ABSTRACT
In this paper, a target-tracking algorithm based on improved unscented particle filter with the Markov chain Monte Carlo (MCMC) is proposed. In the proposed method, the improved unscented Kalman filter (UKF) is used to generate the proposal distribution, and particle swarm optimization (PSO) integrates into the UKF proposal. Moreover, the sample impoverishment created by resampling step is restrained with MCMC move step after the resampling. Experiments are presented to evaluate the performance of the proposed algorithm. The results show that the proposed algorithm has more significant advantages in tracking accuracy than other classical algorithms.
Additional information
Notes on contributors
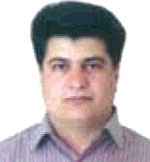
R. Havangi
R. Havangi received his MS and PhD degrees from the K.N. Toosi University of Technology, Tehran, Iran, in 2003 and 2012, respectively. He is currently an Associate Professor of control systems with the Department of Electrical and Computer Engineering, University of Birjand, Birjand, Iran. His main research interests are inertial navigation, integrated navigation, estimation and filtering, evolutionary filtering, simultaneous localization and mapping, fuzzy, neural network, and soft computing.
E-mail: [email protected]