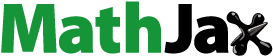
ABSTRACT
Within a broad framework for comprehensive advancement, the Sustainable Development Goals (SDGs) directly address the importance of safe transport to human development. We analysed some 29 000 crashes recorded in Namibia between 2012 and 2015 to identify connections between road safety and sustainable development there and, by extension, throughout Sub-Saharan Africa and other developing countries. The results indicate that the heavy reliance on transport services for basic mobility, especially in rural areas, contributes to negative development outcomes in terms of significant burden of injuries and death, many of which affect younger people of prime working age. The results also suggest that development pressures such as increased commercial traffic and more private vehicles increase the burden of roadway crashes. Many of the underlying factors contributing to these crashes are attributable to driving behaviours and safety cultures that must be addressed as part of programmatic socioeconomic development in order to mitigate the negative effects of transportation and more fully realise its potential to support sustainable development. We document additional ways in which the provision of safe transport is interrelated to successful attainment of the broader SDGs including those not explicitly addressing safe transport such as 4, 5, 8, 9, 16, and 17.
1. Introduction
Over the course of the twentieth century, the global community began focusing its attention on the idea of international development, much of which centred around the concept of economic development. After important events such as the 1972 United Nations Conference on the Human Environment, and the 1992 first UN Conference on Environment and Development (UNCED) in 1992, the international development community begin to focus on the concept of ‘sustainability’. This momentum let to milestones such as Agenda 21 and the 1997 Kyoto Climate Agreement that further integrated the sustainability concept into the international development priorities, policies, and programmes (Allen & Thomas, Citation2000). In 2015, the global development community followed the lead of the United Nations Development Program (UNDP) as it introduced the Sustainable Development Goals (SDGs) to follow the original globally-focused Millennium Development Goals (MDGs). Building on its foundational pillars (social, environmental, economic), the UNDP set out 17 goals articulated though 169 targets by which progress towards each would be measured. The SDGs represented an integrated approach to development in which multiple, sometimes competing, efforts must be balanced to achieve comprehensive progress (Sachs, Citation2012; United Nations, Citation2015).
Within a broad framework for comprehensive advancement, the SDGs recognised safe transport as essential to development – whereas the original MGDs did not do so. In particular, SDGs 3 and 11 directly address the importance of safe transport to the human development endeavour as set out in the following targets:
Target 3.6 – ‘By 2020 halve global deaths and injuries from road traffic accidents’
Target 11.2 – By 2030, provide access to safe, affordable, accessible and sustainable transport systems for all, improving road safety, notably by expanding public transport, with special attention to the needs of those in vulnerable situations, women, children, persons with disabilities and older persons. (United Nations, Citation2015)
2. Development context
It is well documented that transport plays an essential role in human development (e.g. Stewart, Citation1988; Turner & Kwakye, Citation1996; Gannon & Liu, Citation1997; Gakenheimer, Citation1999; Limao & Venables, Citation2001; van de Walle, Citation2002; Bryceson et al., Citation2008; Banjo et al., Citation2012; Laird & Mackie, Citation2014; Porter, Citation2014; Beuran et al., Citation2015; Martincus et al., Citation2017). Several recent high-level studies, however, have documented relationships between the global increase in motorised travel and road crashes (Sperling & Claussen, Citation2004; IHME, Citation2013; Lamont, Citation2013; GRSF, Citation2014; World Bank, Citation2017). The World Health Organization (WHO) recently reported that some 1.3 million people die in traffic crashes each year (WHO, Citation2018). Thus, the need for Target 3.6 and the explicit reference to ‘safe’ transport in Target 11.2 within the SDGs.
2.1. Sub-Saharan Africa
Globally, the burden of death and injury caused by crashes is disproportionally borne by low- and middle-income countries (LMICs). LMICs bear some 90 percent of global road deaths and sub-Saharan Africa (SSA) is particularly impacted (Nantulya & Reich, Citation2002; Nantulya & Reich, Citation2003; Ameratunga et al., Citation2006; Lagarde, Citation2007; Chen, Citation2010; IHME, Citation2013; Onywera & Blanchard, Citation2013; GRSF, Citation2014; Wegman, Citation2017). In many cases, young people bear the burden of crashes most (Adesunkanmi et al., Citation2000; Nantulya & Reich, Citation2002; Okeniyi et al., Citation2005; Ackaah, Citation2010; Burrows et al., Citation2010; Kiser et al., Citation2012; Zimmerman et al., Citation2012; Alonge & Hyder, Citation2013) with an unfortunate impact on otherwise economically productive youth (e.g. Odero et al., Citation1997; Odero et al., Citation2003; Naddumba, Citation2004; Hyder et al., Citation2008; Nakitto et al., Citation2008). Indeed, the WHO (Citation2018) reported that crashes are the leading cause of death among people 15–29 years old and thus have a significant negative impact on the human capital and potential of developing economies. In recognition of the broad challenges of sustainable development in Africa, Njenga & Odero (Citation2014) documented the relevance of transport to meeting the SDGs, noting the particular importance of providing safe mobility and accessibility. Njenga & Odero (Citation2014) mapped the relevance of transport to all of the SGDs and individual targets defining each. In doing so, they emphasised the explicit connections to targets 3.6 and 11.2 noted above. They went considerably farther, however, to describe how the provision of safe transport is essential to supporting socioeconomic activity through access to markets, jobs, education, etc. that reduce poverty and hunger. They report how transport is essential to accessing healthcare and that accessing socioeconomic activity through transport is essential to individual opportunities that support gender equality and community stability, of which contribute to the comprehensive sustainable development set out in the SDGs.
2.2. Namibia
The WHO estimates that the annual death rate due to crashes in Namibia is 30.4 per 100 000 persons. Indeed, from a traffic safety perspective, Namibia is one of the most dangerous countries in SSA where the average fatality rate across the region is 26.6 road deaths per 100 000 persons – by comparison the U.S. is around 12 and Norway is less than 3 annual road deaths per 100 000 persons (WHO, Citation2018).
presents a snapshot of road safety across SSA compiled from the most recent WHO data (WHO, Citation2018). It can be seen that only eight (shaded in grey) of the countries listed have higher road deaths per 100 000 population than Namibia. Interestingly, the experience in North Africa is quite varied with Libya at 26.1 road deaths per 100 000 population (just at the average for the continent), Morocco at 19.6 and Egypt at 9.7.
Table 1. Comparison or road safety across sub-Saharan Africa (WHO, Citation2018).
The table also shows the percentage of road deaths among drivers and passengers of cars and light duty vehicles. While this does not tell the whole story, it is interesting to see the contracts across the continent. For example, some countries exhibit relatively high percentages of road deaths attributable to these modes (e.g. Angola, Botswana, South Africa). By contrast, countries in other parts of the continent where two- and three-wheelers are more prominent exhibit more deaths attributable to these modes.
The table also shows mixed results for whether the five-year trend in road deaths is increasing or decreasing – Namibia is one of the 17 nations with an increasing trend reported. Local sources report that the number of road deaths increased by some 22% from 598 to 731 between 2012 and 2016 (MVA, Citation2016). In addition to the human tragedy associated with deaths and injuries, crashes impact the economic potential of Namibia through lost productivity and elevated insurance costs (Forsyth, Citation2016; MVA, Citation2016).
3. Methodology
We apply statistical analysis to examine underlying relationships among factors contributing to crashes across Namibia. Statistical analysis of crash data is typically conducted using some form of logistic regression such as: multinomial logit models, probit models, tobit models, mixed logit models, latent class models, etc. (Savolainen et al., Citation2011; Mannering & Bhat, Citation2014). Crash records are complex and typically contain a wide range of parameters describing the event in addition to the conditions and circumstance contributing to its occurrence including: crash type, location, time, weather conditions, vehicles involved, demographic information of persons involved. With such a wide range of data and the potential for correlations among them, recent advances in safety analysis have focused on the need to account for unobserved heterogeneityFootnote1 (Mannering et al., Citation2016). Latent class multinomial (LC-MNL) analysis has been shown to effectively account for unobserved heterogeneity across a range of traffic safety data (e.g. Greene & Hensher, Citation2003; Cerwick et al., Citation2014). While superior to the popular random parameters MNL in its ability to address unobserved heterogeneity, the LC-MNL approach used in this study did not account for random parameters within the latent classes as none of the individual variables were found to significantly vary within classes. Nonetheless, the ability to group explanatory data exhibited by the LC-MNL allowed for a richer interpretation of the estimated model results than would be derived from other MNL models. As such, we apply the LC-MNL modelling approach to Namibian crash data to investigate relationships among a range of factors defining individual crash events and the type of transport modes involved.
3.1. Data
Crash data from 2010–12 was obtained from the Namibian National Road Safety Council (NRSC). The crash data comprised records of individual crashes expressed as 60 individual parameters. These crash parameters (i.e. variables) provide specific information across ma range of conditions related to the crash. For example, demographics (age, gender, nationality) of individual involved in the crash are recorded along with any individual parameters that may have contributed to the crash such as a driver being under the influence of alcohol or driving without a valid license. Other parameters convey the conditions under which the crash occurred such as weather, roadway type (paved or unpaved), whether or not it was dark or light conditions and, if dark, whether lighting was present, etc. The data also contained information on what the responding officer determined to be the factors contributing to the crash such as whether or not a vehicle was speeding or violated a traffic control device (e.g. ran a stop sign). All of the parameters in the data were examined for quality (missing values, etc.) and cleaned to ensure consistency in the coding of parameters among record years. In some cases, entire parameter categories (e.g. drink driving, license validity, seat belt usage) were removed from the data set due to inconsistent reporting. In other cases, parameters were grouped logically to increase the overall number of usable crash observations – for example, road surface types such as dirt, sand, and gravel were combined into a single parameter category defined as unpaved. Additionally, information was obtained from the Namibia Weather Network (Citation2017) to replace missing data in the crash records. In other words, the date and time of an individual crash was used to look up the weather conditions (e.g. raining or not) when the crash occurred. The weather data was then used to identify the ‘rainy season’ in Namibia, which typically occurs in January, February, and March.
Vehicle type data were aggregated to create three vehicle mode categories; privately-owned vehicles (PV), transport service vehicles (TSV), and goods vehicles (GV). In most cases, the categorisation of vehicle types was obvious (e.g. motor cars were designated as privately-owned vehicles, while sedan taxis and buses were assigned to the transport service category). Inspection of the crash data, however, indicated that commercial vehicle types defined as ‘light delivery vehicle’ and ‘panel van’ were often recorded carrying passengers. In other words, the crash records indicated that one or more passengers (in a few cases as many as 10) were injured when a light duty vehicle or panel van crashed. This phenomenon of passengers riding in small goods-carrying vehicles (often along with whatever goods are being transported) is typical across sub-Saharan Africa (e.g. Starkey et al., Citation2013; Jones et al., Citation2016; Jones et al., Citation2016). As such, these two vehicle types were included in the transport service category while the goods vehicle category accounted for only large trucks more typically used for commercial purposes such as hauling commodities between cities and to and from the port facilities on the coast. The final transport mode categories are summarised in .
Table 2. Transport modes by Assigned Category.
The three vehicle types also allow the capture of interesting development-related information. For example, examination of crashes involving private vehicles is linked to the rapid motorisation that is currently happening in emerging economies as incomes rise and these vehicles become increasingly available. Goods vehicles are obviously important to development as increased truck traffic is typically associated with increased levels of economic activity. And finally, transport service vehicles are of critical importance in sub-Saharan Africa as they are a primary means of transport, especially for low income persons, women, children, elderly, and those not able to access private vehicles or participate in freight-based transport sectors of the economy (Bryceson & Howe, Citation1993; Porter, Citation2014; Berg et al., Citation2017). It is also worth noting that, although important in other parts of Africa, motorcycles were excluded from the current analysis as they did not represent a significant percentage of crashes in the Namibian data.
The final dataset contained a total of 29 437 individual crash observations including 1849 (6%) serious crashes (comprising fatalities and incapacitating injuries) distributed among the three types of transport modes. The data indicated that PV were involved in 49% of reported crashes while TSV and GV accounted for 47% and 4% of crashes, respectively. The crash data contained over 60 explanatory variables for each individual crash observation. All were initially in the included modelling process to determine which individual variables relate significantly to crashes involving each of the three transport modes.
3.2. Modelling
An LC-MNL model was estimated from the data to ascertain what factors contributed to crashes involving the three vehicle types. The LC-MNL model formulation assumes that outcomes depend on unobserved heterogeneity which is captured by the membership of distinct classes (Mannering & Bhat, Citation2014). As applied herein, the model allows each transport mode to have C different classes so that each distinct class will contain parameters with the probability given as:(1)
(1) where
represents a vector containing probabilities of
for crash
,
is the possible classes
, and
represent the estimable parameters (class specific parameters). The probability of a crash involving transport mode
is given by:
(2)
(2) where
is the probability of crash involving transport mode
for crash
in class
. Based on the Equations (1) and (2), we estimate the LC-MNL model for the three transport modes in l for class
as follows:
(3)
(3) w here
represents the possible number of transport mode types and
is a class-specific parameter vector that takes a finite set of values.
Seventeen of the 60 original crash parameters were found to be significant in the final model. The descriptive statistics of the modelled variables are presented in . The values presented in indicate the proportion (i.e. percentage) of the data coded as 1 in the dataset and the standard deviation gives an information of the degree of balance in the data – this format is commonly employed to provide information on the characteristics of modelled binary variables (e.g. Adanu et al., Citation2017).
Table 3. Descriptive statistics of modelled variables.
As indicated previously, some 6% of crashes recorded were serious injury crashes as reflected in (Mean = 0.063). Further inspection of yielded additional observations about the nature of crashes in Namibia. For example, 74% of total crashes between 2010 and 2012 were attributable to drivers under the age of 30 and only 17% of crashes were caused by female drivers. More than 8% of crashes were caused by drivers from outside Namibia. Other particularly interesting observations include:
Three quarters (Mean = 0.745) of crashes occurred in built-up areas. In other words, these crashes happened in and around cities, towns, and villages. Such crashes are often related to increased traffic levels in built-up areas and are typically less severe. Indeed, it appears that 25% of all crashes were rear-end crashes that are typically associated with traffic congestions or stop-and-go driving near intersections and most often do not result in serious injuries.
Over half of crashes (54.8%) were coded as non-intersection crashes indicating that they occurred on sections of roadway in between major intersections and junctions. Intersection-related crashes include very serious right-angle crashes associated with turning movements within the intersection and/or drivers violating traffic control devices (i.e. running stops sigs and red lights). They also include rear-end crashes as noted above. Non-intersection crashes typically include such serious events such as run-off-road, head-on, and rollover crashes as well crashes where objects or animals in the roadway are involved. Unfortunately, crashes involving vulnerable road users (pedestrians, cyclists, motorcyclists, and animal-powered modes) occur under both intersection and non-intersection conditions and often result in serious injuries.
More than 13% of crashes occurred under low lighting conditions (i.e. night, dawn/dusk). Lack of roadway and intersection lighting, coupled with a lack of adequate signage, could possibly explain the nearly 12% of crashes happening on one-way roads – although driver behaviour (i.e. lack of awareness, risk taking) is likely a factor as well.
Around 15% of total crashes occurred on unpaved roads. And perhaps not unrelated, almost a quarter of crashes were recorded during the rainy months of January through March.
In order to more fully appreciate the significance of these parameters and the interactions among them, the LC-MNL model results must be inspected.
4. Results
The final LC-MNL model yielded three separate classes of parameters contributing to crashes among the three transport modes (PV, GV, and TSV). The model variables were found to be significant at the 0.05 level and the overall model yielded a McFadden Pseudo R-Square of 0.289, indicating reasonably good explanatory power for a latent class model. The results of the LC-MNL model (estimation parameters and fit statistics) are summarised separately for private vehicles, goods vehicles, and transport service vehicles in Tables 4 though 6, respectively. It can be observed that the probability of relationships in Class 1 is 0.62, whereas the probabilities for Class 2 and Class 3 are 0.27 and 0.11, respectively. The results of a latent class model can be interpreted such that relationships found in Class 1 are more likely to occur (i.e. within 62% of observations), whereas those in Class 3 are the least likely (11%). When latent class parameter estimators exhibit different signs (i.e. positive and negative), that gives additional confidence in the results implying there is no remaining unobserved heterogeneity for that parameter. Key observations of factors influencing crashes among the three transport modes are illustrated below and a general discussion within the context of development in sub-Saharan Africa follows.
The positive estimators in Class 1 and 3 in indicate that female drivers contributed to about 38% of crashes involving private vehicles. Furthermore, the female driver parameter estimates found to be significant (i.e. Class 2 and Class 3) are both positive. Whereas the single-carriage way (i.e. two-lane road) parameter was found to positively contribute to crashes in 73% (Class 1 and Class 3) of private vehicle crashes, it also appears to reduce the occurrence of crashes in 27% of cases as suggested by Class 2. Perhaps this is attributable to the fact that single-carriage ways outside of built-up areas (i.e. rural areas) may contribute more to crashes as a result of higher speeds generally observed on such roads – while single-carriage ways in or in the immediate vicinity of built-up areas may exhibit lower speeds and thus less propensity for crashes. A similar pattern of unobserved heterogeneity can be seen for the rear-end crash parameter. First of all, it is interesting to note that showed that rear-end crashes comprised 25% of all crashes while indicates they account for 73% of crashes caused by private vehicles. then goes on to show that driving during the weekend contributed to 73% of private vehicle crashes and that driving on a paved road reduced the chance of private vehicle crashes by 27%.
Table 4. Private Vehicle crash model estimation results (standard error in parentheses).
Another very interesting aspect of latent class models is what can be inferred by the intra-class grouping. Class 1 indicates a relationship among driving on single-carriage way, driving on the weekend, and rear-end crashes for private vehicles in 62% of modelled observations. Whereas, Class 3 contains the same parameters with the addition of female drivers across 11% of modelled observations.
Perhaps the most striking result from is that drivers from outside Namibia contributed to nearly 90% of goods vehicle crashes. Younger drivers contributed to 11% of goods vehicle crashes. Being in a built-up area contributed 27% percent of goods vehicle crashes implying that the majority of these crashes were on the open roads outside of cities, towns, and villages. The non-intersection parameter appears to contribute to goods vehicle crashes in 11% of observations. As might be expected, the majority of goods vehicle crashes occur during the work week (Monday – Thursday) and night conditions appear to only contribute to 27% of goods vehicle crashes.
Table 5. Good Vehicle crash model estimation results (standard error in parentheses).
reveals a very interesting finding in that crashes involving transport service vehicles resulted in serious injury in almost 90% of observations (Class 1 and Class 2). It is interesting to note that young drivers contributed to 62% percent of TSV crashes and that low-lighting conditions (i.e. night or dusk/dawn) contributed to the same percentage. Built-up conditions contributed to only 27% (Class 2) of transport service vehicle crashes indicating that the majority of crashes involving transport services likely happen in rural areas of Namibia. The relative dangers of rural transport services in Namibia is further underscored by the fact that unpaved conditions contribute to 90% of transport service vehicle crashes and the open road (i.e. non-intersection) conditions contribute to 73%. The unpaved roads that are typically found in rural areas may be impacted by rainy conditions as these are shown to contribute to 73% (Classes 1 and 3) of transport service vehicle crashes. And crashes that occur on the open road in rural areas are more likely to happen at higher speeds than those in built-up areas or at intersections. Such conditions may contribute to increased serious crashes especially in light of the reduced access to emergency response and medical services common to rural areas.
Table 6. Transportation Service Vehicle crash model estimation results (standard error in parentheses)
5. Development relevance
The following sections present a discussion of how the results of the Namibian crash analyses relate to sustainable development there. Specifically, the factors found to be contributing to crashes in Namibia are presented in terms of how they relate to important currents of transportation-related development such as reliance on transport services for basic mobility, increased pressures from goods movements, and a rapidly growing fleet of privately owned cars and trucks. Where appropriate, findings are extended to the broader sustainable development context.
5.1. Transport services
While much of the transport planning and development literature emphasise the importance of transport services in providing basic mobility and accessibility, they are too often a dangerous mode of travel. The Namibian crash data indicate that crashes involving transport services vehicles are more likely to be serious than crashes involving the other two modes. The finding that younger drivers have such an impact on the safety of transport service users is quite interesting. For one, it underscores issues related to licensing, training, and overall behaviour of these drivers and the apparent importance of transport services as a source of employment for these individuals.
The relative riskiness of young drivers worldwide is well documented (e.g. Jonah, Citation1997; Møller & Haustein, Citation2014) and it is no different throughout SSA (e.g. Azestop, Citation2010; Porter et al., Citation2010; Sinclair, Citation2013; Amweelo, Citation2016). This is especially true in the instance of drivers participating in informal transport sectors and some researchers have even documented specific psychological conditions within these driver populations (Møller & Gregersen, Citation2008; Ulzen et al., Citation2018). In many cases, transport service vehicles across Africa are poorly maintained and ill-equipped (van Garenne et al., Citation2001; Akloweg et al., Citation2011).
Transport services, however, are essential to sustainable development (Turner & Kwakye, Citation1996; Bryceson et al., Citation2003; Too & Earl, Citation2010; Porter, Citation2014). They are also often the primary form of mobility (other than walking) for many rural women and children who typically do not have access to private vehicles (Porter, Citation2002, Citation2014; UN Women, Citation2015). As such, improvements in rural transport services have the potential to not only address targets 3.6 and 11.2 of the SDGs but also those that explicitly address the advancement of women and children. Goal 5, and the need for safe public spaces set out in target 11.7 (Nhamo et al., Citation2018). The essential mobility afforded by transport services also supports inclusion of elderly populations as contained in target 10.2 (Oppong, Citation2006; Porter, Citation2014; UNDP, Citation2016).
There are enormous possibilities for job creation and innovation (Goals 8 and 9) in the provision of safe transport such as the safety-promoting, mobile phone-based ridesourcing app SafeMotos recently launched in Rwanda (Ismail, Citation2016; Roberts & Lall, Citation2019). Also, such formal promotion of a positive safety culture would specifically contribute to Target 8.8 to ‘promote safe and secure working environments … ’ and support the use of sustainable infrastructure for ‘economic development and human well-being’ as set out in Target 9.1.
5.2. Goods movement
There were two significant findings related to goods vehicle crashes in Namibia. The first is the involvement of younger drivers. Many of the issues elucidated above apply equally to the behavioural issues compounded by lack of education, training, and enforcement of these drivers when transporting various commodities within and across Namibia, often in large trucks – again the importance of participation in the transport workforce for these younger people is underscored. Of particular interest, however, is the role non-Namibians play in goods vehicles crashes. As a country with a robust mining industry itself and one on the coast serving oil-rich Angola, and its landlocked mineral producing neighbours, Botswana and Zambia, as well as economically active South Africa, Namibia accommodates substantial amounts of regional freight traffic (Savage et al., Citation2013; Roberts, Citation2014). The fact that this freight traffic evidently has an adverse impact on road safety in the country is alarming and poses a development challenge.
The challenges and opportunities of providing sustainable transport services (e.g. driver training, technological innovation, and corruption mitigation) relate equally to the movement of goods across Namibia as well and other developing economies (e.g. Maldonado et al., Citation2002; Kircher & Andersson, Citation2013). As such, goods movements place pressures on and can be improved by the same SDGs as the transport service sector.
5.3. Private motorisation
Finally, although not a source of the most serious crashes in Namibia, private vehicles accounted for almost half of the overall crashes recorded during the 2010–12 study period. The number of motor vehicles is rapidly increasing in Namibia and elsewhere in the developing world. The very driving behaviours and unsafe driving cultures that plague heavily motorised countries will no doubt continue to rise in Namibia and other developing countries if left unchecked as new private vehicles are constantly added to the roads. And while the private vehicle crashes were not the primary source of serious injury crashes, even non-serious crashes negatively impact development in terms of direct and indirect costs to society through insurance payouts, time spent resolving crash-related issues, time spent in crash-induced traffic congestion, lost productivity, etc.
Again, the role of behaviours and safety culture are evident. As the prevalence and use of private vehicles grows in Namibia, the exposure of drivers and passengers to potential crashes will also increase. While driving behaviours and related safety culture issues (e.g. corruption) must be addressed, the increased use of private vehicles will require community outreach and education to encourage passenger safety – whether through programmes to enhance seat belt usage and child restraint devices or, in countries where motorcycles are prevalent, the availability and use of helmets. The responsibility for such efforts will fall far beyond that of transport professionals and law enforcement and require a holistic approach involving educators, social workers, policy makers, public relations and marketing businesses, and government agencies at all levels.
5.4. General
The provision of safe transport is essential to meeting the SDGs. In addition to the targets where safe transport is explicitly addressed, the role it plays in creating and supporting social and economic opportunities for all are clear. In order to successfully realise these, however, the importance of transport safety and the issues elucidated above (e.g. driver training, vehicle maintenance and repair) need to be explicitly addressed in Target 4.4 of the SDGs wherein the need for relevant technical and vocational skills is asserted. It could even be said that the call in Target 4.7 to ensure ‘ … all learners acquire the knowledge and skills needed to promote sustainable development … ’ should promote a culture of safety inasmuch as it does ‘peace and non-violence’. Furthermore, Target 4A implores a safe learning environment that must include sensitivity to the well-documented road safety hazards facing school children worldwide (e.g. Hyder et al., Citation2008; Ackaah, Citation2010; Alonge & Hyder, Citation2013).
The widespread and nefarious impacts of corruption also links Targets 16.5 and 16.6 to the provision of safe transport (Lagarde, Citation2007; Hua et al., Citation2010; Jones et al., Citation2016; Jones et al., Citation2016; Peiffer & Rose, Citation2018). Whereas, safe and inclusive transport is an essential component in mitigating fragility and promoting resilient, sustainable communities in Africa as broadly set out in SDG 16 (Jones et al., Citation2019). And finally, the ability to conduct the current study in Namibia and present the findings within a development context was dependent on the availability of quality data – the importance of which is noted in Target 17.18 of the SDGs,
6. Conclusion
Based on the data for the period 2010–12, a latent class multinomial regression model was estimated for three categories of transport modes: Private Vehicles, Goods Vehicle, and Transport Service Vehicles. The purpose of the study was to identify and perhaps better understand factors contributing to crashes in Namibia and how they relate to sustainable development.
The findings of this study reveal that in spite the significant role of transport service vehicles in the developmental of Namibia and other developing countries, the costs (i.e. loss of life and attending socioeconomic impacts) of traffic crashes involving these vehicles can potentially offset development gains. The observation that younger drivers have such an impact on the safety of transport service users and goods movement is quite interesting. This underscores issues related to licensing, training, and overall behaviour of these drivers and the apparent importance of the transport sector as a source of employment for these individuals. Also, the number of motor vehicles is rapidly increasing in Namibia and elsewhere in the developing world. The very driving behaviours and unsafe driving cultures that plague heavily motorised countries are likely to rise in Namibia and other developing countries if left unchecked as new private vehicles are constantly added to the roads. This study has ultimately revealed that the underlying factors contributing to crashes in Namibia are largely attributable to driving behaviours and safety cultures. The study also documented the importance of safe transport to achieving sustainable development in Namibia, especially as expressed in the SDGs.
Disclosure statement
No potential conflict of interest was reported by the authors.
Correction Statement
This article has been republished with minor changes. These changes do not impact the academic content of the article.
Notes
1 Unobserved heterogeneity implies some level endogeneity in the data (i.e., explanatory variables correlated with regression error terms) that can be attributed to (a) missing or omitted parameters or (b) errors or simultaneity at the time of measurement (Mannering et al, Citation2016).
References
- Ackaah, W, 2010. Road traffic fatalities among children in Ghana. Injury Prevention 16(S1), A70.
- Adanu, K, Smith, R, Powell, L & Jones, S, 2017. Multilevel analysis of the role of human factors in sub-regional disparities in crash outcomes. Accident Analysis and Prevention 109, 10–7.
- Adesunkanmi, A, Oginni, L, Oyelami, O & Badru, O, 2000. Road traffic accidents to African children: Assessment of severity using the injury severity score (ISS). Injury 31(4), 225–8.
- Akloweg, Y, Hayshi, Y & Kato, H, 2011. The effect of used cars on African road traffic accidents: A case study of Addis Ababa, Ethiopia. International Journal of Urban Sciences 15(1), 61–9.
- Allen, T & Thomas, A, 2000. Poverty and development into the 21st century. Oxford University Press, Oxford, UK.
- Alonge, O & Hyder, A, 2013. Reducing the global burden of childhood unintentional injuries. Archives of Disease in Childhood 99, 62–9.
- Ameratunga, S, Hijar, M & Norton, R, 2006. Road-traffic injuries: Confronting disparities to address a global-health problem. The Lancet 367(9521), 1533–40.
- Amweelo, M, 2016. The road safety in Namibia: Focus on road traffic accidents. International Science and Technology Journal of Namibia 7, 103–19.
- Azestop, J, 2010. Social Justice approach to road safety in Kenya: Addressing uneven distribution of road traffic injuries and deaths across population groups. Public Health Ethics 3(2), 114–27.
- Banjo, G, Gordon, H & Riverson, J, 2012. Rural transport. improving its contribution to growth and poverty Reduction in Sub-Saharan Africa. SSATP, African transport policy program, working Paper No. 93. The International Bank for Reconstruction and Development/World Bank, Washington, DC.
- Berg, C, Deichmann, U, Liu, Y & Selod, H, 2017. Transport policies and development. The Journal of Development Studies 53(4), 465–80.
- Beuran, M, Gachassin, M & Raballand, G, 2015. Are there myths on road impact and transport in sub-Saharan Africa? Development Policy Review 33(2), 673–700.
- Bishai, D, Quresh, A, James, P & Ghaffar, A, 2006. National road casualties and economic development. Health Economics 15, 65–81.
- Bryceson, D & Howe, J, 1993. Rural household transport in Africa: Reducing the burden on women? World Development 21(11), 1715–28.
- Bryceson, D, Mbara, T & Maunder, D, 2003. Livelihoods, daily mobility and poverty in sub-S3aharan Africa. Transport Reviews 23(2), 177–96.
- Bryceson, D, Bradbury, A & Bradbury, T, 2008. Roads to poverty reduction? Exploring rural roads’ impact on mobility in Africa and Asia. Development Policy Review 26(4), 459–82.
- Burrows, S, van Niekerk, A & Laflamme, L, 2010. Fatal injuries among urban children in South Africa: Risk distribution and potential for reduction. Bulletin of the World Health Organization 88(4), 267–72.
- Cerwick, D, Gkritza, K, Shaheed, M & Hans, Z, 2014. A comparison of the mixed logit and latent class methods for crash-severity analysis. Analytic Methods in Accident Research 3-4, 11–27.
- Chen, G, 2010. Road traffic safety in African countries - status, trend, contributing factors, countermeasures and challenges. International Journal of Injury Control and Safety Promotion 17(4), 247–55.
- Forsyth, S, 2016. Blood on the roads: The importance of improving road safety in Namibia. The Namibian, 2 August.
- Gakenheimer, R, 1999. Urban mobility in the developing world. Transportation Research Part A: Policy and Practice 33, 671–89.
- Gannon, A & Liu, Z, 1997. Poverty and transport. Discussion paper, TWU papers TWU-30. World Bank, Washington, DC, USA.
- GRSF (Global Road Safety Facility, The World Bank; Institute for Health Metrics and Evaluation), 2014. Transport for health: The global burden of disease from motorized road transport. IHME, Seattle, WA; The World Bank, Washington, DC.
- Greene, W & Hensher, D, 2003. A latent class model for discrete choice analysis: contrasts with mixed logit. Transportation Research Part B: Methodological 37(8), 681–98.
- Hua, L, Noland, B & Evans, A, 2010. The direct and indirect effects of corruption on motor vehicle crashes. Accident Analysis and Prevention 42, 1934–42.
- Hyder, A, Muzaffar, S & Bachani, A, 2008. Road traffic injuries in urban Africa and Asia: A policy gap in child and adolescent health. Public Health 122(10), 1104–10.
- IHME (Institute for Health Metrics and Evaluation, Human Development Network, The World Bank), 2013. The global burden of disease: Generating evidence, guiding policy – Sub-Saharan Africa regional edition, IMHE, Seattle, WA.
- Ismail, O, 2016. What is in a job? The social context of youth employment issues in Africa. Journal of African Economies 25(supplement 1), i37–60.
- Jonah, B, 1997. Sensation seeking and risky driving: A review and synthesis of the literature. Accident Analysis and Prevention 29, 651–65.
- Jones, S, Tefe, M, Zephaniah, S, Tedla, E, Appiah-Opoku, S & Walsh, J, 2016. Public transport and health outcomes in rural Sub-Saharan Africa – a synthesis of literature and professional opinion. Journal of Transport & Health 3(2), 211–19.
- Jones, S, Walsh, J & Appiah-Opoku, S, 2016. Rural transport health and safety in Sub-Saharan Africa research needs – a snapshot of expert opinion from an online survey. Development Southern Africa 33(5), 677–702.
- Jones, S, Walsh, J & Høyland, S, 2019. Peace, transport, and understanding – A place for Samfunnssikkerhet in African peacebuilding? Journal of Peacebuilding and Development 14(2), 216–21.
- Kircher, K & Andersson, J, 2013. Truck drivers’ opinion on road safety in Tanzania-a questionnaire study. Traffic Injury Prevention 14(1), 103–11.
- Kiser, M, Samuel, J, Mclean, S, Muyco, A, Cairns, B & Charles, A, 2012. Epidemiology of pediatric injury in Malawi: Burden of disease and implications for prevention. International Journal of Surgery 10(10), 611–7.
- Kopits, E & Cropper, M, 2005. Traffic fatalities and economic growth. Accident Analysis and Prevention 37, 169–78.
- Kudebong, M, Wurapa, F, Nonvignon, J, Norman, I, Awoonor-Williams, J & Aikins, M, 2011. Economic burden of motorcycle accidents in Northern Ghana. Ghana Medical Journal 45(4), 135–42.
- Lagarde, E, 2007. Road traffic injury is an escalating burden in Africa and deserves proportionate research efforts. PLOS One 4(6), e170.
- Laird, J & Mackie, P, 2014. Wider economic benefits of transport schemes in remote rural areas. Research in Transportation Economics 47, 92–102.
- Lamont, M, 2013. Speed governors: Road safety and infrastructural overload in post-colonial Kenya, c. 1963–2013. Africa 83(3), 367–84.
- Le Blanc, D, 2015. Towards integration at last? The sustainable development goals as a network of targets. Sustainable Development 23, 176–87.
- Limao, N & Venables, A, 2001. Infrastructure, geographical disadvantage, transport costs, and trade. The World Bank Economic Review 15(3), 451–79.
- Maldonado, C, Micthell, D, Taylor, S & Driver, H, 2002. Sleep, work schedules and accident risk in South African long-haul truck drivers. South African Journal of Science 98, 319–27.
- Mannering, F & Bhat, C, 2014. Analytic methods in accident research: Methodological frontier and future directions. Analytic Methods in Accident Research 1, 1–22.
- Mannering, F, Shankar, V & Bhat, C, 2016. Unobserved heterogeneity and the statistical analysis of highway accident data. Analytic Methods in Accident Research 11, 1–16.
- Martincus, C, Carballo, J & Cusolito, A, 2017. Roads, exports and employment: Evidence from a developing country. Journal of Development Economics 125, 21–39.
- Møller, M & Gregersen, N, 2008. Psychosocial function of driving as predictor of risk-taking behaviour. Accident Analysis and Prevention 65, 92–9.
- Møller, M & Haustein, S, 2014. Peer influence on speeding behaviour among male drivers aged 18 and 28. Accident Analysis and Prevention 64, 92–9.
- Motor Vehicle Accident Fund, 2016. Road crash and claims report 2016. http://www.mvafund.com.na/ Accessed 1 September 2017.
- Naddumba, E, 2004. A cross-sectional retrospective study of boda-boda injuries at Mulango Hospital in Kampala, Uganda. East and Central African Journal of Surgery 9, 44–7.
- Nakitto, M, Mutto, M, Howard, A & Lett, R, 2008. Pedestrian traffic injuries among school children in Kawempe, Uganda. African Health Sciences 8(3), 156–9.
- Namibia Weather Network, 2017. Yearly rainfall summary. http://weather.namsearch.com/wxrainsummary.php Accessed 1 December 2017.
- Nantulya, V & Reich, M, 2002. The neglected epidemic: Road traffic injuries in developing countries. Education and Debate 324, 1139–41.
- Nantulya, V & Reich, M, 2003. Equity dimensions of road traffic injuries in low- and middle-income countries. Injury Control and Safety Promotion 10(1-2), 13–20.
- Nhamo, G, Muchuru, S & Nhamo, S, 2018. Women’s needs in new global sustainable development policy agendas. Sustainable Development 26(6), 544–52.
- Njenga, P & Odero, K, 2014. African position paper on the role of transport in the sustainable development goals. AFCAP2/ASCAP: GEN2006A. London, UK: UKAID.
- Odero, W, Garner, P & Zwi, A, 1997. Road traffic injuries in developing countries: A comprehensive review of epidemiological studies. Tropical Medicine and International Health 2(5), 445–60.
- Odero, W, Khayesi, M & Heda, P, 2003. Road traffic injuries in Kenya: Magnitude, causes and status of intervention. Injury Control and Safety Promotion 10, 53–61.
- Okeniyi, J, Oluwadiya, K, Ogunlesi, T, Oyedeji, O, Oyelami, O, Oyedeji, G & Oginni, L, 2005. Motorcycle injury: An emerging menace to child health in Nigeria. Internet Journal of Paediatrics and Neonatology 5(1), 1–6.
- Onywera, V & Blanchard, C, 2013. Road accidents: A third burden of ‘disease’ in sub-Saharan Africa. Global Health Promotion 20(4), 52–5.
- Oppong, C, 2006. Familial roles and social transformations - older men and women in Sub-Saharan Africa. Research on Aging 28(6), 654–68.
- Peiffer, C & Rose, R, 2018. Why are the poor more vulnerable to Bribery in Africa? The institutional effects of services. Journal of Development Studies 54(1), 18–29.
- Porter, G, 2002. Living in a walking world: Rural mobility and social equity issues in sub-Saharan Africa. World Development 30(2), 285–300.
- Porter, G, 2014. transport services and their impact on poverty and growth in rural sUb-Saharan Africa: A review of recent research and future research needs. Transport Reviews 34(1), 25–45.
- Porter, G, Hampshire, K, Abana, A, Robson, E, Munthali, A, Mashiri, M & Tanle, A, 2010. Moving young lives: Mobility, immobility and inter-generational tensions in urban Africa. Geoforum; Journal of Physical, Human, and Regional Geosciences 41(5), 796–804.
- Roberts, C, 2014. Profiling the safety needs of the South African truck transportation sector. North-West University, Potchefstroom, South Africa.
- Roberts, P & Lall, S, 2019. Acceleration in Emerging Markets. In: Observing Acceleration. Palgrave Macmillan, Cham, London, UK.
- Sachs, J, 2012. From millennium development goals to sustainable development goals. The Lancet 379(9832), 2206–11.
- Savage, C, Fransman, L & Jenkins, A, 2013. Logistics in Namibia: Issues and challenges. Journal of Transport and Supply Chain Management 7(1), 1–8.
- Savolainen, P, Mannering, F, Lord, D & Quddus, M, 2011. The statistical analysis of crash-injury severities: A review and assessment of methodological alternatives. Accident Analysis and Prevention 43, 1666–76.
- Sinclair, M, 2013. Attitudes, norms and driving behaviour: A comparison of young drivers in South Africa and Sweden. Transportation Research Part F: Traffic Psychology and Behaviour 20, 170–81.
- Sperling, D & Claussen, E, 2004. Motorizing the developing World. Pew Center for Development, Washington, DC, USA.
- Starkey, P, Njenga, P, Kemstop, G, Willilo, S, Opiyo, R & Hine, J, 2013. Rural transport service indicators: Final report. AFCAP/GEN/60, International Forum for Rural Transport and Development, London, UK.
- Stewart, F, 1988. Basic needs strategies, human rights, and the right to development. Human Rights Quarterly 11, 347–74.
- Too, L & Earl, G, 2010. Public transport service quality and sustainable development: A community stakeholder perspective. Sustainable Development 18, 51–61.
- Turner, J & Kwakye, E, 1996. Transport and survival strategies in a developing economy: Case evidence from Accra, Ghana. Journal of Transport Geography 4(3), 161–8.
- Ulzen, T, Higginbotham, JC, Donnir, G, Jerome, L & Segal, A, 2018. Undiagnosed attention deficit/hyperactivity disorder (ADHD) among unionized drivers in Ghana: Public health and policy implications. Accident Analysis and Prevention 114, 12–6.
- United Nations, 2015. Transforming our world: The 2030 Agenda for sustainable development, A/RES/70/1. United Nations general Assembly, 25 September, 2015, New York, NY, USA. http://www.un.org/ga/search/view_doc.asp?symbol=A/RES/70/1&Lang=E Accessed 31 December 2017.
- UNDP (United Nations Development Programme), 2016. Leave no one behind: Ageing, gender and the 2030 Agenda. Issue Brief. UNDP, New York.
- UN Women (United Nations Entity for Gender Equality and the Empowerment of Women), 2015. Progress of the world’s women 2015–2016: Transforming economies, realizing rights. UN Women, New York.
- van de Walle, D, 2002. Choosing rural road investments to help reduce poverty. World Development 30(4), 575–89.
- van Garenne, O, van Niekerk, J & Grobbelaar, B, 2001. Mechanical failures as a contributing cause to motor vehicle accidents - South Africa. Accident Analysis and Prevention 33(6), 713–21.
- Wegman, F, 2017. The future of road safety: A worldwide perspective. IATSS Research 40, 66–71.
- World Bank, 2017. The high toll of traffic injuries: Unacceptable and preventable. World Bank, Washington, DC.
- WHO (World Health Organization), 2018. Global status report on road safety 2016. https://www.who.int/violence_injury_prevention/road_safety_status/2018/en/ Accessed 19 July 2019.
- Zimmerman, K, Mzige, A, Pascience, K, Museru, L & Guereereo, A, 2012. Road traffic injury incidence and crash characteristics in Dar es Salaam: A population based study. Accident Analysis & Prevention 45, 204–10.