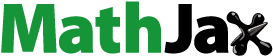
ABSTRACT
This study employed the compensation variation (CV) and propensity score matching (PSM) methods to analyse the impact of government support services and programmes on the welfare of households. The findings revealed that government support programmes, such as Reconstruction and Development Programme (RDP) support, social grants and government-subsidised houses, impact positively on the welfare of households in low- and middle-income classes in the Limpopo province. The findings further revealed that welfare gains of households in the low-income category are higher than those of households in the middle-income category. Moreover, it was found that the ownership of assets such as a television or a motor vehicle, which are associated with routine maintenance, monthly subscription fees, fuel and other costs, significantly reduce the welfare of households in the low- and middle-income categories. The study concludes that these programmes should be sustained and extended to other non-beneficiary households if the aim is to improve household welfare and better the standards of living.
1. Introduction
Governments around the world agree that all citizens have a right to the benefits of government support (UN, Citation1989). Government support, also known as social services, first emerged in Europe in the 1800s in the form of social insurance and social assistance programmes with the purpose of providing economic safety nets for citizens during economic hardship, illness and other shocks (Palacios & Sluchynsky, Citation2006). Marques (Citation2003) stated that government support was understood in a broad sense and includes old age pensions, family allowances, child support grants and other forms of support. Government support was important in poverty reduction and to ensure a basic standard of living for individuals living below the poverty line. It contributes towards achieving a more reasonable income distribution in society. Government support is not new, as most developed and emerging countries have been using it in the hope that it will enhance the welfare of the poor, improve equality, and strengthen the social and political stability of the country, in order to lead to successful policy reform (Yamada, Citation2016). These government programmes are an important policy instrument in poverty elimination, because they ensure that low-income households have the least onerous standard of living possible and provide a more equitable distribution of income in the economy (Triegaardt, Citation2005). Triegaardt (Citation2005) further explained that, as a policy option, government support had been widely accepted in developed and emerging economies.
In 1994, a new democratic dispensation began in South African and, in its development policy initiatives, the South African government focused on rectifying the socio-economic disparities and racial divisions that existed in the past. After the inception of democracy in 1994, the South African monetary policies were dedicated to improving the livelihoods of the poor, where most of the policies to help the poor were implemented in the housing, healthcare and social security sub-sectors (Gyekye & Akinboade, Citation2003). In South Africa, the government adopted a social security system through investment in social wages, which comprise education, health services and social development. The aim of this system is to reduce the cost of living for underprivileged households and individuals (Triegaardt, Citation2006). Moreover, vulnerable households are supported through social development programmes such as the old age grant, child support grant and other forms of social assistance. Government support has been recognised throughout developed and emerging economies to promote uniformity and to protect the poor. It also provides people with proper healthcare, education and basic services (Triegaardt, Citation2006). Taylor (Citation2002) stated that the reality of government support and social welfare in Africa excluded more people than what it covers. This is due to the widespread challenges of poverty, which weaken the capacity of the government to fund contributions.
Understanding the impact of government support on welfare is important. To some degree, households are faced with challenges with regard to issues of government support, such as basic needs. The challenge faced by individuals in South Africa is that many family units still have a low potential of gaining access to quality education, health care, electricity and potable water. Such households are under extreme pressure because they cannot attain minimum living standards, as measured in income and consumption terms (Gyekye & Akinboade, Citation2003). Austin et al. (Citation2004) reported that macroeconomic changes fuel the attention of welfare interests in poor households and stated that high rates of unemployment, teenage childbearing and single-parent households formed part of the simultaneous disadvantages that households face. These households need government support to meet their basic socio-economic needs. They lack access to year-round food, they skip meals on a daily basis, the majority of the individuals are unable to access healthcare services, and they lack services such as potable water for drinking and water for sanitation. Therefore, the majority of households in South Africa cannot manage to pay for basic education for some or all of their offspring, some even lacking basic living quarters (Sachs, Citation2005).
Moreover, the income of these households is low and they can barely meet the basic needs to survive on a daily basis. There has been a substantial growth in government support, such as grants, and much has been done to expand access to services for households. However, the question remains whether the various forms of government support are effective in improving the living standards of households, and to what extent these forms of support are affecting the welfare of people. It is therefore critical to identify the welfare impact of government support on households in the Limpopo province.
The objective of this study was to evaluate the impact of basic government support programmes, such as the Reconstruction and Development Programme (RDP), subsidised houses, and social welfare (grants) for households in the Limpopo province. This province was selected because existing statistics show that Limpopo is one of the most poverty-stricken provinces in South Africa (Statistics South Africa [Stats SA], Citation2002), with 89.3% of its population residing in the rural areas (Stats SA, Citation2006). Furthermore, this province has the highest rate of illiteracy, about 46%, indicating widespread unemployment and low income (Wanka, Citation2014) and lacks basic services and opportunities for lucrative employment (Botha, Citation2010).
This is one of the first studies that used rigorous empirical approaches to quantify the impact of basic government support programmes in the study area. Findings from this study can therefore potentially help to identify the remaining gaps to assist the government in improving the existing support services and policies that would help poor households to improve their standard of living in the Limpopo province.
2. Literature review
Government support programmes are responsible for delivering regular income to the most vulnerable households and comprise one of government’s direct resources to fight poverty (Bhorat & Van der Westhuizen, Citation2012). The extent of poverty and how poor households respond to their economic and physical environment have attracted extensive empirical studies. These studies aim to improve social assistance and household welfare. Some authors have considered the macroeconomic impact of government support and the role of social aid in eradicating poverty and stimulating household improvement, while others have examined the influence of social support on health, education, basic shelter, consumption and unemployment (Samson et al., Citation2004; Wanka, Citation2014; Cecchini et al., Citation2015; Abramo et al., Citation2019).
In Latin America and the Caribbean, the impact of social programmes and how they contribute to poverty eradication and labour inclusion were assessed (Abramo et al., Citation2019). These authors found that poverty and income disparities remain structural problems. It was further argued that there should be continuous integration of economic analysis, production and social strategies, and policies on job creation (Cecchini et al., Citation2015; Abramo et al., Citation2019). In Asia and the Pacific regions, efforts were made by various governments to reduce poverty and improve the quality of life of their people through development policies (Asian Development Bank, Citation2012; Economic and Social Commission for Asia and the Pacific (ESCAP), Citation2017).
In South Africa, the poverty level and wellbeing of households ten years after the fall of apartheid were assessed by Bhorat & Van der Westhuizen (Citation2012), Gumede (Citation2008) and Bhorat & Kanbur (Citation2005). In addition, income distribution and the relationship between poverty, inequality and growth were evaluated (Leibbrandt et al., Citation2001; Gyekye & Akinboade, Citation2003; Van der Berg & Siebrits, Citation2010).
The existing studies, however, have some gaps. First, the literature points to the fact that different governments in developing economies are making efforts to design programmes, strategies and policies to eradicate poverty and inequality (Samson et al., Citation2004; Wanka, Citation2014; Cecchini et al., Citation2015; Abramo et al., Citation2019) although the precise impact of the various strategies on welfare of people have received little research attention. Secondly, most of the studies approached impact assessment from a descriptive perspective, which makes it difficult to pinpoint the precise impact or contribution of the different programmes, strategies and policies on the welfare of people. Additionally, none of the studies researched the impact of government support programmes on the welfare of households in the Limpopo province in particular.
Nonetheless, some authors applied rigorous methods. For instance, Pons (Citation2011) applied a compensation variation (CV) method to estimate the effects of rising food prices on low-income households in India. This author found that households in rural areas were more susceptible to rising food prices than households in urban areas. Furthermore, low-income households in both rural and urban areas felt the effect of increasing food prices more than high-income households. Deaton (Citation1989) applied a CV method to estimate the effect of presumed changes in the price of rice on income levels in Thailand. According to the author, an increase in the prices of rice was beneficial to households’ income in the rural areas of Thailand. Empirically, some studies employed propensity score matching (PSM) to estimate the causal effect of interventions, support programmes and the treatment of covariates (Dehejia & Wahba, Citation1999; Wolfe & Michaud, Citation2004; Li, Citation2012; Donkor et al., Citation2016).
In this study, the CV method, multivariate regression and PSM method was employed to quantity welfare of different support programmes, determinants of welfare and the impact of the support programmes on different income groups. The next section discusses the methods employed in details.
3. Methodology
3.1. Theoretical and empirical framework
This study follows the theory of change in welfare, proposed by Deaton (Citation1989). Deaton defined welfare as an everyday person’s life perspective, and it can be measured on a societal macro-level, according to whether one understands it from an economic or societal perspective. Deaton conceptualised change in welfare into six main indicators, namely income, calorie intake, consumption, expenditure, poverty and growth. These indicators can be used to track and compare the living standards of individuals or household units in society. A study by Greve (Citation2008) defined welfare change as financial support, which is designed to actively encourage the basic physical and material wellbeing of people in need. In the last century, a lack of data restrained the testing of development theories and evaluating policies that can reduce poverty and increase the living standards of the poor. Basic problems with regard to the extent of poverty and the way underprivileged households respond to their economic and physical environment were difficult to quantify due to a lack of data and suitable techniques.
However, in recent years, household survey data had been employed to measure the standard of living and to identify its determinants. Hence, the adoption of government support strategies emerged as one of the approaches to combat low levels of welfare and poverty in developing countries. Based on this conception, we assume that government support programmes provided to society are improving households’ welfare and the living standard of the poor, given the socio-economic characteristics and assets relating to welfare of households.
In this study, estimation approaches, namely the CV method, multivariate regression and PSM are employed to estimate the impact of government support on the welfare of households in the study area.
3.1.1. Implementation of the compensation variation (CV) method
Compensation variation (CV) is a non-parametric method used in the analysis of change in welfare. Zezza et al. (Citation2008) defined CV as the gain and loss of income necessary to bring the household back to its original position before the economic change. Given the change in welfare, the net effect of the household’s welfare change would depend on the household’s condition as a recipient, relative to households that did not receive government support. It was assumed that households that received government support would experience better living standard than households that did not. In order to capture the immediate welfare changes of government support on households in the Limpopo province, the CV model was used. Following Zezza et al. (Citation2008), the CV model is specified in Equation 1:(1)
(1)
In terms of the work of Zezza et al. (Citation2008), is the first-order approximation of the change in welfare of household
in the event of a change in government support;
is the original income of household
;
is the original price at which production is valued;
is the original price at which consumption is valued;
is the production value of household
, as a proportion of income (proxied by own production plus subsistence farming); and
is the consumption value for household
.
Equation (1) estimates the after-effect responses, which takes into account household consumption and production decisions. The equation can be re-specified to account for different degrees of change transmission in household welfare, and the variation in income changes within each household. The assumption behind the equation is that households are well off if they receive any form of government support (social grant). In other words, if the household receives government support, it will experience a welfare gain, while it will experience a welfare loss if it does not receive this kind of support. Households in this study were expected to improve their standards of living after any given change in social welfare because they received government support. Equation (1) reduces to:(2)
(2) The CV method is generally used to assess the impact of price changes on welfare, which is also captured in the Equation (1) model. However, the analysis in this submission does not directly evaluate the change in prices on household welfare as it evaluates the change in household income. The CV method was used because of the realisation that the introduction of government interventions or support programmes influenced food prices and the consumption of households in the study area. Consumption, production and prices used to calculate household welfare was used as the dependent variable in the study’s subsequent analysis.
A multivariate regression model was estimated on the inferred percentage change in a number of household socio-economic characteristics, household assets, and institutional factors of welfare change. This model was used because the dependent variable was estimated for three different income categories. The model helped to isolate the correlates of the simulated welfare impact and to identify factors that influenced the welfare of households besides government support programmes. The model is specified in Equation (3):(3)
(3) where
represents the estimated change in welfare, expressed as a percentage of initial per capita expenditure of household
;
is a constant term;
is a vector of parameters to be estimated; and
is the error term. The variables and their definitions are presented in .
Table 1. Description and summary characteristics of variables included in the model.
It is worth mentioning that the validity of conditional independence assumption associated with the application of the PSM method was tested for. This was done by including selectivity terms, also referred to as Mills ratios (Mills1 and Mills2) in out multivariate analysis.(4)
(4) Bourguignon et al. (Citation2007) indicated that, if one or more of the selectivity terms is significant, then sample unobserved heterogeneity is present. In such circumstances, there would be biased and unreliable estimated coefficients from the gross margin equations if they are estimated without including the selectivity terms. Selectivity effects would be absent if the selectivity variables included in the model are not significant. Hence, in this analysis, it was assumed that the selection of households that would receive government support was not correlated with any unobserved characteristics but was purely a function of observed characteristics.
3.1.2. Implementation of propensity score matching (PSM)
Propensity score matching (PSM) is employed as a robust check to complement the CV approach. While this method determines the causal effect related to the adoption decision, it only addresses the observable factors (Rosenbaum & Rubin, Citation1983). PSM indicates the combination of treatment and control units with similar values on the propensity score, and perhaps other covariates, and the removal of all incomparable units (Rubin, Citation2001). PSM is employed to evaluate the impact of government support on household welfare. The technique involves two stages. In the first stage, propensity scores (probability) of receiving government support are estimated by using a probit regression model. The PSM can be expressed as:(5)
(5) where
is the welfare of household i with characteristics Z;
{.} is the normal cumulative distribution; and Z is a vector of pre-treatment characteristics (Donkor et al., Citation2016).
denotes access to government support (1 if a household receives government support and 0 otherwise).
Two assumptions are required when assessing the treatment effects based on propensity score. The first one is the conditional independence assumption involving common variables that affect the treatment assignment, and results in a noticeable treatment-specific outcome (Donkor et al., Citation2016). According to Donkor et al. (Citation2016), the dependence between treatment assignment and treatment-exact outcome could be removed by conditioning on the observable. Furthermore, the second assumption states that the average treatment effect on the treated (ATT) is only defined within the area of mutual sustenance. According to Heckman (Citation1979), this statement ensures that individuals with the same Z-characteristic values have a positive probability of being both beneficiaries and non-beneficiaries of government support. The second stage involves the estimation of the ATT based on the propensity score. Empirically, the equation is expressed as:(6)
(6)
(7)
(7) where
is the welfare of beneficiaries; and
is the welfare of non-beneficiaries.
A number of methods have been suggested in the literature to match similar participants and non-participants. The most frequently used methods are the nearest-neighbour matching (NNM), kernel-based matching (KBM) and radius approaches (Donkor et al., Citation2016). In this study, the NNM and KBM approaches were employed.
3.2. Description of data
The study was conducted in the Limpopo province, which is located in the northern tip of South Africa between longitude 29.4179°E and latitude 23.4013°S, and borders Botswana, Zimbabwe and Mozambique. The total population of Limpopo is estimated at 5 630 500, which represents 10.4% of the total population of South Africa. The province is rich in wildlife, natural beauty, and historical and cultural treasures. Limpopo produces a wide range of agricultural products and has abundant mineral resources, making mining the critical sector of the province’s economy through its contribution of 22% of the gross domestic product. Limpopo is considered one of the most poverty-stricken provinces in South Africa (Stats SA, Citation2002). The province has low levels of urbanisation, as 89.3% of the population resides in the rural areas (Stats SA, Citation2006). Furthermore, the province has the highest rate of illiteracy, at about 46%, indicating widespread unemployment and low income (Wanka, Citation2014). Botha (Citation2010) stated that the Limpopo province lacks basic services and opportunities for lucrative employment.
A multistage stratified sampling technique was used to select the respondents. The first stage involved the purposive sampling of the province due to its lack of basic services. The second stage involved a simple random sampling of 342, 232 and 121 households, from low-, middle- and high-income households, respectively. The classification of households into low-, middle- and high-income categories was based on Stats SA’s classification. The sampling frame for households and income classification were obtained from Income and Expenditure Survey (IES) data and Stats SA's Master Sample (MS) based on the 2001 Census Enumeration Areas (EAs). The sampling sizes from the different income classes were proportionally based on the actual number of households in each class (Kothari, Citation2004). We used Stata computation (power mean command with an assumed power value of 0.9 and a two-sided test) to attain a representative sample and good statistical power for the analysis. A structured questionnaire was used in the data collection.
The questionnaire requested information on households’ welfare, such as food and non-food expenditure, consumption, production, prices and income, socio-economics factors and household assets before and after the introduction of government support programmes. The socio-economic variables included age, gender, education and household size, while household assets included own businesses, radios, televisions, DVD players and motor vehicles. The government support variables in this study were social grants, RDP support, free electricity, free water, subsidy repayment, government-subsidised houses and medication. The models were estimated by using Stata software. The description of changes in welfare variables, together with their descriptive statistics, are summarised in .
4. Results and discussion
4.1. Descriptive results
The descriptive and summary characteristics of the variables are presented in , along with their mean differences. The results show that the average age of the low-income respondents was 49, and that of the middle-income group 38, with a significant mean difference of 10.48. Only 49.56% of the low-income respondents were males, while most of the middle-income respondents were males (60.74%), suggesting that most of the low-income respondents were females. There was a significant mean difference in the household size of the two income categories, the low-income households being 2.61 times bigger than the middle-income households. Of the low-income households, 31% had no formal education, in contrast with the 14.54% of the middle-income category. This emphasises the importance of education in improving the income status and welfare of households (Orbeta, Citation2005). Of the middle-income respondents, 41% had attained Grade 12 with university education, compared with the 20.17% of low-income households.
In terms of assets, the results show that 57% of middle-income respondents had started their own businesses, compared with the 35% of low-income respondents in that category. This provides a rationale for encouraging low-income respondents to develop entrepreneurial skills and start some businesses of their own. With regard to the valuation of assets such as TVs, radios, DVDs and motor vehicles, the results show significant mean differences between the value of assets belonging to low-income and middle-income households. The assets of middle-income households were of higher value, compared with that of low-income households, as shown by the significant mean differences between the valuation estimates of the two categories.
Pertaining to support programmes, the descriptive results indicate that most of the low-income households (48.89%) benefited from social welfare grants, compared with the 41.46% of middle-income households, reflecting a significant mean difference of 3.43. In addition, 35.35% of the low-income households received houses through the RDP, compared with only 28.98% of the middle-income households. Only 19.76% of the low-income households benefited from state-subsidised houses, relative to the 29.56% of the middle-income households.
4.2. Empirical results
4.2.1. Factors influencing welfare in the low and middle-income households
presents the factors that determine household welfare in the Limpopo province. In this section, other factors that affect household welfare, besides support programmes, were considered. The results for the low-, middle- and high-income groups were estimated. It needs to be stated that the high-income group was used as a reference to compare the effectiveness of government support on the welfare of households in the study area, since the focus of the study was on low- and middle-income households.
Table 2. Determinants of household welfare in the Limpopo province.
For the low-income group, the results show that socio-economic factors such as household size and no formal education had a negative effect on welfare, relative to the high-income group. These are shown by the significantly negative coefficients of 0.942 and 0.835 at the 1% level for household size (Hsize) and no formal education (Edu0). The negative effect of household size on low-income households implies that, as a household’s size increased, its welfare decreased. Large households have greater basic needs and, as such, they require a higher income to attain a higher standard of living and better welfare. This is in line with the findings of Orbeta (Citation2005).
Educational variables, such as Grade 12/Standard 10 with university education (Edu2) and Grade 12/Standard 10 without university education (Edu3) are significantly different from zero and positive, as expected. This finding implies that household heads with these levels of education had better welfare levels among the low-income households in Limpopo, relative to those who could not complete their Grade 10/Standard 8 education. This finding confirms that a higher educational qualification can help a person find a job, receive a better income and have a better standard of living, thereby improving the welfare of the household. This is supported by Branson et al. (Citation2009), who found that education betters the chances of people to get into the job market.
In terms of asset ownership, the results reveal that ownership of assets such as television sets and motor vehicles, had a significantly negative impact on the welfare of households in the lower income category in the Limpopo province. This is indicated by the significantly negative coefficients of the variables television and motor vehicle, at the 1% level. The negative impacts of these assets might be the result of the costs associated with the monthly subscription, maintenance and fuel, and other costs incurred to maintain these assets. However, ownership of assets such as DVD players, which do not require monthly subscription charges, had a positive effect on the welfare of low-income households. Households with their own businesses had an improved welfare relative to those that do not have any small business. This is indicated by the highly significant coefficient estimate of own businesses, which suggests that, in order to improve the welfare of low-income households, there is a need to encourage them to engage in running their own small businesses.
Among the government support variables, the results indicate that RDP support (RDP_support) and government-subsidised houses (Subsidised_hse) had a significantly positive effect on the welfare of households in the low-income category in the Limpopo province. This is indicated by the significantly positive coefficient estimates of the variable government-subsidised house (Subsidised_hse) at the 10% and 1% levels. The implication of these results is that low-income households receiving government support in terms of free houses were more likely to have improved living standards and an increase in welfare than households that did not receive RDP houses. The results further imply that, as government support in the form of subsidised houses for the low-income group increased, their welfare improved. These results are consistent with the findings of Mayo & Gross (Citation1987).
Access to social grants had the expected positive sign for low-income households and is statistically significant at the 1% level. The positive sign for social welfare grants suggests that low-income households with access to social grants tend to experience improved welfare compared with households that did not receive social grants. This suggests that social grants should be extended to the majority of poor people in the low-income category, as confirmed by the findings of Woolard & Klasen (Citation2010).
For the middle-income group, the results show that household size had a negative effect on the welfare of households relative to that of high-income households. This is shown by the significantly negative coefficient of −0.376 at the 1% level. This result implies that the welfare of middle-income households with low household sizes was better than that of large households, ceteris paribus. It concurs with the findings of Arthur (Citation2005), who found that a large household size had implications for the quality of life and ability of household heads to cater for the needs of their families.
In terms of education, the results show that household heads with no formal education experience reduced welfare, as indicated by the significantly negative coefficient of −1.139. This, too, concurs with the findings of Arthur (Citation2005). Household heads who have attained Grade 12/Standard 10 with university education (Edu2) and Grade 12/Standard 10 without university education (Edu3) experienced improved welfare, as indicated by the significantly positive coefficients of these variables. This finding that there is a crucial connection between education and welfare in society is supported by that of UNRISD (Citation2010).
Regarding household assets, the results show that ownership of a television had a significantly negative impact on household welfare, as shown by the significantly negative coefficient of −1.022 at the 10% level. The reason for this finding might be the monthly subscription that households pay to gain access to pay-for-view channels like SuperSport and other international channels. The results in further indicate that both the value of the house (Hsevalue) and ownership of a business had a positive impact on the welfare of households in the middle-income category.
The study further shows that support services like RDP support (RDP_support), social grants and government-subsidised houses (Subsidised_hse) had a significantly positive effect on the welfare of households in the middle-income category in the Limpopo province. This is shown by the significantly positive coefficient estimates of these variables under the conventional levels. These results are consistent with the findings of Woolard & Klasen (Citation2010). None of the Mills ratios included in the model was significant, which justifies the selection of the PSM model and the assumption that the selection for receiving government support is not correlated with any unobserved characteristics, but is purely a function of observed characteristics (Bourguignon et al., Citation2007). This test indicates that none of the selectivity terms were significant and, as such, it was concluded that selection for the government support programme is related to observed factors, confirming that all households have the chance of benefiting from support services.
4.2.2. Impact of government support programmes on welfare
The results of PSM estimates of the impact of government support on household welfare are presented in . The impact of the various support programmes are presented separately for different income categories. For social grants in the low-income group, the results reveal that the causal impact of having access to social grants caused an increase in welfare by 8.06% and 8.02% for the NNM and KBM principles, respectively. These increases in welfare are significantly different from zero, as indicated by the T-values. In terms of percentage change, the results indicate that the implementation of social grants resulted in a change in welfare of 81% relative to non-beneficiaries, using the KBM algorithm. The findings suggest that the implementation of social grants could improve the welfare of low-income households. This concurs with the findings of Woolard & Klasen (Citation2010) that social grants provide households with income transfer and improved welfare.
Table 3. Impact of government support on low- and middle-income category of respondents (PSM).
In terms of RDP support, the results show that there was a significant impact on the beneficiaries’ welfare. The causal impact of the low-income group in Limpopo receiving RDP support revealed an increase in the welfare of beneficiaries, by 8.31% and 8.28% for the NNM and KBM principles, respectively. The percentage change results indicate that there was an increase of 78% in their welfare compared with non-beneficiary households. This result is consistent with that of Mayo & Gross (Citation1987).
Concerning subsidised houses, the results indicate a significant impact on beneficiaries’ welfare. The causal impact of the low-income group in Limpopo receiving subsidies for houses reflected an increase in the welfare of beneficiaries by 5.69% and 4.03% for the NNM and KBM principles, respectively. The results of percentage change show that there was a change of 58% in welfare of the low-income households compared with non-beneficiary households.
For the middle-income category, it was found that the causal impact of having access to social grants caused an increase in welfare by 7.53% and 7.60% for the NNM and KBM principles, respectively. These increases in welfare are significantly different from zero, as shown by the T-values. In terms of percentage change, the results indicate that the implementation of social grants resulted in a 69% change in welfare relative to non-beneficiaries, using the KBM algorithm. The findings suggest that the implementation of social grants could improve the welfare of middle-income households. This concurs with the finding of Woolard & Klasen (Citation2010).
The results show that the causal impact of receiving RDP support reflected an increase in the welfare of beneficiaries by 8% for both matching principles in the middle-income category. Concerning percentage change, the results indicate that there was an increase of about 60% in the welfare of middle-income households compared with non-beneficiary households. This result is consistent with the results of Mayo & Gross (Citation1987).
Concerning subsidised houses, the results show that there was a significant impact on beneficiaries’ welfare. The causal impact of the beneficiaries receiving a subsidy for a house reflected an increase in welfare of 7% for both matching principles in the middle-income group. In terms of percentage change, the results indicate that there was a change in welfare of 64% for middle-income households compared with non-beneficiary households.
5. Conclusions and policy recommendations
The study examined the impact of basic government support programmes, such as the RDP, subsidised houses and social grants, on the welfare of households in the Limpopo province of South Africa by using survey data consisting of 342, 232 and 121 households from low-, middle- and high-income households, respectively. The CV method, multivariate regression and PSM were used to quantity the welfare of different support programmes, determinants of welfare and the impact of the support programmes on different income groups.
The findings revealed that most of the low-income households benefited from social welfare grants relative to the middle-income households. Also, the RDP favoured households with low income, compared with middle-income households. Most middle-income households benefited from state-subsidised houses, relative to low-income households. Empirically, it was found that larger household sizes and no formal education had a negative and significant impact on the welfare of low- and middle-income groups, relative to the high-income group. On the other hand, attaining Grade 12/Standard 10 education with or without university education significantly and positively impacted on the welfare of households with low- and middle- income. Households with their own businesses experienced improved welfare relative to those without any small business. All of the support programmes had a positive effect on the welfare of households in the low- and middle-income groups, compared with the high-income group. The causal impact of having access to social grants and RDP support reflected an increase of 8% in welfare for low-income beneficiary households, whereas the receipt of a subsidy for a house resulted in an increase of about 6% in the welfare of low-income beneficiary households. For both low- and middle-income households, social grants and RDP support yielded higher welfare gains than subsidised houses. Similarly, in the middle-income category, social grants yielded the highest welfare improvement, followed by RDP support and subsidised houses.
Based on the findings, the following conclusions are drawn: firstly, it is concluded that, in order to improve the welfare of low- and middle-income households in Limpopo province, people need to be encouraged to enrol in educational programmes. Regardless of the income category, households with no formal education and larger household sizes are all found to be systematically associated with low levels of welfare. Households in the low- and middle-income categories with large household sizes are likely to experience reduced welfare. Secondly, the ownership of assets, such as televisions and motor vehicles, which are associated with routine maintenance, monthly subscription fees, fuel and other expenses, significantly reduces the welfare of households in the low- and middle-income categories. Thirdly, the study concludes that households that own small businesses experience improved welfare. More importantly, government support programmes such as the RDP, social grants and government-subsidised houses have a positive impact on the welfare of households in the low- and middle-income classes in the Limpopo province. Generally, the findings reveal that the welfare gains by households in the low-income category are higher than that of households in the middle-income category.
Recommendations based on the study’s findings and conclusions are as follows: the Department of Basic Education should design policies to encourage the enrolment in basic educational programmes. For instance, adult educational programmes can be developed to help adults who never got a chance to attain basic education. It is further recommended that households in the study area should be encouraged and supported to start their own businesses or small enterprises. Government support programmes are therefore needed to promote households’ welfare in an effort to achieve a better standard of living. It is suggested that the South African government and the Department of Social Development (DSD) extend these programmes to reach other low- and middle-income households that are not yet beneficiaries. This suggests that the development of welfare-improvement programmes should target specific income groups, with different programmes being developed for different income groups. A proper understanding of the implementation and impact of government support programmes by the DSD and South African government on households’ welfare is crucial in ensuring better standards of living and a reduction in poverty.
These findings provide the insights required to develop and sustain the programmes in the long run. It is recommended that government support should consider the use of selective demographic targeting as a feasible strategy when designing livelihood-improvement strategies for different income groups and households. Since socio-demographics, assets and support services significantly influence a household’s welfare and standard of living, policy makers should pay particular attention to these factors.
Disclosure statement
No potential conflict of interest was reported by the author(s).
References
- Abramo, L, Cecchini, S & Morales, B, 2019. Social programmes, poverty eradication and labour inclusion: Lessons from Latin America and the Caribbean. ECLAC Books, No. 155 (LC/PUB.2019/5-P). https://repositorio.cepal.org/bitstream/handle/11362/44622/1/S1900004_en.pdf.
- Arthur, L, 2005. Family size and its socio-economic implications in the Sunyani municipality of the Brong Ahafo region of Ghana, West Africa. Centre for Development Studies, Faculty of Social Science, University of Cape Coast, Cape Coast, Ghana.
- Asian Development Bank, 2012. Food security and poverty reduction in Asia and the Pacific. Key Challenges and Policy Issues. www.adb.org/sites/default /files/publication/29724/food-security-poverty.pdf.
- Austin, MJ, Chow, J, Hastings, J, Taylor, S, Johnson, M, Lemon, K & Leer, E, 2004. Serving low-income families in poverty neighborhoods using promising programmes and practices: Building a foundation for redesigning public and nonprofit social services. University of California Press, Berkeley.
- Bhorat, H & Kanbur, R, 2005. Poverty and well-being in post-apartheid South Africa: An overview of data, outcomes and policy. Working paper 05/101, Development Policy Research Unit (DPRU), University of Cape Town, Cape Town.
- Bhorat, H & Van der Westhuizen, C, 2012. Poverty, inequality and the nature of economic growth in South Africa. Working Paper 12/151, Development Policy Research Unit (DPRU), University of Cape Town, Cape Town.
- Botha, F, 2010. The impact of educational attainment on household poverty in South Africa. Acta Academia 42(4), 122–47.
- Bourguignon, F, Fournier, M & Gurgand, M, 2007. Selection bias corrections based on the multinomial logit model: Monte Carlo comparisons. Journal of Economic Surveys 21(1), 174–205. doi: 10.1111/j.1467-6419.2007.00503.x
- Branson, N, Leibbrandt, M & Zuze, TL, 2009. The demand for tertiary education in South Africa: Final report. South Africa Labour and Development Research Unit, University of Cape Town, Cape Town.
- Cecchini, S, Filgueira, F, Martínez, R & Rossel, C, 2015. Towards universal social protection. Latin American pathways and policy tools. Economic Commission for Latin America and the Caribbean (ECLAC) Books, No. 136 (LC/G.2644-P). Santiago, November 2015. https://ssrn.com/abstract=2699403.
- Deaton, A, 1989. Rice prices and income distribution in Thailand: A non-parametric analysis. The Economic Journal 99(395), 1–37. doi: 10.2307/2234068
- Dehejia, RH & Wahba, S, 1999. Causal effects in nonexperimental studies: Reevaluating the evaluation of training programs. Journal of the American Statistical Association 94(448), 1053–62. doi: 10.1080/01621459.1999.10473858
- Donkor, E, Owusu-Sekyere, E, Owusu, V & Jordaan, H, 2016. Impact of row-planting adoption on productivity of rice farming in Northern Ghana. Review of Agricultural and Applied Economics 19(2), 3–12. doi: 10.15414/raae.2016.19.02.19-28
- Economic and Social Commission for Asia and the Pacific (ESCAP), 2017. Eradicating poverty and promoting prosperity in a changing Asia-Pacific. https://sdgasiapacific.net/download/AP_SDG_Thematic-Report_2017.pdf.
- Greve, B, 2008. What is welfare? Central European Journal of Public Policy 2(1), 50–73.
- Gumede, V, 2008. Poverty and ‘second economy’ in South Africa: An attempt to clarify applicable concepts and quantify extent of relevant challenges. Working Paper 8/133, Development Policy Research Unit (DPRU). http://www.commerce.uct.ac.za/dpru/ Accessed 10 August 2016.
- Gyekye, AB & Akinboade, OA, 2003. A profile of poverty in the Limpopo province of South Africa. Eastern Africa Social Science Research Review 19(2), 89–109. doi: 10.1353/eas.2003.0005
- Heckman, JJ, 1979. Sample selection bias as a specification error. Econometrica 47(1), 153–61. doi: 10.2307/1912352
- Kothari, CR, 2004. Research methodology: Methods and techniques. New Age Publications, New Delhi.
- Leibbrandt, M, Woolard, I & Bhorat, H, 2001. Understanding contemporary household inequality in South Africa. In Bhorat, H, Leibbrandt, M, Maziya, M, Van der Berg, S & Woola, I (Eds.), Fighting poverty: Labour markets and inequality in South Africa. UCT Press, Landsdowne.
- Li, M, 2012. Using propensity score method to estimate causal effects: A review and practical guide. Organisational Research Methods 16(2), 1–39.
- Marques, J, 2003. Social safety net assessment from Central America: Cross-country review of principal findings. Social Protection Discussion Paper Series No. 0316. www.worldbank.org/sp Accessed 19 September 2016.
- Mayo, SK & Gross, DJ, 1987. Sites, services, and subsidies: The economics of low-cost housing in developing countries. The World Bank Economic Review 1(2), 301–35. doi: 10.1093/wber/1.2.301
- Orbeta, AC, 2005. Poverty, vulnerability and family size from the Philippines. ADB Institute Research Paper Series No 68, Asian Development Bank Institute, Japan.
- Palacios, R & Sluchynsky, O, 2006. Social pensions part I: Their role in the overall pension system. Social Protection Discussions Paper No. 0601. World Bank, Washington.
- Pons, N, 2011. Food and prices in India: Impact of rising food prices on welfare. Paper presented at the Seventh Annual Conference on Economic Growth and Development, Indian Statistical Institute, 23 September, New Delhi.
- Rosenbaum, PR & Rubin, DB, 1983. The central role of the propensity score in observational studies for causal effects. Biometrika 70(1), 41–55. doi: 10.1093/biomet/70.1.41
- Rubin, DB, 2001. Using propensity scores to help design observational studies: Application to the tobacco litigation. Health Services and Outcomes Research Methodology 2, 169–88. doi: 10.1023/A:1020363010465
- Sachs, J, 2005. The end of poverty: How we can make it happen in our lifetime. The Penguin Press, New York.
- Samson, M, Lee, U, Ndlebe, A, Mac Quene, K, Van Niekerk, I, Gandhi, V, Harigaya, T & Abrahams, C, 2004. The social and economic impact of South Africa’s social security system. EPRI Research paper No. 37, Economic Policy Research Institute, Cape Town.
- Statistics South Africa (Stats SA), 2002. Earning and spending in South Africa: Selected findings and comparisons from the income and expenditure surveys of October 1995 and October 2000. Government Printer, Pretoria.
- Statistics South Africa (Stats SA), 2006. Provincial profile 2004: Limpopo. Government Printer, Pretoria.
- Taylor, V, 2002. Report of the committee of inquiry into a comprehensive system of social security for South Africa. Transforming the present – protecting the future. Consolidated Report. March 2002. Department of Social Development, Pretoria, South Africa.
- Triegaardt, JD, 2005. Accomplishment and challenges for partnership in development in the transformation of social security in South Africa. Oxford University Press, Cape Town.
- Triegaardt, JD, 2006. Reflections on poverty and inequality in South Africa: Policy considerations in an emerging democracy. Research Paper, Development Bank of Southern Africa, Midrand, South Africa.
- UN, 1989. Convention on the rights of the child. In United Nations Treaty Collection. GA Resolution 4412549, United Nations, Geneva.
- UNRISD, 2010. Why care matters for social development. Research and Policy Brief 9. UNRISD, Geneva.
- Van der Berg, S & Siebrits, FK, 2010. Social assistance reform during a period of fiscal distress. Paper prepared for the Financial and Fiscal Commission, Midrand, South Africa.
- Wanka, FA, 2014. The impact of educational attainment on household poverty in South Africa: A case study of Limpopo Province. Doctoral dissertation, Department of Economics, University of the Western Cape.
- Wolfe, F & Michaud, K, 2004. Heart failure in rheumatoid arthritis: rates, predictors, and the effect of anti-tumour necrosis factor therapy. The American Journal of Medicine 116(5), 305–11. doi: 10.1016/j.amjmed.2003.09.039
- Woolard, I & Klasen, S, 2010. The evolution and impact of social security in South Africa. https://www.researchgate.net/publication /242595464 Accessed 29 October 2016.
- Yamada, K, 2016. Tracing the impact of large minimum wage changes on household welfare in Indonesia. European Economic Review 87(C), 287–303. doi: 10.1016/j.euroecorev.2016.05.008
- Zezza, A, Davis, B, Azzarri, C, Covarrubias, K, Tasciotti, L & Anriquez, G, 2008. The impact of rising food prices on the poor. ESA Working Paper No. 08-07, Agricultural Development Economics Division, The Food and Agricultural Organization of the United Nations, Rome.