Abstract
Hybrid cloud has found broad acceptance in organizations and in the field of research. Most of the organizations with its own data center maneuver a hybrid cloud model. The organization utilizes its private resources to compute its maximum load. But then move to the public cloud when infrastructure within its premises is inadequate to handle the peak workloads. The fluctuations in workloads lead to the widespread acceptance of pay-as-you-go cloud resource model. In this article, we describe the Adaptive Multiobjective Resource Selection Model (AMORSM) that automatically selects the resources from the private as well as public clouds for the execution of an application within user-specified deadline. The AMORSM is implemented and integrated with Gravitational Search Algorithm (GSA) enhanced with an adaptive elitist NSGA-II (Non-Dominated Sorting Genetic Algorithm-II). The proposed algorithm is used to accomplish multiple conflicting objectives such as minimum execution time, reduction in execution cost and energy consumption by obtaining Pareto frontier optimal solution. The hybrid elitist NSGA-II (A-NSGA2 with GSA) produces number of non-dominated solution satisfying the criteria, in order to facilitate wider preferences to the cloud consumer. It also uses adaptive acceleration coefficient to maintain the diversity in the frontiers. Our simulation demonstrates 32% improvement in performance and cost trade-off optimization, in hybrid cloud environment when compared with standard NSGA-II and SPEA multiobjective optimization algorithm.
Additional information
Notes on contributors
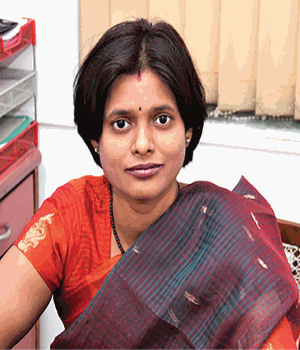
Ketaki Bhalchandra Naik
Ketaki Bhalchandra Naik received her bachelor's degree in computer engineering from Shivaji University (Kolhapur), Maharashtra, India, in 2001, and obtained her master's degree in computer engineering from Bharati Vidyapeeth Deemed University (Pune), Maharashtra, India, in 2008. At present, she is a PhD candidate in the Computer Science and Engineering Department at Sathyabama University, Chennai, Tamil Nadu, India. Corresponding author. Email: [email protected]
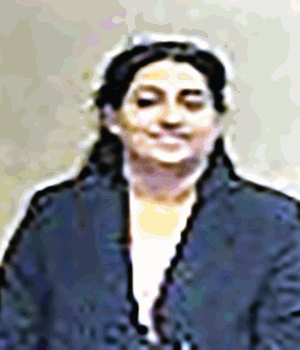
G. Meera Gandhi
G Meera Gandhi is working as professor in the Department of Computer Science and Engineering, Sathyabama Institute of Science and Technology, Chennai. She had served for 22 years in teaching and industry. Her research interests are information security and neural networks. She is also interested in the areas of data mining, machine learning, big data analytics, artificial intelligence, genetic computing and remote sensing and spatial data analysis. Several invited talks in machine learning, data mining have been delivered in different colleges. She has published many papers in international/national journals and proceedings. She has also participated and presented papers in international and national conferences. She is an active reviewer in many reputed journals. Email: [email protected]
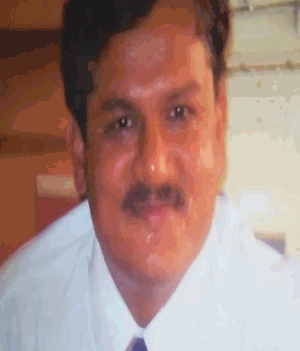
S. H. Patil
S H Patil received the bachelor's degree in computer engineering from Shivaji University (Kolhapur), India. He obtained his Master of Engineering from University of Pune (India) and the PhD degree from Bharati Vidyapeeth Deemed University (Pune), India. He is currently a professor in the Bharati Vidyapeeth University College of Engineering, Pune. His research interests include the operating system, distributed system, and cloud computing. Email: [email protected]