Abstract
In this work, we propose a DnCNN-based method to enhance the contrast of images. Fundus images are widely used in clinical practice for detecting retinal dark spots. These spots trouble ophthalmologists to distinguish and decipher diseases for diabetic retinopathy. This work aims is to describe a fundus picture noise removal with enhancement technique. Our simulation results demonstrate that our DnCNN-based method outperforms other contrast enhancement methods.
This paper presents a denoising Convolutional Neutral Network (DnCNN)-based technique to enhance images for diabetic retinopathy. The contrast of images can be adaptively improved. Denoising convolutional neural networks (DnCNNs) show progress in image denoising architecture, learning algorithm, and regularization method. DnCNN removes the latent clean image in the hidden layers implicitly. Our proposed (DnCNN) method, compared with the various histogram (Equalization (HE), Adaptive Histogram Equalization (ADHE), Contrast Limited Adaptive Histogram Equalization (CLAHE), and Exposure based Sub-Image Histogram Equalization (ESIHE)) pre-processes fundus images for identifying diabetic retinopathy.
The fundus images were collected from the DRIVE database. The Denoising Convolutional Neural Network strategy is analyzed with the execution parameter measurements of PSNR and SSIM. According to the simulation results, the DnCNN method has a higher visual quality than the previous method. In addition, the proposed method’s average PSNR values 25.4303 and 11 dB are higher than those of the existing methods. However, the DnCNN method has a slightly ∼0.2 lower SSIM value of 0.3584 than the previous methods. The decrease in SSIM value indicates a loss of detailed information.
DISCLOSURE STATEMENT
No potential conflict of interest was reported by the author(s).
Additional information
Notes on contributors

R. Alaguselvi
R Alaguselvi received the BE (Electronics and Communication Engineering) from Anna University, Chennai, India, in 2016 and the ME (Applied Electronics) from Anna University, Chennai, India, in 2018. She is currently working toward the PhD (Electronics and Communication Engineering) degree, Kalasalingam Academy of Research and Education, Virudhunagar, India. Her major interests are Networking and image processing. Email: [email protected]
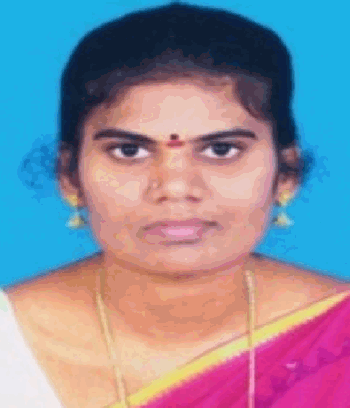
Kalpana Murugan
Kalpana Murugan holds a PhD degree from Anna University, Chennai in 2011. She received BE degree in electronics and communication engineering from P S G College of Technology, under Bharathiar University in 1995. She completed ME in communication systems under Anna University, Chennai in 2006. She has 26 years of teaching experience. She is currently working as a professor and head in Electronics and Communication Engineering at Kalasalingam Academy of Research and Education. She published more than 70 articles in various international journals and conferences. Her research interests include wireless sensor networks, congestion control, mobile ad hoc networks, image processing. She has served as a chair, Organizing Committee Member in numerous international conferences/workshops/ Seminars. She is a reviewer for various renowned international journals that includes IEEE, IETE, IET, Elsevier Hindawi and Springer. She is also serving as an editor of renowned journal. She is a life member of ISTE and IETE.