenditure and the AMPS income (captured in bands) were lower compared with the IES income (captured in exact amount). Nonetheless, in 2010 the GHS total expenditure that was captured in bands was greater than income captured in continuous amounts in NIDS and IES.
Figure 2. Total income or expenditure (Rand million, 2013 prices) of selected surveys taking place in the same year.
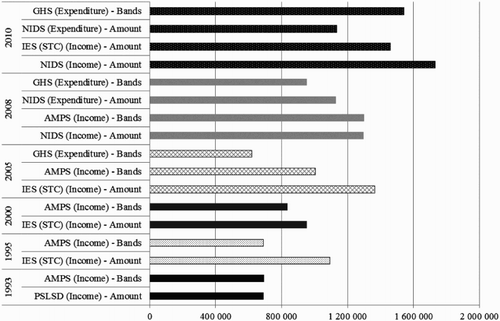
Table A2 also shows that total expenditure in OHSs, LFSs and GHSs (with very few intervals) was lower. Total income in Census 1996 and 2001 (with fewer intervals and very wide intervals in the higher-income categories) was lower than the total income captured in the same years by AMPSs, which has more income intervals and narrower width in each interval. However, the opposite finding was observed when comparing CS 2007 with AMPS 2007. Finally, the AMPS income was always bigger than the GHS expenditure of the same year, except in 2009.
shows that real food expenditure, which was captured entirely by the diary method in IES 2005/06 and 2010/11, was surprisingly lower when compared with the 1995 and 2000 IESs (which captured food expenditure with the recall approach). Food expenditure as a proportion of total expenditure abruptly declined between 2000 and 2005/06, contradicting the results of GHSs, which found that the proportion of households reporting they never experienced adult hunger and child hunger in the past 12 months was higher in recent years.Footnote8
Figure 3. Annual food and transport expenditure (Rand million, 2013 prices) in the IESs (STC approach).
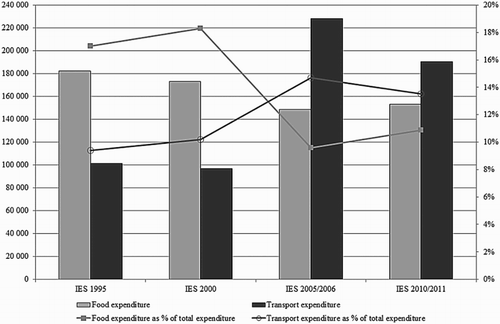
The diary method may have resulted in the under-estimation of food expenditure due to reasons such as the first-day effect and illiteracy of respondents. It is also likely that the recall method adopted in IES 1995 and IES 2000 resulted in an over-estimation of food expenditure due to factors such as recall bias and telescoping, while the IES 2005/06 and 2010/11 food expenditure estimate might actually be more reliable. In contrast, regardless of whether the STC or COICOP approach was adopted, transport expenditure was much higher in IES 2005/06 and 2010/11 (see ). This could be attributable to the use of the diary method to complement the recall method, thereby resulting in a better capture of transport expenditure in these two surveys.
For the three NIDS, Table A2 shows that total income and expenditure derived from the single estimate method was much lower than the amount derived from the aggregation approach. The lower amount captured in the single estimate approach might be attributed to the fact that the respondents did not know which items to include in this ‘one-shot’ amount.
3.3 Poverty levels derived from each survey: 1993–2012
The lower bound poverty line (R322 per capita per month in 2000 prices, or R665 in 2013 prices) proposed by Woolard & Leibbrandt (Citation2006) is used to examine poverty between 1993 and 2012. The focus is the Foster–Greer–Thorbecke poverty headcount ratios.
The results are presented in and the last column of in Appendix A. Looking at the three censuses, the poverty headcount ratio increased between 1996 and 2001, before a rapid decline took place between 2001 and 2011. After taking CS 2007 into consideration, poverty actually increased between 2007 and 2011. The finding could be due to the fact that a higher proportion of households reporting zero income in 2007. However, even after SRMI was conducted, the 2011 poverty headcount ratio remained higher than the 2007 estimate.
Figure 4. Poverty headcount ratios in each survey, 1993–2012 (Poverty line: R665 per capita per month, 2013 prices).
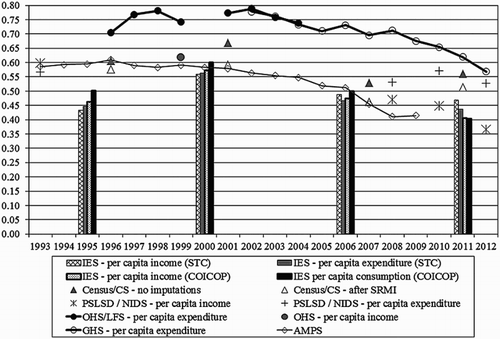
The poverty headcount ratio increased rapidly between IES 1995 and IES 2000, before a downward trend was observed between IES 2000 and IES 2010/11, regardless of whether the STC or COICOP approach was adopted. Van der Berg et al. (Citation2008) argued that the extent of increase of poverty between IES 1995 and IES 2000 could be over-estimated because of the huge drop of recorded income and expenditure between the two surveys, as mentioned earlier.
Looking at the OHS and LFS data, the poverty headcount ratio increased since 1996, before a downward trend was observed from 2002. In the GHSs, a downward trend was observed in 2002–05 and 2009–12, but an unstable trend was observed between 2005 and 2008. In AMPSs, the poverty headcount ratio stabilised at approximately 0.59 between 1993 and 1999, before a continuous downward trend took place between 2000 and 2008. Poverty increased slightly between 2008 and 2009, just like what happened between CS 2007 and Census 2011.
In the NIDS, the poverty headcount ratio was higher if expenditure was used. Poverty declined continuously between 2008 and 2012 using the income variable, but it first increased between 2008 and 2010, before a decline took place in 2012 when the expenditure variable was used. As expected, the single estimate approach resulted in serious under-estimation of total income and expenditure, and hence the poverty headcount ratios using the per-capita variables derived from this approach are much higher when compared with the ratios using per-capita variables derived from the aggregation approach.
However, these NIDS poverty estimates might not be fully comparable, because as mentioned in Section 3.1 all households had specified aggregate income and expenditure as imputations were applied to deal with item non-response, while not all households reported single estimate income and expenditure amounts, due to low response rates for these two questions. Hence, the more precise method is to compare the poverty estimates of those reporting both single estimate and aggregate amounts before imputations. The results are presented in , and confirm that the single estimate approach results in higher poverty estimates. The main reason accounting for the latter finding is that respondents tend to report lower amounts under the single estimate approach as compared with the aggregation approach (see as an example on NIDS 2012 – in 63.3% of the households reporting income amounts under both approaches in the sample, total income derived by the aggregation approach was greater than total income derived by the single estimate approach, as the ratio of the two income amounts exceeds one), and the under-capture of income from the single estimate approach could be attributed to the reasons discussed in Section 2.
Table 2. Poverty headcount ratios in NIDS (Poverty line: R665 per capita per month, 2013 prices), only including respondents reporting income (expenditure) in both the single estimate approach and the aggregation approach before imputations.
Table 3. Total income derived income by the aggregation approach/total income derived by the single estimate approach ratio, NIDS 2012.
Looking at poverty levels of surveys and censuses taking place in the same year, the 1993 PSLSD poverty headcount ratios, regardless of whether income or expenditure was used, were close to the 1993 AMPS ratio. In 1996, the OHS estimate using expenditure was significantly higher (0.704), while the Census and AMPS estimates were lower (between 0.576 and 0.610). In 1999, the OHS estimate using expenditure was higher (0.742) than the OHS and AMPS estimates using income (0.617 and 0.591 respectively). In 2001, the LFS estimate was the highest (0.773), followed by the Census estimate before SRMI (0.670), while the Census estimate after SRMI (0.592) was very close to the AMPS estimate (0.579).
In 2006, the GHS poverty estimate (0.731) was much higher than the AMPS (0.512) and IES (ranging between 0.466 and 0.500) estimates. The GHS estimate was the highest in 2008 (0.712) compared with the estimates of NIDS (0.471 and 0.532 using income and expenditure respectively) and AMPS (0.410). In 2011, the Census poverty headcount ratio estimates (0.560 before SRMI and 0.513 after SRMI) were sandwiched between the GHS (0.619) and IES (between 0.404 and 0.468) estimates. Finally, the LFS 2002–04 poverty headcount ratios were similar to the GHS 2002–04 estimates.
To conclude, using a consistent poverty line across all surveys/censuses, despite the fact that the levels of poverty differed a lot across the surveys, poverty increased until about 2000 before a downward trend took place in the 2000s. The only four exceptions were: a stable poverty level observed in AMPS in the 1990s; poverty increased negligently between AMPS 2008 and 2009; poverty increased between CS 2007 and Census 2011; and poverty increased between NIDS 2008 and NIDS 2010 using the expenditure variable. Poverty levels were much higher in OHSs, LFSs and GHSs, which collected the income and expenditure information in fewer bands. Finally, the single estimate approach in NIDS resulted in higher poverty estimates, when compared with the aggregation approach.
The poverty estimates could be re-visited by adopting a consistent approach (e.g. cross-entropy method) to re-weight all datasets or by adjusting the survey income or expenditure distribution in line with the national accounts mean income (as in Van der Berg et al., Citation2005, Citation2007b). However, this requires a detailed study and would not be conducted here.
3.4 Would the IES poverty levels change significantly if the interval approach is applied?
This section re-visits the IES poverty levels by applying the intervals of the surveys taking place in similar years. The OHS 1996, Census 1996 and AMPS 1995 intervals would be applied to the IES 1995 data, while the GHS 2010, Census 2011 and AMPS 2010 intervals would be applied to the IES 2010/11 data. However, an assumption is made that the respondents would give consistent responses between the two approaches.Footnote9
The results are presented in . Poverty estimates using the continuous per-capita income variables, as they are, are similar to the estimates using the per-capita income variables derived using the single estimate interval methods. For example, in IES 1995 the poverty headcount ratio using the actual continuous income variable was 0.434, but the estimate ranged between 0.418 and 0.435 after the OHS/Census/AMPS intervals were applied. Similar findings could be observed in the other three IESs. However, the results need to be interpreted with great caution, as Section 3.3 already highlighted that the income (expenditure) amount derived by the aggregation approach could be very different from the income (expenditure) amount derived by the single estimate approach.
Table 4. Poverty headcount ratios in IESs (Poverty line: R665 per capita per month, 2013 prices), if the interval method is applied.
4. Conclusion
This article first examined the main factors affecting the comparability and reliability of income and expenditure data across household surveys/censuses, before comparing these data across the surveys/censuses. Real per-capita income and expenditure were used to derive the Foster–Greer–Thorbecke poverty headcount ratios between 1993 and 2012. Since the income and expenditure information was captured differently, this led to the variation in the income and expenditure amounts in each survey, and the levels of poverty were different across the surveys/censuses. Poverty trends were similar in all surveys/censuses, except in AMPS, between CS 2007 and Census 2011, and between the 2008 and 2010 NIDS (expenditure variable). In AMPSs, which captured income in a greater number of bands and a narrower width in each band, the poverty levels and trends were much more stable. The poverty estimates were much higher in the OHSs, LFSs and GHSs, which are associated with fewer bands. Finally, the single estimate variables resulted in higher poverty levels in the NIDS data.
As income and expenditure was captured differently, it is hard to expect the poverty estimates to be similar. It is also difficult to expect the income and expenditure information to be captured in exactly the same way (e.g. expecting Stats SA to have the same interval bands in GHSs and censuses). In order to improve the reliability and comparability of these estimates, the institutes conducting the surveys and censuses should at least consider adjusting the number of intervals and the width of each interval frequently to account for the impact of inflation. Unfortunately, this only happens in the AMPSs and between Census 2001 and CS 2007. Had these adjustments taken place more regularly, it could have improved the reliability of the poverty estimates. Also, because the present analysis strongly indicates that surveys with fewer bands (i.e. LFSs and GHSs) are associated with much higher poverty estimates, Stats SA should consider revising the expenditure intervals in GHSs drastically, in order to improve the capture of expenditure and reliability of poverty estimates. Finally, one must interpret the census and CS 2007 poverty estimates using the income variables as they are with great caution, because these censuses and survey are characterised by the presence of a relatively high proportion of households with zero or unspecified income. The income of these households should be imputed, before more precise poverty estimates could be derived.
Acknowledgement
The author gratefully acknowledges the valuable comments by Servaas van der Berg.
Disclosure statement
No potential conflict of interest was reported by the author.
Notes
1This is the case in IES 2005/06 and 2010/11, as the participants were given a diary with blank pages to fill in the consumption activities.
2For more detailed explanation of the SRMI methodology, refer to Raghunathan et al. (Citation2001), Ardington et al. (Citation2006), Lacerda et al. (Citation2008) and Vermaak (Citation2008).
3Detailed explanation of the methodology of this approach falls beyond the scope of this study, but the reader can be referred to Wittenberg (Citation2010:315–19) as well as Branson & Wittenberg (Citation2014:26–8).
4At the time of writing, the author had requested the 2010–2012 AMPS data from the South African Advertising Research Foundation but did not receive a response.
5If a household had two (three) completed diaries, expenditure from the two (three) diaries was added together and the sum was divided by two (three). This average figure was then used to impute for the remaining two (one) non-completed/missing diaries (diary) (Stats SA, Citation2006).
6For a detailed discussion on the difference between the STC and COICOP approaches, refer to Yu (Citation2008).
7Refer to Yu (Citation2009) for detailed explanation on how SRMI was conducted.
8The proportion of households never experiencing adult hunger increased from 69% in GHS 2002 to above 80% in GHS 2006–12, while the proportion of households never experiencing child hunger increased from 69% to the 80 to 85% range in GHS 2006–12.
9For example, if the total monthly household income of a household was derived as R5500 in IES 1995, it is assumed that the household head would report that his/her household falls in the OHS 1996 ‘R5000–R9999’ category, the Census 1996 ‘R3201–R6400’ category and the AMPS 1995 ‘R5000–R5999’ category.
References
- Agüero, J, Carter, MR & May, J, 2005. Poverty and inequality in the first decade of democracy: Evidence from KwaZulu-Natal. Proceedings from the South African economic policy under democracy: A ten-year review seminar, 16–17 June, Stellenbosch.
- Ahmed, N, Brzozowski, M & Crossley, TF, 2006. Measurement errors in recall food consumption data. IFS Working Paper W06/21. Institute for Fiscal Studies, London.
- Ardington, C, Lam, D, Leibbrandt, M & Welch, M, 2006. The sensitivity to key data imputations of recent estimates of income poverty and inequality in South Africa. Economic Modelling 23(5), 822–835.
- Argent, J, Franklin, S, Keswell, M, Leibbrandt, M & Levinsohn, J, 2009. Expenditure: Report on NIDS Wave 1. Technical Paper No. 4. Southern African Labour and Development Research Unit, Cape Town.
- Battinstin, E, 2003. Errors in survey reports of consumption expenditures. IFS Working Papers WP03/07. Institute for Fiscal Studies, London.
- Bhorat, H & Van der Westhuizen, C, 2012. Poverty, inequality and the nature of economic growth in South Africa. DPRU Working Paper 12/151. Development Policy Research Unit, Cape Town.
- Branson, N & Wittenberg, M, 2014. Reweighting South African national household survey data to create a consistent series over time: A cross-entropy estimation approach. South African Journal of Economics 82(1), 19–38. doi: 10.1111/saje.12017
- Browning, M, Crossley, TF & Weber, G, 2002. Asking consumption questions in general purpose surveys. SEDAP Research Paper No. 77. The Program for Research on Social and Economic Dimensions of an Aging Population (SEDAP), Hamilton.
- Cloutier, NR, 1988. Pareto extrapolation using grouped income data. Journal of Regional Science 28(3), 415–419. doi: 10.1111/j.1467-9787.1988.tb01092.x
- Corti, L, 1993. Using diaries in social research. Social Research Update: March 1993. University of Surrey, Guildford.
- Deaton, A, 1997. The analysis of household surveys: A microeconomic approach to development policy. The John Hopkins University Press, Baltimore.
- Deaton, A, 2001. Counting the world's poor: Problems and possible solutions. World Bank Research Observer 16(2), 125–147. doi: 10.1093/wbro/16.2.125
- Deaton, A, 2005. Measuring poverty in a growing world (or measuring growth in a poor world). The Review of Economics and Statistics 87(1), 1–19. doi: 10.1162/0034653053327612
- Deaton, A & Grosh, M, 2000. Consumption. In Grosh, M. & Glewwe, P. (Eds.), Designing household survey questionnaire for developing countries: Lessons from 15 years of the living standards measurement study – Volume One. The World Bank, Washington, DC, 91–133.
- Fields, GS, 1989. A compendium of data on inequality and poverty for the developing world. Unpublished report, Cornell University, New York.
- Finn, A & Leibbrandt, M, 2013. The dynamics of poverty in the first three waves of NIDS. NIDS Discussion Paper 2013/1 and SALDRU Working Paper Series Number 119. Southern African Labour and Development Research Unit, Cape Town.
- Finn, A, Leibbrandt, M & Woolard, I, 2009. Income and expenditure inequality: Analysis of the NIDS wave 1 dataset. Discussion Paper No. 5. Southern Africa Labour and Development Research Unit, University of Cape Town, Cape Town.
- Gustavsson, M, 2004. Trends in the transitory variance of earnings: Evidence from Sweden 1960–1990 and a comparison with the United States. Uppsala University Economics Working Paper 2004:11. Uppsala University, Uppsala.
- Haughton, J & Khandker, SR, 2009. Handbook on poverty and inequality. The World Bank, Washington, DC.
- Hoogeveen, JG & Özler, B, 2006. Poverty and inequality in post-apartheid South Africa: 1995–2000. In Bhorat, H. & Kanbur, R. (Eds.), Poverty and policy in post-apartheid South Africa. Human Sciences Research Council, Cape Town, 59–94.
- Lacerda, M, Ardington, C & Leibbrandt, M, 2008. Sequential regression multiple imputation for incomplete multivariate data using Markov chain Monte Carlo. SALDRU Paper Series Number 13. Southern African Labour and Development Research Unit, University of Cape Town, Cape Town.
- Leibbrandt, M, Poswell, L, Naidoo, P & Welch, M, 2006. Measuring recent changes in South African inequality and poverty using 1996 and 2001 census data. In Bhorat, H. & Kanbur, R. (Eds.), Poverty and policies in post-apartheid South Africa. Human Sciences Research Council, Cape Town, 95–142.
- Leibbrandt, M, Woolard, I, Finn, A & Argent, J, 2010. Trends in South African income distribution and poverty since the fall of apartheid. OECD Social, Employment and Migration Working Papers No. 101. Organization for Economic Co-operation and Development, Paris.
- Malherbe, JE, 2007. An analysis of income and poverty in South Africa. Unpublished Master thesis, Stellenbosch University, Stellenbosch.
- Meth, C & Dias, R, 2004. Increases in poverty in South Africa, 1999–2002. Development Southern Africa 21(1), 59–85. doi: 10.1080/0376835042000181426
- Özler, B, 2007. Not separate, not equal, poverty and inequality in post-apartheid South Africa. Economic Development and Cultural Change 55(3), 487–529. doi: 10.1086/511191
- Pauw, K & Mncube, L, 2007. The impact of growth and redistribution on poverty and inequality in South Africa. DPRU Working Paper 07/126. Development Policy Research Unit, Cape Town.
- Posel, D & Casale, D, 2005. Who replies in brackets and what are the implications for earnings estimates? An analysis of earnings data from South Africa. Proceedings of the Economic Society of South Africa Conference, 7–9 September, Durban.
- Raghunathan, TE, Lepkowski, JM, Van Hoewyk, J & Solenberger, P, 2001. A multivariate technique for multiply imputing missing values using a sequence of regression models. Survey Methodology 27(1), 85–95.
- Seiver, DA, 1979. A note of the measurement of income inequality with income data. The Review of Income and Wealth 25(2), 229–233. doi: 10.1111/j.1475-4991.1979.tb00096.x
- Simkins, C. (2004). What happened to the distribution of income in South Africa between 1995 and 2001? Unpublished paper. http://www.transformationaudit.org.za/research-database/academic-1/labourmarket/2000–2006/smikins/ Accessed 20 January 2015
- Stats SA (Statistics South Africa), 2006. Income and Expenditure Survey 2005/2006: Report on progress made. Statistics South Africa, Pretoria.
- Van der Berg, S & Louw, M, 2004. Changing patterns of South African income distribution: Towards time series estimates of distribution and poverty. South African Journal of Economics 72(3), 546–572. doi: 10.1111/j.1813-6982.2004.tb00125.x
- Van der Berg, S, Louw, M & Du Toit, L, 2007a. Poverty trends since the transition: What we know. Stellenbosch University, Stellenbosch.
- Van der Berg, S, Louw, M & Yu, D, 2008. Post-transition poverty trends based on an alternative data source. South African Journal of Economics 76(1), 58–76. doi: 10.1111/j.1813-6982.2008.00161.x
- Van der Berg, S, Burger, R, Burger, R, Louw, M & Yu, D, 2005. Trends in poverty and inequality since the political transition. Stellenbosch Economic Working Papers: 1/2005. Stellenbosch University, Stellenbosch.
- Van der Berg, S, Burger, R, Burger, RP, Louw, M & Yu, D, 2007b. A series of national accounts-consistent estimates of poverty and inequality in South Africa. Stellenbosch Economic Working Papers: 09/07. Stellenbosch University, Stellenbosch.
- Vermaak, C, 2005. Trends in income distribution, inequality and poverty in South Africa, 1995 to 2003. Proceedings of the Economic Society of South Africa Conference, 7–9 September, Durban.
- Vermaak, C, 2008. The impact of multiple imputation of coarsened data on estimates of the working poor in South Africa. Proceedings of the Development Policy Research Unit (DPRU) Conference, 27–29 October, Muldersdrift, South Africa.
- Von Fintel, D, 2006. Earnings bracket obstacles in household surveys – how sharp are the tools in the shed? Stellenbosch Economic Working Papers: 08/06. Stellenbosch University, Stellenbosch.
- Whiteford, A & McGrath, M, 1994. The distribution of income in South Africa. 1st edn. Human Sciences Research Council, Pretoria.
- Wiseman, V, Conteh, L & Matovu, F, 2005. Using diaries to collect data in resource-poor settings: Questions on design and implementation. Health Policy and Planning 20(6), 394–404. doi: 10.1093/heapol/czi042
- Wittenberg, M, 2010. An introduction to maximum entropy and minimum cross-entropy estimation using Stata. Stata Journal 10(3), 315–330.
- Woolard, I & Leibbrandt, M, 2006. Towards a poverty Line for South Africa: Background note. Southern Africa Labour and Development Research Unit, University of Cape Town, Cape Town.
- Yu, D, 2008. The comparability of Income and Expenditure Surveys 1995, 2000 and 2005/2006. Stellenbosch Economic Working Papers: 11/2008. Stellenbosch University, Stellenbosch.
- Yu, D, 2009. The comparability of Census 1996, Census 2001 and Community Survey 2007. Stellenbosch Economic Working Papers: 21/09. Stellenbosch University, Stellenbosch.