ABSTRACT
Parkinson's disease (PD) has affected millions of people worldwide and is more prevalent in people, over the age of 50. Even today, with many technologies and advancements, early detection of this disease remains a challenge. This necessitates a need for the machine learning-based automatic approaches that help clinicians to detect this disease accurately in its early stage. Thus, the focus of this research paper is to provide an insightful survey and compare the existing computational intelligence techniques used for PD detection. To save time and increase treatment efficiency, classification has found its place in PD detection. The existing knowledge review indicates that many classification algorithms have been used to achieve better results, but the problem is to identify the most efficient classifier for PD detection. The challenge in identifying the most appropriate classification algorithm lies in their application on local dataset. Thus, in this paper three types of classifiers, namely, Multilayer Perceptron, Support Vector Machine and K-nearest neighbor have been discussed on the benchmark (voice) dataset to compare and to know which of these classifiers is the most efficient and accurate for PD classification. The Voice input dataset for these classifiers has been obtained from UCI machine learning repository. ANN with Levenberg–Marquardt algorithm was found to be the best classifier, having highest classification accuracy (95.89%). Moreover, we compared our results with those obtained by Resul Das [“A comparison of multiple classification methods for diagnosis of Parkinson Disease,” Expert Systems and applications, vol. 37, pp 1568–1572, 2010].
Additional information
Notes on contributors
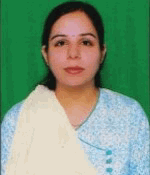
Gunjan Pahuja
Gunjan Pahuja received MTech from Guru Jambheshwar University of Science and Technology in 2006. Currently, she is pursuing her PhD in the department of Computer Science and Engineering from Dr A P J Abdul Kalam University, Lucknow. Her research focuses on machine learning and medical image processing. She is a member of ISTE. Corresponding author. Email: [email protected]
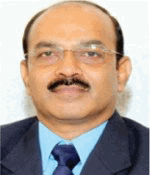
T. N. Nagabhushan
T N Nagabhushan received his master’s degree and PhD in electrical engineering from Indian Institute of Science, Bangalore in the year 1989 and 1996, respectively. He has over 32 years of experience in teaching, research and industry besides holding the position of principal. His main area of focus is machine learning, development of new tools for supervised learning and applications to image processing. He is a member of ISTE and CSI. Email: [email protected]