Abstract
An episode rule mining to extract useful and important patterns or episodes from large event sequences represents the temporal implication of associating the antecedent and consequent episodes. The existing technique for mining precise-positioning episode rules from event sequences, mines serial episodes resulting in enormous memory consumption. To resolve this issue, the proposed work ensures the generation of fixed-gap episodes and parameter settings through the use of Particle Swarm Optimization mechanism. Fixed-gap episodes are generated using Natural Exponent Inertia Weight-based Particle Swarm Optimization algorithm. In this paper, a new technique called Mining Serial Episode Rules (MSER) is proposed, which utilizes the correlation between episodes and the generation of parameter selection where the occurrence time of an event is specified in the consequent. Further, a trie-based data structure to mine MSER along with a pruning technique is incorporated in the proposed methodology to improve the performance. The efficiency of the proposed algorithm MSER is evaluated on three benchmark data sets Retail, Kosarak, and MSNBC where the experimental results outperform the existing methods with respect to memory usage and execution time.
ACKNOWLEDGEMENT
This work is supported by the Centre for Research, Anna University, Chennai (No. CFR/ACRF/2016/23) by granting Anna Centenary Research Fellowship (ACRF 2016–2018).
Disclosure statement
No potential conflict of interest was reported by the author(s).
Additional information
Notes on contributors
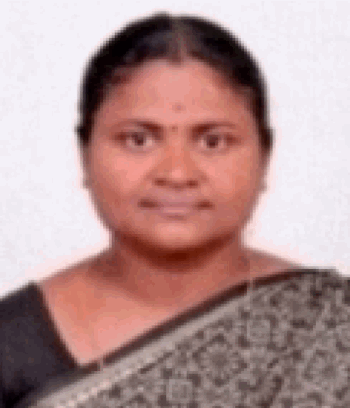
K. Poongodi
K Poongodi is a PhD student in the Department of Information Technology, Anna University, MIT Campus, Chennai. She received the MCA degree with distinction from Bharathiar University, Coimbatore, in 2003. She was awarded an ME degree in computer science and engineering with distinction and received 39th rank from Anna University, Chennai, in 2014. She has nine years of teaching experience and received the best teacher award in the year 2012 by Akshaya Institute of Management Studies, Coimbatore. Her research interests include data mining, big data, database management system, and computer networks.
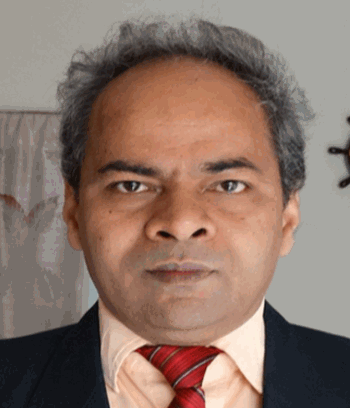
Dhananjay Kumar
Dhananjay Kumar received his bachelor degree in electronics and telecommunication engineering from the Institution of Engineers (India), Calcutta, in the year 1997. He was awarded a Master of Engineering (ME) degree in industrial electronics engineering by the Maharaja Sayajirao University of Baroda in the year 1999. He also holds a Master of Technology (MTech) degree in communication engineering from Pondicherry University through Pondicherry Engineering College, Pondicherry, in the year 2001. A Doctor of Philosophy (PhD) degree was awarded to him by Anna University, Chennai, in the year 2009 for his research work on high-quality multimedia support in next-generation multi-carrier wireless mobile networks. Currently, he is working as a professor and head in the Department of Information Technology, Anna University, MIT Campus, Chennai. Kumar and his team are working on steaming media data analysis and prediction. Email: [email protected]