Abstract
Considering the escalating rates of exhaustion of non-renewable energy resources, coupled with the harmful environmental side effects of harnessing them (e.g. damage to public health via air pollution), the need for a near-complete transition to renewable energy production seems inevitable. In recent times, renewable energy production has seen a strong support from investors, governmental initiatives, and industries across the world. Globally installed wind power capacity has seen an increase of 345.24% over the past decade. This increase brings along a need for robust power production management systems having a potential for predicting wind turbine power outputs primarily based on real-time input wind velocities. We propose and compare five optimized robust regression models for forecasting the wind power generated through turbines based on wind velocity vector components, out of which the Extreme Gradient Boosting regression model provided the best results. The forecasted output of our model can be compared with a city’s daily average threshold power requirement in order to make informed decisions about either shutting down an appropriate number of turbines to avoid excessive power production and wastage, or to compensate forecasted shortcomings in production on less windy days via alternative energy generation methodologies.
Acknowledgements
We are grateful to T. Hong et al. [Citation17] who released the dataset that served as the foundational basis for this research.
Correction Statement
This article was originally published with errors, which have now been corrected in the online version. Please see Correction (http://dx.doi.org/10.1080/03772063.2021.1879417)
Additional information
Notes on contributors
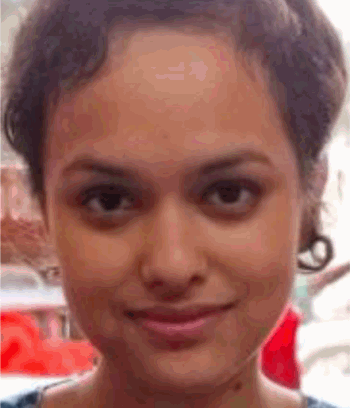
Rachna Pathak
Rachna Pathak is a Bachelor of Technology graduate majored in instrumentation and control engineering, from Guru Gobind Singh Indraprastha University, Delhi, India. Her work is related to the field of artificial intelligence, specifically machine learning and deep learning algorithms and their applications to the fields of computer vision, robotics and power systems. Email: [email protected]
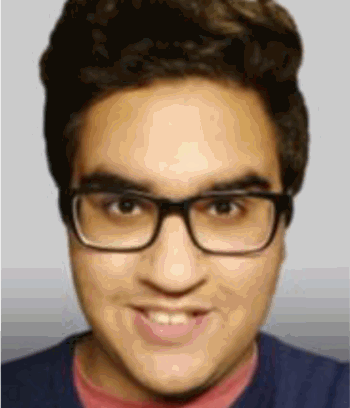
Arnav Wadhwa
Arnav Wadhwa is a Bachelor of Technology graduate majored in information technology, from Guru Gobind Singh Indraprastha University, Delhi, India. His research interests revolve around in-depth analysis of real-world data, and developing machine learning and deep learning models and algorithms to build AI integrated solutions to problems having societal benefit. Email: [email protected]
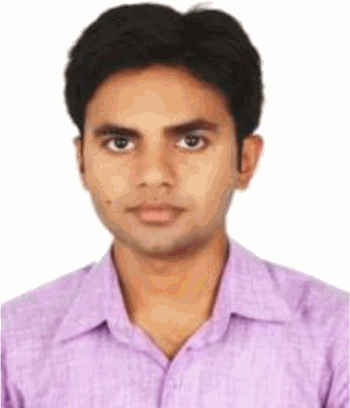
Poras Khetarpal
Poras Khetarpal is currently working as an assistant professor in Instrumentation and Control Engineering Department at Bharati Vidyapeeth’s College of Engineering (affiliated to Guru Gobind Singh Indraprastha University) New Delhi, India. His current research area focuses on renewable energy systems. Email: [email protected]
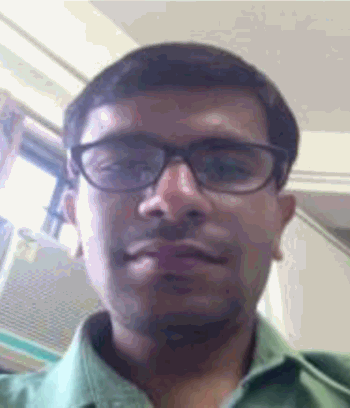
Neeraj Kumar
Neeraj Kumar is currently working as an assistant professor in Electrical and Electronics Engineering Department at Bharati Vidyapeeth’s College of Engineering (affiliated to Guru Gobind Singh Indraprastha University) New Delhi, India. His current research focuses on artificial intelligence applications to power systems.