Abstract
Feature extraction and classification plays a crucial role in the automated analysis of breast ultrasound (BUS) images. Due to varying sonographic characteristics of benign and malignant lesions, the texture and shape features are mostly employed for designing computer-aided diagnosis (CAD) systems of BUS images. The existing CAD systems use features that are extracted either from the lesion segmented area obtained through segmentation techniques or a rectangular region of interest (ROI) extracted under the guidance of expert Radiologists. However, the significance of features extracted from region comprising only the lesion area is still little explored. This paper investigates the significance of features extracted from the lesion area, lesion surrounding area and rectangular ROI for classification of BUS images. The experiments were conducted on the database of 294 BUS images (104 benign and 190 malignant). Initially, the acquired BUS images were preprocessed through speckle reducing anisotropic diffusion (SRAD) for speckle noise removal. The preprocessed images are segmented using a hybrid segmentation approach including a combination of region-based active contour driven by region-scalable fitting (RBACM-RSF) model and multi-scale Gaussian kernel fuzzy c-means clustering with spatial bias correction (MsGKFCM_S) for getting ROI confined area. The segmented images were further partitioned into two parts (lesion area and lesion surrounding area). Subsequently, a total of 457 texture and shape attributes were extracted from within the lesion area, lesion surrounding area and rectangular ROI comprising of both lesion and its surrounding area. The significance of these features is evaluated using different classifiers (i.e. support vector machine (SVM), Back-propagation artificial neural network (BPANN), Random Forest, AdaBoost). The results indicate that features extracted from within lesion area achieve a maximum classification accuracy of 98.980% with the lowest computational time when linear kernel-based SVM is used.
Acknowledgements
We are thankful to Dr Satyabhuwan Singh Netam (Associate Professor and Head of Department) and Dr Bhagyashri, Dr Bhimrao Ambedkar Memorial Government Medical Hospital, Raipur, Chhattisgarh, India, for providing us the data. We are also thankful to ethical committee NIT Raipur for providing ethical permission.
ETHICAL APPROVAL
All procedures performed in this study involving human participants were in accordance with the ethical standards of the institutional and/or national research committee and with the 1964 Helsinki declaration and its later amendments or comparable ethical standards.
Additional information
Notes on contributors
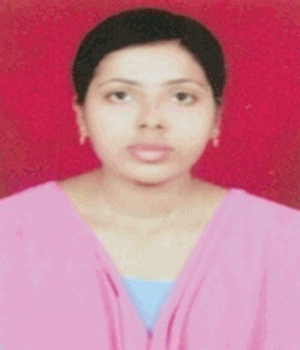
Lipismita Panigrahi
Lipismita Panigrahi, was born in India in 1989. She received her master’s degree with first class in computer applications from SOA University, Odisha, India in 2012. She is currently regular PhD scholar in the Department of Computer Applications, NIT Raipur, India. She has around 3.5 years of teaching and research experience. Her research interests include digital image processing and analysis, data mining and machine learning. She has received Chhattisgarh Young Scientist Award-2015. Email: [email protected]
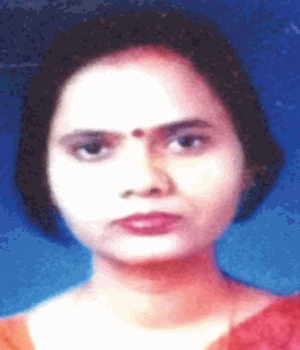
Kesari Verma
Kesari Verma, received her master’s degree with first class honors in computer applications from Government Engineering College Raipur, India in 1998 and her PhD degree from Pt RSU Raipur, India in 2007. She is currently an assistant professor in the Department of Computer Applications, NIT Raipur, India. She has around 16 years of teaching and research experience. She has around 40 paper in international journal and conferences. Her research interests include digital image processing and analysis, data mining and machine learning. Email: [email protected]
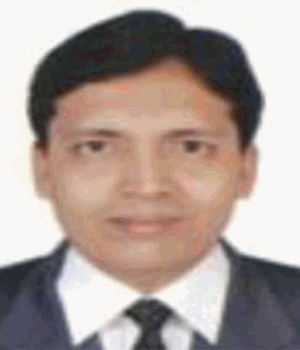
Bikesh Kumar Singh
Bikesh Kumar Singh, was born in India in 1981. He received his PhD degree from Department of Biomedical Engineering, NIT Raipur. He is currently working as an assistant professor in the Department of Biomedical Engineering. He has more than 10 years of teaching and research experience. He has published more than 35 papers in various international journals and conferences. He has guided six MTech projects in the area of medical imaging. He is life member of IE, IETE, and CSI India. He has received IEI Young Engineer Award 2012 and Chhattisgarh Young Scientist Award-2010.