Abstract
The appropriate treatment of cancers critically depends on the precise identification of the tumor types. The Magnetic Resonance Imaging (MRI) provides a non-invasive approach for detecting the tumors and classifying their types. Computer-aided diagnosis (CAD) systems using image analysis and machine learning algorithms assist radiologists in making error-free decisions. This paper presents an integrated approach for the segmentation and classification of three types of brain tumors (i.e. meningioma, glioma, and pituitary tumor) in real MRI images. First, the images quality is enhanced by removing the noises using wiener and median filters. Then, the support vector machine (SVM) classifier is performed to segment the MR image. Following this, 42 features are extracted, among which eleven most practical features are chosen by the Differential Evolution (DE). Using the selected features, tumor slices are categorized using the k-nearest neighbors (KNN), the weighted kernel width SVM (WSVM) and the histogram intersection kernel SVM (HIK-SVM) classifiers. Afterwards, these classifiers are combined using a multi-objective differential evolution (MODE)-based ensemble technique to attain the classification accuracy of 92.46%, evaluated using the five-fold cross validation method. The results of the proposed approach are also compared with the experienced radiologist ground truth which shows that the proposed MODE-based ensemble technique is able to achieve high classification scores, measured also by sensitivity and specificity indices.
Additional information
Notes on contributors
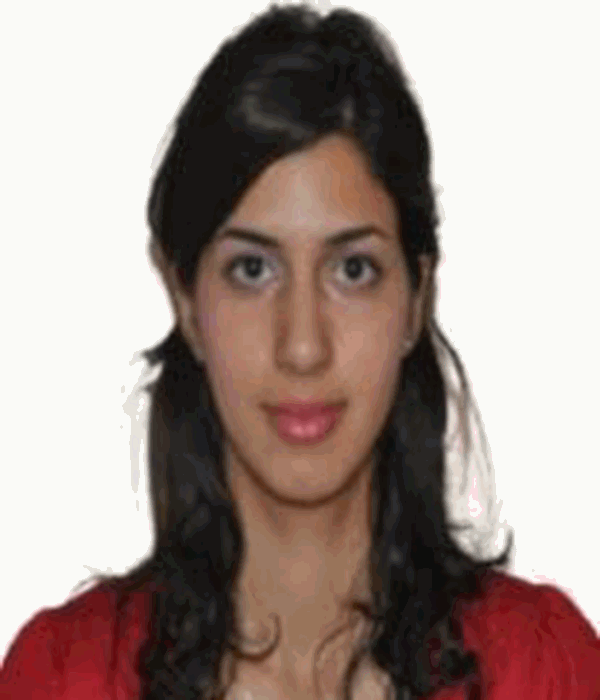
Kimia Rezaei
Kimia Rezaei received her BSc from Islamic Azad University, Fasa Branch, Fasa, Iran, and the MSc in telecommunications engineering from Islamic Azad University, Shiraz Branch, Shiraz, Iran. She is currently working as a telecommunications engineer in Sahand Telecommunication Company in Iran. Her research interests are pattern recognition and image processing for practical applications. Email: [email protected]
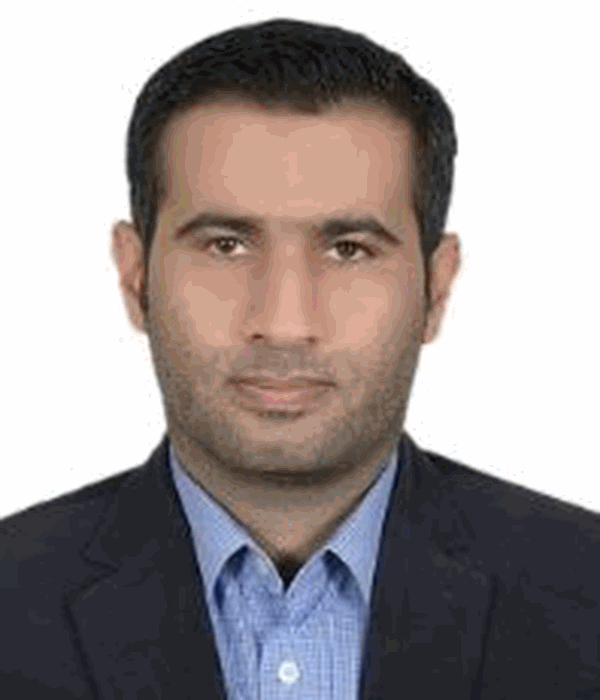
Hamed Agahi
Hamed Agahi received BSc, MSc and PhD degrees in electrical engineering from University of Shiraz, Amirkabir University of Technology and University of Tehran, Iran, in 2005, 2008 and 2013, respectively. From 2009, he was with the Islamic Azad University, Shiraz Branch, Shiraz, Iran. His research interests include pattern recognition, image and signal processing, and fault detection and diagnosis applications. Corresponding author. Email: [email protected]
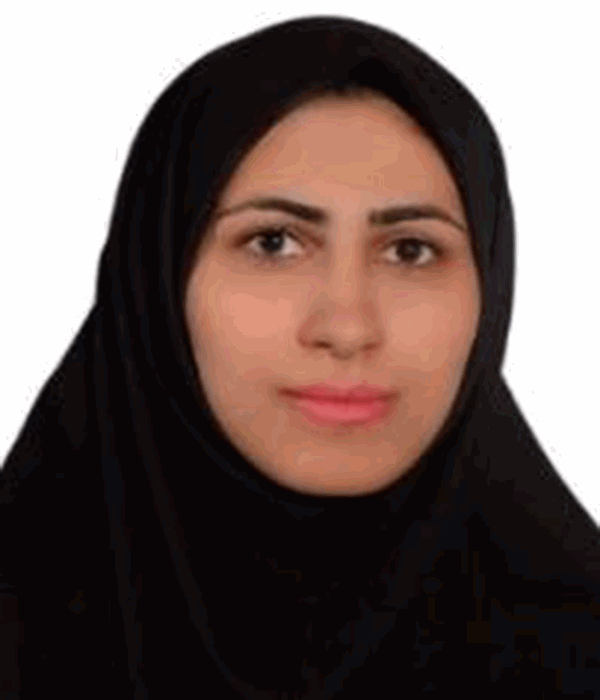
Azar Mahmoodzadeh
Azar Mahmoodzadeh received BSc, MSc and PhD degrees in electrical engineering from University of Shiraz, University of Shahed and University of Yazd, Iran, in 2005, 2008 and 2013, respectively. From 2009, she was with the Islamic Azad University, Shiraz Branch, Shiraz, Iran. Her research interests include pattern recognition and image and signal processing. Email: [email protected]