ABSTRACT
Genetic algorithms (GAs) have been widely used in solving multiobjective optimization problems (MOP). The foremost hindrance limiting strength of GA is the large number of nondominated solutions and the computational complexity involved in selecting a preferential candidate among the set of nondominated solutions. In this paper, we analyze the approach of applying aggregation operator in place of density-based indicator mechanism in cases where Pareto dominance method fails to decide the preferential solution. We also propose a new aggregation function () and compare the results obtained with prevailing aggregation functions suggested in the literature. We demonstrate that the proposed method is computationally less expensive with overall complexity of
. To show the efficacy and consistency of the proposed method, we applied it on different, two- and three-objective benchmark functions. Results indicate a good convergence rate along with a near-perfect diverse approximation set.
DISCLOSURE STATEMENT
No potential conflict of interest was reported by the authors.
Additional information
Notes on contributors
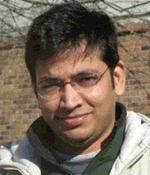
Muneendra Ojha
Muneendra Ojha is assistant professor in the Department of Computer Science and Engineering at International Institute of Information Technology – Naya Raipur, Chattisgarh, India. He holds PhD from Indian Institute of Information Technology – Allahabad, India and MS (Research) from University of Missouri, Columbia, USA. His area of research is machine learning, multiobjective optimization, evolutionary algorithms and multiagent systems.
E-mail: [email protected]
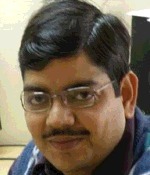
Krishna Pratap Singh
Krishna Pratap Singh is assistant professor in the Department of Information Technology at Indian Institute of Information Technology-Allahabad. He holds PhD from Indian Institute of Technology, Roorkee. His current research interests include machine learning, pattern recognition, multiobjective optimization, and evolutionary algorithms.
E-mail: [email protected]
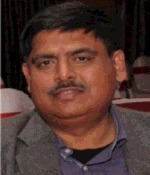
Pavan Chakraborty
Pavan Chakraborty is associate professor in the Department of Information Technology at Indian Institute of Information Technology-Allahabad. He holds PhD from Indian Institute of Astrophysics. His current research interests include robotics, image processing, graphics, data analysis, and parallel and distributed computing.
E-mail: [email protected]
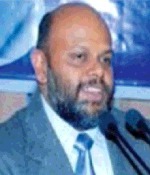
Shekhar Verma
Shekhar Verma is professor in the Department of Information Technology at Indian Institute of Information Technology-Allahabad. He holds PhD from Indian Institute of Technology, BHU. His current research interests include wireless sensor networks, cryptography and cryptanalysis, network security and machine learning.
E-mail: [email protected]
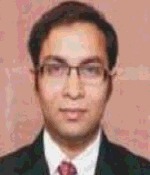
Purnendu Shekhar Pandey
Purnendu Shekhar Pandey is assistant professor in BML Munjal University. He is pursuing PhD from Indian Institute of Information Technology – Allahabad, India. He holds BTech (CS) and MTech (Software Engineering) degrees. His areas of specialization include mobile IPv6, wireless network, network coding, and cellular networks. He is a professional member of various Technical bodies such as ACM, IEEE, and IDES.
E-mail: [email protected]