ABSTRACT
This paper focuses on estimation of a quadratic functional (QF) of a random signal in dynamic systems described by a linear stochastic differential equations. The QF represents a quadratic form of state variables, which can indicate useful information of a target system for control. The optimal (in mean square sense) and suboptimal estimators of a QF represent a function of the Kalman estimate and error covariance. The proposed estimation algorithms have a closed-form estimation procedure. The quadratic estimators are studied in detail, including derivation of the exact formulas for mean square errors. The obtained results we demonstrate on practical example, and comparison analysis between optimal and suboptimal estimators is presented.
Research highlights
▸ | An optimal mean square estimator for an arbitrary QF in linear stochastic systems is derived. | ||||
▸ | The proposed estimator is a comprehensively investigated, including derivation of matrix equation for its mean square error. | ||||
▸ | Performance of the optimal and suboptimal estimators is illustrated on theoretical and practical examples for real QFs. |
DISCLOSURE STATEMENT
No potential conflict of interest was reported by the authors.
Additional information
Funding
Notes on contributors
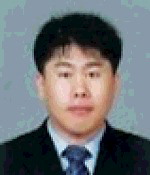
Il Young Song
Il Young Song received the BS degree from the Department of Control and Instrumentation Engineering, Changwon National University, Korea, in 2007, and the MS degree from the School of Information and Mechatronics, Gwangju Institute of Science and Technology (GIST), Gwangju, in 2008. He received the PhD degree from the Department of Information and Communications, GIST in 2012. He is currently a senior researcher in Hanwha Corporation R&D Center, Pangyo, Korea.
E-mail: [email protected]
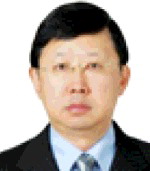
Vladimir Shin
Vladimir Shin received the BSc and MSc degrees in applied mathematics from Moscow State Aviation Institute, Moscow, Russia, in 1977 and 1979, respectively. In 1985, he received the PhD degree in mathematics from the Institute of Control Science, Russian Academy of Sciences, Moscow. From 1984 to 1999, he was the Head of the Statistical Methods Laboratory with the Institute of Informatics Problems, Russian Academy of Sciences. During 1995, he was a visiting professor at the Department of Mathematics, Korea Advanced Institute of Science and Technology, Daejeon, Korea. From 1999 to 2002, he was a member of the technical staff at the Samsung Institute of Technology. He is currently a professor with the Department of Information and Statistics, Gyeongsang National University, Jinju, Korea. His research interests include estimation, filtering, tracking, data fusion, stochastic control, identification, and other multidimensional data processing methods.
E-mail: [email protected]
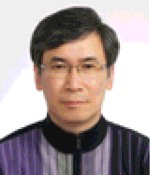
Won Choi
Won Choi received his PhD degree at Sung Kyun Kwan University under the direction of Yeong Don Kim. Since 1993 he has been at the University of Incheon. In 1996 and 2003, he was in Steklov Mathematical Institute of Russia and University of Iowa, respectively. His research interests include stochastic processes and bio-mathematics.
E-mail: [email protected]