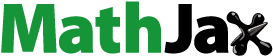
ABSTRACT
Using the 2009/10 Botswana Core Welfare Indicator Survey (BCWIS) and 2015/16 Botswana Multi-Topic Household Survey (BMTHS) datasets, this article discusses inequality in food and non-food expenses in Botswana using the Lerman & Yitzhaki [1984. A note in the calculation and interpretation of the Gini Index. Economics Letters 15, 363–8] inequality decomposition method. The main aim is to investigate how aggregate consumption inequality translates into inequality within each spending component, in order to better understand the distribution of resources held, as well as the population's living standards, and to make recommendations on redistributive government policies. The means, standard errors, and confidence intervals for the component Gini coefficients estimates are also calculated using a simple bootstrap method. The average Gini coefficient of consumption expenditure within the groups increased from 0.498 to 0.533 between 2009/10 and 2015/16, according to the findings. This increase in total expenditure inequality may be due to the increased burden of non-food spending in the household budget, which is more unequal than food spending. Food and clothes & footwear have lower Gini coefficients than other goods since they are considered necessities. Finally, this article suggests several policy options for reducing consumption expenditure inequality.
1. Introduction
Inequality, both high and growing, remains a major policy issue for both advanced and developing economies. Botswana, like other African nations, has experienced high and increasing inequality over the years, as shown by income and consumption disparities that have remained constant over time. Botswana ranks sixth among the world's most unequal nations, after South Africa, Namibia, Zambia, Lesotho, and Swaziland, due to its high-income Gini coefficient (World Bank, Citation2020). Historically, the national income inequality has always remained high even though it declined marginally between 1985/86 and 1993/94 from 0.558 to 0.539. However, it increased between 1993/94 and 2002/2003 from 0.539 to 0.573, stood at 0.605 in 2009/10 and slightly increased to 0.666 in 2015/16. Similarly, consumption inequality stood at 0.495 in 2009/10 and increased to 0.522 in 2015/16 (Statistics Botswana, Citation2013, Citation2018; World Bank, Citation2015).
With evidence that high and sustained levels of inequality will result in high social costs and lower poverty reduction gains, it is critical to break down inequality indices into their various consumption components in order to inform and direct policymakers. This allows for the identification of which components contribute the most to household consumption spending and the understanding of how changes in a specific component's consumption would impact overall consumption inequality. The latter is especially useful for evaluating the efficacy of social policies aimed at reducing consumption disparity, such as those aimed at education and health.
Despite rising inequality in Botswana, less work has been done to decompose both poverty and inequality. Amongst the few available studies is the one done by the International Poverty Centre of UNDP and BIDPA (2005) which used Kakwani’s decomposition approach of poverty changes into redistribution and growth and found out that the growth component has accounted for more poverty reduction between 1985 and 2002/03. Furthermore, considering the level of growth rate between 1985 and 2002/03, the findings indicate a substantial slowdown in poverty reduction. Interestingly, despite the strong annual economic growth rate, IPC and BIDPA (Citation2005) discovered that the Gini index of per capita consumption increased from 58.1% in 1985–86 to 64.7% in 2002–03. The conclusion reached was that the benefits of economic growth in Botswana were not spread equitably among population groups, with the non-poor benefiting proportionally more than the poor.
On the one side, Okatch et al. (Citation2013) conduct a decomposition study of income inequality by factor components in Botswana using a regression-based approach developed by Fields (Citation2003) to identify factors driving income inequality at the household level. The study's findings show that secondary school education, training, the value-added tax, the number of children in the household, and the number of working adults in the household all play a role in inequality in Botswana. Income disparity is reduced by having a primary education, being older, and owning between one and ten head of livestock (Okatch et al., Citation2013)
In addition to the studies mentioned above, the World Bank (Citation2015) analysed trends in the monetary and non-monetary aspects of poverty in Botswana, based on Household Income and Expenditure Survey of 2002/03 and the Botswana Core Welfare Indicator Survey of 2009/10. The study examined the drivers of poverty reduction, and sources of inequality by systematically looking at the demographic, labour, and human capital dimensions. Income inequality as calculated by the Gini coefficient is still high, although it has decreased slightly, from 64.7% in 2002/03 to 60.5% in 2009/10, with the majority of the decrease attributed to welfare changes in rural areas. To provide a partial explanation for inequality shifts, the World Bank (Citation2015) decomposed inequality into within-group and between-group components. In 2009/10, between-group inequality accounted for 14.8% of total inequality (as measured by the Theil inequality index) and 19.1% of total inequality (as measured by the number of children). Region and education-level variables, on the other hand, play a smaller but still significant role in determining inequality. Regional inequalities accounted for 8.3% of disparity, while education accounted for 7.8%. Gender and labour groups, which have lower between-group components, explain fewer of the discrepancies (World Bank, Citation2015).
The studies mentioned above primarily focused on the decomposition of income inequality by households, sources of income, and other related attributes. Little attention has been paid to consumption inequality and its decomposition into different components to see how total inequality translates into inequality within each expenditure component. Therefore, the objective of this study is to decompose the expenditure Gini coefficient into food and non-food components using the data sets from the 2009/10 BCWIS and the 2015/16 BMTHS.
This research is important because it aims to decompose the Gini coefficient of consumption expenditure rather than income inequality, as it has been argued in the literatureFootnote1 that income-based inequality indicators are more likely to be skewed due to market cycles and income misreporting than consumption expenditure-based inequality measures. This research also used Lerman & Yitzhaki (Citation1984, Citation1985) covariance method, which has been applied previously to study income inequality by income source in other countries. These include the United States of America (e.g. Ahearn, Johnson, and Strickland, Citation1985). Yitzhaki (Citation1990) also applied this approach to assess the progressivity of commodity taxation in Israel, while Yitzhaki and Thrisk (Citation1990) used it to determine the welfare dominance of excise taxation for Cote d'Ivoire. The main benefit of this method is that it can use individual data rather than clustered data, resulting in more reliable estimates. This method is also unaffected by the order in which each component's contributions are calculated. Another valuable aspect of this method is that the Gini decomposition can be used to measure non-parametric estimates of elasticities with respect to total consumption expenses (Yitzhaki, Citation1990; Garner, Citation1993).
The study of consumption inequality will provide crucial information on understanding household welfare and expenditure distribution in key measures of well-being such as food consumption, housing expenditure, health expenditure, and education expenditure, among others.Footnote2 Furthermore, the effect of marginal adjustments in expenses for specific goods on the inequality of total expenditures is identified in this study. This could contribute to the dissemination of useful information about the impact of various policies, such as the imposition of percentage product taxes and subsidies, on consumer purchasing habits (Garner, Citation1993).
The rest of the article is organised as follows: Section 2 details the theoretical framework of inequality, while Section 3 describes the methodology. Section 4 and 5 discuss the decomposition and the bootstrap results, respectively. Finally, Section 6 of the article provides the conclusions.
2. Theoretical background
Classical economics paved the way for the study of inequality, as Adam Smith (Citation1937) demonstrated when he stated that no society will prosper and be happy if the vast majority of its members are poor and unhappy. It continued with David Ricardo emphasising the importance of income distribution by stating that ‘political economy should be aimed at deciding the laws that govern the distribution of income’ (Bigsten, Citation1983; Atkinson, Citation1997; Gallo, Citation2002). The 1950s saw a continuation of this literature, with Lewis (Citation1954) and Kuznets (Citation1955) continuing the history of development and distribution. This classical economics has left inequality as a significant or current problem in development and policy debates, demonstrating that inequality does matter. This section, therefore, discusses the theoretical background of inequality, its different forms, measures, and properties.
In the 1970s, there was an increasing concern about the standard of living in the developing world, which expressed itself in demonstrations against the negative effects of economic development, such as pollution and natural resource depletion. The connection between economic growth and income distribution was at the centre of these concerns. Several countries that had witnessed higher-than-average growth rates realised that this had a negative effect on income distribution, leading to increased inequality and a failure to eradicate or minimise poverty (Todaro, Citation1994; Gallo, Citation2002).
The revival of neoclassical economics in the 1980s occurred in the backdrop of a debt crisis that primarily affected Latin American countries, refocusing the discussion on economic development. These discussions were also focused on the expansion of economic activity, which was followed by growing inequality and a worsening of poverty. Any redistribution, according to the neoclassical viewpoint, will be at the cost of one of the two efficient variables, resulting in economic inefficiency. As in classical economics, the key problem was the factor distribution of income (Gallo, Citation2002). All of these controversies culminated in the 1990s with the introduction of income distribution into the main body of economic research. High-income inequality can slow the accumulation of physical and human resources, which are the key sources of economic growth, according to the Inter-American Development Bank (1998–1999), and, as a result, poor income distribution hurts economic growth. These debates eventually indicated that if people’s welfare was the primary target, nations should also be concerned about the impact of economic development on income distribution (IADB, Citation1998–1999; Gallo, Citation2002). All of these discussions concluded that an undesirable economic growth is one that raises income inequality and widespread poverty, causing social unrest and political dissatisfaction, and jeopardising society's well-being. This literature relates to Botswana where we have seen high economic growth accompanied by high level of both income and consumption inequality.
2.1. Definition of inequality
Inequality refers to the capacity of elements to be compared, and it is typically based on particular characteristics that can be calculated using appropriate indexes or measures. Furthermore, inequality is calculated across the whole population, not just for those who fall below a certain level, such as those who live below the poverty line. Income, property, pay/wage, tax payments, and other continuous variables are often used to quantify inequality measures. In this context, income inequality refers to the degree to which income is distributed unequally within a group of individuals, and income may be measured on a person or household basis. Pay inequality, on the other hand, refers to the disparity in pay between employees within a corporation or among all employees in a county. Wealth, on the other hand, refers to an individual's or household's total assets. This may include financial assets, such as bonds and stocks, property, and private pension rights. Wealth inequality, therefore, refers to the unequal distribution of assets in a group of people (Stand, Citation2011).
In many debates on inequality, income or wealth are the most discussed. However, when it comes to economic wellbeing, wealth, and income are not the only important players, consumption is also vital. The definition for consumption inequality is complex because, it is normally based on expenditure data which includes annual expenses for various data sets across countries, although it is generally unclear which expenditures should be included in annual consumption. In an ideal world, the consumption indicator will capture all consumption flows for the entire year, minus any savings or deferred consumption. This would include all nondurable expenses as well as durables consumption flows. Furthermore, direct, and indirect investments, such as life insurance premiums, lumpy durable expenses, and so on, must be included in savings and deferred consumption (Crossley & Pendakur, Citation2002). This article thus, sums inequality as a state whereby people have different degrees of income or consumption.
2.2. Properties of inequality
Litchfield (Citation1999) emphasises that good inequality measures should satisfy five properties namely anonymity, scale independence, population independence, transfer principle and decomposability. Since the anonymity axiom demands that an inequality metric not be based on the labelling of individuals in an economy, attention should be centred solely on income distribution. This characteristic distinguishes the notions of inequality and justice. As a result, an inequality measure should concentrate on how wealth is distributed rather than what kind of income people deserve. The scale independence property, on the other hand, emphasises that the measure of inequality should not be influenced by uniform proportional shifts in all individuals’ income. If everyone's income in a given economy is doubled (or multiplied by some positive constant), the total measure of inequality should remain unchanged. The income inequality index should be unaffected by the overall amount of income. The population independence axiom states that the measure of inequality should not be affected by population size, so combining two similar distributions should have no effect on inequality (Shorrocks, Citation1980). In its most basic form, the transfer principle (also known as the Pigou–Dalton transfer principle) states that if any wealth is shifted from a rich individual to a poor person while maintaining the order of income ranks, the calculated inequality does not rise. At its most extreme form, however, the measured degree of inequality should actually decrease. Cowell (Citation1980, Citation1988) and Cowell & Kuga (Citation1981) also argue that there must be a consistent relationship between inequality in society as a whole and inequality in its constituent parts, emphasising the importance of a decomposable inequality measure.
2.3 . Measures of inequality
Several techniques to measure inequality in a population have notably been developed and tested over time. A popular measure of inequality is the Gini coefficient, which is discussed below.
2.3.1. The Gini coefficient
The Gini coefficient is a ratio between 0 and 1 that measures distribution of inequality. The rate of inequality increases as it gets closer to 1 and decreases as it gets closer to zero. Based on the Lorenz curve, where y-axis represents the cumulative proportion of income for a given proportion of population, i.e. the income share calculated by taking the cumulated income of a given share of the population, divided by the total income , gives the Lorenz Curve Function as follows,
(1)
(1) where
is the position of each individual in the income distribution,
is the total number of individuals in the distribution,
is the income of the
individual in the distribution,
is the total income,
is the cumulated income up to the
individual, and ranges between
for
and
for
.
It is apparent to indicate that an income distribution of a finite population of individuals is an ordered list of incomes (from the lowest to the highest) where each income yi is attached to a given individual or household
. Analytically it is given by
, where
represents a vector of individual incomes. If household incomes are considered, then to each household income there should also be attached a number
reflecting household size, in order to make meaningful comparisons among income levels: therefore,
Then based on the Lorenz curve, the Gini coefficient can then be expressed as
(2)
(2)
Other inequality measures that satisfy the inequality axioms include the well-known Theil’s T and L indexes, which both belong to the family of generalised entropy inequality measures. They allow for inequality within areas (for example, rural and urban) as well as between areas (for example, the rural-urban income gap) (World Bank, Citation2015). In addition, Atkinson (Citation1970) introduced a class of inequality measures that are commonly used around the world, and this class has a weighting parameter (ϵ) that measures aversion to inequality. The theoretical properties of Atkinson's class of inequality tests are similar to those of the extended Gini index.
The decile dispersion ratio, which is commonly used, is the ratio of the average intake of income of the richest 10% of the population divided by the average income of the bottom 10% of the population. Other percentiles can also be used to measure this ratio (for instance, dividing the average consumption of the richest 5% – the 95th percentile – by that of the poorest 5% – the 5th percentile). By expressing the income of the top 10% (the wealthy) as a multiple of that of the lowest decile (the poor), the decile ratio can be easily interpreted. It, on the other hand, ignores data on wages in the middle of the income distribution, as well as data on income distribution between the top and bottom deciles. Pearson, on the other hand, introduced the Coefficient of Variation in his 1896 work, which is a measure of dispersion relative to the mean (Porter, Citation1986). The Standard Deviation is expressed as a percentage of the arithmetic mean in this calculation of variance. The deciles and quantiles dispersion ratios are two other measures.
Among the above-mentioned measures, the Generalised Entropy class (Theil’s T and Theil’s L) helps one to decompose inequality into that which is caused by inequality within areas (e.g. urban, rural) and that which is caused by differences between areas (e.g. the rural-urban income gap). Despite the fact that the Theil index has all of the necessary properties, DeClue (Citation2017) criticises it for not having the same straightforward representation and interpretation as the Gini coefficient. Although the Gini coefficient has a number of desirable characteristics, it is difficult to distinguish the part that is due to inequalities between areas (for example, urban and rural) from the part that is due to disparities between areas (for example, the rural-urban income gap). Nonetheless, Yitzhaki (Citation1983) suggested an extended Gini coefficient that considers various aversions to inequality and allows for component inequality decomposition analysis.
Other key advantages of the Gini coefficient over alternative inequality measures are the Gini coefficient, as a statistical measure of variability, can accommodate negative wealth, which is a property that some other inequality indicators lack. Since the income of certain households can be negative, this is found to be significant when dealing with the effect of a policy change on income inequality. Another benefit of the Gini coefficient and similar terms like the Gini income elasticity is that their statistical properties are better understood than those of other inequality indicators. As a result, it is possible to determine whether a policy change's effect on income/consumption disparity is statistically important at the margin (Wodon & Yitzhaki, Citation2002).
The Gini coefficient also has a geometrical representation, allowing one to see variations in inequality between alternative distributions as well as the influence of different income or consumption sources (Wodon & Yitzhaki, Citation2002). The Gini coefficient can also be derived as an inequality measure from axioms on social justice, which is not the case for some other inequality measures. As a normative index, the Gini reflects the principle of relative deprivation, which is a sociological theory describing the level of deprivation among individuals in society, and the Gini coefficient can also be derived as an inequality measure from axioms on social justice, which is not the case for some other inequality measures (Runciman, Citation1966; Yitzhaki, Citation1979, Citation1982; Shorrocks, Citation1980, Citation1982; Ebert & Moyes, Citation2000; Wodon & Yitzhaki, Citation2002). Based on the above discussion and findings from previous research, this study uses the expenditure Gini coefficient as a measure of inequality.
3. Methodology
Different methods have been developed to decompose inequality. This study, however, adopts the Gini decomposition method introduced by Lerman & Yitzhaki (Citation1984), which was later used by Garner (Citation1993), and Çami (Citation2017). The Gini coefficient is treated as an indicator of inequality in the distribution of household expenditures in the population, and, the formula for the Household Expenditure is given as the covariance of the total expenditure , the cumulative distribution
and the mean of total expenditures
and is expressed as follows:
(3)
(3)
Then following Lerman & Yitzhaki (Citation1984, Citation1985), consumption expenditure is decomposed in components such that:
(4)
(4)
The components are food, alcohol and tobacco, clothing and footwear, housing costs, household goods and services, medical health/ health care, transport, communication, recreation and culture, education, restaurants, and hotels, and miscellaneous. represents the cumulative distribution of
and
is the mean. Therefore, the Gini coefficient of the
component is calculated as:
(5)
(5) Then utilising the cumulative distributions and averages of the expenditure components, the Gini coefficient of the Total Expenditures would be expressed as:
(6)
(6) Based on Lerman & Yitzhaki (Citation1985), we combine the equations above then show that the relative Gini coefficient can be decomposed as follows:
(7)
(7) Thus:
(8)
(8) where
is the correlation between expenditures of the
component with total expenditures,
is the Gini coefficient for each one of the expenditure components and
is the contribution to total expenses of the
component.
on the other hand, is the product of the three decomposed elements for the
component. Equation (8) indicates that any change in the variables
,
, or
will be mirrored by changes of
. The higher the value of one of these components, the more
increases, and the more the expenditure inequality increases since
. In our analysis we also measure the relative effects of inequality by calculating both the relative expenditure inequality
, and, the relative marginal effects
. Lastly, we also calculated the elasticity
for each component to determine whether it is consumed as a luxury, necessity or inferior good.
The decomposition method discussed above allows for the estimation of bootstrapped standard errors and confidence intervals to ascertain the robustness of results. Therefore, in this article bootstrapping was applied over 1000 replications to provide the bias, standard errors, and intervals for the variable estimates.
4. Data and results
4.1. Data sources
This research requires data sets that provide detailed household economic activity, and the household economic activity in Botswana is provided by the Household Income and Expenditure Survey (HIES). The HIES were conducted every 10 years, in 1985/86, 1993/94 and 2002/03, to provide data on household incomes and expenditures, and computation of Poverty Datum Lines (PDL), and to provide up to date information to update statistics required in monitoring and development planning. The HIES were improved into a Core Welfare Indicator Survey (BCWIS) in 2009/10, which was upgraded into the Botswana Multi-Topic Household Survey (BMTHS) in 2015/16. All these surveys were conducted by Statistics Botswana country-wide, and they provide a detailed account of consumption expenditures. The BCWIS was conducted between April 2009 and March 2010 and covered additional welfare measures to enable comprehensive understanding of 7732 households’ wellbeing. On one hand, the BMTHS was conducted between November 2015 and October 2016 and covered 7188 households. The indicators covered by these surveys include, among others, health status, nutrition, food security, participation/exclusion, personal security, access to and satisfaction with services provided in Botswana. Furthermore, it included households’ self-assessment poverty, community, health centre, employment/labour, and school modules.
These surveys have two formats of data- aggregated and disaggregated data. The aggregated data is with respect to the various income groups, for example, the average monthly consumption per household in a certain group, and so on. According to Idrees & Ahmad (Citation2010), this classification suppresses essential details and makes it difficult to investigate consumption inequality within households in the same income group. This research, therefore, is focused on disaggregated micro data from the two surveys due to this limitation. Furthermore, many consumption components are calculated using household expenses in many surveys that provide data on economic activity. Çami (Citation2017), on the other hand, claims that these two meanings are significantly different. Consumption that is not dependent on business transactions is occasionally excluded from expenditures, which can be a major difference, given the value of domestic output in developing and transiting countries. Furthermore, expenditure applies to the procurement of a particular product or service, while other products are not immediately consumable or have long-term benefits (Çami, Citation2017). Since the data bases do not include real household spending data, expenditureFootnote3 inequality is used as a proxy for consumption inequality in this analysis.
4.1.1. Data limitations
As pointed out in the introductions section, the previous related research mainly used the three HIES’ data sets: 1985/1986, 1992/1993 and 2002/2003. Though all these surveys were conducted by Statistics Botswana, the HIES 1985/86 vary in duration with the others. It was conducted from August 1985 to October 1986 (14 months) while the other four were conducted for 12 months each (the 1992/93 HIES: November 1993 to January 1995; the 2002/2003 HIES: July 2002 to August 2003; the 2009/10 BCWIS: April 2009 to March 2010; and the 2015/16 BMTHS: November 2015 and October 2016). These surveys vary in sample size as well: 2077, 3608, 6053, 7732 and 7188, respectively. Moreover, the 1993/94 to 2015/16 surveys stratified the country the same way: cities/towns, urban villages and rural areas, while there was no urban villages’ stratum for 1985/86. The difference in stratification between 1985/86 and other periods may affect comparability of the inequality measures and decomposition components.
While examining the food intake patterns from the HIES data sets, Buthali (Citation1997) revealed that the food eaten by households was often not the optimal diet. Not only was the food eaten different, but it was also more costly and nutritionally deficient in some cases. In the 1985 HIES, for example, a table of food specifications prepared with the aid of nutritionists from the Ministry of Health & Wellness offers what is called the ideal diet – one that is balanced in terms of calories and other nutrients. Beer, in some form, stood out as being especially significant among the goods consumed by low-income groups. Similarly, intake of carbonated beverages was high in desert regions (Buthali, Citation1997). This study finds neither of the two items as part of the ideal diet and choose not to use these data sets.
Furthermore, according to the 2002/03 commodities price list, some commodities have no costs, suggesting that the prices could not be obtained from suppliers due to factors beyond their control, such as a lack of records. These costs include clinic visits, administrative costs such as primary school and sports fees, and traditional healer consultations, which are popular in rural areas of the country. This data was therefore not included, as the lack of expenses data would affect the components expenditures, thus affect the components inequality.
Based on the limitations mentioned above, this research used only the BCWIS (2009/10) and BMTHS (2015/16) datasets. These data sets have never been used together before and are very similar in the modules and number of households. However, we recognise that the estimation of inequality is affected by the resource being measured, the data source, sample weighting, and the unit of analysis. Thus, for both datasets there were many reported zeros for the components which could bring an increase in the Gini coefficient, and could deflate the total household expenditure. In order to obtain more accurate values, we drew the sample from a subset of the total observation, by truncation and excluded those households that had reported zero total expenditure, and those households that reported only food expenditure and zeros for all other components. This resulted in a total of 4,950, and 5,047 observations from the BCWIS and BMTHS datasets, respectively.
4.2. Description of variables
gives the details of the variables used in this article. describes the variables’ variations.
Table 1. Description of components.
Table 2. Description of variables.
4.3. Decomposition analysis results
The expenditure inequality effects by budget component are presented in and .
Table 3. 2009/10 inequality effects by budget component.
Table 4. 2015/16 inequality effects by budget component.
4.3.1. Total consumption expenditure Gini coefficient
The total in column 1 ( and ) gives the overall Gini based on total consumption expenditure at 0.498 in 2009/10 and 0.533 in 2015/16. The increase in total expenditure Gini coefficients is attributed to non-food items. This adds to Botswana's distribution policy issues, as consumption spending measures how much people spend on food, housing, transportation, and other goods and services, giving a more accurate image of their circumstances and well-being.Footnote4
4.3.2. Component Gini coefficient (
)
Colum 3 in and shows the expenditure Gini coefficients of each component. Component Gini coefficients for the two periods are high in non-food items like restaurants and & hotels, health, education, transport, and recreation and culture, which implies that there is high probability that these components are highly unequally distributed compared to other components. In 2009/10, restaurants and hotels assume the highest Gini of 0.992, which goes down significantly in 2015/16 to 0.796. A bit lower Gini coefficient is observed for food for the two periods. This may also indicate a higher probability that food expenditures are being equally distributed among the population, compared to other components. The results also show that food has been replaced by non-food items in the two periods and are confirmed by the reported expenditures in the two periods.Footnote5 This is consistent with Engel's Law, which states that as a household's income increases, the budget allocation changes to the point that the budget allocated to food decreases as the household spends more on ‘luxury’ products.
4.3.3. Contribution to total consumption expenditure inequality (Ck)
Column 1 of and represents the contribution of each component to the total consumption inequality. The expenditures for food items account for only 8% of the inequality expenditures. On one hand, non-food items expenditures account for 92% of the inequality expenditures. During the two periods transport dominates the contribution to the total consumption expenditure Gini by a contribution of 36% followed by miscellaneous and household goods at 11% and 10%, respectively. Food expenditure contributes to consumption inequality by 8%. These results, however, also show that in 2009/10 amongst those components with a bit lower expenditure Gini coefficient like food and housing, contribute more to the total consumption expenditure Gini, while health, education, recreation and culture, restaurants and hotels contribute less to the total consumption expenditure Gini coefficient regardless of having high component Gini coefficients. These findings indicate that, despite low component Gini coefficients, commodities such as food and housing should not be ignored as significant measures of inclusive inequality.
4.3.4. Expenditure share and share expenditure inequality
Other two important columns in and are 2 (Expenditure Share (Sk)) and 5 (Share Expenditure Inequality (IK)). With respect to reductions in inequality, there should be considerations to change expenditures for commodities which have their expenditure shares (Sk) greater than their shares of expenditure inequality (Ik). This emphasises that for inequality to go down, there is need for a change in expenditure of commodities like food, clothes & footwear, housing, and communication.
4.3.5. Expenditure elasticity
Column 8 in both and shows expenditure elasticity figures. When the Gini coefficient is high, it means high overall consumption expenses and high individual expenditure on one commodity. The expenditure elasticity, on the other hand, represents both the concentration and correlation of expenses. The higher the commodity Gini, given the correlation, the higher the expenditure elasticity (Garner, Citation1993). When all elasticity figures are positive, it indicates that all the expenditure components are normal goods. Restaurants & hotels, recreation & culture, transportation, miscellaneous, household goods & services, and education have high expenditure elasticities in both 2009/10 and 2015/16. Specifically, restaurants & hotels, recreation & culture, transportation, miscellaneous, education, household goods & services, and clothing & footwear have the highest elasticity values (>1) in 2009/10. This suggests that increasing spending on these components would increase the expenditure consumption Gini coefficient significantly. In comparison to other goods, alcohol and tobacco, food, housing, and health have relatively low elasticities (<1). This indicates that these items are regarded as necessities.
In 2015/16, the same pattern is observed with transport (e increased), restaurants & hotels, recreation s& culture, miscellaneous, education, household goods and services having elasticity values greater than 1. Moreover, expenditure elasticity for alcohol & tobacco, health and housing significantly increased. For the same period, relatively low expenditure elasticities for food (decreased), alcohol and tobacco, and clothing and footwear are observed.
In terms of policy and regulation, there is a need for caution when it comes to addictive substances, since elasticity also indicates whether companies will pass on higher costs to customers, and addictive substances like alcohol and tobacco appear to fall into this category. For example, among daily smokers who are somewhat addicted, demand for cigarettes is relatively inelastic, and raising the price of cigarettes may not really reduce the number of cigarettes smoked by adults. As a result, if the government raises taxes on tobacco producers, the supply of cigarettes will decrease and prices will rise, and as a result, taxes will primarily be passed on to customers in the form of higher prices. Higher cigarette taxes would bring in more revenue for the government but will have no impact on the number of people who smoke, and quantity of smoking in general (Greenlaw & Taylor, Citation2017).
4.3.6. Relative marginal effects
We also calculated the relative marginal effects, and the results are shown in column 7 of and . A positive marginal effect coefficient indicates that an increase in expenditure for the component will increase the inequality in consumption expenditure. This implies that any increase in the expenditures for transport, recreation and culture, restaurants and hotels, household goods and services, miscellaneous, housing, education and health will increase the Gini consumption inequality. However, a rise in the expenditures of food, alcohol and tobacco, clothing and footwear, and communication will bring a decrease in the Gini of consumption expenditure. These results confirm the discussion under shares of expenditure and inequality above. That is, these results imply that increases in expenditures of food items, clothes & footwear, communication and miscellaneous would decrease overall inequality n total expenditures. It is important to note, however, that this study did not consider the household income, costs, and/or household prices, so there was no way to argue about the best way to maximise or optimisation of household expenses and consumption.
5. Bootstrap results
Since the samples were truncated and moderated to 4950 and 5047 respectively, the decomposition analysis of inequality, faced an important issue related to the accuracy of our parameters. Therefore, bootstrap is applied as a resampling method that enables assessment of the accuracy of an estimator by random sampling with replacement from an original dataset (see Efron & Tibshirani, Citation1986, Citation1994; Efron, Citation1977). The results indicate the probabilistic sensitivity analysis through the bootstrap approach and the results are provided in and . The simple bootstrap allows assigning measures of accuracy such as bias, variance, and confidence intervals to the sample estimates (Mills & Zandvakili, Citation1997). Columns 3 and 4 show the bias and standard errors of the expenditure components, respectively. On one hand, column 5 indicates the confidence interval within which the marginal effect lies. The difference between the marginal effect in and and the observed change in and , is very minimal and almost non-existent which generally indicates the significance of the results.
Table 5. Bootstrap Results – 2009/10.
Table 6. Bootstrap Results: 2015/16.
6. Concluding remarks and policy implications
This article decomposes the Botswana consumption expenditure Gini into categories of food and non-food components, based on the evidence that high and sustained levels of inequality will result in significant social costs and lower poverty reduction efforts gains. In 2009/10, the consumption expenditure Gini coefficient was 0.498, and in 2015/16, it was 0.533. Necessities like food, housing, and clothing & footwear contribute more to the overall inequality, and at the same time show low levels of elasticity to expenditure changes. This result may have policy implications in that it could be necessary to reduce taxes or exclude essentials like food, or essential goods and services from the tax base in order to increase their expenditures. Households’ disposable income rises when taxes are reduced, leaving them with more capital. Tax adjustment policy interventions have been used in countries such as the United States to provide relief on household spending by exempting a number of goods from taxation. Food for home consumption, utilities for heating and cooling, and prescription drugs and medical services are among these commodities (Case & Ebel, Citation1989; ACIR, Citation1990; Garner, Citation1993).
Luxury goods, on the other hand, have high expenditure elasticities and positive marginal effects. As a result, raising taxes on commodities like transportation and leisure & culture could aid in reducing consumption inequality, as higher taxes reduce disposable income, resulting in lower spending on those luxury items. Overall, this result suggests that improving tax progressivity is important for reducing consumption expenditure inequalities.
Aside from tax policy considerations, increased public spending in priority sectors such as education, health, and housing is needed to provide disadvantaged groups such as the youth and the poor with more opportunities, to counter existing inequalities, and to avoid further increases. For example, differences in early education and education quality may have led to the persistence of inequality across generations, so increased investment in early childhood will improve economic mobility and, in turn, reduce consumption inequality. China and India, for example, are two of the countries in the world that are implementing strategies that raise the income of low-income households (Salidjanova, Citation2013). These strategies are aimed at boosting minimum wages to at least 40% of average salaries, loosen controls on lending and deposit rates and, and increase spending on education and affordable housing.
To counter housing expenditure inequality, policies that significantly increase building assets for working families must be promoted. Haas Institute (Citation2014) found that policies and programmes that promote higher savings rates and lower the cost of building assets for working- and middle-class households can help struggling and disadvantaged families achieve greater economic security. In the United States, for example, programmes that automatically enrol employees in retirement plans and offer a savings credit or a federal match for retirement savings accounts have been developed to assist lower-income households in building wealth (Haas Institute, Citation2014). Furthermore, in some countries, policies that increase access to equitable, low-cost financial services and home ownership have been seen as means of reducing inequality by increasing income.
Finally, more research is required to resolve both income and consumption disparities in Botswana, as well as regional disparities in the distribution of household consumption expenditures across different components. Considering the new adopted tax strategies suggested in the Botswana Budget of 2021/22, this research could use other methods such as hackman selection when there is new data. Such research would aid in the investigation of changes in consumer expenditure distribution over time, as well as the examination of possible factors leading to either the increase or decline of inequality.
Disclosure statement
No potential conflict of interest was reported by the author(s).
Additional information
Funding
Notes
1 See Meyer & Sullivan (Citation2003), Meyer, James and Sullivan (Citation2008) and Krueger & Perri (Citation2005).
2 This information would in turn be useful in understanding the various implications of inequality, such as the impact on investment in human capital through education and health (Damásio & Neves, Citation2015).
3 These are household spending expenses on goods and services over a 30-day span during the survey period, excluding capital formation and investment/savings, insurance, income tax, loan repayments, and cash transfers.
4 Consumption is the sole end and purpose of all production and the welfare of the producer ought to be attended to, only so far as it may be necessary for promoting that of the consumer (Smith, Citation1937).
5 The expenditure data reported in 2009/10, confirms a change in order of importance, with transport, food and housing being allocated the highest shares of 20.7%, 17.7% and 13.6% of the consumption expenditure budget in cities/towns only, in urban villages and rural areas households increased their share allocated to transport at lesser magnitude such that food remained the most important commodity. Likewise, in 2015/16, at national level, households allocated significant proportions of their consumption expenditure to transport, at 23.9%, followed by housing costs and food at 17.8% and 12.8% respectively. In cities/towns, households allocated 22.2% of their consumption expenditure to transport, followed by housing costs at 19.7% and miscellaneous items at 9.7%. For urban villages, the largest consumption expenditure was allocated to transport at 25.0%, followed by housing costs and food at 18.8% and 12.8% respectively. In rural areas, the largest share of consumption expenditure was allocated to transport at 24.7% followed by food at 21.4% and housing costs at 12.4%. Evidently, food was replaced by transport and transport consumables like fuel in the order of importance.
References
- Ahearn, M, Johnson, J & Strickland, R, 1985. The distribution of income and wealth of farm operator households. American Journal of Agricultural Economics, Agricultural and Applied Economics Association 67(5), 1087–1094.
- Advisory Commission on Intergovernmental Relations, 1990. Significant features of fiscal federalism. Vol. 1, Budget Processes and Tax Systems, M-169.
- Atkinson, AB, 1970. On the measurement of inequality. Journal of Economic Theory 2, 244–263. Faculty of Economics and Politics, University of Cambridge, England.
- Atkinson, AB, 1997. Bringing income distribution in from the cold. The Economic Journal 107, 297–321.
- BIDPA and IPC, 2005. Poverty status report for Botswana: Incidence, trends and dynamics. UNDP Research Project.
- Bigsten, A, 1983. Income distribution and development: Theory, evidence, and policy. Heinemann, London.
- Buthali, D, 1997. Poverty measurement in Botswana. Central Statistics Office Botswana Presentation at a Seminar on Poverty Statistics in Santiago. 7–9 May 1997.
- Çami, O, 2017. Consumption inequality: An analysis based on the decomposition of the Gini coefficient of expenditures in Albania. Microeconomic Research Division, Research Department, Tirana, Bank of Albania.
- Case, B & Ebel, RD, 1989. Using state consumer tax credits for achieving equity. National Tax Journal 42, 323–337.
- Cowell, FA & Kuga, K, 1981. Additivity and the entropy concept: An axiomatic approach to inequality measurement. Journal of Economic Theory 25, 131–143.
- Cowell, FA, 1980. On the structure of additive inequality measures. Review of Economic Studies XLVII, 521–531.
- Cowell, FA, 1988. Inequality decomposition: Three bad measure. Bulletin of Economic Research 40, 309–312.
- Crossley, T & Pendakur, K, 2002. Consumption inequality. McMaster University and Simon Fraser University. August 2002.
- Damásio, B & Neves, D, 2015. Lerman: A stata module to decompose inequality using sampling weights. Portuguese Stata Users Group Meeting.
- DeClue, D, 2017. A comprehensive study of issues affecting poverty and inequality in Brazil. Partial Fulfilment of the Requirements for the Degree Masters of Science in the School of Economics, Georgia Institute of Technology.
- Ebert, U & Moyes, P, 2000. An Axiomatic characterization of Yitzhaki’s index of individual deprivation. Economics Letters 68(3), 263–70.
- Efron, B, 1977. Bootstrap methods: Another look at the Jackknife. The Annals of Statistics 7(1), 1–26. The 1977 Rietz Lecture, Stanford University.
- Efron, B & Tibshirani, R, 1986. Bootstrap methods for standard errors, confidence intervals, and other measures of Statistical accuracy. Statistical Science 1, 54–75.
- Efron, B & Tibshirani, R, 1994. An Introduction to the Bootstrap. Boca Raton, London, New York, and Washington, DC, Chapman & Hall/CRC. Available: https://cds.cern.ch/record/5526679/files/04120423_TOC.pdf.
- Fields, GS, 2003. Accounting for income inequality and its change: a new method, with application to the distribution of earnings in the United States. Research in Labour Economics 22, 1–38.
- Gallo, C, 2002. Economic growth and income inequality: Theoretical background and empirical evidence. Working Paper No. 119. Development Planning Unit, University College London.
- Garner, T, 1993. Consumer expenditures and inequality: An analysis based on decomposition of the Gini coefficient. The Review of Economics and Statistics 75(1), 134–8.
- Greenlaw, SA & Taylor, T, 2017. Principles of macroeconomics. OpenStax, Rice University, Rice University.
- Haas Institute, 2014. Responding to rising inequality policy interventions to ensure opportunity for all. Policy, brief. Haas Institute for a Fair and Inclusive Society University of California, Berkeley, CA.
- IADB, 1998-1999. Facing up to inequality in Latin America. Economic and Social Progress in Latin America, Washington.
- Idrees, M & Ahmad, E, 2010. Measurement and decomposition of consumption inequality in Pakistan. The Lahore Journal of Economics 15(2), 97–112.
- Krueger, D & Perri, F, 2005. Does income inequality lead to consumption inequality? Evidence and theory. NBER Working Paper No. 9202. Issued in September 2002. NBER Program(s): Economic Fluctuations and Growth, Labour.
- Kuznets, S, 1955. Economic growth and income inequality. American Economic Review 45, 1–28.
- Lerman, RI & Yitzhaki, S, 1984. A note in the calculation and interpretation of the Gini index. Economics Letters 15, 363–368.
- Lerman, RI & Yitzhaki, S, 1985. Income inequality effects by income source: A new approach and applications to USA. Review of Economics and Statistics 67, 151–156.
- Lewis, WA, 1954. Economic development with unlimited supplies of labour. Manchester School of Economics and Social Studies 22, 139–181.
- Litchfield, JA, 199. Inequality: Method and tools. The World Bank, Washington DC. Available on http://www.worldbank.org/poverty/inequal/index.htm.
- Meyer, BD & Sullivan, JX, 2003. Measuring the well-being of the poor using income and consumption. The Journal of Human Resources 38, 180–220.
- Meyer, BD, James X & Sullivan, JX, 2008. Changes in the consumption, income, and well-being of single mother headed families. American Economic Review 98(5), 2221–2241.
- Mills, JK & Zandvakili, S, 1997. Statistical inference via bootstrapping for measures of inequality. Journal of Applied Econometrics 12(2), 133–150.
- Okatch, ZA, Siddique, A & Rammohan, A, 2013. Determinants of income inequality in Botswana. Economics Discussion/Working Papers. The University of Western Australia, Department of Economics.
- Porter, TM, 1986. The rise of statistical thinking. 1820-1900. Princeton Univ. Press, Princeton, NJ.
- Runciman, WG, 1966. Relative deprivation and social justice: A study of attitudes to social inequality in twentieth-century England. University of California Press, Berkeley, CA.
- Salidjanova, N, 2013. China’s new income inequality reform plan and implications for rebalancing U.S. China Economic and Security Review Commission, Washington, DC.
- Shorrocks, AF, 1980. The class of additively decomposable inequality. Econometrica 48(3), 613–625.
- Shorrocks, AF, 1982. Inequality decomposition by factor components. Econometrica 50, 193–211.
- Smith, A, 1937. The wealth of nations. 1937 Modern Library Edition, pp. 625.
- Stand, DW, 2011. An overview of growing income inequalities in OECD countries: Main findings.
- Statistics Botswana, 2013. Botswana core welfare main report, Volume 1. Gaborone, Botswana.
- Statistics Botswana, 2018. Botswana multi-topic household survey. Report 2015/16. Gaborone.
- Todaro, Michael., 1994. Economic Development. Fifth edition, Longman Group.
- Wodon, Q & Yitzhaki, S, 2002. Inequality and social welfare. World Bank, April.
- World Bank, 2020. Gini Index Estimates. Development Research Group. https://data.worldbank.org/indicators/SI.POV.GINI.
- World Bank Institute, 2015. Botswana poverty assessment report. Report No. 88473-BW.
- Yitzhaki, S, 1979. Relative deprivation and the Gini coefficient. Quarterly Journal of Economics 93(2), 321–24.
- Yitzhaki, S, 1982. Relative deprivation and Economic welfare. European Economic Review 17, 99–113.
- Yitzhaki, S, 1983. On an extension of the Gini inequality index. International Economic Review 24(3), 617–28.
- Yitzhaki, S, 1990. On the progressivity of commodity taxation. Working Paper No. 187, Department of Economics, Hebrew University, Jerusalem, Israel.
- Yitzhaki, S & Thrisk, W, 1990. Welfare dominance and the design of excise taxation in the cote d'Ivoire. Journal of Development Economics 33, 1–18.