Abstract
In recent years, Deep Learning (DL) models, including Convolutional Neural Networks (CNNs), Recurrent Neural Networks (RNNs), and Long Short-Term Memory (LSTM), have been widely used for Human Activities Recognition (HAR). They have achieved considerable performance improvements over classical Machine Learning (ML) approaches due to their excellent feature representation capabilities. Simple human activities are performed in sequential order with no overlaps or concurrent actions. The paucity of labeled training activity samples, high computational cost, and system resource requirements of deep learning architectures compared to lightweight models are some of the research challenges confronting the HAR community. We framed the lightweight DL-based CNNs, RNNs, and LSTM model for HAR to tackle these research challenges. The RNNs and LSTM have single layers with softmax activation functions, whereas the one-dimensional CNNs have two convolutional and single max-pooling layers. To evaluate the performance of our models, we used the publicly available benchmark WISDM experimental dataset, which includes the reading of six activities (walking, jogging, upstairs, downstairs, sitting, and standing) performed by 36 participants using a single accelerometer sensor. The experimental result illustrates the efficacy of our models in activity recognition, demonstrating that they attain higher accuracy while being computationally efficient.
ACKNOWLEDGEMENT
Prabhat Kumar sincerely acknowledges the University Grants Commission (UGC), New Delhi, India, for awarding the Non-Net Fellowship Scheme [FILE NO.: R/Dev/IX-Sch.(UGC Res. Sch.) 2020-21/13674, Dated: 01-10-2019].
Disclosure statement
No potential conflict of interest was reported by the author(s).
Additional information
Notes on contributors
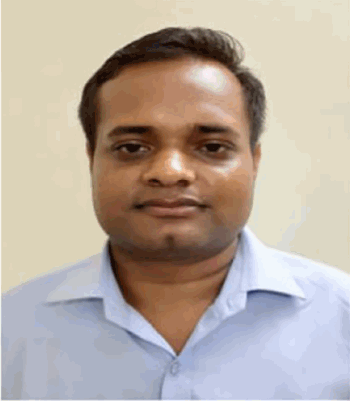
Prabhat Kumar
Prabhat Kumar is pursuing his PhD in Department of Computer Science, Institute of Science, Banaras Hindu University, Varanasi, India. He did his MCA from Dr Hari Singh Gour Vishwavidyalaya (A Central University), Sagar, Madhya Pradesh, India, in 2017. His research areas are machine learning, deep learning, wireless sensor networks, and feature engineering.
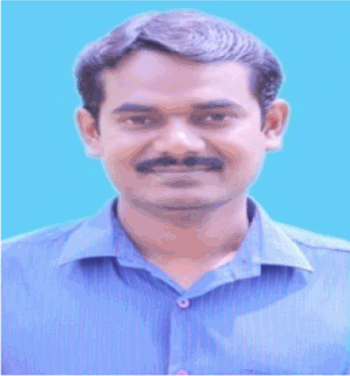
S. Suresh
S Suresh is an assistant professor in the Department of Computer Science, Institute of Science, Banaras Hindu University, Varanasi, India. He obtained PhD from National Institute of Technology, Tiruchirappalli, India. His areas of interest include theoretical computer science, machine learning and IoT, big data analytics, distributed and cloud computing. He has served as session chair of program committee in several conferences and workshops. Email: [email protected]