ABSTRACT
The South African wheat industry has been under pressure in recent years from global economic instability and a fast-changing domestic policy environment. This has manifested itself in declining wheat production and profitability at the farm level. Wheat quality plays a key role in wheat buying decisions, with quality improvements correlating negatively with yield and, ultimately, productivity. Yet any new wheat cultivar released for commercial production must still meet the standards of the country’s wheat classification system, which has led to wheat sometimes being imported in the face of a domestic shortfall. This situation has inevitably led to tension within the industry and affected both performance and pricing. Using various data sources and a dynamic linear programming (DLP) approach, this paper takes an in-depth look at the link between wheat quality and yield, with a view to determining the impact of wheat quality standards (ceteris paribus) on the profitability of South African wheat producers. Results indicated that were these classification discrepancies removed, the country-wide effect on net farm income is estimated to range between ZAR606 million and ZAR920 million per annum.
1. Introduction
Over the past couple of decades, the wheat industry in South Africa has been buffeted by strong headwinds both locally and internationally, and today is a virtual shadow of its former self. According to Vink and Kirsten (Citation2000), drastic changes in policy have induced structural changes in the industry, not only affecting the financial position of farmers but also changing land usage patterns, farm sizes and the ecological footprint left by farmers as they face mounting pressure to improve their productivity. The global market also started to play an increasingly important role in the growth trajectory of local wheat production. Set against this changing landscape, South African wheat production has declined significantly in recent years.
Fossati et al. (Citation2010) believe that the declining productivity in the local industry can be ascribed to certain quality-related characteristics of wheat. In these authors’ view, quality characteristics, such as protein content (regarded as one of the key factors influencing wheat buying decisions in South Africa), are negatively correlated with yield and consequently have a negative effect on productivity. Karaman et al. (Citation2009) hold a similar view, asserting that wheat has the defect of conversion (that is, yield declines as quality improves) as a general characteristic. When yield suffers in the face of quality improvements, the demand for wheat in South Africa exceeds available supply. As a result, the necessary quantities of wheat for the local grain milling industry have to be procured from foreign sources of supply (Karaman et al., Citation2009).
Notwithstanding the above, wheat quality plays a critical role in the end product – whether it is bread, biscuits or pasta – and must be closely monitored if consumers are to receive an acceptable product (Engelbrecht, Citation2008). Wheat quality in South Africa is regulated by a so-called wheat classification system, introduced in 1989. Following extensive deliberations between the different role players, a number of primary and secondary characteristics were identified to guide the evaluation of any new wheat cultivar released for commercial production. Part of the evaluation process entails identifying specific wheat cultivars that serve as biological standards, that is, all new cultivar releases should be equal to or better than the biological standards, which helps to improve the quality of the South African wheat crop. The process was designed in such a way as to ensure that cultivars that do not meet consumers’ and end users’ requirements are not released (De Villiers, Citation2004).
However, the process spawned many classification discrepancies which have generated much controversy, notably between the primary wheat producers and the wheat processors. Primary producers see the ‘system’ preventing the release of new cultivars that do not comply with the set biological standards yet it allows processors to import wheat that does not comply with the set biological standards. Imported wheat is then mixed with locally produced wheat to attain a quality that is acceptable to consumers. Another factor contributing to the controversy is that the price for locally produced wheat is determined by the import parity price. Thus, the classification discrepancy between local and imported wheat influences not only the yields of producers, but also the prices farmers receive for their produce.
The question that comes to mind is whether or not the enforcement of a certain wheat quality standard among commercial producers is the cause of the primary wheat sector’s declining competitiveness.
The importance of both quality and yield in the wheat industry means that a balance must be achieved between the two. Because some role players in the wheat industry are naturally quality-driven (buyers) and others naturally yield-driven (producers), there is a constant threat of the one factor being promoted at the expense of the other. In a free market system, this balance is determined by consumer demand for a specific quality of product (Blakeney et al., Citation2009).
Ideally, the prescribed wheat quality must be justified by the demand for such quality in any of the marketing stages in the wheat industry. One can therefore argue that if the current quality prescribed for commercial production is justified by the demand in the market, then the low yield, low productivity and lack of performance among wheat producers in South Africa is also justified. This would reflect free market conditions. However, if the quality prescribed is not justified by the demand in the market, then the lack of yield, productivity and performance among wheat producers is not warranted and modifications to the current criteria must be made and measured. Quantifying the adjustments made can give an indication of the effect of wheat quality standards on the competitiveness of wheat producers in South Africa.
In order to establish whether or not declining wheat production in South Africa can be attributed to prescribed standards of wheat quality, this paper must firstly determine whether or not the quality required for the release of new cultivars for commercial production is justified by the demand for such quality in the market. Only after this has been done can the extent to which the prescribed quality influences the profitability of the South African wheat producers be determined. In order to accomplish this, a farm level, dynamic linear programming (DLP) model was used as it provides a more satisfactory analytical description of whole farm situations over time than most other tools (Louw et al., Citation2007).
2. Literature review
As mentioned, declining productivity and profitability of the South African wheat producers can potentially be linked to the high prescribed wheat quality of new wheat cultivars. However, the first question that must be asked is whether the wheat quality standards can be solely held responsible for the decline in performance, and ‘if so, to what extent?’ To answer this important question, a literature review of studies that quantified this impact must be reviewed.
In South Africa, high standards are set for the release of new wheat cultivars as far as their bread-making characteristics are concerned. Before a new cultivar can be released for commercial production, the quality characteristics are discussed by the Research Technical Committee (RTC). If the cultivar does not comply with the RTC’s quality requirements, its name will not be included on the cultivar list for bakers and millers, and silos will not accept the produce from producers (Du Plessis et al., Citation2005, Citation2008). These criteria do not constitute a regulation, but are rather a suggested framework for producers. Yet the criteria are effectively forced on producers, who face the prospect of buyers not accepting their produce if the cultivar does not appear on the list of accepted cultivars.
For a wheat variety to be approved for commercial production and accepted by silos nationally, the rheological and baking characteristics of the wheat and wheat flour must first be determined. Rheology is the study of the deformation and flow of matter under the influence of applied stress. The different tests that are conducted to determine the rheological and baking characteristics of a specific cultivar are listed in . Once the rheology results are known, the performance of the dough during baking can be predicted (Engelbrecht, Citation2008). The RTC sets strict criteria regarding the rheological and baking characteristics during the final approval stages of potential wheat cultivars in South Africa. The primary rheological and baking characteristics are fixed and non-negotiable, and include characteristics as illustrated in (South African Grain Laboratory [SAGL], Citation2010).
Table 1. Average biological standard for the three production regions in South Africa.
South Africa has three distinct wheat production regions that play a major role in quality variations, with a specific cultivar that sets quality standards in each region. The regions are: the Western Cape, which is suitable for hard red spring wheat production; the Free State, which is suitable for hard red winter (HRW) wheat production; and the Northern Cape irrigation region, which is ideal for hard red spring wheat production (Van Schalkwyk & Van Deventer, Citation2005).
In order to adjust for seasonal variations in quality, cultivars with the desired quality characteristics are used as biological standards for new cultivars and fixed variations of these standards are allowed. The cultivar that is used as the quality standard and prescribed by the millers and bakers in the Western Cape (dry land) is the Kariega cultivar. The Elands cultivar is used as the quality standard in the dry land Free State regions, and the SST806 cultivar is used in the Northern Cape irrigation region. These cultivars are all hard red wheat cultivars and possess excellent milling and bread-making qualities.
Newly developed cultivars are conventionally tested for three years in five different environments in the three main wheat production regions. The cultivars that are used as quality standards in each region are also included in these trials for reference purposes. The South African Grain Laboratory (SAGL) performs the quality analysis. As mentioned, the rheological and baking characteristics of a wheat cultivar play a key role in determining whether or not the cultivar can be released for commercial production. To determine these characteristics, 15 tests are conducted.
depicts data from recent trials of the biological standards of Elands, Kariega and SST806, as well as the variation that is allowed for the release of new cultivars. As can be seen, each cultivar has its own weaknesses and strengths. For instance, the falling number of Elands (350.20 sec) is significantly lower than that of SST806 (443.57 sec) and Kariega (427.33 sec), while the P/L (Stability/Distensibility) value of Elands (1.27) is higher than that of SST806 (0.67) and Kariega (0.77). The deviation allowed shows the maximum variation that can be tolerated for a cultivar to still be released for commercial production. For instance, a maximum of -4 gram from the biological standard will be tolerated for the 1000 kernel mass test.
In order to determine whether the quality required for the release of new, commercially accepted wheat cultivars in South Africa is justified and has an impact on the productivity of the primary wheat industry, three separate comparisons were made by Van der Merwe (Citation2015). In this regard, the quality demanded by three different marketing stages in the wheat industry was compared to the quality of the cultivars prescribed to producers. Furthermore, to quantify the impact on productivity (i.e. performance), the yields of existing cultivars were compared with the yields of cultivars exhibiting the qualities demanded by each of the marketing stages.
2.1. Wheat quality standards and supplied quality
The first comparison looked at wheat quality provided to buyers (millers, bakers, etc.) over a specific period by analysing data obtained from the SAGL. The SAGL analyses local and imported wheat annually to determine the rheological and baking characteristics of all wheat in South Africa, which allows for comparison.
The quality of locallyproduced wheat and imported wheat was used to simulate the wheat quality supplied to buyers over the period in question. The eight-year weighted average wheat quality was calculated by including the characteristics of local and imported wheat.
In comparing the average wheat quality of local and imported wheat to the biological standards in each region as set by the RTC, a somewhat disturbing picture emerged, namely the quality provided to processors and the quality standards prescribed by the RTC were not aligned.
Out of the 15 tests performed by the SAGL, six values in the Elands cultivar were considered to be too strict, while eight and nine values in the SST806 and Kariega cultivars respectively, were considered to be too strict (see Van der Merwe, Citation2015, for a detailed description of these test values).
2.2. Wheat quality standards and flour quality
In order to verify the findings from the first comparison, a survey of existing wheat flour quality that is sold in South Africa was conducted. As the flour had already undergone processing, certain tests could not be conducted. In view of this, the eight-year average quality supplied to processors was used. Similar to before, the flour quality obtained from the tests was compared with all three biological standards representing the major production regions in South Africa.
Out of the 15 tests performed by the SAGL, seven values in the Elands cultivar were considered to be too strict, while 10 values in both the SST806 and Kariega cultivars respectively were considered to be too strict (see Van der Merwe, Citation2015, for a detailed description of these test values).
According to Blakeney et al. (Citation2009), any classification and grading system must reflect both the demand from consumers and the ability of producers to achieve the requisite standards. As has been proved, the quality demanded by consumers is not accurately reflected in the criteria for releasing new cultivars. Therefore, alterations to the existing release criteria for new wheat cultivars are essential if the competitiveness of the primary production sector in South Africa is to improve.
2.3. Wheat quality standards and price in wheat-importing countries
Results from a hedonic price analysis conducted by Van der Merwe (Citation2015) revealed that wheat originating in Argentina, Brazil, Germany and Ukraine, together with other quality factors, had a meaningful impact on price volatility. As mentioned, South Africa is a net importer of wheat, and prices are therefore determined by the import parity price which, in turn, is derived from the price of imported wheat. This means that the price obtained by South African producers is dictated by the wheat imported from Argentina, Brazil, Germany and Ukraine, and these countries’ associated qualities. As a result, the average quality of imported wheat from the mentioned countries was compared with the biological standards in each of the three production regions in South Africa.
Out of the 15 tests performed, 12 values in the Free State dry land cultivar and Western Cape dry land cultivar respectively were considered to be too strict when compared with the new proposed criteria, while 11 values in the irrigation cultivar were considered to be too strict (see Van der Merwe, Citation2015, for a detailed description of these test values).
2.4. Assessment of wheat quality standards studies
In each of the three comparisons, new and more relaxed criteria were derived for the purpose of determining whether or not previously rejected cultivars with higher yields could have been released for commercial production in South Africa. The yields of these cultivars were compared with the yields of already-accepted cultivars to determine the difference in productivity and therefore competitiveness. As pointed out by Blakeney et al. (Citation2009), a delicate balance must be established between the optimal wheat quality and yields – which is ultimately determined by consumer demand.
In comparison 1 (wheat quality standards compared with wheat quality supplied), a total of eight previously rejected cultivars were available and compared with the suggested new criteria. When looking at the dry land Free State region (using the Elands cultivar as the biological standard), one of the eight cultivars could have been accepted if the proposed new criteria had been used. This specific cultivar (WNT 2005/2007) had an average yield of 3.43 tonnes per hectare in the 2006/2007 production season (). Comparing this figure with the yield of 2.7 tonnes per hectare (average yield of accepted cultivars in the Free State production region), a potential increase of 26.43% could have been realised if the criteria had been relaxed.
Table 2. Potential increase in yield if criteria had been relaxed.
By comparing rejected cultivars with the proposed new criteria in the Northern Cape irrigation region (in comparison 1), it was found that two cultivars (BSP09/17 and BSP07/11) would have been acceptable. By comparing the yields of these cultivars with the average yield of the accepted cultivars, an increase of 12.62% could have been achieved if the proposed criteria had been implemented in the irrigation region (see ). Furthermore, there were two cultivars that could have been accepted in the Western Cape production region if the proposed criteria had been implemented, namely W11-02 and W11-04. By comparing the highest yielding ‘new’ cultivar (W11-04) with the average yield in the Western Cape production region, an increase of 7.04% could have been realised if the proposed new criteria had been implemented (see ).
Assuming that 57% of dry land wheat with a potential yield increase of 7.06% was produced in the Western Cape and 24.3% with a potential yield increase of 26.43% was produced in the Free State – all being produced using dry land practices – it can be assumed that wheat yields using dry land practices could have been increased by 12.80% if the proposed new criteria had been implemented. As mentioned, cultivar yields under irrigation, amounting to 18.7% of total wheat produced in South Africa, could have been increased by 12.62% if the proposed new criteria for the release of the new cultivars had been implemented. The average increase in yield over all areas in South Africa was expected to amount to 12.80%.
Unfortunately, due to the absence of additional data on rejected cultivars and their yield potentials, the same cultivars were identified in comparisons 2 and 3. This means that the following two comparisons, though constituting very conservative estimates, support the potential wheat yield increase of 12.80% in South Africa if the proposed criteria had been implemented (see ).
When the release criteria are altered to reflect wheat demanded by the end user, a conservative average estimate of 12.8% increase in South African wheat yields can be realised. This statement is based on the findings from the previous three comparisons. However, according to the wheat breeders (personal consultation, 2013), yields of newly developed cultivars can be improved substantially if the focus is shifted slightly away from the unsubstantiated high-quality requirements (see previous analyses) towards a more balanced approach between yields and quality. Currently, the focus of the wheat breeders is still on providing high-quality wheat rather than on achieving a balance between quality and yields. The impact of reducing wheat quality in line with the current average market supply and demand requirements may therefore be much higher than originally perceived.
In order to simulate the possible impact on productivity, the yields of cultivars with lower-quality characteristics were compared with the yields of the average commercial yield in each production region in the same production season. However, the yields of new cultivars are determined under more intensive production conditions in experimental farms and therefore cannot be directly compared with commercial yields realised in each region. To avoid this problem, a fixed percentage of 20% difference between test result yields and commercial yields was used (personal consultation with wheat breeders, 2014). According to the wheat breeders, a 20% difference in test yields and commercial yields can be expected under normal circumstances. It must be noted, however, that this percentage change was merely a suggestion and many factors could influence the difference in yields.
Comparing the yields of the cultivars that could be accepted for commercial production if the criteria were relaxed in the Free State production region, it is evident that the new cultivars realised a yield of 3.43 tonnes per hectare in the 2006/2007 production season. Subtracting the suggested 20% from this yield, it can be assumed that this cultivar would realise a yield of 2.75 tonnes per hectare, while the average yield in the Free State in that production season was 2.17 tonnes per hectare. Therefore, a potential increase in yield of 26.74% could have been expected with more relaxed criteria ().
Table 3. Potential higher yields if the criteria were relaxed and the focus is shifted to yield.
Comparing the yields of the ‘new’ cultivars, less the suggested 20% in the Northern Cape irrigation region, with the average commercial yield, it can be seen that the average commercial yield would have been higher. It would therefore be advisable to stay with current cultivars in this region. Comparing the average yield of 2.64 tonnes per hectare in the 2011/2012 production season in the Western Cape region to the average yields of ‘new’ cultivars of 2.91 tonnes per hectare (calculated by subtracting 20% from 3.64 tonnes per hectare), it can be seen that a potential yield increase of 21.98% could have been realised if the criteria had been more in line with the quality supplied and demanded in South Africa (see ). Taking into account the number of tonnes produced in each region in the 2012/2013 production season, an average increase in wheat yields of 19.03% across South Africa could have been expected if the criteria for new wheat cultivars in South Africa had been relaxed.
With reference to the second objective of the study, the impact of policies, projects or programmes on the competitiveness of the South African wheat industry (SAWI) need to be analysed. In this regard, quantifying the effect of the mentioned increased wheat yields can give an indication of the effect that the concentrated wheat market has on wheat producers in South Africa. The following section discusses the approach taken to quantify this effect.
3. Methodology
Mathematical programming has become an important and widely used tool for analysing similar impact studies in the agricultural economics environment (Mills, Citation1984, as cited by Buysse et al., Citation2007). The use of mathematical programming optimisation models, such as linear programming, can be seen as a communication-facilitating instrument for the various stakeholders in a changing policy environment – in particular, the farmer and the policymaker (Fernagut et al., Citation2004, as cited in Buysse et al., Citation2007). Linear programming in its simplest form is a method of determining a profit-maximising combination of enterprises which is feasible with respect to a set of fixed constraints (Hazell & Norton, Citation1986).
Applying this approach on the farm level gives a good indication of the impact of the change in production. Furthermore, it is necessary to make explicit allowance for the peculiar influence of time on the structure of the system under study. Of the many ways in which this can be achieved, DLP provides a more complete analytical description of whole farm situations over time than most other tools (Throsby, Citation1962). Louw et al. (Citation2007) hold a similar view, stating that DLP is an extremely useful tool for simulating the farm system and addressing ‘what if’ questions. As a result, Louw et al. (Citation2007) developed a DLP farm-level model. The model’s accuracy and complexity result in a more accurate representation of the real world than a new model with limited resources. With the assistance of Louw et al. (Citation2007), the model was adjusted for the purposes of this study.
Louw and Van Schalkwyk (Citation1998) developed a farm linear planning model in South Africa called OPTIMA, the objective of which is to establish a holistic, user-friendly farm planning model to aid farm planning and extension and the formulation of agricultural policy. During 2000, this basic model was converted from spreadsheet to algebraic formulation. Louw et al. (Citation2007) stated that in regional spatial modelling, the use of DLP models was previously limited by computer capacity and that only in recent years has it become both possible and practical to embed several DLP whole farm models into a spatial framework and solve these models within a reasonable time. According to the authors, this increased the capacity and flexibility of the model substantially. Louw et al. (Citation2007) further stated that the DLP technique, used in this study, has been extensively used in other parts of the world, especially the United States of America (USA), the European Union (EU) and South Africa. This modelling framework is probably the most comprehensive and the largest of its kind ever attempted when using DLP in a spatial context in South Africa (Louw et al., Citation2007).
Risk management in agriculture has become an important part of the management function among primary producers, industry bodies and government alike. Analytical tools used as part of a framework to assist decision makers in formulating risk management strategies can be extremely useful. However, decision makers will only use these analytical tools if their ability to produce better decisions can be proven (Louw et al., Citation2007). The value of this model lies in its ability to calculate the Net Farm Income (NFI) that is generated by a specific set of activities and objectives through cash flow statements. The impact of higher yield on the NFI of farmers can thus be accurately estimated.
3.1. Data used and application of the model
The importance of analysing and applying the information recorded in cash flow statements to make sound farm-related business management decisions cannot be overemphasised. A lack of cash flow is the stumbling block to many plans being realised. Identifying periods in which there is a potential cash flow surplus or cash flow deficit allows the manager to take advantage of opportunities as they arise or to plan for periods when cash is short (Saskatchewan Ministry of Agriculture, Citation2014). As a result, the model places specific emphasis on the effects of externalities on the cash flow statements on a farm level.
In the process of adjusting the model for the purposes of this study, it became evident that differences in input structures between dry land and irrigated wheat production practices resulted in the model behaving differently under these two conditions. The model therefore had to make special reference to each of these conditions. To accomplish this, a dry land wheat farm model and an irrigated wheat farm model were used to simulate increased yields. Although different input structures are required for different dry land regions in South Africa, the effect on results obtained by the model proved to be minor. As a result, a dry land wheat-producing farm in the Moorreesburg area (Western Cape region) was used as an example to determine the effects of increased yields on dry land wheat production regions in South Africa. In an effort to determine the effects of increased yields on irrigated wheat production regions, an example of an irrigated wheat-producing farm in the Douglas region was used. As this model attempts to analyse the average overall effect of the reduced yields, an enterprise budget of each typical farm was calculated based on its average yields, prices and input costs from 2013 to 2015.
A typical dry land wheat farm of 1000 hectares in the Moorreesburg region was used to simulate the possible effects of increased yields, while a 256-hectare farm unit was used to simulate the effects of increased yields in the irrigated regions of Douglas. On these typical farm units, alternative commodities were also produced and the effect thereof was considered. In each case, a base scenario with an unchanged yield, representing the current situation in the specific area, was compared with four additional scenarios, each representing a different yield increase. provides a description of the wheat farm scenarios. As can be seen, the base scenario produced no change to existing cultivar yields, but allowed 50% upwards and downwards variation on areas cultivated.
Table 4. Description of wheat farm scenarios.
The first scenario, which is also the most likely scenario to occur if wheat quality standards were to be adjusted, assumes that wheat yields were increased by 12.80% in all wheat production regions in South Africa. The second most likely scenario was the 19.03% increase in yields represented by Scenario 3, which allows for a 20% increase in wheat yields. Two additional scenarios, representing an increase in yield of 15% (Scenario 2) and 25% (Scenario 4) are included for comparison purposes.
3.2. Theoretical model specification
In an effort to analyse the conversion from a conventional to an organic farming system over time, Acs et al. (Citation2007) developed – similar to Louw et al. (Citation2007) – a DLP model for a typical farm in the central clay region of the Netherlands. The general structure of the DLP model is summarised as follows (Hazell & Norton, Citation1986, as cited in Acs et al., Citation2007):(1)
where:
where: Z is the discounted labour income; t is the year; i is the discounted rate; x stands for the vector of activities; c is the vector of gross margin or costs per unit of activity; f is the vector of fixed costs per year; A is the matrix of technical coefficients; b is the vector of the right-hand side value.
Activities and constraints are included in each period (year) for all the relevant decisions, and many of them are duplicated from one year to the next (e.g. annual crop activities). The link between the years is provided by the conversion of the land area and the objective function (Acs et al., Citation2007).
Although a basic knowledge of the model’s theoretical background is necessary, Louw et al. (Citation2007) stated that the first step in the development of a decision-making framework is to describe the system. As mentioned, this study focuses on the SAWI and the possible impact that concentration in the market may have on the release criteria and subsequently on the productivity and competitiveness of primary wheat producers. The model developed by Louw and Van Schalkwyk (Citation1998) was utilised to determine the exact impact that a decline in productivity of primary wheat producers in South Africa has on the entire industry. The model was thus developed based on a typical wheat farm in South Africa, with special reference to dry land and irrigated production practices.
Louw et al. (Citation2007) stated that the second step in developing a decision-making framework is to create a modelling framework based on the identified ‘system’. This enables decision makers to acquire a better understanding of how the system reacts to impulses from the outside. As this model was developed in 1998 by Louw (Citation1998) and improved since then, the following section gives a brief description of the model and the methods implemented by Louw et al. (Citation2007) to accomplish the above.
3.3. Basic modelling framework
Similar to the model developed by Louw et al. (Citation2007) to determine the effects of irrigated agriculture in the Northern Cape, a DLP model was constructed for the purposes of this study. In this model, a typical farm unit in the dry land and irrigated wheat production regions of South Africa was used to simulate the possible effects of increased production on capitalised investment and net farm income.
According to Louw et al. (Citation2007), the first step in simulating a farm system should be to identify the objectives of the farm unit, the resources available to reach these objectives, and alternative activities that could assist. The essential parts of a whole farm planning model are depicted in .
Figure 1. Typical farm DLP modelling structure. Source: Louw et al. (Citation2007).
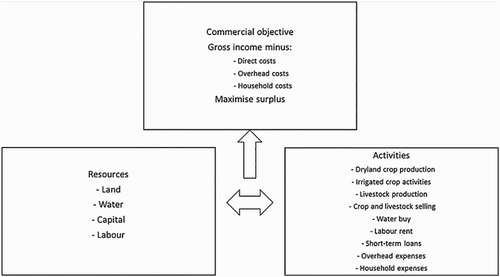
In most cases, a farmer’s principal objective is to make a decent living from farming activities. The approach would be to specify the farmer’s basic or minimum consumption requirements and add these to the fixed costs of each period, thereby making financing of these requirements compulsory. Any surplus could then be reinvested in the business or invested off-farm, and the compounded (future) value of surplus, plus terminal values, could be maximised at the end of the planning period (Louw et al., Citation2007).
The resource restrictions normally included in mathematical modelling (see ) are usually fixed, meaning that they can only be acquired at a cost (Louw et al., Citation2007).
The farm activities represented in make use of scarce resources, while the DLP approach aims to optimise the use of resources through activities that will maximise farmers’ objectives (in quantitative terms). The activities compete for the same resources to generate revenue to pay for operating expenses, interest on loans and household expenses. Any revenue surpluses are available as reserves which can earn interest (Louw et al., Citation2007).
The model was developed in General Algebraic Modelling System (GAMS) and is solved with the CPLEX (IBM ILOG CPLEX Optimization Studio) solver. A database was developed by Louw et al. (Citation2007) for each of the representative farm models. The databases are in Microsoft Excel and were developed in such a way that they can easily be updated. To give the reader some insight into the workings of the typical farm models, typical farm equations are included in the model that was developed by Louw et al. (Citation2007) (see Louw et al., Citation2007, for a detailed description of all these equations).
According to Louw et al. (Citation2007), the objective function of the model specifies that the end balance, minus the sum of discounted expected MEAN outcomes, minus a Risk Aversion Parameter (RAP) multiplied by Fischer’s approximation of the standard error, be maximised. Since the deviations from the mean in year mt are discounted, the SE is also discounted.
Alternatively, it is possible to maximise the annual cash flow instead of the end balance minus the Minimization of Total Absolute Deviations (MOTAD) specifications, which was the case in this study. The objective function basically consists of the following code (Louw et al., Citation2007):
Cost..
+ Endb
*+ sum(mt,Ancashfl(mt))
- SUM((c,b,mt),MEAN(c,mt)*crst(c,b,mt)*LUPB(c,b))
- SUM((a,mt),MEAN(a,mt)*LSTTOT(a,mt))
-rap*SE
=e= Z.
4. Effects of increased yields on the performance of the wheat industry
The performance of an industry can be successfully determined from the profitability of the role players. Smith (Citation2000) stated that agricultural producers are generally concerned about maximising the profitability of their operations while avoiding excessive financial risks.
According to Hofstrand (Citation2009) and Van Zyl et al. (Citation1993), profit is the difference between revenues and costs, and an industry or business can generate a profit through productivity growth or price over-recovery, or both. The particular approach taken has important implications for long-term competitiveness (Van Zyl et al., Citation1993). A constraint to either productivity growth or prices can thus severely affect the performance and competitiveness of an industry. As has been proven, quality standards set by the RTC have negatively affected the productivity of wheat producers in South Africa. However, the impact of low productivity on the economy has not yet been quantified.
According to Louw et al. (Citation2014), calculating the effect of decreased productivity on the NFI can directly measure the effect on the performance of the industry.
4.1. Changes in NFI
NFI accounts are designed to provide an annual measure of income returned to the operators of agricultural businesses from the production of agricultural commodities. The numbers are used to assess the state of the agricultural industry and to form the basis of various policy measures (Statistics Canada, Citation2013).
The NFI of farm businesses is derived by subtracting operating expenses from farm cash receipts. It represents the amount of cash generated by the farm business that is available for debt repayment, investment or withdrawal by the operators (Statistics Canada, Citation2013). depicts the expected increase in NFI on a dry land wheat farm unit of 1000 hectares. Under current conditions (base scenario), it is estimated that this specific farm unit will generate an NFI of approximately ZAR17.8 million over a 20-year period, amounting to ZAR17 807 per hectare over the period or ZAR890 per annum.
Table 5. Expected increase in NFI under dry land conditions.
As can be seen, the 12.80% increase in yield (Scenario 1) on dry land in South Africa resulted in NFI increasing from ZAR17 807 per hectare to ZAR25 911 per hectare over a 20-year period. This amounts to ZAR1296 per hectare per annum, representing an increase of 46%. As can be seen in , NFI per hectare increased from ZAR17 807 to ZAR30 333 with a 20% increase in yields, which represents an increase of 70% compared with the base scenario. Two additional scenarios (Scenario 2 and Scenario 4) are also included in the analysis for reference purposes. If yields were to increase by as much as 25%, NFI would increase from ZAR890 per hectare per annum to an estimated ZAR1668 per hectare, representing an 87% increase in NFI.
shows the expected increase in NFI on an irrigated wheat farm of approximately 256 hectares due to increased yields. Similar to the case of the dry land regions, a base scenario was compared with four different scenarios, each representing different levels of increased yields (see ). As mentioned, Scenario 1 with a 12.80% increase in yield is the most likely scenario to occur if the wheat quality standards are adjusted. As can be seen, the NFI per hectare over a 20-year period will increase from ZAR21 516 to ZAR28 106 under the assumption of Scenario 1. This amounts to ZAR1 405 per hectare per annum, representing an increase in NFI of 31%. The NFI in Scenario 3 (second most likely scenario), representing an increase in yield of 20%, will increase from ZAR21 516 to ZAR30 555 per hectare over a 20-year period. This amounts to ZAR1528 per hectare per annum, representing an increase in NFI of 42%.
Table 6. Expected increase in NFI on irrigated land.
4.2. National effects of increased wheat yields on the performance of the wheat industry
In order to gain a clear understanding of the extent of the impact of market concentration on wheat producers, the total effect in South Africa must be determined. As depicted in and , the farm-level effects of decreased productivity were determined on a per-hectare basis. Multiplying this effect by the total hectares in each region therefore determines the national effect. As can be seen in , the potential increase in NFI in the Free State generated by a 12.80% increase in yield amounts to ZAR152 million per annum, while the effect is much greater in the Western Cape region at ZAR358 million per annum. The increase in NFI in the Northern Cape irrigation region amounts to ZAR95 million per annum. The combined effect in all these regions due to a 12.80% increase in yield is estimated to be ZAR606 million per annum or just over ZAR12 billion over a 20-year period. To put this into context, the NFI generated by the entire agricultural industry in 2012/2013 was ZAR58.9 billion. This means that the strict criteria enforced by the RTC resulted in the NFI generated by the entire agricultural industry shrinking by an estimated 1.03%.
Table 7. Effect of increased yields on NFI on a national scale.
As mentioned earlier, Scenario 3, which represents a 20% increase in yield, is regarded as the second most likely scenario to occur. The effect of the 20% percent increase in NFI amounts to ZAR236 million in the Free State, ZAR553 million in the Western Cape and ZAR130 million in the Northern Cape irrigation region per annum. The total effect thus amounts to an estimated ZAR920 million per annum or approximately ZAR18.4 billion over a 20-year period. If this increase in yield had, in fact, occurred, the NFI generated by the entire agricultural industry could have been 1.56% higher.
5. Conclusion
It was concluded that the DLP method provides a more satisfactory analytical description of whole farm situations over time than most other tools. A DLP farm model, as developed by Louw et al. (Citation2007), was thus used to simulate the possible increases in yields that would most likely occur if the wheat quality requirements were relaxed. Because this study has mainly focused on determining the effect on the performance (profitability) of the wheat industry in South Africa, results from the DLP farm model pertaining to NFI were analysed and discussed.
When comparing the application of the model to a dry land situation and to an irrigated situation respectively, significant differences were seen. As a result, two different analyses had to be performed to improve the accuracy of the model. In both cases, a base scenario – representing the status quo – was compared with four different scenarios, each representing different increases in yield. The results showed that a 12.80% increase in yield generated a 46% and 31% increase in NFI on dry land and irrigated land respectively. A 20% increase in yield generated a 70% and 42% increase in NFI on dry land and irrigated land respectively.
The country-wide effect on NFI was estimated at ZAR606 million per annum due to a 12.80% increase in yield or approximately ZAR18.4 billion over a 20-year period. An estimated ZAR920 million per annum or just over ZAR12 billion over a 20-year period was estimated when yield was increased by 20%.
Knowing that these NFIs could have been realised by wheat producers and that the benefits could have found their way to a struggling wheat industry certainly amplifies the need to give urgent attention to these factors. Moreover, considering the knock-on effects on factors such as job creation, the balance of payments and exchange rates, it prompts the question why government has not intervened as yet. Increasing the competitiveness of the South African wheat industry is a particular priority since many government policies focus on welfare issues, including the problems of unemployment and food insecurity, through the agricultural sector.
Disclosure statement
No potential conflict of interest was reported by the authors.
References
- Acs, S, Berentsen, PBM & Huirne, RBM, 2007. Conversion to organic arable farming in The Netherlands: A dynamic linear programming analysis. Agricultural Systems 94, 405–15. doi: 10.1016/j.agsy.2006.11.002
- Blakeney, AB, Cracknell, RL, Crosbie, GB, Jefferies, SP, Miskelly, DM, O’Brien, L & William, RM, 2009. Understanding Australian wheat quality. wheat quality objectives group/grain research and development corporation, Kingston, Australia.
- Buysse, J, Van Huylenbroeck, G & Lauwers, L, 2007. Normative, positive and econometric mathematical programming as tools for incorporation of multifunctionality in agricultural policy modelling. Agriculture, Ecosystem and Environment 120, 70–81. doi: 10.1016/j.agee.2006.03.035
- De Villiers, JF, 2004. Maize and wheat quality — future industry requirements. Executive Director, Chamber of Milling.
- Du Plessis, L, Du Toit, G, Gebrehiwet, Y, Kirsten, J, Lemmer, W, Meyer, F, Punt, C, Rantho, L, Reynolds, S, Strauss, PG, Troskie, D, Van Deventer, C, Van Schalkwyk, H, Van Schoor, M. and Vink, N. 2005. The competitiveness of wheat production in the Western Cape, South Africa. A report to Grain South Africa.
- Du Plessis, L, Du Toit, G, Gebrehiwet, Y, Kirsten, J, Lemmer, W, Meyer, F, Punt, C, Rantho, L, Reynolds, S, Strauss, PG, Troskie, D, Van Deventer, C & Engelbrecht, M, 2008. Assessment of variance in measurement of hectolitre mass of wheat and maize, using equipment from different grain producing and exporting countries. Presented in partial fulfilment of the requirements for the degree Master of Science in Food Science, Department of Food Science, Faculty of AgriSciences, University of Stellenbosch.
- Engelbrecht, M. 2008. Assessment of variance in measurement of hectolitre mass of wheat and maize, using equipment from different grain producing and exporting countries. Presented in partial fulfilment of the requirements for the degree Master of Science in Food Science, Department of Food Science, Faculty of AgriSciences, University of Stellenbosch.
- Fernagut, B, Nasse, L, Lauwers, L, Buysse, J, Van Huylenbroeck, G, Harmingie, O, Polomé, P & De Frahan, B, 2004. Organisation of knowledge transfer in agricultural policy analysis. In van Huylenbroeck, G, Verbeke, W & Lauwers, L (Eds.), Role of institutions in rural policies and agricultural markets. Elsevier B.V., Amsterdam, 183–96.
- Fossati, D, Brabant, C, & Kleijer, G, 2010. Yield, protein content, bread making quality and market requirements of wheat. Tagung der Vereinigung der Pflfl Anzenzüchter und Saatgutkauflfl Eute Österreichs 61, 179–82.
- Hazell, PBR & Norton, RD, 1986. Mathematical programming for economic analysis in agriculture. Macmillan Publishing Company, New York, NY.
- Hofstrand, D, 2009. Understanding profitability. Ag decision maker. Available online at: http://www.extension.iastate.edu/agdm. Accessed 1 December 2014
- Karaman, S, Cetin, B, Oguzlar, A & Yagdi, K, 2009. Hedonic price estimation for the turkish bread wheat characteristics. Quality & Quantity 43, 895–902. doi: 10.1007/s11135-008-9165-z
- Louw, DB & Van Schalkwyk, HD, 1998. The development of a methodology to determine the true value of water in the berg river basin. Unpublished background report. Water Research Commission.
- Louw, DB, Van Schalkwyk, HD, Grovè, B, & Taljaard, PR, 2007. Market risk, water management and the multiplier effects of irrigation agriculture with reference to the Northern Cape. Final report to the Water Research Commission. Department of Agricultural Economics, University of the Free State.
- Louw, DB, Van Schalkwyk, HD, Grovè, B, & Taljaard, PR, 2014. Market risk, water management and the multiplier effects of irrigation agriculture with reference to the Northern Cape. Final report to the Water Research Commission — Adjusted for the purposes of this study. Department of Agricultural Economics, University of the Free State.
- Mills, G, 1984. Optimisation in economic analysis. George Allen and Unwin Ltd., London.
- SAGL, 2010. Analysis procedure and evaluation norms for the classification of wheat breeders’ lines for the RSA. September 2010 revision.
- SAGL, 2013. Analysis procedure and evaluation norms for the classification of wheat breeders’ lines for the RSA. September 2013 revision.
- Saskatchewan Ministry of Agriculture, 2014. Comprehensive guide to farm financial management: Programs and services. Module 7: Cash flow statement analysis. http://www.agriculture.gov.sk.ca/CGFFM_Cash_Flow_Statement_Analysis. Accessed 12 August 2014
- Smith, VH, 2000. Wheat quality and wheat yields: Trade-offs among price, yields, profit and risk. U.S. Department of Agriculture, Special Report No.5.
- Statistics Canada, 2013. Net farm income. Statistics Canada Catalogue no. 12-591-XWE. http://www23.statcan.gc.ca/imdb/p2SV.pl?Function=getSurvey&SDDS=3473. Accessed 24 October 2013
- Throsby, CD, 1962. “Some notes on ‘Dynamic’ linear programming: A reply,” review of marketing and agricultural economics. Australian Agricultural and Resource Economics Society 30(4), 230–2.
- Van der Merwe, JD, 2015. Quality, pricing and the performance of the wheat industry in South Africa. Submitted for the degree: Philosophiae Doctor. North-West University (Potchefstroom Campus).
- Van Schalkwyk, HD & Van Deventer, CS, 2005. The profitability and competitiveness of the South African wheat industry. Aginfo trading as AMT (PTY) LTD, Pretoria.
- Van Zyl, J, Van Schalkwyk, HD & Thirtle, C, 1993. Entrepreneurship and the bottom line: how much of agriculture’s profits is due to changes in price, how much to productivity? Agrekon 32(4), 223–9. doi: 10.1080/03031853.1993.9524746
- Vink, N & Kirsten, J, 2000. Deregulation of agricultural marketing in South Africa: Lessons learned. The Free Market Foundation, Sandton. Monograph 25.