ABSTRACT
Handwritten numeral recognition has gained much interest in recent times because of its diverse application potentials. Bangla and Hindi are the two major languages in Indian subcontinent and a large number of population in vast land scape uses Bangla and Devnagari numeral scripts of these two languages. Well-performed handwritten numeral recognition system for Bangla and Devnagari is challenging because of similar shaped numerals in both scripts; few numerals differ from their similar ones with a very few variation even in printed form. In this study, convolutional neural network (CNN) based two different methods have been investigated for better recognition of Bangla and Devnagari handwritten numerals. Both the methods use rotation-based generated patterns along with ordinary patterns to train CNN but in two different modes. In multiple CNN case, three different training sets (one with ordinary patterns and two with clockwise and anti-clockwise rotation-based generated patterns) are prepared; three different CNNs are trained individually with each of these training sets; and their decisions are combined for final system decision. On the other hand, in the case of single CNN, combination of above three training sets is used to train one CNN. A moderated pre-processing is also employed while generating patterns from the scanned images. The proposed methods have been tested on prominent benchmark handwritten numeral datasets and have achieved remarkable recognition accuracies. The achieved recognition accuracies are found better than reported recognition accuracies of prominent existing methods; and such outperformance mounted proposed methods as better recognition systems. Moreover, CNN's performance improvement due to use of generated patterns has also been clearly identified from the presented experimental results.
ACKNOWLEDGEMENT
The authors would like to express gratitude to Dr Ujjwal Bhattacharya (Computer & Communication Sciences Division, Indian Statistical Institute, Kolkata, India) for providing the benchmark dataset used in this study. The authors would like to thank the anonymous reviewers for their constructive comments and insightful suggestions on the paper which have helped to improve the quality of the paper.
DISCLOSURE STATEMENT
No potential conflict of interest was reported by the authors.
Additional information
Notes on contributors
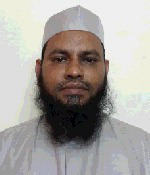
M. A. H. Akhand
M. A. H. Akhand received his BSc degree in electrical and electronic engineering from Khulna University of Engineering and Technology (KUET), Bangladesh in 1999, the ME degree in human and artificial intelligent systems in 2006, and the Doctoral degree in system design engineering in 2009 from University of Fukui, Japan. He joined as a lecturer at the Department of Computer Science and Engineering at KUET in 2001, and is now a professor. He is also head of Computational Intelligence Research Group of this department. He is a member of Institution of Engineers, Bangladesh (IEB). He has more than 40 refereed publications. His research interest includes artificial neural networks, evolutionary computation, bioinformatics, swarm intelligence and other bio-inspired computing techniques. Dr Akhand received several best paper prizes in international conferences.
E-mail: [email protected]
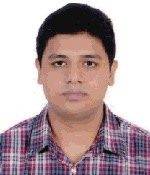
Mahtab Ahmed
Mahtab Ahmed received his BSc and MSc degree in computer science and engineering (CSE) from Khulna University of Engineering and Technology (KUET), Bangladesh in 2014 and 2017, respectively. Currently, he is serving as a lecturer in the Department of CSE, KUET. His research interest includes pattern recognition, speech and image processing and advanced machine learning.
E-mail: [email protected]
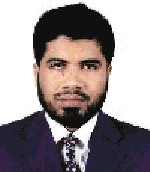
M. M. Hafizur Rahman
M. M. Hafizur Rahman received his BSc degree in electrical and electronic engineering from Khulna University of Engineering and Technology (KUET), Khulna, Bangladesh, in 1996. He received his MSc and PhD degree in Information Science from the Japan Advanced Institute of Science and Technology (JAIST) in 2003 and 2006, respectively. Dr Rahman is now an assistant professor in the Department of Computer Science, KICT, IIUM, Malaysia. Prior to join in the IIUM, he was an associate professor in the Department of CSE, KUET, Khulna, Bangladesh. He was also a visiting researcher in the School of Information Science at JAIST and a JSPS postdoctoral research fellow at Research Center for Advanced Computing Infrastructure, JAIST & Graduate School of Information Science (GSIS), Tohoku University, Japan in 2008 and 2009 & 2010–2011, respectively. His current research includes parallel and distributed computer architecture, hierarchical interconnection networks and optical switching networks. Dr Rahman is member of IEICE of Japan and IEB of Bangladesh.
E-mail: [email protected]
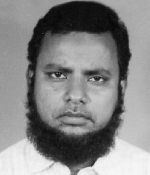
Md. Monirul Islam
Md. Monirul Islam received the BSc degree from the Khulna University of Engineering and Technology (KUET), Khulna, Bangladesh, in 1989, the ME degree from the Bangladesh University of Engineering and Technology (BUET), Dhaka, Bangladesh, in 1996, and the PhD degree from the University of Fukui, Fukui, Japan, in 2002. He was a lecturer and assistant professor from 1989 to 2002 with KUET. He moved to BUET as an assistant professor of computer science and engineering (CSE) in 2003, where he is currently a professor. He was also Head of the Department of CSE, BUET. He has also worked as a visiting associate professor supported by the Japanese Society for Promotion of Sciences at the University of Fukui. He has more than 80 refereed publications. His major research interests include evolutionary robotics, evolutionary computation, neural networks, machine learning, pattern recognition, and data mining. Dr Islam won several best paper prizes in international conferences.
E-mail: [email protected]